1 Introduction
Three-dimensional intracellular structure data are often necessary in biological studies. Thus, it may be helpful to detect cell events if one can localise fluorescent probes targeted to cell compounds such as cell proteins or DNA sequences. These measurements may be obtained by multi-labelling techniques involving multi-wavelength acquisition 〚1〛. The numbering of chromosome copies within interphase cells using alpha-satellite or locus specific fluorescent probes is a powerful approach to detect chromosomal number abnormalities 〚2〛, but requires accurate optical conditions in order to localise and separate fluorescent sites within nuclei 〚3〛.
The 3-D data can be acquired by Optical Sectioning Microscopy (OSM), which is performed by axial stepping of the object versus the objective. A stack of 2-D images is the optical equivalent of a series of microtome slices, allowing a 3-D reconstruction of a specimen. Most of the three-dimensional fluorescence microscopy techniques now use a confocal microscope 〚4〛. Compared to the conventional fluorescence microscope, the confocal microscope design reduces haze from out of focal-plane objects. Although confocal microscopy has many advantages, it does have some limitations. The amount of excitation light required to produce a confocal image may be a problem for fixed specimens labelled with several different dyes and for long-term 3-D imaging of living samples.
The image formed by a conventional fluorescence microscope contains light from throughout the specimen. What is actually present in the focal plane may be blurred by this ‘out-of-focus’ fluorescence. Reduction of this side effect is the aim of 3-D microscopy 〚5〛. Particularly in conventional microscopy, this reduction is carried out computationally by deconvolution process.
The characterisation of the out-of-focus light is based on the 3-D image of a point-like source, the so-called Point Spread Function (PSF). The image formation process can be described by the following convolution equation:
Image processing is a key element, and a lot of efforts have been devoted to develop specialised computer tools. To summarise, one can split this process in four steps: image acquisition, deconvolution, feature extraction, which give various data: number, size and intensity distributions of fluorescent sites for example, and eventually at the end the biological interpretation of processed data. The data acquisition requires full hardware control of the acquisition system (filter selection, stage scanning, illumination and image acquisition synchronisation). As a consequence, specialised softwares are provided by manufacturers (EPR™, DeltaVision™) of the processing system.
A tremendous work has been dedicated to fluorescence image deconvolution in recent years. This has led to the development of special image processing softwares (XCOSM, Bit-Plane™…). The data analysis is often performed using more general tools like Noesys™ or Analyze™. So, to analyse a single specimen, the biologists often have to use several platforms and/or softwares.
In the mind of simplifying the 3-D fluorescent images exploitation, a package integrating all the tools necessary for a complete biological analysis, called VIEW3D, has been developed. It is a package allowing in a simple environment modelling and identification of microscopy systems, as well as deconvolution, visualisation and measurement of data. Since this processing takes into account the experimental conditions, the results obtained using this method are supposed to be more reproducible, improving the reliability in medical analysis. An example of application in the fields of cytogenetics is provided in section 4.
2 Methods
2.1 System identification
In order to characterise the optical system, it is necessary to measure the PSF, which describes the properties of the image acquisition system. The three-dimensional Fourier transform of the PSF is called the Optical Transfer Function (OTF) of the system. The OTF describes the spatial frequency distribution. The PSF is a function of different instrumental parameters, such as numerical aperture (NA), emission wavelength (λem), refractive indices of the different media along the optical path and optical aberration of the lens 〚8〛. Consequently, it is useful to quantify the variations in the PSF distribution induced by different conditions of observation. A PSF can be either measured or computed. Experimental PSFs are obtained by imaging small fluorescent latex beads (Interchim or Molecular Probes). Ideally the microspheres should be as small as possible, but the smallest ones are weak in intensity and bleach rapidly. A trade-off must be found to define the microsphere diameter according to the characteristics of the microscope objective in use. Usually beads with a diameter of about one third of the Rayleigh criterion are used 〚9〛. Computed PSFs are noiseless, however they do rely on a simplified model of the microscope. Furthermore, the assumed experimental parameters in the computational model may differ from their actual values. Statistical tools have been developed to quantify the variation of the PSF as a function of the optical parameters: fluorescence wavelength, NA of the objective, refractive index of the immersion oil, optical tube length and specimen thickness. They are based on higher order statistical moments, known as excellent descriptors of the energy distributions and symmetries of the PSFs as well as of the aberrations of the system 〚10〛.
The study of the system identification has been first carried out on the PSFs computed with the Gibson and Lanni model 〚11〛, then applied to experimental PSFs. Even if a good correlation is observed between simulations and experiments, experiments are the only methods allowing to characterise optical aberrations like chromaticity and asymmetry of the system.
A specific adapted protocol may be proposed by determining the precise influence of the different experimental parameters on the PSF.
2.2 Deconvolution
The main objective of deconvolution is to reduce the image distortions by redistributing the fluorescence intensities and reconstructing geometrical shape of the sample. Numerous deconvolution methods have been described in the literature 〚12, 13〛. Up to now, we have focused our attention on methods using the PSF and techniques well adapted to fluorescence microscopy, such as non-iterative LLS (Linear Least square Solution, developed by Preza 〚14, 15〛) and Maximum A Posteriori (MAP) 〚16〛 algorithms, iterative ML–EM (Maximum Likelihood Expectation Maximisation, developed by Holmes 〚17〛) and the Carrington 〚18〛 algorithms. The reader may refer to published literature for a detailed description of these algorithms. These methods do take into account the system singularity and the presence of noise. However, a number of regularisation parameters should be introduced by users, which may modify the subjectivity of the results. In order to determine this parameter automatically, the sum of the image bias error and the noise variance is minimised according to the image acquisition system PSF. In this way, the reproducibility of the measurements is improved.
3 Materiel
3.1 Microscope
An upright epifluorescence microscope BX-51 PROVIS (Olympus) with a 100 W xenon lamp as excitation source is used. Image acquisitions are carried out with an apochromatic, infinity corrected, 100 × oil immersion objective lens (numerical aperture of 1.4).
The microscope is equipped with the EPR™ optical sectioning acquisition system: a piezoelectric translation device is inserted in between the motorised revolving nosepiece and the objective. The minimum scanning step is 0.25 μm and the maximum scanning range is 100 μm.
The image sensor used is a Peltier-cooled charge-coupled device CCD-1317K camera from Princeton Corporation. The pixel size is 6.8 × 6.8 μm2 and the matrix dimension are 1317 by 1035 pixels. The intensity is 12 bits coded.
3.2 Software
Combining our 3-D fluorescence microscopy with some biologist expertise, a set of tools for the analysis of images from wide-field or confocal microscopy has been developed. This all-in one toolbox VIEW3D is developed with PV-WAVE™ (Unix and Windows compatible). The graphical user interface provides direct access to various operations: image loading and visualisation, deconvolution, segmentation, 3-D geometrical measurements and morphological description. The results may be collected and saved under various formats to export them to other softwares (spreadsheets or presentation tools).
VIEW3D graphical interface includes several modules (Fig. 1):
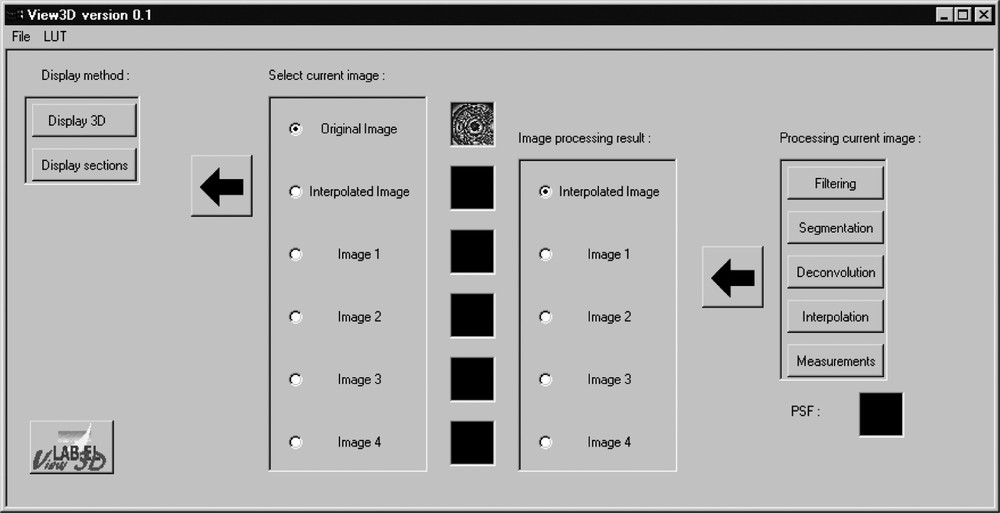
‘VIEW3D' main menu, the left buttons are dedicated to visualisation and the right ones to image processing tools. The icon images represent the central plane of loaded data.
- • data acquisition (Fig. 2);
- • XY and XZ plane by plane interactive viewing (Fig. 3);
- • 3-D visualisation (Fig. 4);
- • confocal or conventional microscope PSF computation;
- • deconvolution, segmentation, connected components labeling (Figs. 5 and 6);
- • data analysis – shape descriptor, volume and intensity measurements – (Fig. 7).
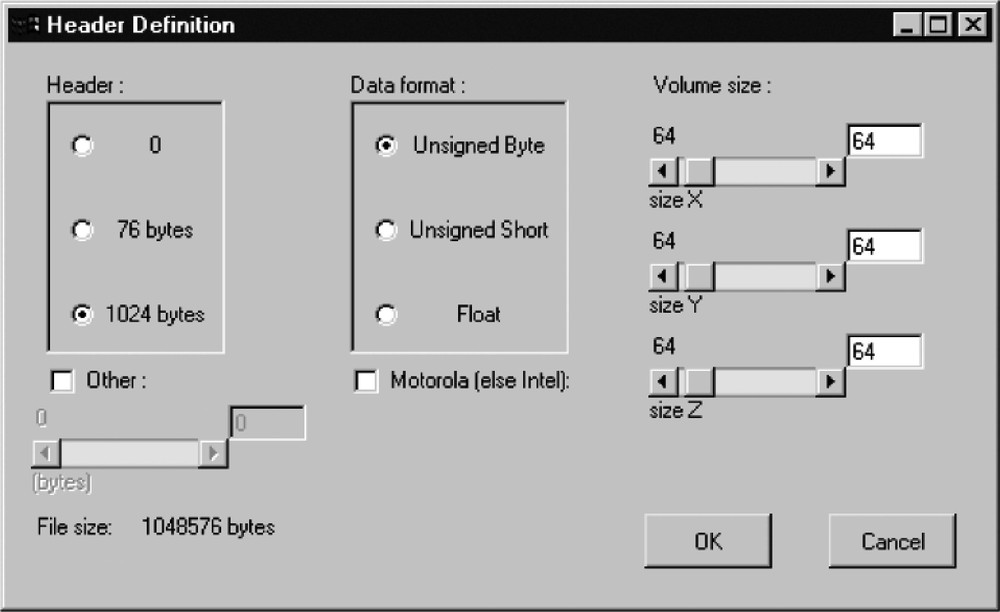
View box for raw data acquisition; the user is invited to precise header size, data format and volume size.
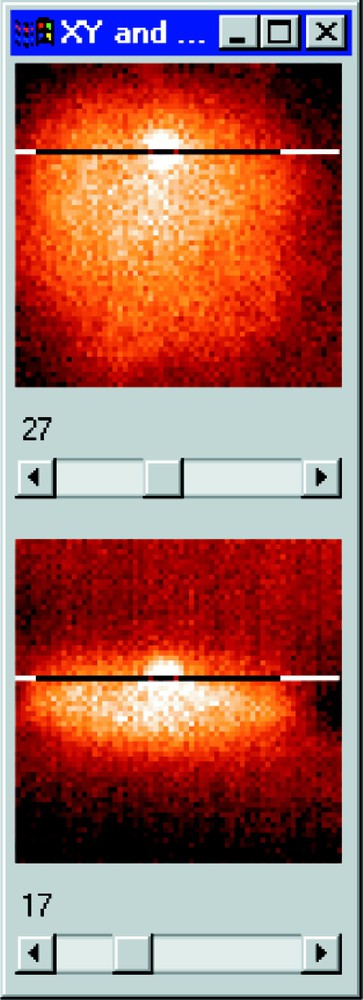
Section visualisation of a cell nucleus. The 17th XY plane is presented above and the 27th XZ section below.
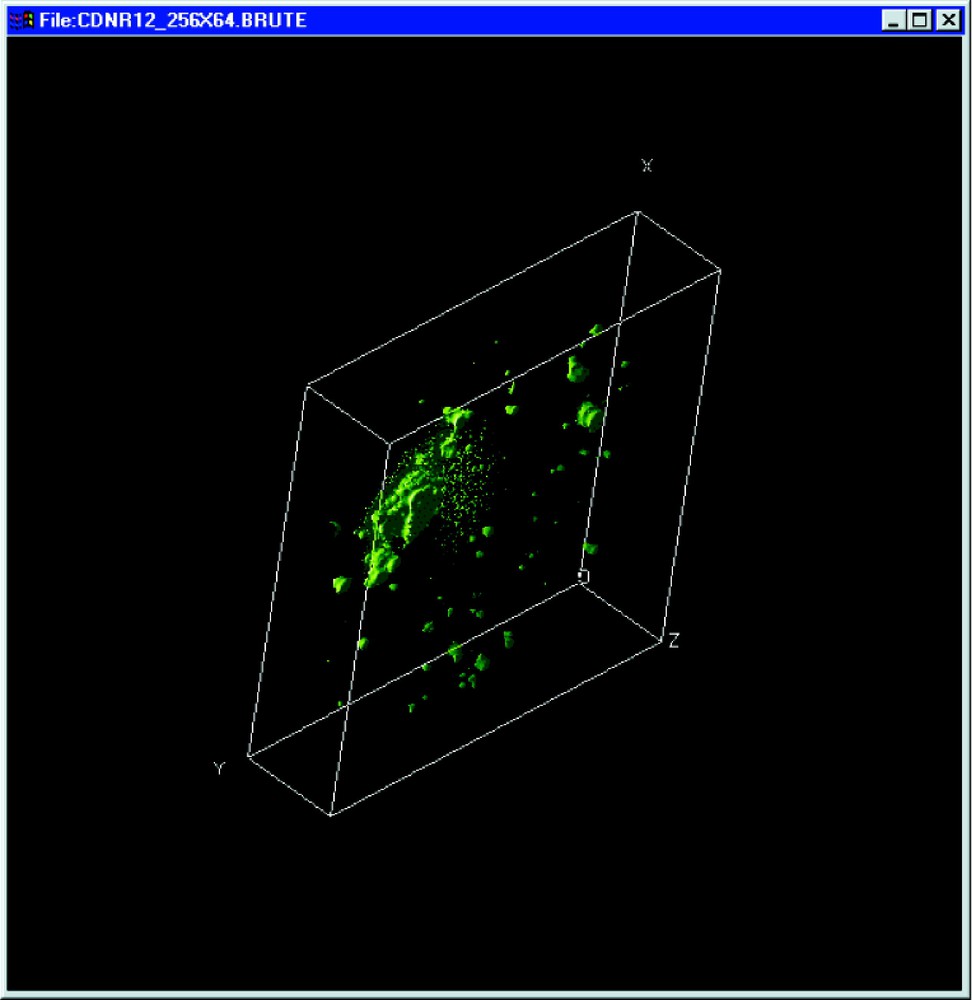
3-D visualisation of a cell nucleus. The embedding box is indexed by the X, Y and Z coordinates.
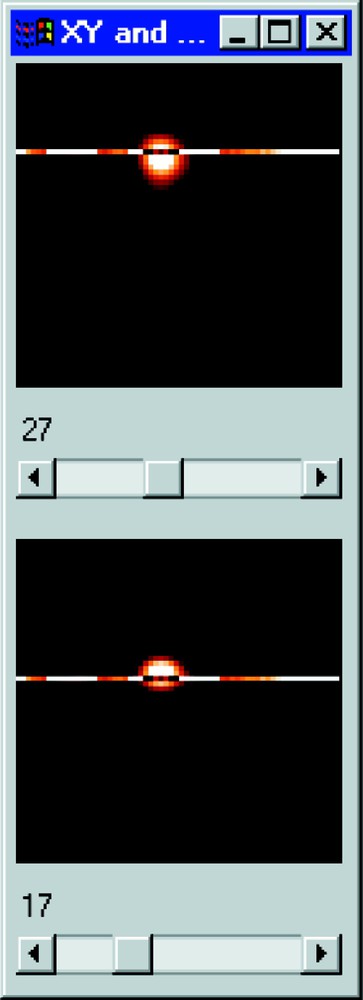
Section visualisation of a cell nucleus after MAP deconvolution. The 17th XY plane is presented above and the 27th XZ section below.
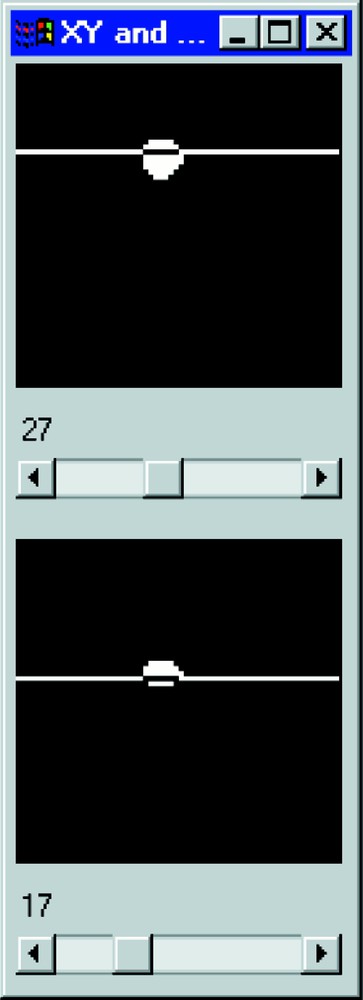
Section visualisation of the deconvolved cell nucleus after segmentation. The 17th XY plane is presented above and the 27th XZ section below.
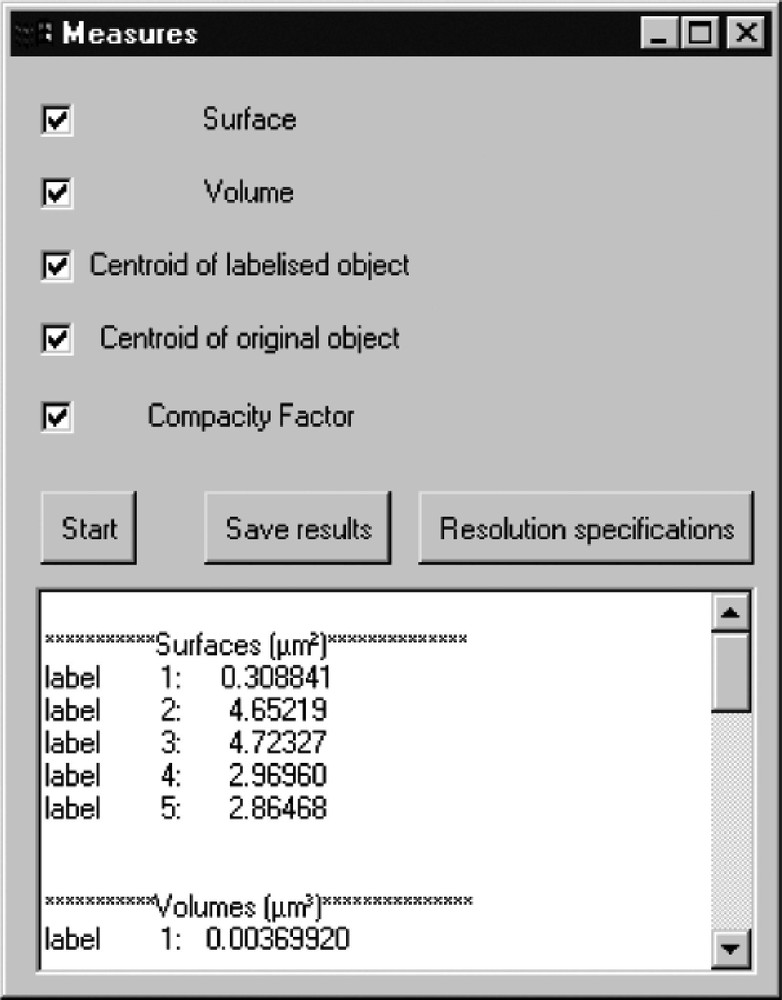
Results in the box of measurements obtained after processing are presented. Five different fluorescent sites are detected; surfaces and volumes are calculated.
The following deconvolution algorithms are available: LLS, MAP, ML–EM and Carrington’s methods. Segmentation is performed by intensity thresholding or via mathematical morphology. The 3-D labelling is provided for analysis and measurements of fluorescence sites. Volumes, surfaces, compactness factors and up to the 4th order centred moments (mean, variance, skewness and kurtosis) are implemented. Such information allows quantitative measurements (volumic and intensity distributions) of the fluorescence sites, thus facilitating specimen classification.
3.3 Biological samples
Human uncultured amniocytes have been obtained by amniocentesis and spread on microscope slides after centrifugation of 3 ml of amniotic fluid at 1 000 rpm for 8–10 min. Fluorescent in situ hybridisation (FISH) probes were commercial products available from Vysis (Downers Grove, IL, USA) and applied according to the manufacturer’s instructions. Rhodamine-labelled locus specific probes (LSP) D21S259, D21S341 and D21S342, spanning the region 21q22.13-q22.2, have been assayed in order to numerate the chromosome 21 copies on interphasic nucleus.
4 Results
To validate our program, VIEW3D was firstly experimented using images of calibrated fluorescent beads.
Identification tools allow characterising aberrations in order to determine if the system is under the design conditions. Some parameters, such as the refractive oil immersion index, the specimen depth and the NA, are the most interesting parameters in order to get an optimal acquisition 〚19〛.
Deconvolution methods have been compared regarding to intensity reassignment, geometrical reconstruction and speed. As previously published 〚20〛, the Z elongation and dissymmetries are the main distortions due to both index mismatch and specimen thickness.
The four methods, implemented in VIEW3D, improve image contrast and reduce the axial elongation. However, ML–EM is more appropriate for volume reconstruction, Carrington’s method for intensity reassignment of thin specimens and, because of their rapidity, LLS and MAP are more adapted to routine processing 〚21〛.
The practical application using some biological samples is currently under evaluation and preliminary results already show a significant improvement in the signal analysis after FISH, using LSP labelling of the 21q22.13-q22.2 chromosomal region. Fig. 8 shows the wide intensity spread of the fluorescent spots without image processing. Fig. 9 shows the same nucleus area after MAP deconvolution.
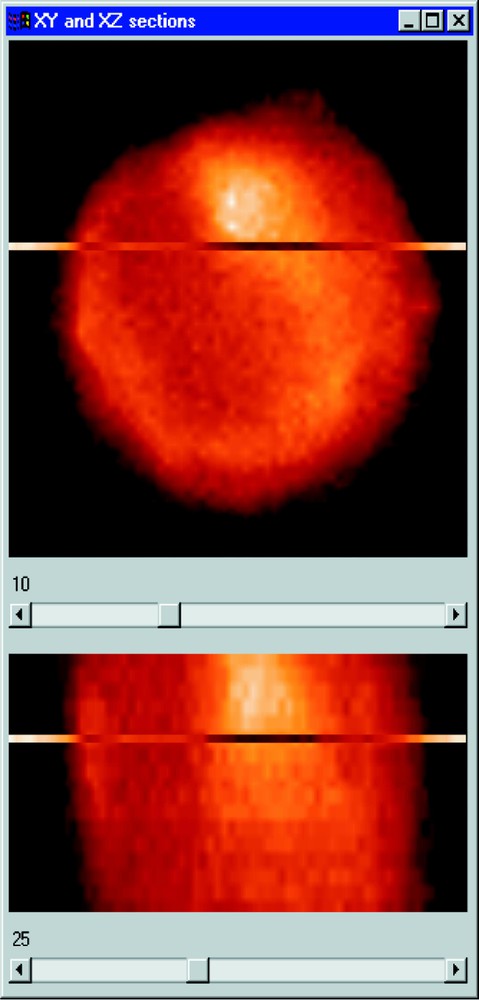
Sections of amniotic cell nucleus labelled with rhodamine-labelled DNA probe targeted to the 21q22.13-q22.2 chromosome region before deconvolution.
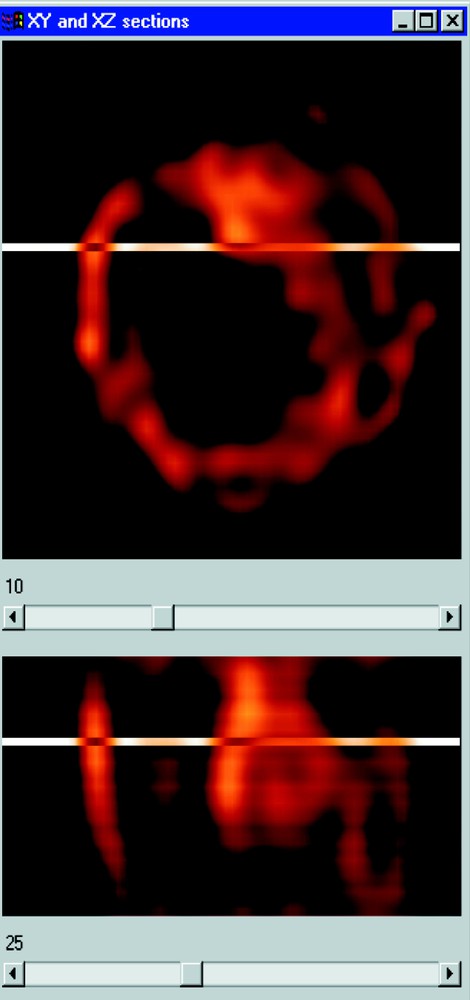
Same sections of amniotic cell nucleus labelled with rhodamine-labelled DNA probe targeted to the 21q22.13-q22.2 chromosome region after MAP deconvolution.
5 Discussion
Image restoration in 3-D fluorescence microscopy is mandatory to remove artefacts of raw data, allowing thus a reliable and reproducible biological analysis.
The precise determination of experimental parameters during the data acquisition is critical to the restoration process. However, many experimental parameters are both specimen-dependent, (refractive indices, thickness) and optical condition-dependent (oil refractive indices, NA). The Gibson and Lanni model used in VIEW3D is a user-friendly as well as efficient tool, since most of the experimental parameters are clearly defined. It may be not adapted to high NA objectives, because of its scalar model of diffraction underpinning. Therefore, vectorial theories of diffraction should be more appropriate 〚22, 23〛.
Preliminary results obtained with FISH on uncultured amniotic fluid cells show a significant improvement in both fluorescent signals localisation and separation, as presented in Figs. 8 and 9. This claims for the efficiency of the method to reduce the wideness of the fluorescence intensity spread, mainly due to the poor resolution along the microscope optical axis (Z), which usually induces artefacts in spots numbering 〚3〛.
For some biological specimens, intensity distribution and morphology may vary significantly from one sample to another. The photon noise is signal-dependent. It implies that the deconvolution process should be adaptive, i.e. regularisation parameters, even the deconvolution algorithm, have to be data-driven.
Data volume characterisation has been carried out. The purpose of this pre-processing is to split the entire volume into regions characterised by homogeneous statistical and morphological properties. This allows applying separately appropriate restoration techniques to each of them.
Furthermore, it is mandatory for users to be aware of the shorts of artefacts that can arise and what must be done to reduce them.
6 Conclusion
An embedded 3-D image-processing tool the VIEW3D has been developed to analyse fluorescent images of 3-D biological specimen. It enables the possibility to perform all quantification steps within the same environment. It integrates most of the functions required in 3-D biological measurements, i.e., 3-D specimen representation, system identification to define experiment protocol, raw data restoration for artefacts correction, segmentation, geometrical and morphological measurements of fluorescent sites (necessary for specimen analysis).
According to the nature of the studied specimen, this multi-platform compatible software allows users to choose one of the four types of implemented deconvolutions. VIEW3D has been designed in a modular way, making it easy to further incorporate new modules.
Both experiments on calibrated reference beads and on biological samples are very encouraging. There is no best deconvolution method. As a general rule, methods that require more computer time yield better results. However, in some cases speed may be critical. A panel of different algorithms being ‘at hand’ in the same package allows choosing first a rapid deconvolution method in order to select interesting samples on which time-consuming algorithms will be run.
This experimental approach leads to improved reproducibility of geometrical measurements on biological samples.
Acknowledgements
This work has been partially financially supported by a CNRS PCV98/082 project (15.24 k€), in collaboration with the Department of Membrane Protein and Receptors (CNRS UPR 9050), directed by Dr Jean-Luc Galzi. We also thank the biologists from the research laboratory in oncology of the ‘Centre Alexis-Vautrin’ (Vandœuvre-les-Nancy, France), directed by Pr. Jean-Louis Merlin, especially Corinne Bourg-Dill for her contribution on biological applications. The authors are grateful to Dr D. Dumas (laboratoire d’angiohématologie–hémorhéologie, faculté de médecine, Vandœuvre-les-Nancy, France) for his contribution to 3-D microscopy acquisitions.
Version abrégée
La microscopie photonique trouve un nouvel essor avec l’avènement de nouvelles technologies et passe d’observations classiques en deux dimensions à des acquisitions d’images tridimensionnelles d’échantillons biologiques vivants fluorescents.
L’acquisition d’images tridimensionnelles d’objets transparents repose sur l’utilisation d’un dispositif spécifique, qui permet d’effectuer un « découpage optique » (Optical Sectioning Microscopy) de l’objet par déplacement axial de l’objectif. Les caractéristiques particulières (perte de fréquences spatiales ou missing cone, singularité à l’origine) de la fonction de transfert optique (Optical Transfer Function ou OTF) rendent indispensable la restauration (ou déconvolution) des images avant toute mesure quantitative 3D.
La réponse impulsionnelle optique, appelée PSF (pour Point Spread Function) caractérise le système d’acquisition. Elle peut être mesurée ou calculée à partir de modèles scalaires ou vectoriels. Utilisant le model scalaire de Gibson et Lanni, associé à des données expérimentales, un outil statistique permettant l’identification de la réponse impulsionnelle a été développé. L’analyse théorique et expérimentale de l’influence des paramètres d’acquisition est essentielle pour optimiser le protocole d’acquisition sur échantillons biologiques.
La restauration devra améliorer la qualité des données : corriger les distorsions géométriques et réassigner les intensités à leurs origines. Une restauration de qualité nécessite la connaissance du système d’acquisition et donc de son OTF. Des informations a priori sur le spécimen étudié peuvent aussi s’avérer utiles. Quatre algorithmes de déconvolution ont été implémentés, réalisant, soit une inversion directe régularisée (LLS et MAP), soit une inversion itérative (ML–EM et l’algorithme de Carrington). Pour mener à bien la restauration, les paramètres de régularisation sont déterminés par une procédure automatique minimisant une fonction de coût adaptée. Cette automatisation doit permettre une plus grande facilité d’utilisation et une meilleure répétitivité inter-utilisateur.
Si l’on décompose en quatre parties le processus d’analyse d’images en microscopie de fluorescence, on distinguera : l’acquisition des données, la déconvolution, la quantification et enfin l’interprétation des données. Les outils d’investigation ont été intégrés dans un logiciel appelé VIEW3D, dans le but de faciliter leur utilisation. Dans cet environnement sont regroupés les outils de visualisation 3D, d’identification de la fonction de transfert, quatre algorithmes de déconvolution automatisés et des outils de mesure 3D.
À l’aide de billes fluorescentes de référence, ces outils sont testés en particulier pour déterminer le facteur d’élongation axiale afin d’effectuer la correction. Les analyses statistiques, géométriques ou morphologiques deviennent alors significatives.
Pour illustrer ces travaux, cette méthodologie est appliquée sur des images de noyaux cellulaires marqués par une molécule fluorescente constituée d’une sonde d’ADN marquée à la rhodamine, utilisée en cytogénétique.
Les premiers résultats obtenus sont prometteurs. Cependant, les études sur échantillons biologiques sont assez complexes, en raison de la diversité de la distribution de l’intensité et de la morphologie des zones d’analyse. Ceci implique de rendre le processus de déconvolution adaptatif par l’utilisation de pré-traitements des zones d’intérêt biologique.