1 Introduction
The Orinoco River is an important water way that will be of great significance for the development of the Orinoco Oil Belt (the region with the major proven oil reserves on the planet). Nowadays, there is a critical need to strengthen our understanding of the hydro-sedimentary behavior and of the fluvial morphology in the lower stretch of the Orinoco River to support the dredging projects and to ensure the navigability towards the Atlantic Ocean.
The Orinoco River is the third largest river of the world, after the Amazon and Congo in terms of discharge of water to the oceans with 37,600 m3·s−1 (Laraque et al., 2013). The specific discharge of the Orinoco River to the ocean is around 37.6 l·s−1·km2, among the highest ones for large rivers with catchment larger than one million square kilometers or more. This value corresponds to more than three times the value of specific discharge (11.6 l·s−1·km2) of the Congo River (Laraque et al., 2013), and even has a value higher in order of magnitude (34.6 l·s−1·km2) than that reported for the Amazon River (Callède et al., 2010).
For large catchments such as the Orinoco River basin, it is difficult and costly to develop in situ studies related to sediment flux. However, in recent decades, new technologies based on remote sensing have been increasingly used to obtain accurate spatiotemporal information of water quality. It has been demonstrated that suspended sediments increase the reflected light from the water surface in the visible (VIS) and near-infrared (NIR) part of the electromagnetic spectrum (Aranuvachapun and LeBlond, 1981; Doxaran et al., 2002; Froidefond et al., 1991; Islam et al., 2001; Liew et al., 2003; Lobo et al., 2015; Ma and Dai, 2005; Mertes et al., 1993; Ritchie and Cooper, 1988; Ritchie et al., 1987, 1990; Shi et al., 2015; Wang et al., 2009; Watanabe et al., 2015; Wu et al., 2015; Zhou et al., 2006), making the satellite images an essential tool to study the variability of the SSC in fluvial systems, in particular in large rivers.
Among the investigations of imaging and field spectroscopy in large river basins, where relations were established between in situ SSC and reflectance for inland waters (turbid waters), those by Espinoza Villar et al., 2013 and Martinez et al., 2015 were notable for their broad, regional scale and the fact that they characterize with great precision the optical properties of inland waters. The analysis of field radiometric measurements of the remote sensing reflectance and of time series of satellites images (MODIS satellite imagery) allows us to conclude that there exists a relationship with SSC that was sufficiently robust to be used for the accurate monitoring of surface-suspended sediment discharge in the largest river of the world (Martinez et al., 2015). Park and Latrubesse, 2014, carried out another important reference investigation, where they concluded that this type of model is a tool that can be applied to characterize the suspended sediment distribution patterns at a suitable resolution, not just in the Amazon River, but even in other complex and dynamic branches of other large rivers.
Since 2013, a new satellite of the Landsat series is available, called Landsat-8 OLI, which was designed with similar characteristics to Landsat-5 and Landsat-7 ETM+ sensors in terms of spatial resolution. This feature of the OLI images offers a great advantage over previous global ocean color imagers, such as MODIS satellite imagery, providing a much higher spatial resolution that allows resolving the fine-scale distribution of suspended sediments and bio-optical water constituents in coastal and estuarine environments (Concha and Schott, 2014; Franz et al., 2015). Additionally, OLI has the required spectral bands and sufficient radiometric performance to support the standard atmospheric correction approach used for NASA's global ocean color missions, including determination and removal of aerosol contributions based on realistic aerosol models (Franz et al., 2015).
In this paper, we analyze the variability of remote sensing reflectance as a function of the concentration of suspended sediment on the water surface of the Orinoco River at Ciudad Bolivar station. This station is marked by a “funnel effect”, which homogenizes the SSC (Laraque et al., 2013), facilitating the analysis with remote sensing technologies. There exists a significant body of work that have developed statistical relationships (algorithms) between SSC and the radiance or reflectance for a specific date or site. This research study intends to build an inversion algorithm to estimate suspended sediments in the Orinoco River, without limiting itself to any particular region or to any specific time interval.
Based on the above considerations, an assessment of three types of atmospheric correction models to correct OLI scenes was developed. The three methods were: Dark Object Subtraction (Chavez, 1996), Fast Line-of-sight Atmospheric Analysis of Hypercubes (FLAASH) (Anderson et al., 2002) and the new product provisional Landsat-8 (L8SR) “Surface Reflectance” (USGS, 2015), recently developed for the U.S. Geological Survey (USGS). These algorithms were evaluated to establish the best model to mitigate the atmospheric contribution over the Landsat-8 scenes.
This study seeks to reveal and quantify the seasonal changes in the SSC pattern caused by environmental factors and geomorphologic controls, and attempts to clarify their relationships. We used remote sensing data from Landsat-8 satellite over a 2.5-year period (2013–2015) with the following three objectives:
- • to evaluate three methods of atmospheric correction to establish the best model to mitigate the atmospheric contribution over the Landsat-8 scenes;
- • to develop a model, based on the Landsat-8 OLI images, that addresses seasonal differences and atmospheric corrections to estimate SSC in the lower Orinoco River with extreme variation;
- • to provide spatial information of SSC through remote sensing technologies and understand its relationship to environmental factors and geomorphologic controls over the main channel characterized by an alternation of contraction and expansion zones.
2 Overview of the Orinoco basin
The Orinoco basin is located in the Northern Hemisphere, specifically in the northern region of South America, between 2° and 10° N and 75° and 61° W, where 70% of its basin area lies in Venezuela and 30% in Colombia (Fig. 1). The basin is divided in three major geographic zones: (i) the Andes and Caribbean Coastal Ranges, where most of the suspended sediment is generated; (ii) the lowlands and floodplain, locally named “Llanos”, which are crossed by all major tributaries coming from the Andes, and (iii) the Precambrian Guiana Shield, which is drained essentially by black waters with very low suspended sediment content (Depetris and Paolini, 1991; Lewis and Saunders, 1984, 1989; López and Perez-Hernandez, 1999; Paolini et al., 1987; Warne et al., 2002).
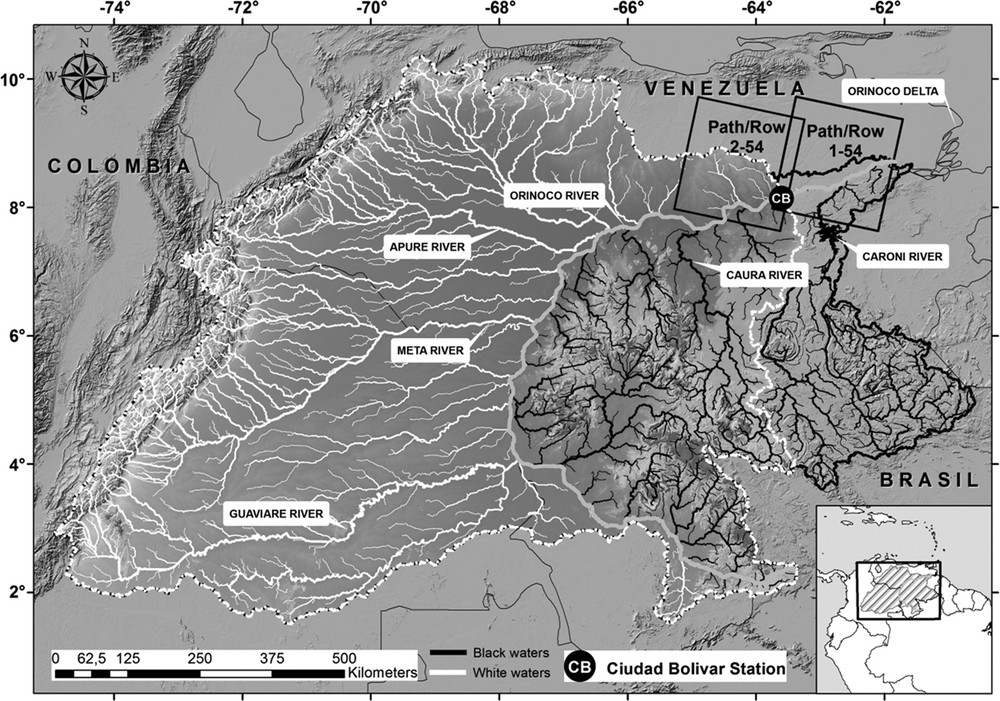
Catchment area of the Orinoco River, representing the principal tributaries and spatial coverage of the Landsat-8 OLI scenes in the lowest part of the basin (path/row: 1-54 and 2-54). The gauging station Ciudad Bolivar (CB) drains 89% of the catchment area.
The study site, which is the focus of the present research, is located in the main gauging station at Ciudad Bolivar. The mean annual discharge is around 33,000 m3·s−1 (average during the 1926–2015 period in this study). This station drains 89% of the total catchment area of the Orinoco River, which corresponds to 819,252 km2 according to the watershed analysis combined with GIS techniques on the Digital Elevation Model–SRTM (Farr et al., 2007).
3 Methods
3.1 Available data
3.1.1 Water discharge and suspended-sediment concentration data
Daily water stage records and discharges are available at Ciudad Bolivar gauging station (Fig. 2) since 1926, which has been registered by the Venezuelan Hydrological National Service (INAMEH) and by the Central University of Venezuela (UCV), using traditional gauging techniques with current meters before 2010 and Acoustic Doppler Current Profiler (ADCP) thereafter. The hydrological cycle of the lower Orinoco at Ciudad Bolivar is characterized by a unimodal regime with high flows between August and September, and low flows between February and March. The sedimentological cycle is bimodal, as displayed in Fig. 2, marked by two SSC peaks respectively, before and after the maximum water discharge peak. This singularity was explained and commented on by Laraque et al. (2013), where the authors observed that, during the hydrological cycle of the Orinoco River, the presence of hysteresis between discharge and SSC and the two peaks of SSC (Fig. 2) invalidates the use of relations (SSC = f(Q)) to calculate the sediment discharge.
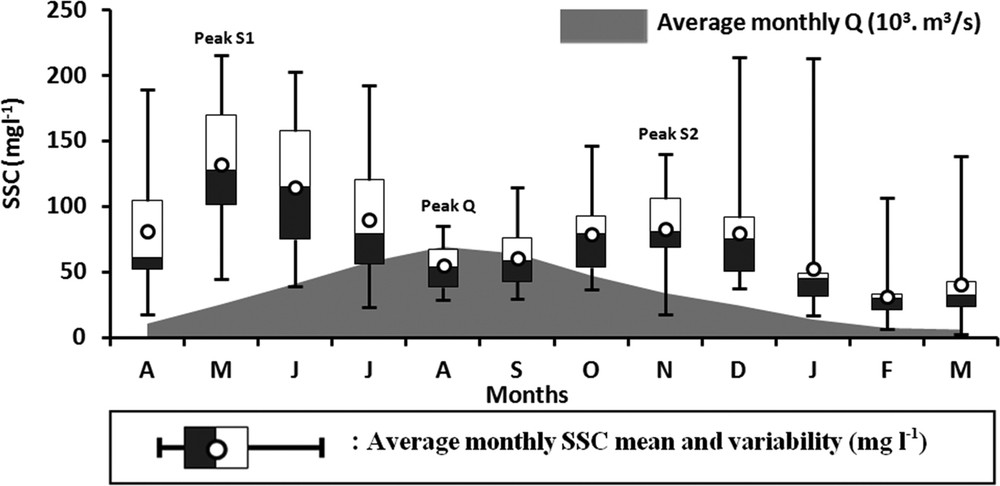
Mean monthly discharge (gray area) and average monthly suspended-sediment concentration (white dots) between 2007 and 2015 at the Ciudad Bolivar station. The box plots (white/black) indicate the variability of SSC derived from samples collected by the HYBAM observation service.
The HYBAM observation service (http://www.so-hybam.org/index.php/eng/Data) collected 266 surface water samples each 10 days for SSC determination between January 2007 and December 2015 at the Ciudad Bolivar gauging station (8° 9′1.79″ N, 63° 32′25.26″ W, 8 m a.s.l.) from which 33 were acquired after June 2013. It is on this date that Landsat-8 satellite began operating.
A second set of 15 SSC data (in situ) were used to test the SSC retrieval model. The data retrieved from the HYBAM sampling at the Ciudad Bolivar station coincides with the acquisition of OLI cloud-free images during the year 2016. The data is independent of that used to build the SSC retrieval model.
3.1.2 Landsat-8 OLI data
Landsat-8 (L-8) was launched on February 11, 2013 and normal operations commenced on May 30, 2013. This satellite has a ground track repeat cycle of 16 days, which crosses the equator at 10:00 a.m. The Operational Land Imager (OLI) on L-8 is a nine-band push broom scanner with a swath width of 185 km and eight channels at 30 m, and one panchromatic channel at 15 m spatial resolution. Compared to the Thematic Mapper (L4-5/TM) and the Enhanced Thematic Mapper Plus (L-7/ETM+) previous Landsat missions, L-8/OLI offers higher signal-to-noise ratios (SNR)—mainly because of longer integration times on the push broom scanner—and an improved quantization (12 bits instead of 8 bits for radiometric digitization). This new sensor shows great potential, in terms of radiometric and spatial accuracy for monitoring turbid waters (Case 2) (Gerace et al., 2013).
The Ciudad Bolivar station is located at the overlap of Landsat-8 frames (path/row: 1/54–2/54, with an overlapping of approximately ∼ 22 km). This situation results in an increased revisit frequency of 3–4 images per month instead of 1 or 2 per month (Fig. 1). After a preliminary analysis of the 81 images without cloud cover, 27 scenes were selected. We examined the correspondence of 27 in situ SSC (HYBAM dataset) with their corresponding satellite images to derive empirical relationships between SSC (mg·l−1) and reflectance in both path/row at the Ciudad Bolivar station.
The foundation of remote sensing approach for SSC estimation is that the amount of sediment in water directly affects the reflectance of solar radiation in the VISIBLE and NIR portions of the spectrum. We decided to evaluate only the VIS and NIR bands of Landsat-8 OLI (Band 1–coastal/aerosol: 433–453 nm, Band 2–blue: 450–515 nm, Band 3–green: 525–600 nm, Band 4–red: 630–680 nm and Band 5–NIR: 845–885 nm). Shortwave infrared (SWIR) bands were excluded from this analysis.
In reference to the validation section, 15 new OLI images from January 19, 2016 to December 10, 2016 were used to extract surface reflectance values at the Ciudad Bolivar station. Subsequently, the SSC retrieval model (Eq. (1)) was applied to predict the 15 SSC values. These measures were used to assess the algorithm's performance and product uncertainties at the pixel level.
3.2 Spatial-temporal representativeness of SSC sampling on the study section
A total of 33 samples from June 2013 to December 2015 (HYBAM dataset) were collected monthly at the river water surface in the middle of the channel at the Ciudad Bolivar station. Out of 33 samples, only 27 were realized during Landsat-8 acquisition day or very close to. The 500-ml water samples were transported to the laboratory and filtered using 0.45-mm cellulose acetate filters to assess the SSC. After filtration, the filters are dried for 24 h at 60 °C and weighed. SSC is computed as the difference between the weights of the filter after and before filtration divided by the volume of water collected (Martinez et al., 2015).
Ten-river sediment discharge assessments were realized from 2007 to 2010 using the sampling protocol (Filizola and Guyot, 2004), which uses a point sampler at various depths in selected vertical profiles within the sampling transect. Based on a larger dataset of sediment discharge measurements acquired at the same station, Laraque et al. (2013) demonstrate that one sample taken close to the water's surface in the middle of the transect is representative of the average SSC across the whole of the river's reach. According to Laraque et al. (2013), this particular situation was also reported by other authors (López and Perez-Hernandez, 1999; Meade, 1994; Mora, 2011), and can be explained by the fluvial geomorphology affecting the course of the river upstream (presence of closed meanders, a tectonic threshold, fluvial islands, etc.), which generates rapid and strong vortexes in the river's reach, dominated by a “funnel effect”. Indeed, the width of the channel changes rapidly from 8 km to 1 km upstream in the Ciudad Bolivar “estrecho”, inducing a SSC homogenization in the water column, which is dominated by a rate fine distribution with a particle size distribution D50 = 8 to 10 μm (Laraque et al., 2014).
3.3 Processing of Landsat-8 OLI data
3.3.1 Atmospheric correction evaluation
In this study, we compared three types of atmospheric correction models aiming at producing surface reflectance from radiance L-8 OLI images. For this assessment, we used two field spectroradiometers (ASD Field Spec 3 & 4 model) to collect remote sensing reflectance data on the river's surface in May, August, and November 2014 near the Ciudad Bolivar station. To limit the effects of the external factors, all radiometric measurements were acquired within the viewing geometry defined by Mobley (1999) under low-wind conditions (0–4 m·s−1), clear-sky conditions and sun zenith angle values ranging from 0 to 30°. These measurements correspond to different water stages, considering seasonal changes, lighting conditions, aerosol, acquisition geometry, among others. Additionally, these spectral profiles were measured simultaneously to the L-8 satellite acquisition, and were used as a reference to benchmark the performance of the three atmospheric correction models:
- • the first method is the Dark Object Subtraction, which removes the effects of atmospheric scattering from an image by subtracting the darkest pixel value that represents a background signature from each band. This value can be the band minimum, an average based upon a region of interest (ROI), or a fixed value (Chavez, 1988, 1996);
- • the second method is the Fast Line-of-sight Atmospheric Analysis of Spectral Hypercubes (FLAASH). The algorithm derives its first-principles physics-based calculations from the MODTRAN4 radiative transfer code (Anderson et al., 2002). The main objective of FLAASH is to eliminate the atmospheric effects caused by molecular and particulate scattering and absorption from the “radiance-at-detector” measurements to retrieve “reflectance-at-surface” values (Felde et al., 2003);
- • the third method is the L8SR (Landsat 8 Surface Reflectance) algorithm, which is distributed by the USGS (USGS, 2015).
The comparison of these three methods is illustrated in Fig. 3; due to editorial constraints, only the analysis for August 2014 was displayed and described in the Supplementary Material.
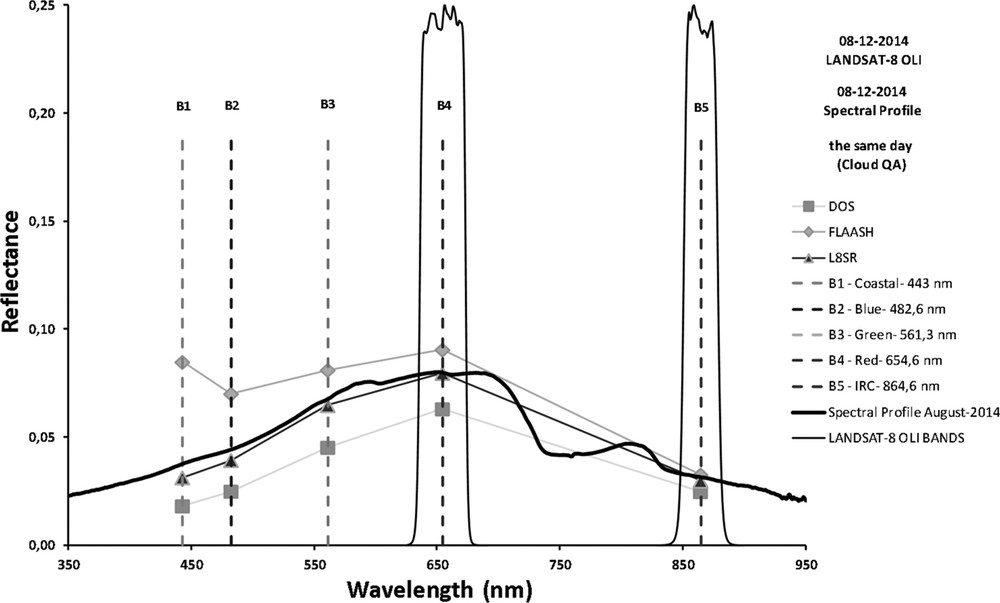
Spectral profile of water surface (black line) with the comparison of Landsat-8 image corrected by means of the three algorithms DOS (grey square), FLAASH (grey rhombus), and L8SR (grey triangle).
For evaluating the results obtained for each algorithm, a multispectral analysis has been developed. The spectra obtained in the L-8 scenes (for each method) are compared with the in situ spectral profiles that were acquired at the same time as the L-8 scenes. For this comparison, only the first five bands of the images L-8 were processed (Fig. 3). This was due to the fact that the bands from VIS and NIR are the bands most sensitive to SSC changes in water surface. The scatter plots have been generated between each method, as well as the calculation of the Root Mean Square Error (RMSE), which is taken as an indicator of the accuracy, the correlation (R2) and the mean difference between the pixels of the images processed with the values of spectral profiles for each spectral range (simulation of the five spectral bands of L-8).
3.3.2 Flowchart and mask applying
Landsat-8 (OLI) images acquired over almost three annual hydro-sedimentological cycles were used to develop a robust regression model that was exploited to assess the superficial SSC in the downstream part of the Orinoco River, making it possible to infer and interpret suspended sediment distribution patterns. Detailed procedures for deriving SSC from satellite data (L-8) are described in Fig. 4. Initially, the images were requested from the website: http://www.earthexplorer.usgs.gov/. Because of comparisons with methods of atmospheric correction applied to L-8 images, it was concluded that the product L8SR represented the best choice. This is because the spectral signature of water in the image is the most similar to the spectral response of the water that was measured on the river.
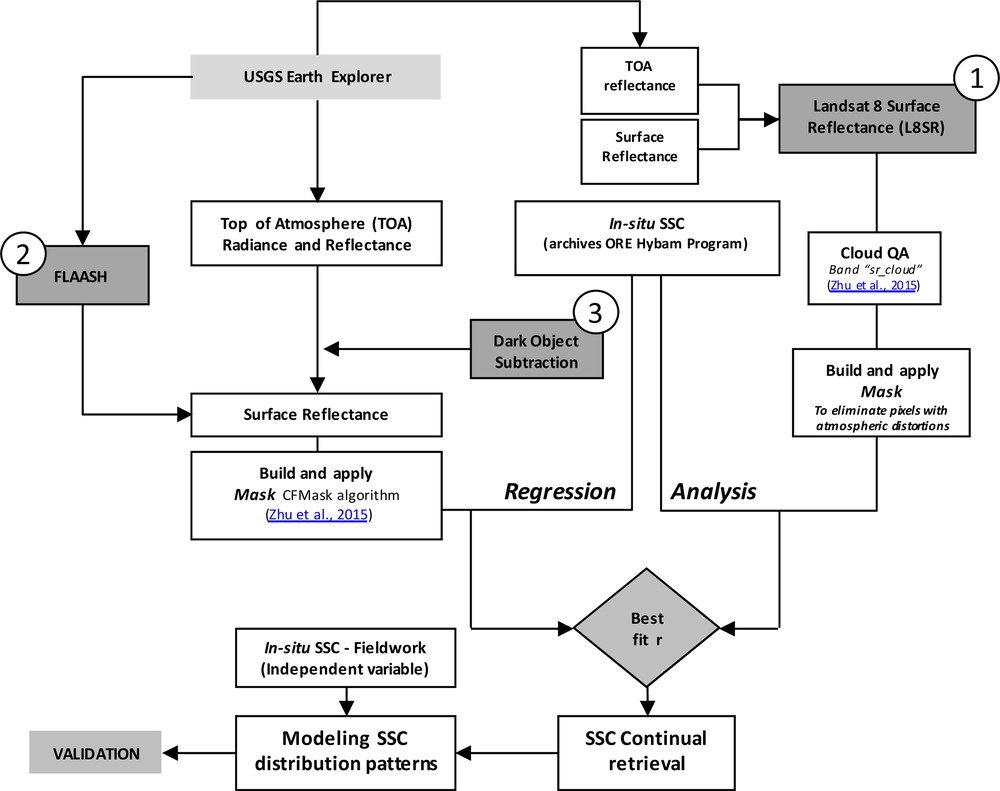
Flowchart that describes the methodology and the processing of Landsat-8 images for retrieval of SSC.
Otherwise, if it is not possible to have access to the product L8SR (1), it becomes necessary to implement the module FLAASH (2), which has excellent results for the conversion from “radiance-to-reflectance”. In the case of Dark Object Subtraction (3), it does not need additional information on the atmospheric transmittance to be implemented.
For obtaining better results on the models of linear regression to estimate SSC, the construction and application of a mask using the information of the product Quality Assurance (USGS, 2015) is necessary. This facilitates the selection of pixels in reflectance that are not affected by atmospheric distortions (clouds, cloud shadows, aerosols, among others). For the construction of the mask, the values in bits of the “sr_cloud” band are used. For this case, the values 16 and 32 bits were selected. This codification in binary numbers corresponds to the classes:
- • 16 bits → 00010000 = climatology-level aerosol content;
- • 32 bits → 00100000 = low aerosol content.
If the mask was not put into practice, this might lead to errors in the model. After the atmospheric correction and the application of the quality masks, we can identify which bands show the most significant regressions with in situ SSC values.
Once identified, the bands or band ratios with the more significant linear relationship with the SSC. This allows the construction of SSC distribution maps, which help to understand the variability of the load suspended of the Orinoco River.
The three Landsat-8 OLI images (WRS path1/row54) acquired on May 24, 2014, August 12, 2015, and February 20, 2015, covering: rising (A), peak (B) and low (C) water stages were used to map the SSC distribution. For each image, we used GIS techniques and supervised the classification using the Spectral Angle Mapper (SAM) method (Kruse et al., 1993) to extract water bodies from the river. We subsequently applied the regression relation algorithm to estimate SSC from reflectance for each pixel of the water bodies.
Finally, a validation of the results of estimated SSC was realized, using a comparison with observed SSC data from diverse sampling at Ciudad Bolivar during the year 2016.
4 Results and discussion
4.1 Atmospheric correction results
The accuracy of each atmospheric correction method was assessed using the reflectance's spectra collected during the August 2014 fieldwork as a reference (Table SM1). For this analysis, the field reflectance spectra were resampled using the spectral response functions available for the L-8 images and for the bands B1 → B5.
The RMSE for L8SR algorithm was 0.004 (sr−1), being reported as the most precise reflectance estimation with regards to the values obtained from in situ spectral signatures. On the other hand, reflectance that was processed with DOS algorithm showed a RMSE much smaller than the FLAASH algorithm, when evaluating the overall average of the five bands. However, if we analyze the results in detail band by band, it is possible to appreciate that the values for the bands Red and NIR reported in FLAASH model correspond much more closely to the true values calculated from the spectral profile (August 2014), in comparison with the DOS model.
We can conclude that the first option to correct the images atmospherically is the L8SR produced by USGS. However, it is important to consider that this algorithm does not work properly, in scenes with solar zenith angle greater that 72° (USGS, 2015). A second option is to use the FLAASH algorithm. There are, however, many factors that must be considered. For example, the first bands may be affected by an overestimation of the aerosols and other atmospheric contributions to the total reflectance. Finally, we can affirm that the accuracy of the DOS method is lower than the physical-based correction, but however is very useful when no atmospheric measurements are available.
4.2 SSC retrieval model
Fig. 5 shows the results of the variation of the surface reflectance as a function of SSC for 27 different dates for the red channel (left), near-infrared (NIR) channel (center), and for the NIR/red ratio (right). The best retrieval algorithm for SSC was found empirically by deriving a regression model for all possible bands and band ratio combinations, and then selecting the algorithm with the highest R2.
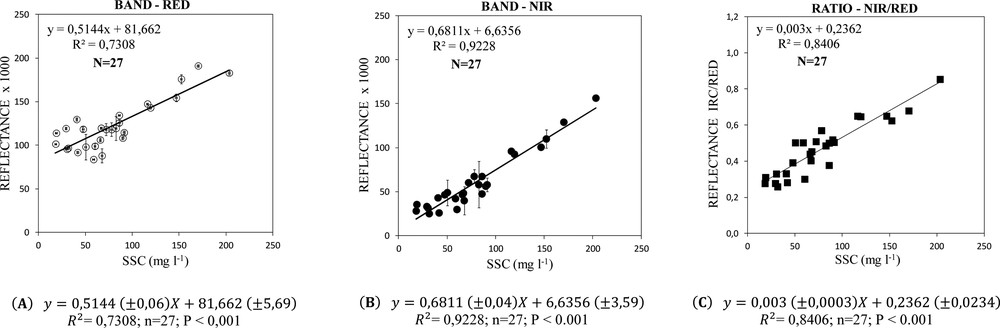
Regressions between water reflectance and SSC (using HYBAM dataset) at RED (A) and NIR (B) bands as well as the ratio NIR/RED (C) in the lower Orinoco at the Ciudad Bolivar station (the error bars indicate the standard deviation for each sample). We used the best-fit line to data with errors in both variables using a least-squares solution (York et al., 2004).
NIR reflectance showed the best performance and bootstrapping resampling technique that was used to calculate the retrieval model robustly. We made use of the bootstrapping procedure described by Carbonneau (2005) and Wang et al. (2009), called Jackknife procedure, which was introduced by Tukey (1958). Using this procedure, which consisted in creating new datasets by removing alternatively one sample from the initial dataset. For each new dataset, the slope and intercept of the linear retrieval model was calculated using least square regression and then used to estimate the SSC of the excluded sample (the estimated SSC value was obtained from the reflectance value). We returned the excluded sample to the original dataset, and repeated the process by excluding the next sample from the model. This process was repeated by excluding all the samples in the calibration table, one by one, resulting in a series of 27 slope values, intercept values, R2 values, and estimated SSC values.
The calibration curve (Fig. 6A) was then taken as the mean of slope and intercept with goodness-of-fit as given by mean of the R2 values, as follows:
(1) |
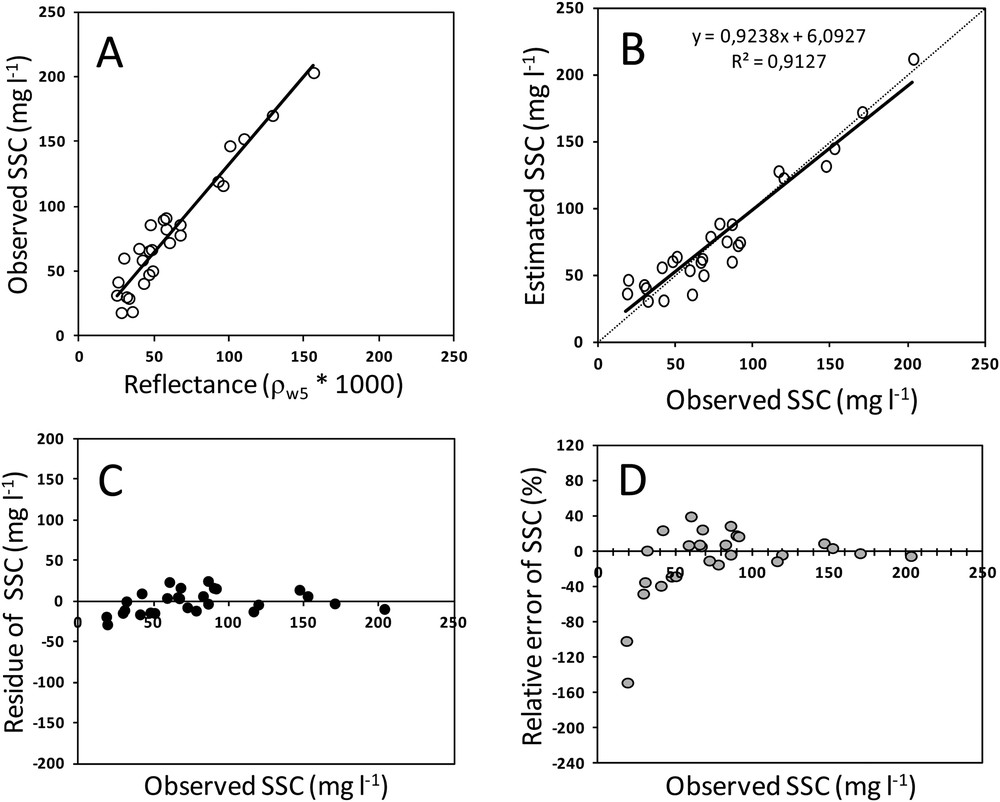
Details on the results of regression between SSC and water reflectance at Band 5 within the SSC range 18–203 mg·l−1 in the lower Orinoco River at Ciudad Bolivar. A. Raw data with the bootstrapped calibration curve. B. Validation results. C. Residue of SSC versus observed SSC. D. Relative error of SSC in percentage versus observed SSC. Note that “Residue of SSC (mg·l−1)” refers to (“Observed SSC (mg·l−1)” − “Estimated SSC (mg·l−1)”), and “Relative error of SSC (%)” refers to ((“Observed SSC (mg·l−1)” − “Estimated SSC (mg·l−1)”)/“Observed SSC (mg·l−1)”) × 100 (%). Masquer
Details on the results of regression between SSC and water reflectance at Band 5 within the SSC range 18–203 mg·l−1 in the lower Orinoco River at Ciudad Bolivar. A. Raw data with the bootstrapped calibration curve. B. Validation results. C. Residue of SSC Lire la suite
The mean absolute percentage error (MAPE) on slope and intercept was calculated by Eq. (2)
(2) |
where n is the number of samples, and yi and
Fig. 6B shows the predicted SSC versus observed SSC. The satellite-derived SSC estimates agree very well with field measurement, with a R2 of 0.91, and a bias of 7%. Retrieval mean absolute error is of 11.64 mg·l−1 with a mean relative error of −9.63% and a standard deviation of the error of 13.90 mg·l−1. Fig. 6C and B present the retrieval residual and error as a function of SSC concentration, showing that the model performs relatively uniformly over the whole SSC range of 18–210 mg·l−1. However, it can be noted that the model slightly overestimates SSC in the 18–40 mg·l−1 range.
Many authors have proposed the use of the NIR wavelength to assess SSC from different remote sensing data (Doxaran et al., 2002; Ma and Dai, 2005; Wang et al., 2009; Zhou et al., 2006) or Landsat-8 OLI (Zheng et al., 2015). Our results are consistent with the results of studies conducted under controlled experimental conditions, as detailed in the work of Wang et al. (2009). In particular, with reference to Chen et al. (1992), it was demonstrated that the relations between SSC and water reflectance were generally linear within a SSC range of 0–590 mg·l−1, and they indicate that the coefficient of determination (R2) between SSC and reflectance was 0.90. Other authors, such as Han and Rundquist (1994), agreed that the SSC–reflectance relation for wavelengths 700–900 (especially 726–868 nm) is linear over the range 50–600 mg·l−1, and pointed out that the relation is non-linear at higher SSC levels (600–1000 mg·l−1).
Fig. 7 compares the temporal behavior of SSC as calculated from OLI images and measured with the HYBAM observation service from 2013 to 2015 at the Ciudad Bolivar station. A good fit was found between the two datasets, illustrating the capability to monitor the temporal variability of SSC using satellite data, even in the rainy season where the sector of study is strongly affected by atmospheric artifacts (from May to November). This hydrologic station is overlapped by two orbits of the Landsat-8 satellite, which favors the continuity in the SSC monitoring due to the increase in the number of revisits on the station. An important aspect of the applicability of the technique is to estimate the SSC on the river, where in situ observations are absent, which can be observed between the months from March to April in 2014. In this period, SSC measurements were not collected in the river due to social and political demonstrations at national and regional levels. However, using the retrieval algorithm, Eq. (1), it was possible to estimate and interpret the suspended sediment concentration during these months, demonstrating the great potential of satellite time series in studying the spatiotemporal variability in sediment transport.
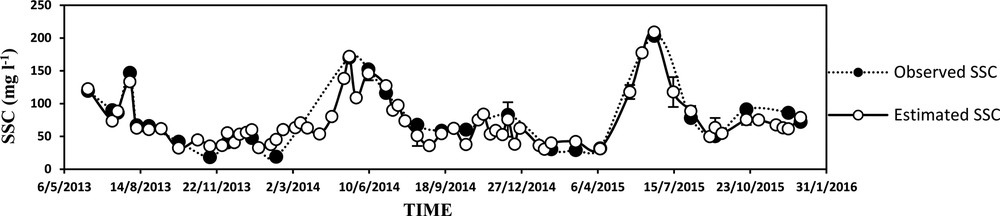
SSC time series retrieved from 65 L8SR images (gray dots) assessed monthly from 27 water samples (HYBAM) from 2013 to 2015 (black dots) at the Ciudad Bolivar station. The error bars indicate the standard deviation of the estimation.
4.3 Modeling suspended-sediment distribution patterns and validation
The SSC retrieval model was validated a second time using data collected specifically during 2016. For this comparison, 15 satellite-derived SSC estimates were compared with 15 field SCC samples collected at the same date or close to (< 4 days apart) (Table SM2). Fig. 8 shows that there was a significant correlation between the observed SSC data and the OLI-based SSC values, at a significance level of p < 0.001. The SSC retrieval model showed fine performance with a mean absolute percentage error of 19.8%, and RMSE of 12.8 mg·l−1. These results confirmed the robustness of the SSC retrieval model for a large dynamic range. However, it is noted that the SSC retrieval model still slightly overestimated the SSC values for the 18–40 mg·l−1 range.
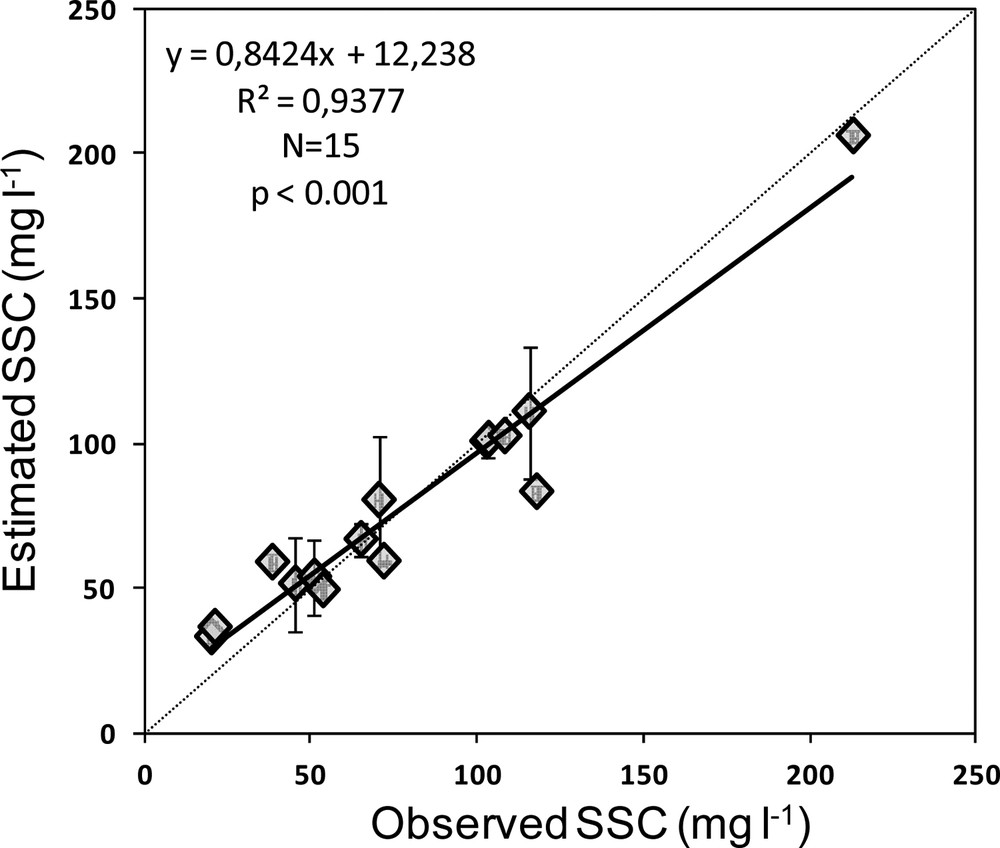
Second validation using the SSC-derived model during the year 2016. Fifteen estimated SSC values versus 15 in situ SCC values were compared to assess the algorithm's performance and product uncertainties at the pixel level.
Fig. 9 shows SSC distribution maps that were derived from satellite images to analyze the hydro-sedimentary processes at the river surface near the Ciudad Bolivar station for different periods of the hydrological cycle. The Orinoco River currently does not receive any major tributaries around Ciudad Bolivar. The Caura River, which is very small in proportion to the Orinoco, flows from the Guyana Shield about 190 km above Ciudad Bolivar. On the left bank, the Apure River, flowing from the Llanos, joins the Orinoco River approximately 360 km above Ciudad Bolivar, indicating that the mainstream at Ciudad Bolivar may show homogeneous suspended sediment lateral distribution at the river surface (Lewis and Saunders, 1984).
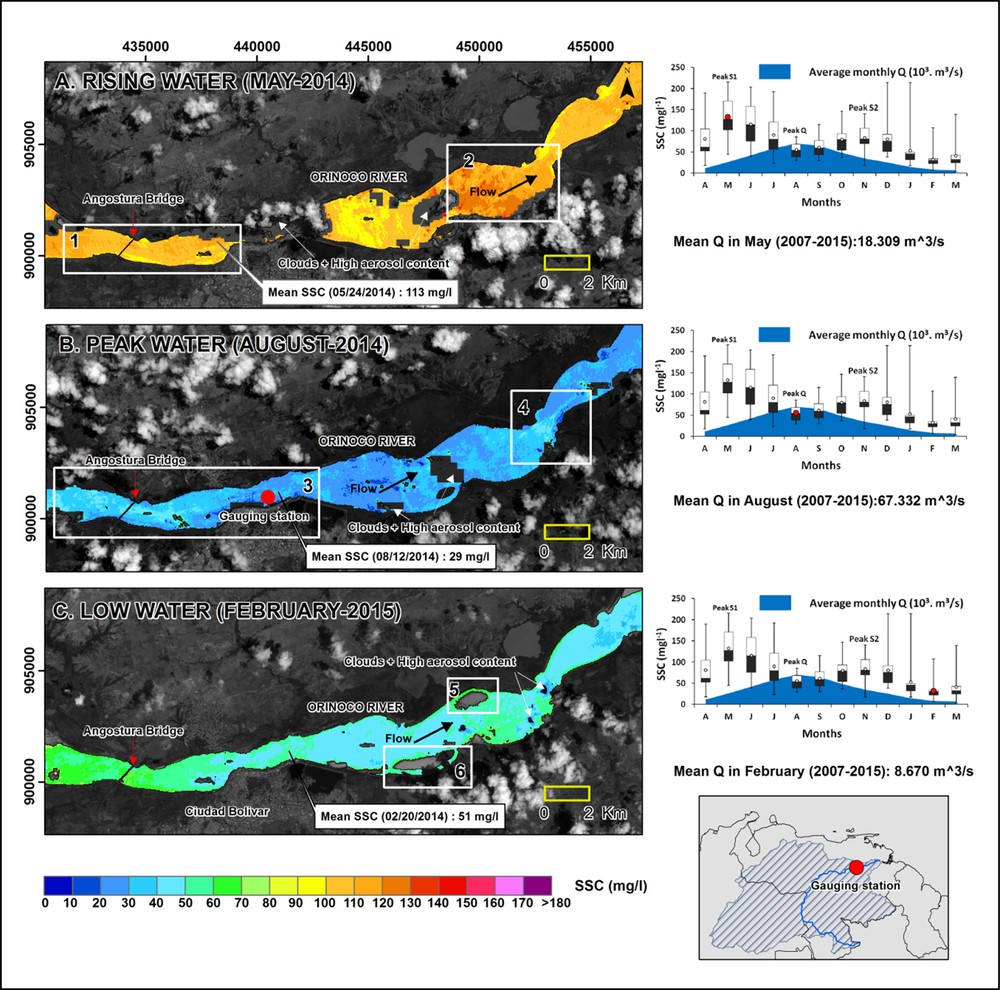
Comparison of surface sediment concentration maps during rising (A), peak (B) and low (C) waters stages in 2014 and at the beginning of the 2015 around Ciudad Bolivar (note that the same legend was used in the three maps to highlight the SSC variations). SSC values were retrieved directly from images Landsat-8 OLI (WRS path1/row54), acquired on May 24, 2014, August 12, 2014, and February 20, 2015 using the regression Eq. (1).
The satellite-retrieved mean SSC values at Ciudad Bolivar on May 24, 2014; August 12, 2015 and February 20, 2015 were of 113 mg·l−1, 29 mg·l−1 and 51 mg·l−1, respectively. The satellite-retrieved SSC values match well the ranges of SSC values registered in the hydrological station at Ciudad Bolivar (see hydrographs between 2007 to 2015 shown in Fig. 9).
Fig. 9A shows the Orinoco River SSC map during the flood peak of May 2014. The white window #1 divided by Angostura Bridge, shows that there is a slight contrast in SSC values. In general, upstream from Ciudad Bolivar, SSC tends to increase from the riverbanks toward the center of the river associated with higher velocity flow. However, at Ciudad Bolivar, the satellite-derived SSC map shows very high homogeneity of SSC, with a mean SSC value of 113 mg·l−1, denoting a “funnel” effect for this reach.
Fig. 9B matches the river discharge peak of August 2014 with a mean river discharge of 67,332 m3·s−1 at the Ciudad Bolivar station. The white window #3 shows a mean SSC value of 29 mg·l−1. Again, this sector shows high SSC values in the mainstream until the river broadens downstream, inducing a decrease in SSC. The SSC at peak discharge also shows an increase from the sides toward the center. In the white window #4, it is possible to observe the remobilization of suspended sediments due to increased turbulence and vorticity, particularly in the center of the mainstream.
Fig. 9C corresponds to a low-water stage (February–March). The white window #2 shows an increase in SSC, due to resuspension processes of the fine material that was deposited during the previous flood on islands that appeared during the dry-period stage. This can be seen clearly in the white windows #5 and #6. The image shows a very high homogeneity in SSC with a mean SSC value of 51 mg·l−1 along almost the entire length of the channel.
These three satellite-derived SSC maps illustrate successfully that remote sensing can be used either to study seasonal variability of SSC or to infer transport processes related to fluvial geomorphology.
5 Conclusions and perspectives
Three atmospheric correction methods applied to L-8 OLI images were compared using spectral profiles at different river stages to determine the most accurate method, considering: seasonal changes, lighting conditions, aerosol, acquisition geometry, among others. The L8SR product used in this study appears to be the most adequate for atmospheric correction. The application of the quality mask from the “sr-cloud” band (Zhu et al., 2015) makes it possible to filter out the pixels that are affected by atmospheric distortions. However, the accuracy of the product L8SR used in this study should be further investigated in the future, as it can be viewed as a provisional product from USGS.
This study established a procedure to estimate and map the SSC in turbid waters by developing and applying linear regression models to Landsat-8 OLI data, using the lower Orinoco as a case study. The method described in this paper can be used to map and analyze spatial patterns of SSC, as well as statistical analyses using multi-spectral imagery. Considering its temporal coverage, spatial resolution and data availability, the Landsat-8 OLI system is well suited for the study suspended sediments in the Orinoco River.
The band 5 (NIR) L-8 OLI reflectance channel demonstrated very good SSC retrieval performances (R2 = 0.92) and for different river stages. The stability in time of the linear regression model using band 5-NIR (Eq. (1)), indicated that it is feasible to apply this equation to SSC estimate in different seasons in any reach of the lower Orinoco, even if no in situ data are available.
One river reach was studied with more attention, where a funnel effect at Ciudad Bolivar promotes the homogenization of suspended sediments throughout the water column, making that SSC at the river surface is representative of the average suspended sediment concentration in the whole river section. For “non-homogenized” river sections in the upper reaches of the Orinoco River, the relationship between SSC at the river surface and in the whole water column should be investigated, as it is currently largely unknown. Performing systematic measurements of SSC at different water depths using sampling protocols developed for large rivers (Filizola and Guyot, 2004), it may be possible to assess the average SSC value from the satellite-derived surface SSC value for the fine fraction of the suspended sediment.
The methods outlined in this paper could be applied to other inland waters, but the specific coefficients of the model may vary because of the optical characteristics of the SSC, based on size of particle, form, color, and type of mineral, as well as on organic substances that can affect the optical characteristics of turbid waters. These aspects will be addressed in future studies to fully understand the suspended-sediment transport processes of the Orinoco River.
Our upcoming efforts will be directed towards coordinated measurements related to in situ SSC and satellite acquisitions over tributaries, floodplains, and lagoons nearby the Orinoco River mainstream. In particular, we will explore the potential of new spaceborne sensors satellites such as the Sentinel-2A (available since June 2015) and Sentinel-2B (available from mid-2017). By combining with Landsat-8 images, it will be possible to increase the revisit frequency down to 2–3 days, enhancing the remote sensing monitoring capacity for suspended sediment retrieval and supporting the modeling of sediment discharge along the Orinoco River.
Acknowledgements
This study is part of the two bi-national cooperation projects between Venezuela and France. The SO-HYBAM monitoring project (www.so-hybam.org) and the Ecos-NORD/Fonacit (V14U01) project financed the collection of in situ SSC data and laboratory analysis. Mr. Santiago Yepez’ PhD thesis and stay at GET Laboratory (GET, UMR5563, CNRS/IRD/UPS3) in Toulouse is funded through Venezuela's FUNDAYACUCHO Grant No. E-223-14-2014-2 and Campus France. We wish to thank all Venezuelan and French participants in these two projects who contributed to this study. Landsat Surface Reflectance products were downloaded from the U.S. Geological Survey. The authors are also grateful to the SAMSAT2 and OBS2CO projects funded by the French Space Agency CNES.
Vous devez vous connecter pour continuer.
S'authentifier