1 Introduction
Turmeric (Curcuma longa), a highly important plant in the family Zingiberaceae, comprises about 100 accepted species [1]. Its rhizome has been widely used in traditional Asian medicine for numerous hepatic disorders [2]. C. longa has been used as a therapeutic herb over centuries in traditional medicinal systems. Several pharmacological effects of turmeric as anti-ulcerogenic, anti-inflammatory, anti-tumour and anti-cancerous ones, have been reported earlier [3–6]. In Ayurveda, C. longa is used to strengthen the overall energy of the body, relieve gas, dispel worms, improve digestion, regulate menstruation, dissolve gallstones, and relieve arthritis [1]. microRNAs (miRNAs) are approximately 20–22 nucleotide long and endogenous small RNA molecules. Post-transcriptional regulation of the gene expression made by miRNAs take part in almost all biological and metabolic processes of plants and animals [7–10]. Growing evidences suggests their role in signal transduction, leaf, and root development, reproduction and in response to environmental biotic and abiotic stress [11–14]. Computational screening of potential miRNAs is utilized frequently to avoid the high cost, lower expression and time consuming process of experimental techniques. It has also been successful for the discovery of conserved miRNAs as well as new miRNAs [15–19].
Plants produce a large number of secondary metabolites that possess a lot of biological activities. These metabolites protect the host plant against competitors, predators, pathogens, and ultraviolet light, helping them to adapt to the environmental niche, and some of those biologically active metabolites have medicinal properties [20]. In the case of turmeric, curcumin is the most active bioactive compound. It has been reported to alter the expression profiles of microRNAs in human pancreatic cancer cells [21]. Except for curcumin, numerous other secondary metabolites are reported to have several therapeutic properties. A total of 720 compounds, including 102 diphenylalkanoids, 19 phenylpropene derivatives, 529 terpenoids, 15 flavonoids, 7 steroids, and 3 alkaloids were already reported in Curcuma [1]. Its diterpenoid compound C fought against gastric cancer [22]. The role of sesquiterpenes from its essential oil was reported to have inhibitory effects on nitric oxide production [23,24]. A large amount of flavonoid and alkaloid reported from turmeric suggests that they contribute to its antioxidant activities [25].
miRNAs have been demonstrated to play an active role in secondary metabolism regulation [26]. The regulation of secondary metabolites by an endogenous target mimic [eTM] of particular miRNAs has been reported as a new approach. This technique has been used to enhance the production of nicotine in Nicotiana tabacum [27]. This was the first successful as well as initial story for the regulation of secondary metabolite biosynthesis by a miRNA-eTM regulatory module in plants [27]. More efforts are needed to identify a significant role of miRNAs in other plants.
Despite the immense importance of turmeric as a culinary and medicinal plant, no miRNAs have been reported in miRBase [28–30]. In addition to other work related to miRNAs and their activity in Curcuma [31–34], we have identified miRNAs from turmeric showing regulation for different secondary metabolic pathways as well as their involvement in growth and development process.
2 Materials and methods
2.1 miRNA and target prediction
ESTs, nucleotide and SRA (SRX627351) data were downloaded from the public database NCBI (http://www.ncbi.nlm.nih.gov/). For the identification of putative miRNA and target candidates, C-mii [35] was used. Filtering criteria were also applied to narrow down the false predictions. For miRNA, the prediction criteria were as follows: (a) the length of mature miRNA should be in range of 19–25 nucleotides; (b) more than four mismatches were not allowed between candidate mature miRNAs and known mature miRNA; (c) the number of flank bases should not be more than 3; (d) the AU content should be higher than the GC content; (e) a smaller number (≤ 1) of bulges and their size; (f) MFEI values should be highly negative (≥ 0.90 (–kcal/mol)). To enhance the prediction probability, miRNA-dis [36] and imiRNA-PseDPC [37] were used. Thus, the results were again verified at the sequential and structural levels, as miRNA-dis used structural-order information into the prediction, while imiRNA-PseDPC used a formulated novel feature vector based on pre-miRNA sequence.
The target prediction was performed by two channels using turmeric data. The first one was for turmeric miRNAs, and the second one was for all miRNAs reported in miRBase. The targets were predicted using the following criteria: (a) more than four mismatches were not allowed in complementary sites between miRNA and mRNA levels; (b) no mismatches were allowed at positions 10 and 11.
The lncRNAs of Arabidopsis thaliana reported in CANTATAdb [38] was used as a reference data set for the prediction of lncRNA targets of the predicted miRNAs by psRNATarget [39]. A biological network was constructed by Cytoscape [40].
2.2 Pathway and phylogenetic analysis of the identified miRNA
Selected target transcripts were considered for functional annotation. Annotation and pathway analyses were performed by Blast2GO [41] and KASS sever (http://www.genome.jp/tools/kaas/), respectively. The phylogenetic tree was constructed using maximum likelihood method based on Kimura2-parameter substitution model from MEGA 6.0 [42].
3 Results
3.1 miRNA identification and characterization
The prediction results showed 145 miRNA families by C-mii using default parameters. The number of predictions was reduced to 50 miRNA candidates by applying the above-mentioned filtering criterion. To further improve the accuracy, filtered miRNA precursors were again cross validated at sequence and structure level by using imiRNA-PseDPC and miRNA-dis. After removing the redundancy and false positive values, the results were optimized with eighteen miRNAs, which were finally considered for the study.
3.1.1 Length variation
A variation from 20 to 22 nucleotides was observed for mature miRNA sequences. On the other hand, precursor miRNA candidates showed a large variation within the range of 38 to 574 nucleotides (Table 1). The finding was in accordance with earlier reports [36,43,44].
Characterization of identified Curcuma miRNAs.
miRNA | Plant family | NB | BZ | PL | MFE (-kcal /mol) |
GC Content | AU Content | All Content | Predicted miRNA sequence | miRNA-dis results | imiRNA-PseDPC |
miR156a | Arabidopsis thaliana | 1 | 1 | 82 | 50.9 | 50 | 50 | A(17), U(24), G(22), C(19), N(0) | 5’:UGACAGAAGAUAGAGAGCAC:3’ | Real Pre-miRNA | Real Pre-miRNA |
miR157d | Arabidopsis thaliana | 1 | 1 | 82 | 50.9 | 50 | 50 | A(17), U(24), G(22), C(19), N(0) | 5’:UGACAGAAGAUAGAGAGCAC:3’ | Real Pre-miRNA | Real Pre-miRNA |
miR159a | Arabidopsis thaliana | 0 | 0 | 167 | 74.9 | 47.9 | 52.1 | A(42), U(45), G(39), C(41), N(0) | 5’:CUUGGACUGAAGGGAGCUCCU:3’ | Real Pre-miRNA | Real Pre-miRNA |
miR170 | Arabidopsis thaliana | 0 | 0 | 85 | 30.9 | 35.29 | 64.71 | A(24), U(31), G(16), C(14), N(0) | 5’:CGAUUGAGCCGUGCCAAUAUC:3’ | Real Pre-miRNA | Real Pre-miRNA |
miR171b | Arabidopsis thaliana | 0 | 0 | 91 | 36.1 | 34.06 | 65.94 | A(28), U(32), G(17), C(14), N(0) | 5’:UUGAGCCGUGCCAAUAUCAAA:3’ | Real Pre-miRNA | Real Pre-miRNA |
miR2936 | Arabidopsis thaliana | 0 | 0 | 241 | 154.5 | 37.75 | 62.25 | A(71), U(79), G(46), C(45), N(0) | 5’:CUAGAGAGAGAGAAAAAAGGCG:3’ | Real Pre-miRNA | Real Pre-miRNA |
miR319a | Arabidopsis thaliana | 0 | 0 | 169 | 75.1 | 47.33 | 52.67 | A(43), U(46), G(39), C(41), N(0) | 5’:UUGGACUGAAGGGAGCUCCUU:3’ | Real Pre-miRNA | Real Pre-miRNA |
miR396a | Arabidopsis thaliana | 0 | 0 | 80 | 30.5 | 40 | 60 | A(22), U(26), G(18), C(14), N(0) | 5’:UUCCACAGCUUUCUUGAACUG:3’ | Real Pre-miRNA | Real Pre-miRNA |
miR5015b | Arabidopsis thaliana | 0 | 0 | 56 | 15.7 | 28.57 | 71.43 | A(16), U(24), G(12), C(4), N(0) | 5’:UUUGUUGUUGUUGUUGUUGUU:3’ | Real Pre-miRNA | Real Pre-miRNA |
miR5021 | Arabidopsis thaliana | 1 | 1 | 37 | 14.4 | 32.43 | 67.57 | A(12), U(13), G(7), C(5), N(0) | 5’:UCAGAAGAAGAAGAGGAAGA:3’ | Real Pre-miRNA | Real Pre-miRNA |
miR5649a | Arabidopsis thaliana | 1 | 1 | 82 | 23.5 | 28.04 | 71.96 | A(24), U(35), G(15), C(8), N(0) | 5’:UUUGAGUAUGUUGUUUUCUAU:3’ | Real Pre-miRNA | Real Pre-miRNA |
miR1424 | Oryza sativa | 1 | 1 | 39 | 16.7 | 46.15 | 53.85 | A(8), U(13), G(10), C(8), N(0) | 5’:AUGCACACUGAUCCUGUUGCU:3’ | Real Pre-miRNA | Real Pre-miRNA |
miR1435 | Oryza sativa | 0 | 0 | 62 | 17.8 | 27.41 | 72.59 | A(25), U(20), G(9), C(8), N(0) | 5’:UUUCUUAAGUCGAAUUUUAA:3’ | Real Pre-miRNA | Real Pre-miRNA |
miR2105 | Oryza sativa | 0 | 0 | 56 | 14 | 26.78 | 73.22 | A(14), U(27), G(8), C(7), N(0) | 5’:UUGGGUAGUGAAUGAUUCAU:3’ | Real Pre-miRNA | Real Pre-miRNA |
miR2866 | Oryza sativa | 0 | 0 | 46 | 13.2 | 30.43 | 69.57 | A(16), U(16), G(8), C(6), N(0) | 5’:UUUUAUUUGUGUUCAGCAAC:3’ | Real Pre-miRNA | Real Pre-miRNA |
miR2919 | Oryza sativa | 0 | 0 | 37 | 33.4 | 67.56 | 32.44 | A(7), U(5), G(13), C(12), N(0) | 5’:AAAGGGGGGGGGGGGGAAA:3’ | Real Pre-miRNA | Real Pre-miRNA |
miR535 | Oryza sativa | 0 | 0 | 81 | 48.4 | 51.85 | 48.15 | A(17), U(22), G(23), C(19), N(0) | 5’:CGACAACGAGAGAGAGCACGU:3’ | Real Pre-miRNA | Real Pre-miRNA |
miR5538 | Oryza sativa | 0 | 0 | 574 | 471.1 | 41.28 | 58.72 | A(173), U(164), G(121), C(116), N(0) | 5’:AUCCAUCUCAAUCACUUGCUGC:3’ | Real Pre-miRNA | Real Pre-miRNA |
3.1.2 AU and GC contents
Higher AU content is a feature to distinguish miRNA from other non-coding RNAs. We observed a high AU content, which was in the range from 32.00 to 73.22 (Table 1), with an average of 59.84, as reported in earlier studies [19,43,45].
3.1.3 Position-specific nucleotide dominance
Uracil was observed as the dominant nucleotide at the first position of mature miRNA sequences (Fig. 1a) as reported earlier [17,18,46]. Uracil (61.11%) was considered as most dominant nucleotide in pre-miRNA also [47–50]. Guanine (44.44%) was the preferred base for the 4th, 8th, and 16th positions. The dominancy of adenine (61.11%) was high for the 5th position (Fig. 1b). Overall, the average nucleotide composition of turmeric miRNAs was found to be 26.73% adenine, 17.82% cytosine, 27.63% guanine, and 27.82% uracil, as reported in an earlier study [46].

(a) Position-specific nucleotide dominance and (b) nucleotide percentage analysis in the identified turmeric mature miRNA sequences.
3.1.4 MEF and MFEI
MFE (Minimum Folding Energy) and MFEI (Minimum Folding Energy Index) values are good indicators of the structural stability of the miRNAs. MFE of the identified C. longa miRNAs showed a range from 13.2 to 471.1 (–kcal/mol), with an average of about 65.11 (–kcal/mol). MFEI is another important criterion to distinguish miRNAs among all non-coding RNAs. The observed number of MFEI of the predicted miRNAs were also highly negative in the range of 0.92 to 1.98 (–kcal/mol) (Fig. 2) with the average of 1.15 (–kcal/mol) which were relatively higher than in earlier reports ([15,19]; B. H. [51]). In this study, the stability of the secondary structure of predicted pre-miRNAs was also supported by very less number of bulges and their size (0–1). Precursor miRNA sequences and their secondary structures are presented in Supplementary data 1.
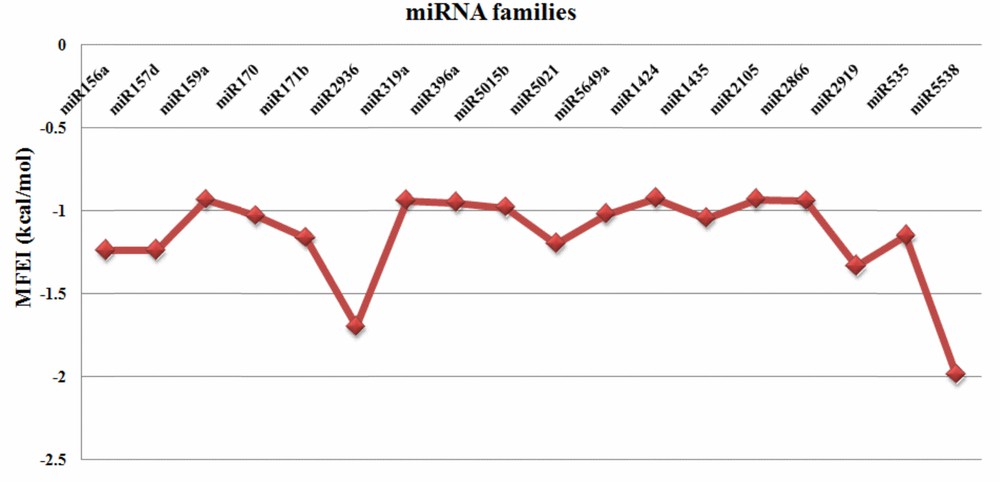
MFEI values of the predicted turmeric miRNAs.
3.2 miRNA target Identification
Growing evidence suggests that miRNAs can regulate the expression level of target transcripts by binding their complementary sites, whether they are coding or non-coding [52]. LncRNAs are the emerging non-coding transcripts reported to be regulated by miRNAs in plants as target mimic [53]. In this study, we have predicted both coding and non-coding (lncRNA) targets of Curcuma miRNAs. A total of 238 targets were functionally annotated for 16 predicted miRNAs (Supplementary data 2) with 74 lncRNA targets of seven miRNA family (Supplementary data 3) (Fig. 3). Both results indicated that the maximum numbers of target transcripts were regulated by miR5015 and miR5021. Target prediction results also deciphered the involvement of predicted miRNAs in various processes of plant development.
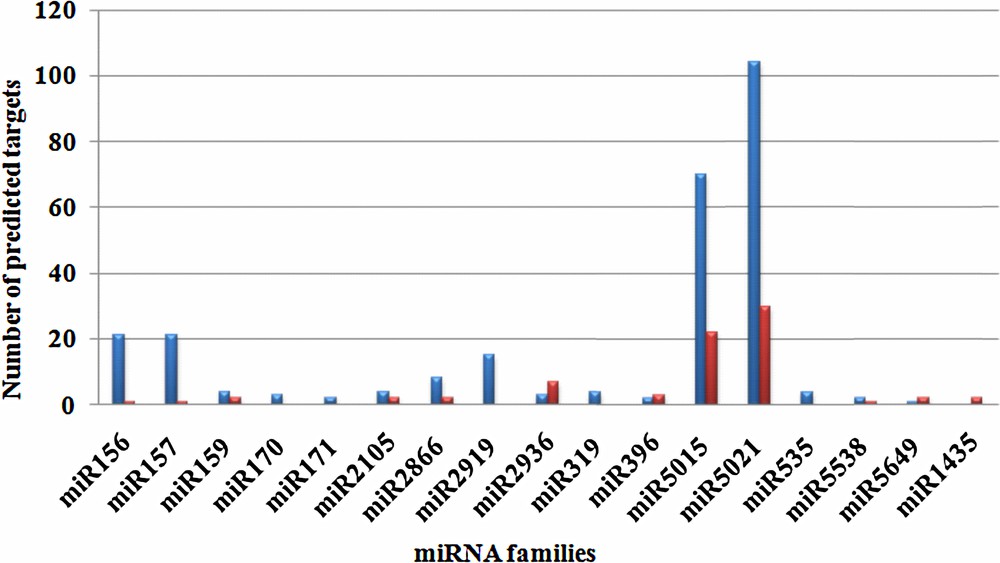
Numbers of predicted target transcript regulated by the predicted miRNAs.
3.2.1 miRNAs involved in the rhizome development
miRNAs are known to play an important role in root development [54]. miR156 genes are differentially expressed in specific cells/tissues of lateral roots. Plants over-expressing miR156 produce more lateral roots, whereas reduced miR156 levels lead to fewer lateral roots [55]. In our study, miR156 showed response for squamosa promoter-binding-like protein (SPL) as reported earlier [54]. Auxin response transcription factors (ARFs) are known to control root development in many plants [14,56,57]. The regulation of ARFs by miR5015 and miR5021 was observed (Supplementary data 2).
3.2.2 miRNAs involved in transcriptional regulation
Several transcription factor viz., AP2, RAX3, MYB factors (MYB86), basic helix-loop helix (bhlh) and WRKY regulated by miR5015 and miR5021 (Supplementary data 2) were identified. miR159 and miR319 were observed to modulate the expression of TCP2 TF, which is reported to control the leaf development and leaf morphogenesis [58]. Our results revealed the significant role of the miR5015 and miR5021 in modulating the expression of several TFs involved in different biological processes of turmeric.
3.2.3 miRNAs involved in secondary metabolic pathways
Curcumin is known as an active secondary metabolite of curcuma. Several other secondary metabolites were reported to have therapeutic properties as its diterpenoid compound C fought against gastric cancer [22]. The essential oils of curcuma and paclitaxel are reported to enhance the apoptosis of the SKOV3 human ovarian cancer cell line [59]. Sesquiterpenes from its essential oil were reported to have inhibitory effects on nitric oxide production [23,24]. In this study, we found two miRNAs (miR5021 and miR5649) to be involved in the backbone synthesis of terpenoid by modulating the expression of geranylgeranyl-diphosphate synthase (Table 2). In other reports, miR5021 was mentioned to be involved in terpenoid backbone synthesis [16,18,60].
miRNAs involved in secondary metabolic pathways.
miRNA | Target | Enzyme encoding | Pathways |
miR5649a | Geranylgeranyl-diphosphate specific | EC: 2.5.1.85, EC: 2.5.1.84 | Terpenoid backbone biosynthesis |
miR5021 | Geranyl-diphosphate synthase | EC: 2.5.1.1 | |
miR5021 | 1-deoxy-d-xylulose-5-phosphate synthase (DXS) | EC: 2.2.1.7 | |
miR5649a, miR5021 | Geranylgeranyl-diphosphate synthase | EC: 2.5.1.29 | |
miR2919 | Flavanone synthase | EC: 2.3.1.74 | Flavonoid biosynthesis |
miR5021 | Aromatic-amino-acid transaminase | EC: 2.6.1.57 | Isoquinoline alkaloid biosynthesis |
miR5021 | Aspartate transaminase | EC: 2.6.1.1 |
Apart from terpenoids, a large amount of flavonoids and alkaloids has been reported in turmeric, contributing to its antioxidant activities [25]. The results indicate the regulation of miR2919 for flavonoid biosynthesis by modulating the expression of flavanone synthase (EC: 2.3.1.74). Isoquinoline alkaloid biosynthesis was observed to be regulated by miR5021.
3.2.4 miRNAs involved in growth and development
Our results indicate the role of predicted miRNAs in the different type of cellular and biological process (Fig. 4). The annotation results revealed that a high number of target transcripts had a role in response to the stimulus. Most of the targets were involved in the signalling, localization, and reproductive processes. Pathway analysis suggested the possible role of miR5015 in the biosynthesis of antibiotics, thiamine, pyrimidine, purine, starch and sucrose metabolism, lysine, and sphingolipid metabolism (Supplementary data 3).
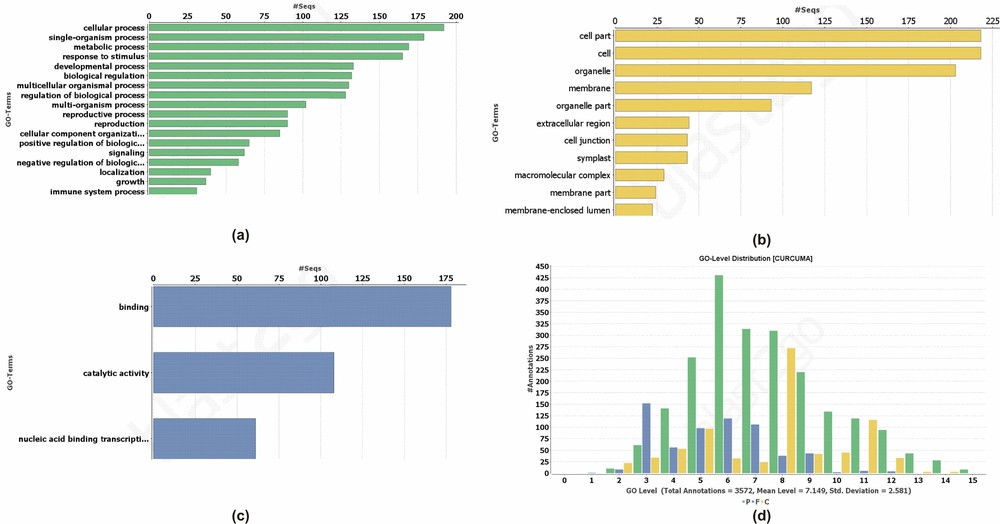
Function of the target transcripts regulated by the identified miRNAs (a) biological, (b) molecular, (c) cellular, and (d) Go level distribution of the predicted target transcripts.
3.2.5 Co-regulated targets by miRNA families
A biological network was constructed to analyse the miRNA-mediated gene regulation and to facilitate the visualization of combinatorial regulation. The difference between the distributions of MFE values of miRNAs and their targets demonstrated that predictions with low MFE values occur more often and could be used for prioritizing miRNA target predictions [61]. After removing the duplicate targets, the miRNA-mediated gene regulatory network of six miRNA families and their 86 targets was constructed based on attributes of MFE (Fig. 5). It was observed that 19 predicted and annotated targets were co-regulated by more than one miRNA family. miR156 and miR157 were observed to regulate a maximum number of annotated targets (10). The smaller variation was observed in the comparative distribution of MFE values for common targets by miR156 and miR157 (Fig. 6). Only one target was regulated by miR170 and miR171, which may be due to the closeness of some families, as miR156 and miR170 share similarities with miR157 and miR171, respectively. miR5021 showed dominancy for its targets with relatively high MFE values with other miRNAs (miR5649a, miR5015b and miR535). Network analysis revealed the co-regulation of two lncRNA targets. One shared the same UPE value, while for the other target (CNT0042900), dominancy of miR5021 was observed.
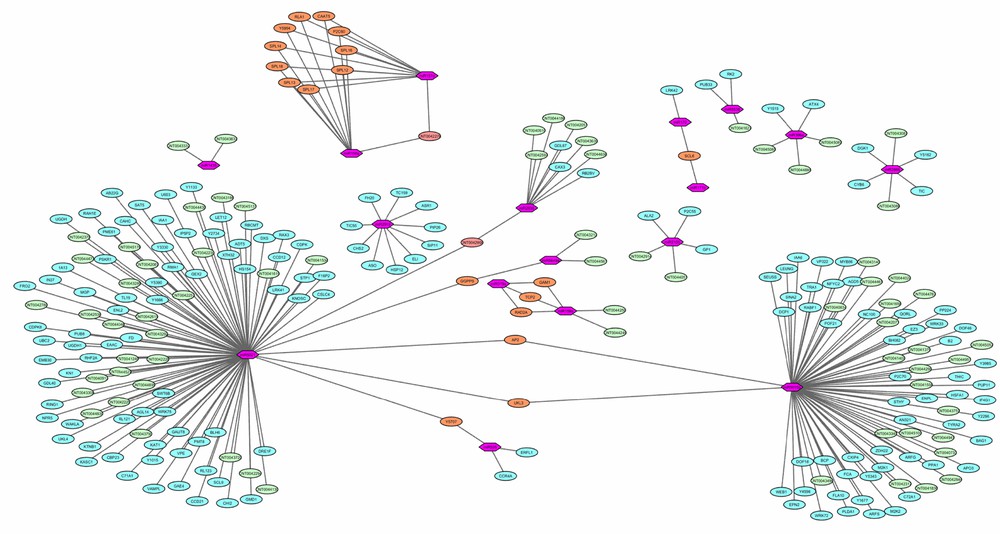
miRNA-mediated gene regulatory network (in this image, the pink hexagons stand for miRNA, the blue (lncRNAs) and green (coding transcripts) ellipses stand for the predicted targets, the orange ellipse stands for the common predicted target between two or more than two miRNAs and the calculated energy value was used as weight between miRNAs and their predicted targets).
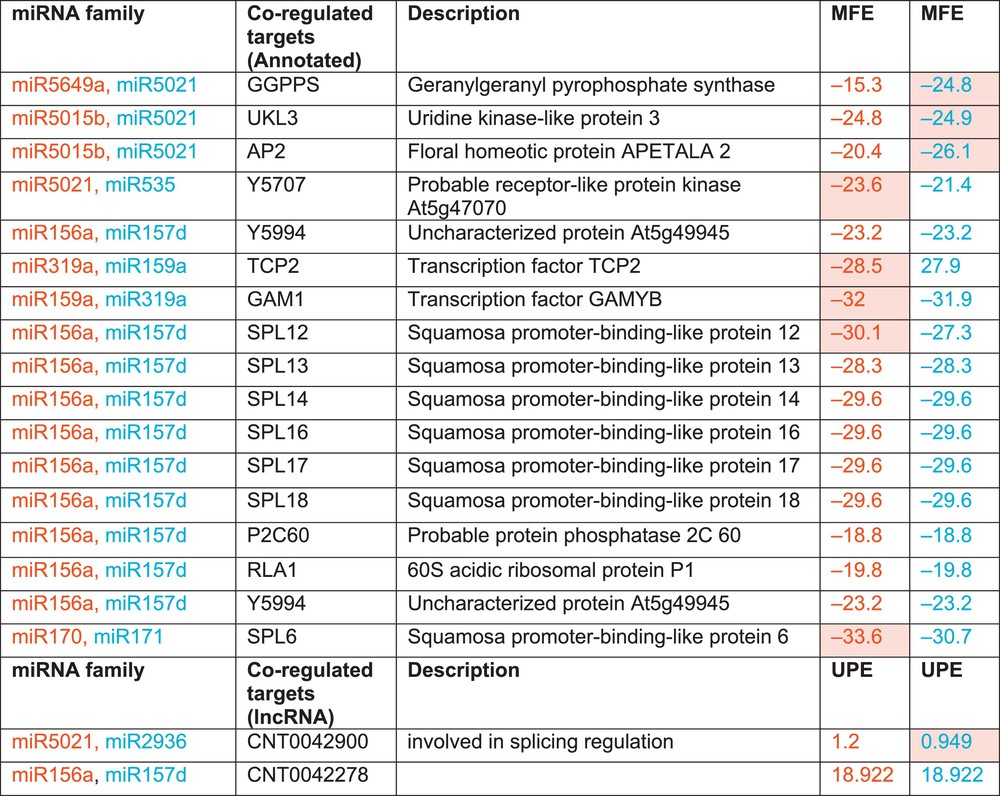
Co-regulated targets by miRNA families and their respective calculated energies. The respective colours represent the energy values (MFE/UPE) of the relative miRNA families.
3.2.6 Predicted targets of turmeric for all reported miRNAs in miRBase
To maximize the target prediction probability of turmeric, the target prediction module of C-mii was used. All reported miRNAs of miRBase (1241 miRNA families) were selected as source against the Curcuma transcripts (SRA, ESTs and nucleotide sequence of turmeric). A total of 16,908 target transcripts were predicted, which were involved in several biological processes. To illustrate the miRNA regulation in the secondary metabolites of turmeric, pathway analysis was also performed by KAAS server. We mainly analysed the miRNAs and targets involved in curcumin production. As per the reports, these compounds are derived from intermediates in the phenylpropanoid pathway, which are condensed with other molecules, derived in turn from the acetate and short and medium-chain fatty acid pathways [62]. Condensation of two molecules of p-coumaroyl-CoA with one molecule of malonyl- CoA could be give rise to the production of curcuminoids. trans-Cinnamate 4-monooxygenase (CYP73A) [EC:1.14.13.11] was reported to be involved in the conversion of trans-cinnamate/cinnamoyl-CoA into p-coumaroyl-CoA (Fig. 7). In this analysis, the regulation of CYP73A was observed by miR1168.2 of Chlamydomonas reinhardtii. 4-coumaroyl-CoA synthetase (4CL) [EC:6.2.1.12] was also observed to be regulated by miR156b (Arachis hypogaea) and miR1858 (Oryza sativa). The involvement of 4CL was reported for the conversion of an intermediate compound (cinnamoyl-CoA) of trans-cinnamate and p-coumaroyl-CoA. Our analysis revealed the probable involvement of three miRNAs (miR1168.2, miR156b and miR1858) in curcumin biosynthesis.
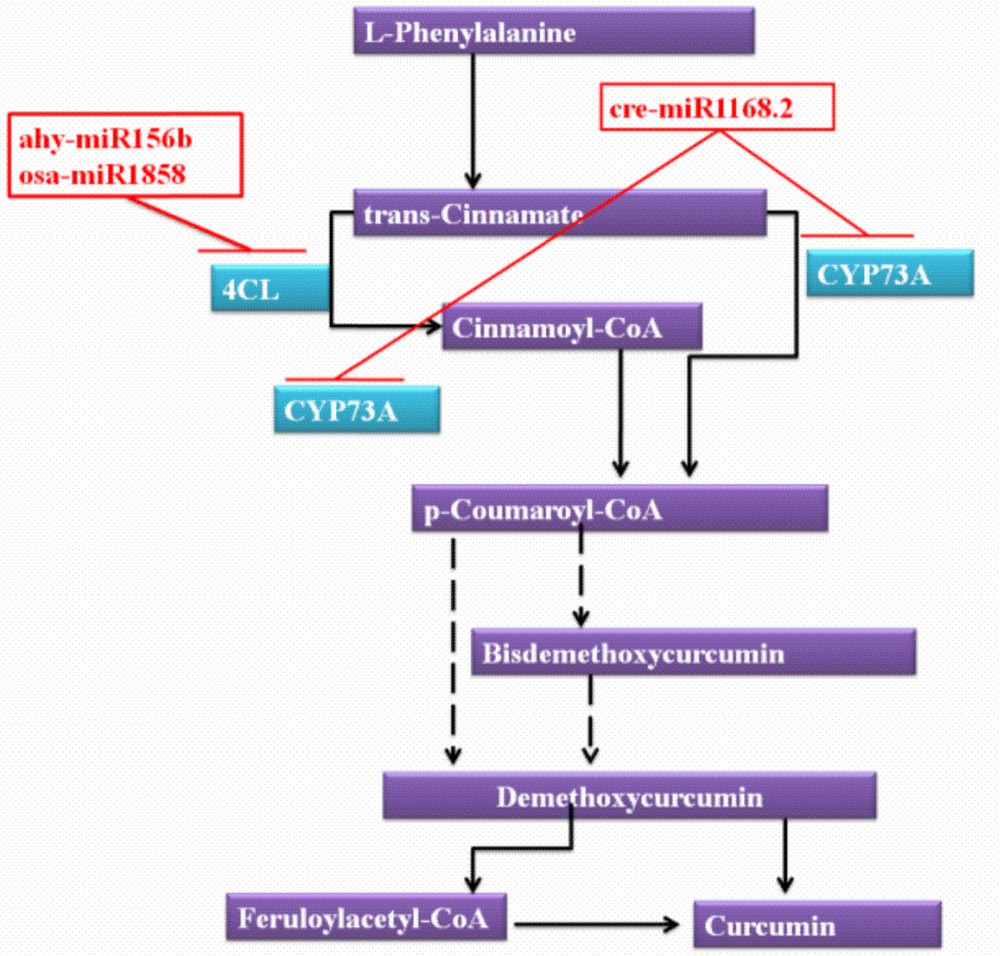
miRNAs involved in the curcumin biosynthesis pathway.
3.2.7 Phylogenetic analysis
Plant miRNAs are highly conserved in nature [10]. In this analysis, we constructed a phylogenetic tree of identified precursor miRNAs of turmeric. Sixteen miRNA families (miR156a, miR157d, miR159a, miR170, miR171b, miR2936, miR319a, miR396a, miR5649, miR1424, miR1435, miR2105, miR2866, miR2919, miR535, miR5538) were reported for the first time from Zingiberaceae as there is no report available for these families in miRBase. In the case for some miRNA families, very less data was available (reported only in Arabidopsis or Oryza), so they were not considered for phylogenetic analysis. A high percentage of similarity was observed among miR156-miR157 and miR170-miR171 during the blast search against miRBase. To reduce the complexity of the phylogenetic tree, only one miRNA candidate from each similar group was considered. Thus, phylogenetic analysis was performed for five miRNA families. The results revealed that all predicted miRNAs shared high percents of sequence similarity, which was supported by their bootstrap values within their nodes (Fig. 8). Phylogenetic analysis indicated that different miRNAs evolved differently at different rate, not only within the same species, but also in different plants [15].
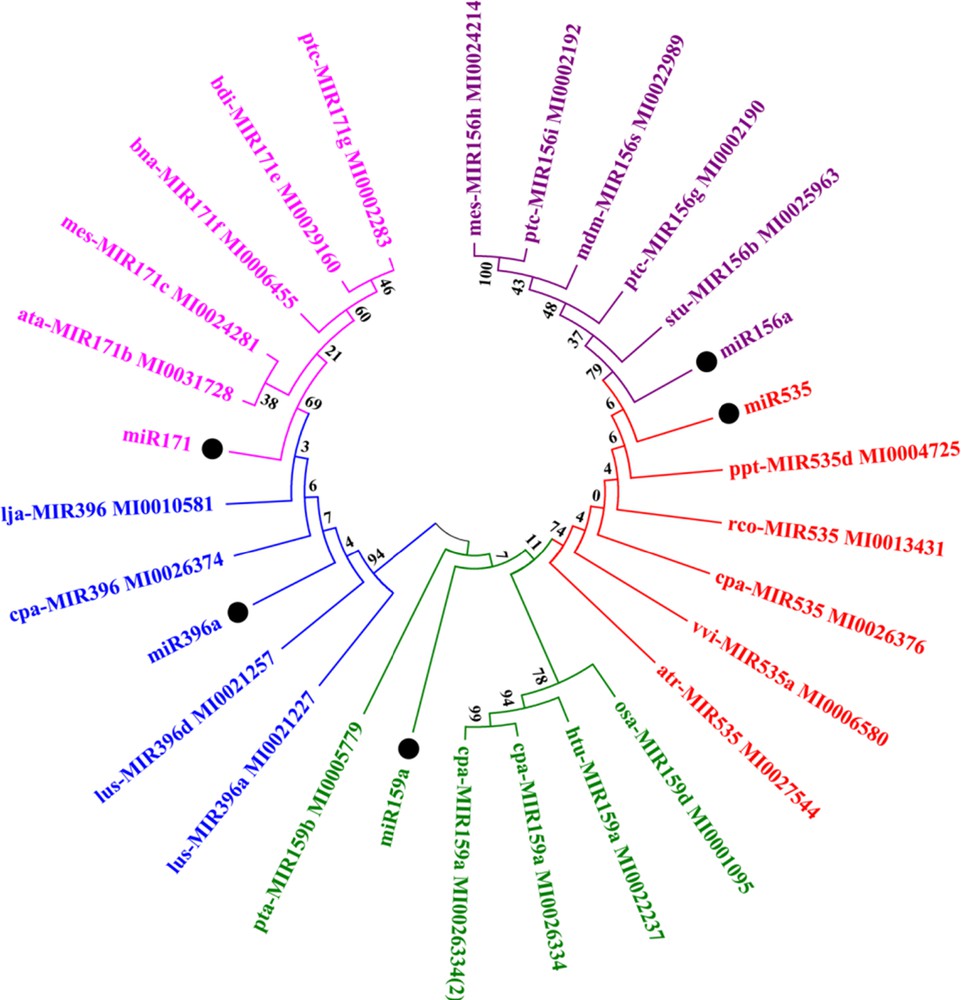
Phylogenetic analysis of the identified pre-miRNA sequence along with their closely related miRNA families. The precursor sequence of turmeric miRNAs appears as black circles. Each group of miRNA family was represented by a different colour.
4 Discussion
Plant miRNAs have high evolutionary conserved nature across the species [10,63], which also offers the possibility of miRNA identification in a new plant species through homologue identification [18]. Since no miRNAs have been reported in turmeric so far, we used a homology based approach to search for conserved miRNAs. Initially, based on a homology search approach, 145 miRNA families were predicted. To improve the accuracy and reduce the false positive results, major characteristics of pre-miRNAs were analysed at the sequential and structural levels. At the sequential level, length variation and position-specific dominance of miRNAs were analysed. The range of pre-miRNAs length was observed from 38 to 574. Two miRNAs (miR5021 and miR2919) were observed with 38 nt of pre-miRNAs. These precursor miRNAs were again evaluated based on distance structure status pairs by miR-dis. A total of 18 miRNAs were considered for the study. Uracil was the dominant base at the first position of mature miRNAs. The AU content was higher as compared to the GC content. A higher AU content may result in a less stable pre-miRNA secondary structure and thus be easier to be processed into mature miRNA by the RISE complex (B. H. [51]). To check the stability of the secondary structure of miRNAs, MFE and MFEI were also calculated. In contrast with transfer RNAs and ribosomal RNAs, the majority of the miRNA sequences clearly exhibit a folding free energy that is considerably lower than that for shuffled sequences, indicating a high tendency in the sequence towards a stable secondary structure [64]. The average MFE value of Curcuma miRNAs was 65.11 (–kcal/mol). This value was much lower than folding free energies of transfer RNA (tRNA, 27.5 (–kcal/mol)) and ribosomal RNA (sRNA, 33 (–kcal/mol)) [43]. On other side, the observed MFE values of Curcuma miRNAs were highly negative as compared to the Helianthus (35.83 (–kcal/mol)) and cotton (35.19 (–kcal/mol)) [43,45]. MFEI was observed with an average value of 1.15 (–kcal/mol), which was relatively higher than in earlier reports ([15,19]; B. H. [51]). These parameters and the supporting literatures are also consistent with the accuracy level of the miRNA prediction.
In order to control the gene expression in plants, miRNA binds to its target site with high complementarity to cleave the transcript or inhibit the translation [49]. The functionality of the any miRNA candidate can be understood only by its regulating targets. Target prediction of Curcuma miRNAs was performed for the coding as well as for the non-coding transcripts. A total of 238 annotated and 74 lncRNAs targets were identified. The abundance of non-coding RNA genes in plant genomes suggests the high prediction rate of lncRNA targets. Networking analyses suggested the dominancy of miR5021 for most of the co-regulated targets. In the case of ginger, miR5021 was found to be involved in the co-regulation of many targets.
The root development system of turmeric was observed to be governed by miR156 and miR5015, which were modulating the expression of SPL and ARF genes, respectively. Additionally, miR5015 showed response for bhlh, earlier reported for root hair development [65].
Transcription factors have been earlier reported as key regulators for plant development, progression including plant defence, stress response, root and shoot development [12,66–69]. Our results revealed the significant role of the miR5015 and miR5021 in modulating the expression of several TFs involved in different biological processes of turmeric.
In this study, we found two miRNAs (miR5021 and miR5649) to be involved in the backbone synthesis of terpenoid by modulating the expression of geranylgeranyl-diphosphate synthase. The terpenoids of turmeric are known to have anti-cancerous properties [59] and inhibitory effects on nitric oxide production [23,24]. Our results indicate the regulation of miR2919 for flavonoid biosynthesis by modulating the expression of flavanone synthase (EC: 2.3.1.74) and by contributing to antioxidant activities [25]. Isoquinoline alkaloid biosynthesis was observed to be regulated by miR5021.
In another analysis, a target search was performed against all reported miRNAs of miRBase. The results indicated the probable involvement of three miRNAs in curcumin biosynthesis. miR1168.2 was observed to regulate the gene encoding CYP73A, while miR156b miR1858 was observed to modulate the expression of 4CL. Gingerol and curcumin share some initial steps for biosynthesis [70], which also suggests the probable involvement of these three miRNAs (miR1168.2, miR156b and miR1858) in gingerol production. Other miRNAs were observed to be involved in several other growth and developmental process of Curcuma viz., signalling, response to stress (heat, cold and stimulus), fatty acid biosynthesis, embryo development, seed development, and cell differentiation.
5 Conclusion
Eighteen miRNA families were predicted using a combined assembly of reported nucleotide sequence, ESTs and SRA data. Uracil was observed to be the dominant nucleotide at the first position of mature miRNAs with predominant nucleotide content from pre-miRNA sequences. The results demonstrated the regulation of 238 targets by 16 miRNA families. This study presents a first report on the identification of miRNA and their targets involved in secondary metabolic pathways of turmeric. Our results showed the regulatory control of curcuminoid, terpenoid, flavonoid and alkaloid biosynthesis pathways by Curcuma-miRNAs. Our finding may also helpful for strategies aiming at enhancing secondary metabolite production based on small non-coding RNAs. Many predicted miRNA candidates also showed regulation for signalling, localization, and reproductive processes. These initial finding may improve the understanding of the miRNA and their regulation in turmeric as well as family Zingiberaceae. Further research is required to determine the role of miRNAs in the regulation of secondary metabolites production of this important medicinal plant.
Disclosure of interest
The authors declare that they have no competing interest.
Acknowledgments
The Department of Science and Technology (DST), New Delhi, India is gratefully acknowledged for the DST-INSPIRE-SRF fellowship (IF Code: IF120740) granted to author Noopur Singh. Financial support from CSIR 12th five-year plan project BSC0203 (ChemBio) is also acknowledged.