1 Introduction
The principal goal of pharmacogenomics is clear: Use information on the molecular profiles of tumor cells to individualize therapy for cancer or select more appropriate therapy for particular subgroups of patients. For the most part, that goal is being pursued through study of clinical tumors, but the task has proved more difficult than expected. There are several reasons [1]: (i) clinical tumors are difficult to study, given anesthesia effects, surgical trauma, and constraints (both logistical and ethical) on the design of clinical trials; (ii) clinical tumors often have complex, fragmentary histories. Demographic and clinical information may be difficult to obtain because of ethical or legal issues; (iii) clinical studies are expensive; (iv) clinical tumors are heterogeneous – a mixture of cancer cells and stromal components, including endothelial cells, fibroblasts, and infiltrating leukocytes. Any molecular profile obtained for a bulk tumor is a mixture of the characteristics of those components. Techniques such as laser capture microdissection [2] can be used to isolate tumor cells, for example in the pseudo-glandular epithelial structures of adenocarcinomas. But then an amplification method must generally be used to generate enough DNA or mRNA for study. Methods of amplification available include T7-viral amplification, rolling circle amplification, two-primer PCR, and single-primer PCR, but their fidelity is still a significant question [1].
In contrast, cell lines have the advantage of being homogeneous in cell lineage (though not in cell cycle state). They can be obtained in quantity; they are reproducible from experiment to experiment and year to year; they can be manipulated by transfection, knockout, selection for resistant forms, or exposure to siRNA, antisense RNA, drugs, or radiation. The problem, of course, is that they are not really representative of cancer cells in vivo. Even primary cultures of cancer cells have been removed from the influence of other cell types, cytokines, extracellular fluid, and the three-dimensional architecture of the tumor. They have been selected for growth on plastic in standard medium with relatively fast cell-cycling. Therefore, prediction forward from cultured cells toward the clinic is uncertain; we can, at best, obtain clues to formulate hypotheses to be validated in real tumors, either clinically or through pathological studies, for example using tissue arrays. When one extrapolates backwards from cell line studies to the basic biology or pharmacology, however, one is on reasonably sound ground. Most of our knowledge of the biology and pharmacology has, in fact, been obtained from cultured cells or else from molecular studies, not from clinical materials [1].
For pharmacological purposes, we would like to study cancer cell types that have been exposed to large numbers of potential drugs. The most prominent such cell set is the 60 human cancer line panel (the NCI-60) used by the Developmental Therapeutics Program (DTP) of the US National Cancer Institute (NCI) to test for potential anticancer agents. The cells have been characterized pharmacologically by exposure to more than 100,000 defined chemical compounds (plus a large number of natural product extracts), one at a time and independently.
2 The NCI-60 cancer cells and screen
As of 1985, the NCI was using P388 murine leukemia to screen compounds for anticancer activity. That strategy identified agents active against leukemias but was not thought to be effective in identifying activity against the common solid tumors of humans. Therefore, the decision was made to seek a different strategy for screening. The result after many competing factors were taken into account was the NCI-60 cell screen, which went into production mode in April of 1990. Since then, chemically defined compounds (plus natural product extracts) have been screened. Since 1991, the 60 cell lines have included leukemias, melanomas, and cancer cells of renal, ovarian, colon, breast, prostate, lung, and central nervous system origin. That list is by no means complete, but it includes the most common human tumors. The guiding hypothesis was that selective activity against cancers from a particular tissue or organ would predict clinical activity against the same type of tumor. Such predictiveness has not been demonstrated, but the NCI-60 system took on a new role: increasingly, it has been used for secondary profiling of compounds already found to attack a defined molecule or pathway. Even more generally, it became a system for profiling both the compounds tested and the cell lines.
Fig. 1 shows the NCI-60 system in highly schematic form. Database (A) of activities can be mapped into a database (S) of structural characteristics of the compounds tested and a database (T) of molecular targets and other cell characteristics. This set of databases provides the conceptual architecture for the pharmacogenomic studies to be described here. The first topic to be discussed will be the screen itself.

Simplified schematic overview of an information-intensive approach to cancer pharmacogenomics and pharmacoproteomics based on the NCI-60 cancer cell lines. Each row of the activity (A) database represents the pattern of activity of a particular compound across the 60 cell lines. The A database can be mapped into a structure (S) database containing 2D or 3D chemical structure descriptors of the compounds and a target (T) database containing molecular profile information on the cells. The T database consists of data on individual molecules and omic data at the DNA, mRNA, protein, and functional levels. The bioinformatic challenge is to analyze and understand each of these databases separately, then to integrate them with each other and with public information resources for pharmacogenomic purposes. Modified from [25].
2.1 Methodology of the NCI-60 screen
The methodology of the NCI-60 screen has been described in detail elsewhere (see http://dtp.nci.nih.gov). Briefly, on day zero the human tumor cell lines are plated in 96-well microtiter format in RPMI 1640 medium with 5% fetal calf serum and 2 mM l-glutamine. On day 1, the drug (dissolved in DMSO) is added to achieve five concentrations at 10-fold intervals, plus a negative control. The usual concentration range is 10−8 to 10−4 M. After 48 h of drug exposure at 37 °C, the cells are fixed in situ with trichloroacetic acid. The supernatant is discarded (along with floating cells and cell fragments), and the plates are washed five times, then air-dried. Colorimetric measurement of sulforhodamine B (SRB) dye is used to quantitate the cell material remaining attached to the well at the end of the incubation period. The 50% growth inhibition (GI50) is calculated as the concentration of drug required to inhibit cell growth by a factor of two. The fundamental parameter used as a measure of potency is −log10GI50. The details of this protocol are important to an understanding of the meaning and limitations of the data from it.
2.2 The Activity (A) database
While analyzing data from pilot studies for the screen in the late 1980's, Kenneth D. Paull realized that the absolute potency of a compound gave much less information on its mechanisms of action and resistance than did the pattern of relative activities across the cell lines. He therefore subtracted out the log-mean over the 60 cell lines to obtain the very useful ‘mean-graph’ representation of activity data. The lack of information on mechanism in absolute potency values was later corroborated formally by principal components analysis [3–5].
The mean graph representation of patterns led to the COMPARE algorithm [6,7]. Given one compound as a ‘seed’, COMPARE searches the database of screened agents and compiles a list of those most similar to the seed in their patterns of activity against the NCI-60 panel. Similar patterns generally indicate similarity in mechanism of action, mechanism of resistance, and/or molecular structure. The similarity metric was initially taken as the Euclidean distance, later as the Pearson correlation coefficient. COMPARE has been applied productively to topoisomerase inhibitors [8–13], pyrimidine biosynthesis inhibitors [14], compounds with preferential effects against Nm23-expressing cells [15], anti-mitotics [16–19], and agents active against epidermal growth factor-expressing cells [20], among many other classes of compounds.
In 1992, we introduced feed-forward, back-propagation neural networks (with statistical analysis by cross-validation and sensitivity analysis) to discriminate among various possible mechanisms of drug action on the basis of activity patterns [21]. A large number of other statistical and artificial intelligence techniques have since then been applied to the relationship between pattern and mechanism. Among those methods have been principal components analysis [3–5] and Kohonen self-organizing maps [5,22,23]. Self-organizing maps in this context are used to represent the structural or functional similarities of compounds in the form of two-dimensional maps.
In 1994, we introduced clustering and ‘clustered image maps’ (CIMs) for analysis and visualization of the pharmacological and molecular data [24,25]. Fig. 2 shows a CIM that correlates the activity patterns with molecular characteristics (‘targets’) of the cells, including gene expression data. CIMs have since become the most popular way to represent gene expression data sets visually (although they by no means capture all of the information available in those data).

Clustered Image Map of the relationship between compounds tested and molecular targets in the NCI-60 cells. This normalized A·TT product matrix (where the superscript T indicates the matrix transpose) correlates target patterns with patterns of growth inhibition for a set of 3989 important compounds. A red or orange point (high positive Pearson correlation coefficient) indicates that the agent tends to be selectively active in the SRB assay against cells lines that express the target in large amounts (or in functional form). A dark blue point (high negative correlation) indicates the opposite. The 113 columns correspond to 76 distinct target molecules or functions, some represented multiple times in different mathematical transformations. Compounds and targets have been cluster-ordered by an average linkage algorithm to bring like together with like. To the right is shown one 61-leaf ‘twig’ of the overall 3989-leaf cluster tree of compounds. Symbols for mechanisms of action are as follows: T1, topoisomerase 1 inhibitors; T2, topoisomerase 2 inhibitors; A, alkylating agents; Pt, platinum compounds; Pt–Si, platinum agents containing a silane moiety; ?, mechanism unknown. The most prominent features are a red patch that indicates compounds (2802–3309) that tend to be active in the assay in cell lines with intact p53 function and a blue patch that indicates compounds (513–667) selectively inactive in Mdr-1/Pgp-expressing cells. Modified from [25].
2.3 The Structure (S) database
The chemical structures in S can be coded in terms of any set of one-, two-, or three-dimensional descriptors. Useful structural codings can be found at the DTP's web site. Analyses that relate the S and A databases can be thought of as generalizations of the Q-SAR (‘quantitative structure-activity’ relationships) paradigm. A number of studies have highlighted various aspects of these relationships for the NCI-60 [4,26–30]. Genetic function approximation [31] (an amalgam of genetic algorithm for variable selection and regression splines for data fitting) proved a useful approach [4,26,28]. Since structural descriptors are available for compounds [32], it has been possible to map interesting patterns of activity into the S database and develop abstract pharmacophore templates with which to search the compounds not yet screened and bring candidate compounds into the testing process.
2.4 The Target (T) database
2.4.1 Miscellaneous molecular targets
The first molecular target analyzed experimentally and analytically was the drug resistance transporter P-glycoprotein (Pgp), encoded by the multi-drug resistance gene Mdr-1 [33–36]. Fig. 2, a clustered image map obtained by combining information from the T and A databases, shows the importance of Pgp/Mdr-1 to the pattern of drug sensitivities of the cell lines. The dark blue patch for compounds 513–667 indicates that those compounds are negatively correlated with targets 81 to 88, which are the indices of Pgp/Mdr-1 expression and function. The statistics were impressive. We analyzed NCI-60 data for a set of 35 compounds of diverse structure and mechanism that had been reported previously, on the basis of transport assays, to be Mdr-1 substrates [33,35,37,38]. Of those, 18 (51%) fell within the blue patch, whereas only 4% would have been expected to do so by chance. The probability (exact binomial) of such an extreme enrichment being found by chance is <0.0001. Eighteen of the 35 reported substrates fell within the blue patch, whereas 0 of 12 compounds studied and reported not to be substrates [33,35,37,38] did so (P=0.001 by Fisher's exact test). As might have been expected from the known pharmacophoric properties of Pgp substrates, compounds 513–667 were highly enriched for natural products of high molecular weight, often cationic. By linear discriminant analysis, we found that those three factors could predict with a specificity of 84% and sensitivity of 78% which compounds would be found in the blue patch (P<0.0001). Columns 76 and 77 in Fig. 2 are indices of mRNA expression for Mrp-1, another transporter molecule associated with multidrug resistance [34]. There was little overlap between compounds sensitive to Mrp-1 and those sensitive to Pgp/Mdr-1. These calculations provided a proof of principle for the pattern recognition process [25]. Various other molecular targets have been assessed in the NCI-60 system, most prominently a set of molecular characteristics associated with p53 function [39]. Data on miscellaneous targets can be found at http://dtp.nci.nih.gov.
2.4.2 ‘Omic’ profiling
To complement studies in our laboratory and many others of individual targets in the NCI-60, we have taken an ‘omic’ approach [40,41], characterizing DNA, mRNA, and protein species in the cells in aggregate. The result is the richest, most varied profiling of any set of cells that we know of.
2.4.2.1 Proteomic and DNA-level profiling.
We began with proteins in the early 1990's, doing 2-D gel electrophoresis [42] and developing a MALDI-TOF mass spectroscopic protocol for identifying proteins on the gels [43]. However, by that time it was clear that identification of hundreds or thousands of proteins was not a job for a small academic laboratory. Hence, we decided to wait for the proteomic technologies to improve and, meanwhile, dropped back to the transcript level, where the task appeared easier. Those studies will be described in the next sections.
In parallel, with the transcriptomic studies, we have undertaken collaborations with a number of laboratories for profiling at the DNA level: with the laboratory of Ilan Kirsch and Anna Roschke (NCI) for spectral karyotyping (SKY) and comparative genomic hybridization (CGH) ([44]; also Roschke, et al., in preparation); with that of Kenneth Buetow (NCI) using Affymetrix SNP chips for single nucleotide polymorphisms (Alexander, et al., in preparation); with that of Joe Gray at the University of California Cancer Center for CGH based on BAC arrays (Chen, et al. and Bussey, et al., in preparation); with the laboratories of David Munroe (NCI) and Andrew Feinberg (Johns Hopkins University) for detailed sequence analysis of cytosine methylation in the promoter regions of cancer-related genes (Reinhold, et al. and Maunakea, et al., in preparation).
Most recently, at the protein level, with Lance Liotta (NCI) and Emanuel Petricoin (Food and Drug Administration), we have developed high-density ‘reverse-phase’ protein lysate microarray for proteomic profiling of the 60 lines without the need for spot identification ([45] and Nishizuka, et al., in preparation). For validation of hypotheses directed toward the clinic, we have used tissue arrays produced by the TARP (Tissue Array Program) Consortium at the NCI [45]. The arrays consist of cores from 503 human tumors of disparate types plus 62 normal human tissues. Although this article focuses on the transcriptome, it is worth noting that a major part of our effort is devoted to understanding, and capitalizing on, the relationship among the various types of data. Not entirely in jest, we refer to this enterprise as ‘integromics’.
2.4.2.2 Transcriptomic profiling.
In part, the challenge at the mRNA level appeared easier because there are ‘only’ 30–60 000 independent transcripts and perhaps 200,000 splice variants of those transcripts, rather than the 500 000–2 000 000 functional protein states. We were able to generate transcript profiles for the NCI-60 using four different platforms: a 7907-clone cDNA array with the Brown/Botstein laboratory [46,47], a 6800-gene Affymetrix oligonucleotide chip (Hu6800) with the Golub/Lander group [48], and the Hu95 and Hu133 Affymetrix oligonucleotide chips with Uwe Scherf at Gene Logic, Inc. Versions of the first two data sets used for our calculations are available at http://discover.nci.nih.gov.
2.4.2.2.1 Transcript expression profiling by cDNA array.
The methods used in this study have been described in detail elsewhere [46,47]. Very briefly, cells were harvested (with less than 1 minute from incubator to stabilization of the preparation) at approximately 80% confluence. Total RNA was stored and then further purified to obtain poly-A mRNA shortly prior to hybridization with microarrays (Synteni, Inc.; now Incyte, Inc.) consisting of robotically spotted, PCR-amplified cDNAs on coated glass slides [49].
The 9703 DNA elements on the array were cDNAs from the Washington University/Merck IMAGE set, obtained from Research Genetics, Inc. The array included 3700 named genes, 1900 human genes homologous to those of other organisms, and 4104 ESTs of unknown function but defined chromosome map location. For each hybridization, cDNA from the test cell's mRNA was labeled by incorporation of Cy5-dNTP during reverse transcription. cDNA synthesized from pooled mRNA of 12 highly diverse cell lines out of the 60 [47] was analogously labeled by incorporation of Cy3-dNTP. Cells for the pool were selected to satisfy 3 criteria [47]: (i) at least one cell line from each organ of origin; (ii) diversity of growth rates; (iii) diversity in terms of protein expression pattern, based on prior two-dimensional gel studies [42]. After appropriate filtering, we settled on a data set of 1376 clones for detailed analysis and added forty miscellaneous cancer-related targets from the DTP database.
Fig. 3a shows a cluster tree that represents the patterns of gene expression across the cell lines. As indicated by the accompanying annotations, there is considerable, but not complete, regularity by organ of origin. Fig. 3b shows the strikingly different tree obtained when the same cells are clustered on the basis of drug activity. The ‘correlation of correlations’ [47] between the two trees was only +0.21. The correlation of correlations, rc, is a parameter we developed to quantitate the similarity of two clusterings. In the present context, rc is the mean Pearson correlation coefficient of the Pearson correlation coefficients relating all 1770 possible pairs of cell types in terms of their response to drugs and in terms of their gene expression. More generally, rc can be used to quantitate the similarity of any two distance matrices such as those used in hierarchical clustering. For example, we have used it to compare different distance metrics applied to one data set and to compare the data obtained from different microarray platforms [50]. Values of 1, 0, and −1 indicate perfect similarity, no similarity, and perfect inverse similarity, respectively. We should perhaps have expected the low correlation of correlations between the drug- and gene-based clusterings, but we did not. The reason for it appears to be that certain gene products, most prominently Pgp, have a disproportionate effect on activity profiles that cuts across organ of origin distinctions.

Dendrograms showing average-linkage hierarchical clustering of human cancer cell lines. (a) Cluster tree of the 60 cell lines based on their gene expression profiles for 1376 genes and 40 individual targets. 100% of the colon cancer lines (CO) (7/7) the central nervous system lines (CNS) (6/6), and the leukemias (LE) (6/6) clustered together. Seven out of 8 melanoma lines (ME) clustered together, the exception being the one reported to lack melanin production (LOX-IMVI). Seven out of 8 renal carcinoma lines (RE) clustered together, as did four out of 6 ovarian lines (OV). Non-small-cell lung cancer cells (LC) clustered on two different branches, and those of breast origin (BR) appeared most heterogeneous. The estrogen receptor-positive breast lines, T-47D and MCF7, appeared together and grouped with the colon lines, whereas the estrogen receptor-negative HS578T and BT-549 clustered with CNS malignancies. NCI/ADR-Res is of unknown origin (UK). (b) Cluster tree for the cells based on their patterns of sensitivity to 1400 compounds tested. The color of the cell line name indicates its assigned organ of origin classification. The distance metric used was (1 – Pearson correlation coefficient). ∗ Indicates two cell lines (MDA MB435 and MDA-N) with the gene expression and drug sensitivity signatures of melanotic melanoma but derived from a pleural effusion of a patient with breast cancer. Modified from [47].
Fig. 4 shows a CIM that summarizes all possible pairwise relationships between the gene database and a set of 118 drugs of putatively known mechanism of action. Each patch of color represents a story – which may be causally interesting, epiphenomenal, or statistical coincidence. There is clearly not sufficient statistical power to eliminate most of the false positive associations without losing most of the true positive ones. Hence, we must generally consult the literature and public databases for clues to determine which relationships are worth pursuing.
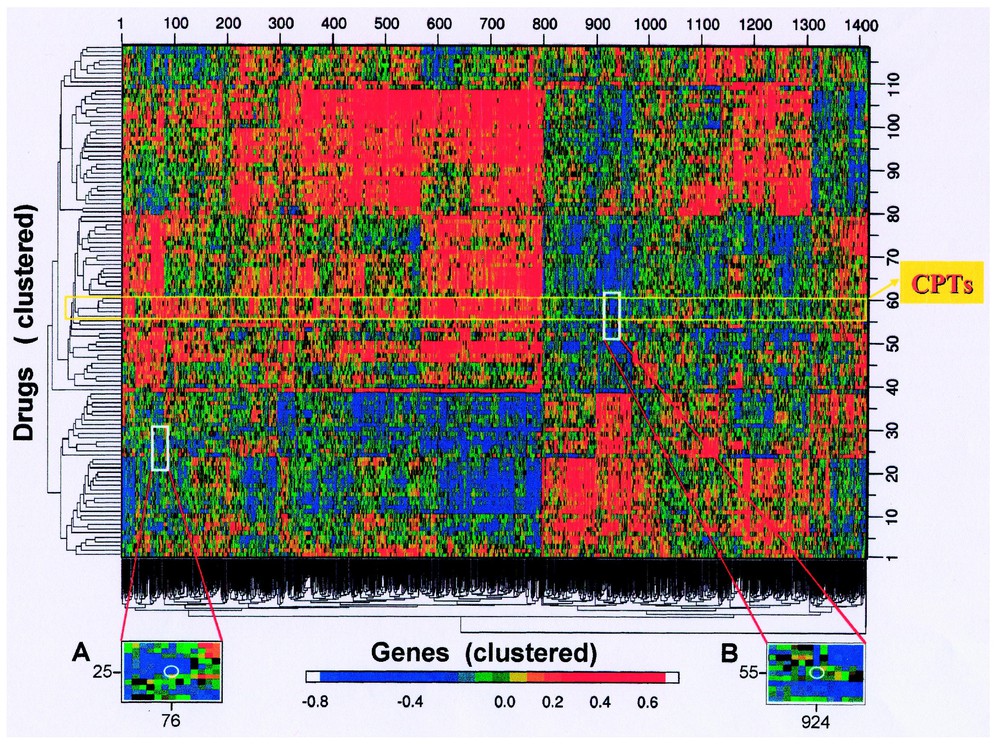
Clustered image map (CIM) relating activity patterns of 118 tested compounds to the expression patterns of 1376 genes in the 60 cell lines. Included in addition to the gene expression levels are data for 40 molecular targets assessed one at a time in the cells. A red point (high positive Pearson correlation coefficient) indicates that the agent tends to be more active (in the two-day assay) against cell lines that express more of the gene; a blue point (high negative correlation) indicates the opposite tendency. Genes were cluster-ordered on the basis of their correlations with drugs (mean-subtracted, average-linkage clustered with correlation metric); drugs were clustered on the basis of their correlations with genes (mean-subtracted, average-linkage clustered with correlation metric). Sharp edges of the colored patches reflect deep forks in the corresponding cluster tree. The position of the topoisomerase 1 inhibitor camptothecin (and its analogues) is indicated. Insert A shows a magnified view of the region around the point (white circle) representing the correlation between the dihydropyrimidine dehydrogenase gene and 5-fluorouracil. Insert B is an analogous magnified view for the asparagine synthetase gene and the drug l-asparaginase. Modified from [47].
2.4.2.2.2 Gene expression profiling by Affymetrix oligonucleotide chip.
The methods used have been described previously [48]. Very briefly, mRNA was obtained from the cells [47] and used to prepare biotinylated cDNA, which was hybridized to Hu6800 arrays (Affymetrix, Santa Clara, CA). The resulting cell clusters generally reflected what we had found with the cDNA arrays. We then cross-compared the oligonucleotide and cDNA array data to generate a robust database of >1600 transcripts for which results from the two very different technologies are reasonably concordant across the 60 cell types [50]. That ‘mutually validated’ database has proved particularly useful when we want a firm statistical basis for further analyses.
2.4.2.3 The bioinformatics of transcript profiling.
Anyone who does gene expression profiling (or similar omic experiments) for molecular targets finds that most of the time and energy are spent after the experiment – in statistical analysis of the data and then in biological interpretation. The problems are particularly acute in integromic studies because we are trying to integrate so many types of information – at the DNA, RNA, protein, functional, and pharmacological levels. Motivated by the needs of our experimental program, we have developed a number of algorithms and computer program packages to assist in the analysis and interpretation steps. These programs, publicly available at http://discover.nci.nih.gov, are proving useful to others as well.
CIM-Miner generates color-coded Clustered Image Maps (CIMs) (also called clustered heat maps) to represent ‘high-dimensional’ data sets such as gene expression profiles. We introduced CIMs in the mid-1990's for data on drug activities, target expression levels, gene expression values, and proteomic profiles [24,25,51]. The clustering of both axes (or sometimes only one if there is another organizing principle for the second axis) puts like together with like to create patterns of color. A program for producing CIMs can be found at http://discover.nci.nih.gov. Each patch of color in a CIM (e.g., in Fig. 4) represents a possible story. But how can we determine whether a patch represents a causally interesting story, an epiphenomenal correlation (which still may identify a useful molecular marker), or statistical coincidence? As noted in the last section, the usual answer is that we must consult the biomedical literature and public databases. Since that can be a tedious process, we developed a program package called MedMiner for efficient searching and organization of the literature on complex gene, gene–gene, and gene–drug relationships.
MedMiner[52] publicly available at http://discover.nci.nih.gov) uses a combination of GeneCards from the Weizmann Institute, PubMed from the National Library of Medicine (NLM), syntactic analysis, truncated-keyword filtering of relationals, and user-controlled sculpting of Boolean queries to identify key sentences from pertinent abstracts. Those sentences are then organized so that the user can access the most pertinent ones directly by clicking on a relational relevance-term. Whole abstracts of interest can then be accessed quickly through a direct link to PubMed and dropped into a ‘shopping basket’ for display or for automated entry into a library under EndNote (ISI ResearchSoft, Berkeley, CA) or other bibliographic software. Experienced users have estimated that MedMiner speeds up 5- to 10-fold the process of capturing and organizing the literature from PubMed searches on gene–gene and gene-drug relationships.
MatchMiner[53] publicly available at http://discover.nci.nih.gov provides a solution to the major problem of translating among various gene identifier types for lists of hundreds or thousands of genes. Currently included are GenBank accession numbers, IMAGE clone ids, common gene names, gene symbols, UniGene clusters, FISH-mapped BAC clones, Affymetrix identifiers, and chromosome locations. The LookUp function in MatchMiner makes such translations, providing the user with diagnostics that indicate how the translation was done. The Merge function finds the intersection of two lists of genes, which may be designated by either the same or different identifiers. This functionality is particularly important to our ‘integromic’ efforts to meld information from the variety of different data types on the NCI-60.
GoMiner[54] publicly available at http://discover.nci.nih.gov provides an answer to the vexing question, “Now that I've done the gene expression experiment and identified a set of ‘interesting’ genes, what do those genes mean biologically?” To address that question, GoMiner batch-processes and organizes lists of thousands or tens of thousands of genes and provides two fluent, robust visualizations of the genes embedded within the framework of the Gene Ontology hierarchy. One is a tree-like structure; the other is a ‘directed acyclic graph’. GoMiner calculates summary statistics indicating for each GO category whether it is enriched with, or depleted of, ‘interesting’ genes and gives p-values with which to assess the statistical robustness of the enrichment or depletion.
LeadScope/LeadMinerTM[55] provides a firm link between molecular markers and the drug discovery process. More precisely, it links gene expression profiles for the NCI-60 (or other cell panels used for screening) to a set of 27 000 chemical substructure descriptors of the compounds tested against the cells. One can use it, for example, to identify substructure classes that are found in compounds active in the screen against cell types that express large amounts of a particular gene. That is precisely what a medicinal chemist or researcher designing a directed combinatorial library would like to be able to do in pursuing pharmacogenomic goals.
2.4.3 Pharmacogenomic use of NCI-60 transcript profiles: An example
The white rectangle on the gene expression vs. drug sensitivity CIM in Fig. 4 points to a story with likely causal significance on the basis of literature information. That story [47] involves the gene asparagine synthetase and the bacterial enzyme-drug l-asparaginase. Many acute lymphoblastic leukemias (ALL) lack asparagine synthetase and therefore must scavenge exogenous l-asparagine to survive. This dependence is exploited by treating ALL with bacterial l-asparaginase, which depletes extracellular l-asparagine and selectively starves the cancer cells. Fig. 5 shows the relationship between l-asparaginase activity and asparagine synthetase expression across the NCI-60. As might have been predicted on the basis of the above mechanism, there was a statistically robust negative correlation (−0.44; bootstrap 95% confidence interval −0.59 to −0.25) between expression of the asparagine synthetase gene and l-asparaginase sensitivity in the 60 cell lines [47]. Although statistically robust, the correlation was only moderately strong. We knew, however, to focus specifically on the leukemic subpanel, and in that case the correlation was a striking −0.98 (bootstrap 95% confidence interval −1.00 to −0.93). This value survived even a Bonferroni correction for statistical multiple comparisons. Furthermore, the two ALL-derived lines expressed the lowest levels of asparagine synthetase mRNA and were the most sensitive to l-asparaginase, as might have been predicted. These results supported the possible use of asparagine synthetase as a marker for clinical decisions about l-asparaginase therapy [47].
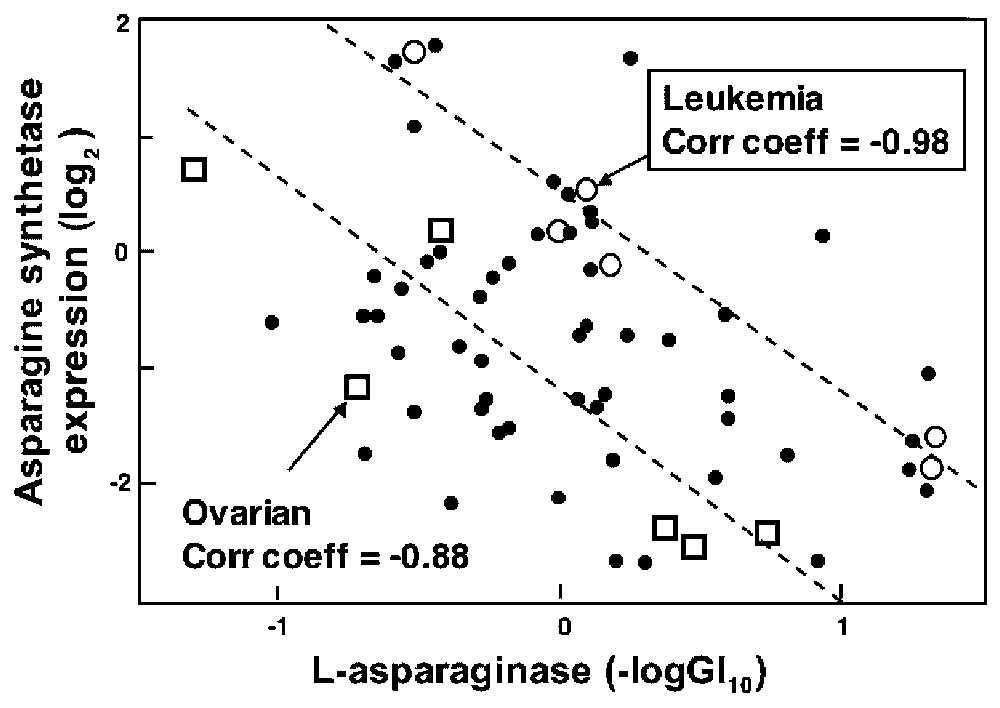
Relationship between asparagine synthetase expression levels and chemosensitivity of the NCI cell lines to l-asparaginase. Main effects have been removed for both cells and drugs. Hence, a negative log(GI50) value of 1 for sensitivity indicates a 10-fold higher than average sensitivity of the cell line to the agent. The asparagine synthetase expression level is plotted as the abundance of the asparagine synthetase transcript, relative to its abundance in the reference pool of 12 cell lines. A value of +2 indicates 4-fold higher expression than in the reference pool. The large circles indicate leukemia cell lines. The linear regression line (correlation coefficient=−0.98; P value <0.01) was fitted to the leukemia data. Modified from [47].
We then asked whether any other cell line panel showed similar correlation. The answer was ‘yes’, though not as strongly. The correlation coefficient for the ovarian lines was −0.88 (confidence interval −0.23 to −0.99) [47]. Early clinical trials done with an assortment of solid tumors showed occasional responses to l-asparaginase in melanoma, chronic granulocytic leukemia, lymphosarcoma, and reticulum cell sarcoma but not in other tumor types (see [47] for references). The microarray findings, however, support a closer look at l-asparaginase therapy for solid tumors, particularly for a subset of ovarian cancers low in asparagine synthetase. Further studies of this correlation are underway in collaboration with D. von Hoff (Arizona Cancer Center). The preferred material for a clinical trial would be the polyethylene glycol-modified forms of l-asparaginase, which shows much better pharmacokinetic and immunological properties than does the native bacterial form of the enzyme.
3 Concluding remarks
Pharmacogenomic profiling – or, in accord with the title of this contribution, should we call it ‘pharmacotranscriptomic profiling – holds undeniable promise for molecular subsetting of patients and for individualization of therapy. Much of the research to realize those aims can be done with clinical materials, rather than cultured cells, if a number of purely technical challenges are overcome. But the limitation of clinical tumors that cannot be overcome, is this: they have not been exposed to large numbers of chemical compounds one at a time and independently under well-defined experimental control.
Acknowledgements
We are grateful to E.A. Sausville, A. Monks, D.A. Scudiero, K.D. Paull, and others in the NCI DTP, whose work over the years and in collaboration with us has made these studies of the NCI-60 cell lines possible. We thank D.A. Ross and others in the Brown/Botstein laboratory at Stanford University and J. Staunton and others in the Golub/Lander group at the Whitehead Institute for collaborations that generated the transcript profiles discussed here. We are most grateful to P. Blower and colleagues at LeadScope, Inc. for collaborating in development of the LeadScope/LeadMiner program package; to M. Wang's group at the Georgia Institute of Technology and Emory University for major contributions to the GoMiner program; to J. Riss and J.C. Barrett at the NCI, also for contributions to GoMiner. We particularly thank the many members of the NCI Laboratory of Molecular Pharmacology, past and present, and SRA International staff (on contract for software development) whose efforts in recent years have made the work described here possible. These developments have been made possible largely by funding from the Center for Cancer Research, NCI.