1 Introduction
There are now multiple and coherent lines of evidence for a discernible anthropogenic influence upon climate causing globally averaged near-surface and free-troposphere temperatures to rise, among other changes to the climate system. Climate model projections suggest that global mean temperatures will further increase in coming decades, causing a substantial net climate change. The 21st century range of global warming is projected to reach 1.5 to 6.4 K according to the fourth International Panel for Climate Change (IPCC) assessment report (AR4) published in 2007. For governments, society and industry, two connected elements are required to seriously consider effective action to mitigate and adapt to the effects of such possible outcomes: scientific evidence of a demonstrable anthropogenic cause of past and present changes and reliable and quantitative climate projections for the future. In addition, these two elements will be strengthened with decreasing spatial scale, going from, say, a global to a sub-regional or country-size assessment.
However, difficulties remain in reliably simulating and attributing past and present observed temperature changes at regional and country scales. On these scales, natural internal climate variability is large making it harder to distinguish changes due to either anthropogenic or natural external forcings. Uncertainties in local forcings such as aerosols and feedbacks also make it difficult to unambiguously attribute observed small-scale temperature changes to a specific and well-defined combination of factors. Therefore, uncertainties remain with regard to the detailed understanding of regional temperature past evolution. Note that this state of affairs applies even more so to other climate variables, such as precipitation.
Uncertainty is indeed also present in future climate projections. Here, the term “projection” indicates a conditional dependence of a climate prediction on a given emission scenario with no likelihood attached to it. Climate projections must be based on the most robust science and also take into account the sources of uncertainties that arise mainly because (but not entirely) of our insufficient knowledge. Climate models are our best, albeit imperfect, approximations of the real climate system and they are routinely used to make climate projections. Large coordinated simulation exercises (known as coupled model inter-comparison projects – CMIPs) are now performed on a semi-regular basis by many groups worldwide. They thus provide a multi-model ensemble (MME) of historical simulations (for instance of the 20th century) and climate projections under a range of emission scenarios. The total amount of climate projections may be on the order of a few hundred. How to best use an MME and its wealth of data is a complex and yet unsolved problem (Collins et al., 2012; Knutti et al., 2010; Weigel et al., 2010). For example, coherency among climate model results does not necessarily guarantee increased confidence in climate projections, simply because model errors might be strongly correlated and not random. Similarly, lack of coherency does not always imply that some of the models are better than others. Climate projections may differ (even in sign) only due to large natural internal variability relatively to externally forced changes (of anthropogenic or natural origin). An example, albeit based on a single climate model study, is that of the mid-to-high latitude Northern Hemisphere atmospheric circulation changes along the 21st century (Deser et al., 2010). This also applies to temperature and precipitation changes at continental, regional, and local scales (Deser et al., 2012). Furthermore, the usual reasoning about the link between signal-to-noise ratio (SNR) and spatial scales (namely that SNR decreases with scale) is not always valid. Regions with low internal variability may be more predictable (meaning that the anthropogenic fingerprint can be more readily identified), even at smaller scale, than others (Deser et al., 2012; Joshi et al., 2011).
Here, we wish to address the difficult question of future climate change at smaller scale (sub-regional or country scale) than current regional (continental in nature) assessments. A key related question is that of the uncertainty estimation of the projected changes. While in principle, regional climate models (RCM) appear to be the appropriate tools to tackle these questions, their current computational cost is still too high to perform the full range of needed model integrations. Therefore, we first focus on global climate model projections as performed in the framework of the recent CMIP5 exercise (Taylor et al., 2012). Furthermore, as lateral boundary conditions from global models are always needed to constrain RCMs, the assessment of projections based on global models is a required first step that provides a useful benchmark.
Based on current knowledge (using both observed and simulated data), can we say anything about future climate changes at these spatial scales? What are the main sources of uncertainty at these scales? How to best estimate and quantify them? Are there new ideas and strategies to better constrain them? The outline of the paper is as follows. As a case study, we are focusing here on the climate of France between 1900 and 2100. We first describe the observed and simulated datasets used throughout this work. We then present and analyze the simulated evolution of the near-surface air temperature and precipitation over France during the 20th century. We use various ensembles of historical simulations driven by different combinations of external forcings (both anthropogenic and/or natural). We then compare the simulated evolutions with observations and comment on whether the influence of anthropogenic forcing on temperature and precipitation can or cannot already be detected in the available observed datasets. We then analyze future projections from global models for the 21st century and provide simple estimates for annual and seasonal changes in France temperature and precipitation. We focus on two specific 30-year periods: the near future defined as 2020–2049 and the end of the 21st century as given by 2070–2099. All changes are estimated relatively to the 1900–1929 period. We specifically discuss how to define the related various uncertainty sources associated with the model projections. We further provide estimates of the latter and study how they evolve along the 21st century. We then report on some recent work dealing with the reduction of epistemic uncertainty, namely that related to the representation of physical processes in climate models. Finally, we address the questions “When will the 2-K global threshold be exceeded and what are the implications regarding France climate change?” We then conclude by a short summary and some new research directions as a perspective.
2 Observed and simulated data
2.1 Observed temperature and precipitation data
We first use a mean France thermal indicator based on a Météo France network of 30 station-based raw observations of daily and monthly mean temperature covering the 1947–2011 period and merged with 32 monthly homogenized temperature data (longues séries de données homogénéisées, LSDH dataset, Moisselin et al., 2002) time series from 1900 up to 1946 (http://onerc.developpement-durable.gouv.fr/fr/indicateur/temperature-moyenne-en-metropole).
The raw data stations have been screened to have no breaks since January 1947. LSDH station time series have been corrected in order to detect and remove breaks and outliers. Note that all the temperature stations have been selected to yield a quite uniform repartition and rather complete France coverage. For precipitation, we first use a large set of homogenized precipitation time series aggregated to form 51 time series that sample a large fraction of France and cover the 20th century (Moisselin et al., 2002). The mean of the 51 time series is used as the France mean precipitation. We have checked that it is a reasonable hypothesis by comparing to a high-resolution meso-scale atmospheric analysis (which includes several thousand spatial observations for precipitation) for the 1958–2011 period (Vidal et al., 2010). We also use the Global Precipitation Climatology Center (GPCC) dataset (http://gpcc.dwd.de) (Rudolf and Schneider, 2005). We specifically use the full Data Reanalysis (Version 5) for the period 1901 to 2009 based on quality-controlled data from all stations in GPCC's database available at the time with a varying coverage over time. This product is optimized for best spatial coverage and use for water budget and therefore not optimal for variability studies. However, comparison with the Météo France survey shows very small differences in term of variability (of precipitation averaged over France) and allows us to extend the observed record up to 2009.
2.2 Climate model data
We use the MME performed within the framework of CMIP5 (Taylor et al., 2012 and http://cmip-pcmdi.llnl.gov/cmip5/; see also Table 1 for the models and simulations used). These ensembles can be split into two categories: the first ones are the historical sets covering the 1850–2005 period. The different historical ensembles vary with regard to the applied external forcing, number of included models and ensemble size (see Table 1). The first historical ensemble (25 models) is forced by all anthropogenic and natural forcings (named the ALL ensemble from now on), while the second one (nine models) is driven by natural forcings only (the NAT ensemble). The second type includes the 21st-century projections driven by several greenhouse gases (GHG) scenarios, namely the radiative concentration pathways (RCP), RCP2.6, RCP4.5, and RCP8.5 (Meinshausen et al., 2011; van Vuuren et al., 2011a). The RCP8.5 is the highest scenario and its CO2 concentration reaches 936 ppm by 2100. RCP4.5 reaches 538 ppm by 2100, while RCP2.6 peaks in 2050 at 443 ppm and then declines down to 421 ppm in 2100. The different CMIP5 scenario ensembles (simply named RCP8.5, RCP4.5 and RCP2.6) differ with regard to the included models and ensemble size (see Table 1). With regard to projections, we only consider the near-surface air temperature (SAT) and precipitation (PR) variables. We also use cloud cover (CC) to illustrate our epistemic uncertainty analysis. All model data are interpolated on a regular grid at 1.5° resolution. We have used the land-sea fraction file of the models to properly take into account the surface data homogeneity in the interpolation process (meaning that land values on the common target grid use only land point values from the source grid). To estimate model mean France SAT and PR, we define a mask including France boundaries and simply average all relevant grid point values.
CMIP5 models and experiments used in this study (Taylor et al., 2012 and http://cmip-pcmdi.llnl.gov/cmip5/).
ALL | NAT | RCP8.5 | RCP4.5 | RCP2.6 | |
bcc-csm1-1 | 3 | 1 | |||
CanESM2 | 5 | 5 | 5 | 5 | |
CNRM-CM5 | 10 | 8 | 5 | 1 | 1 |
CSIRO-Mk3-6-0 | 10 | 5 | 10 | ||
inmcm4 | 1 | 1 | |||
IPSL-CM5A-LR | 6 | 3 | 4 | 4 | 3 |
IPSL-CM5A-MR | 2 | 1 | |||
FGOALS-g2 | 3 | 3 | 1 | 1 | 1 |
MIROC5 | 5 | 3 | |||
MIROC-ESM | 1 | 1 | |||
MIROC-ESM-CHEM | 3 | 1 | |||
HadGEM2-CC | 1 | 3 | |||
HadGEM2-ES | 4 | 4 | 4 | 4 | 3 |
MPI-ESM-LR | 3 | 3 | 3 | 3 | |
MPI-ESM-MR | 3 | 1 | 3 | 1 | |
MRI-CGCM3 | 3 | 1 | 1 | 1 | |
GISS-E2-H | 5 | 5 | |||
GISS-E2-R | 6 | 5 | 1 | ||
CCSM4 | 6 | 2 | 5 | 6 | 6 |
NorESM1-M | 3 | 1 | 1 | ||
GFDL-CM3 | 5 | 1 | |||
GFDL-ESM2G | 3 | 1 | |||
GFDL-ESM2M | 1 | 1 | |||
CESM1-BGC | 1 | 1 | |||
CESM1-CAM5 | 3 | 3 | 3 | 3 | |
CESM1-WACCM | 1 | 1 |
For most of the analysis, we only show the results with the scenario RCP8.5. We further discuss this choice at the end of the paper. However, for some of the results and analysis, we also describe the uncertainty related to the use of the other RCP scenarios. As the number of historical or scenario simulations per model varies from one to 10, we do not want our results to be biased by the most populated models. Consequently, we take the mean of the MME model means (of historical or scenario simulations) as our best estimate of the forced climate response to the applied combination of forcings. In case of RCP8.5, we use 60 21st-century simulations performed with 25 models. Our best estimate is then based on the average of 25 model ensemble means. The MME variance of variable x is then estimated by with Var(i) the variance of model i with ni members being estimated as with the average over all models and members. The MME approach relies on the hypotheses that there are partially cancelling errors between the various models and that internal variability is also averaged in the multi-model averaging process (see Knutti et al., 2010; Tebaldi and Knutti, 2007 and for an in-depth discussion of these issues). We also provide the distribution of changes assuming that all model outcomes have the same probability (meaning that we systematically give the inter-model range defined as the 5–95% confidence interval of the model distribution). Acknowledging that there is no evidence that the CMIP5 MME model distribution can provide an either upper or lower bound to the true model uncertainty, we nevertheless suggest that it is a simple and useful starting point to measure model (or epistemic, see below) uncertainty (Knutti et al., 2010; Raisanen, 2007). We suggest some directions on how to move beyond that assumption at the end of the paper by using process-based metrics to reduce epistemic uncertainty. When we compare the results between different RCPs, we select models that have performed all three scenarios (10 models) in order to avoid different prior sampling and mix of different uncertainty sources. The same approach has been followed when comparing the ALL and NAT historical ensembles.
3 Mean temperature and precipitation evolution over France during the 20th and 21st century: observations and climate simulations driven by the RCP8.5 scenario
Here, we first compare observed changes to the distribution of CMIP5 models simulated 20th century changes. It is important to note that we do not expect a perfect match between observed changes and our best estimate of the forced response. We do expect differences between the two due to the influence of internal variability, particularly in the early 20th century when the anthropogenic forcing is considered to be weaker. However, we expect that the observed changes lie within the full distribution of simulated changes spanned by individual model and ensemble member realizations. The contrary (over an extended period) would suggest an underestimation of simulated internal variability and/or a biased response to external forcings. In what follows, consistency between observations and models means that observations lie within the inter-model range as defined above. Unless specified otherwise, the terminology “simulated/projected changes” in the next sections is meant to be our best estimate of the forced response to external forcings.
3.1 Surface air temperature
Observed annual mean SAT in France have been relatively stable in the 20th century until the 1980s with a warm period (20–30 years) in the 1940s followed by a cold period of roughly the same length in the 1960s (Fig. 1, black curve; see also Abarca-Del-Rio and Mestre, 2006). In contrast, the last 30 years are characterized by a strong warming trend, which is clearly outside of the range of low-frequency variability of the previous period. For example, the last 30-year period (1982–2011) is 1 K warmer than the 1900–1929 reference climatology and is by far the warmest three-decade period of the whole 20th century and beyond (up to 2011). The simulated change (Fig. 1, red curve) is slowly increasing until the late 1970s and then rises steadily (note that the reduced interannual variability compared to the observations simply results from multi-model averaging). A purely observational analysis does not provide any strong evidence of the likely causes of the recent warming. In the observations, the response of the climate system to external forcings (whether anthropogenic and/or natural) is inextricably mixed with internal variability. Dedicated simulations provided by coupled general circulation models constrained by various sets of external forcings are needed to separate, attribute and understand (in terms of physical processes) the different contributions. Fig. 1 (insert) suggests that the recent observed warming cannot be explained by natural forcings alone, as it is not consistent with the NAT ensemble. On the opposite, it is consistent with the ALL ensemble, suggesting that anthropogenic forcing is the dominant factor of the observed recent changes. Note in addition that the two ensembles are significantly different (at the 5% level) since the late seventies. This suggests that the influence of anthropogenic forcing could be detected from the late 1970s and early 1980s, as already suggested by several detection and attribution studies (Planton and Terray, 2007; Ribes et al., 2009; Spagnoli et al., 2002). Note also that observations of the last decade are close to the upper range of the model distribution, suggesting a significant contribution of internal variability and/or a possible underestimation of the forced response (Van Oldenborgh et al., 2009). The observed 20th century (1900–1980) multi-decadal SAT evolution is also remarkably well correlated with the simulated climate response to combined anthropogenic and natural forcings (and not with that to natural forcings alone). This suggests that anthropogenic forcing has had an influence upon mean France SAT multi-decadal variations well before 1980. Anthropogenic aerosols have been suggested to be an important factor of the transition between the 1940s’ warm phase and the cool phase of the 1960s and 1970s. In addition, one cannot exclude a contribution from natural forcings to the early 20th century slow temperature rise.
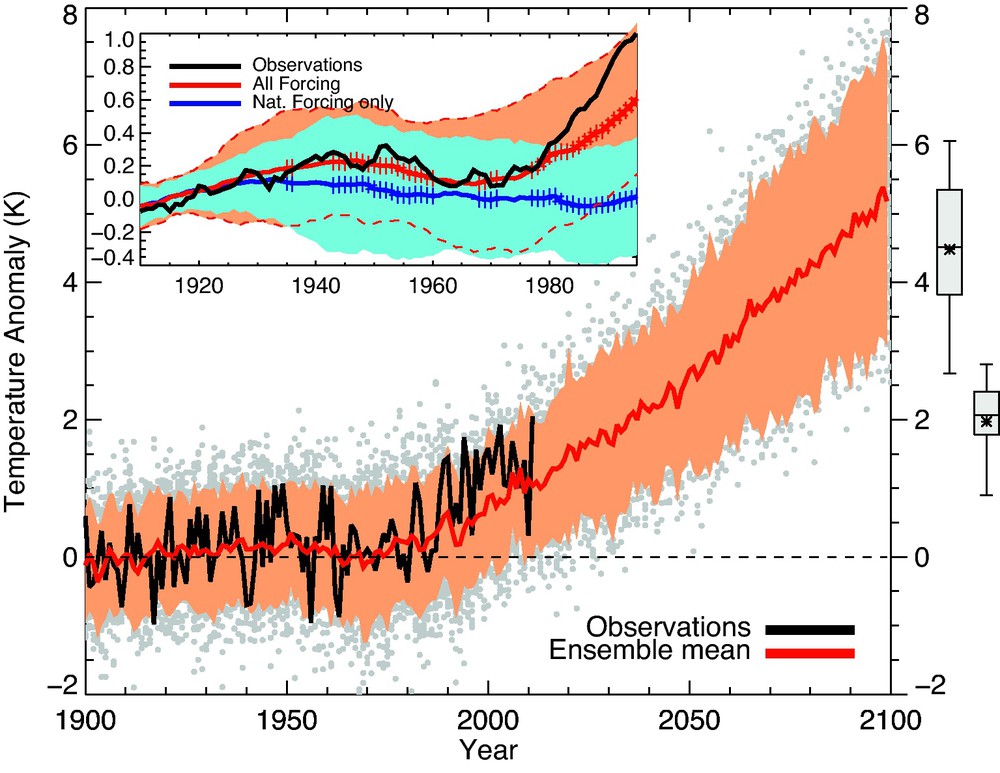
Main panel: time evolution of France annual mean surface air temperature anomalies (K) relatively to the 1900–1929 climatology: observations (black line) and the ALL multi-model ensemble mean merged with that of the RCP8.5 ensemble (red line). Orange shading gives the envelope defined by the [5–95%] model range, and grey dots indicate annual means from individual simulations. The grey histograms on the right give the model distribution [min, 25%, 50%, 75%, max, and mean given by the asterisk symbol] of the changes averaged over the near future [2020–2049] and the end of 21st century [2070–2099]. If there are data beyond 1.5 times the 25 and/or the 75 percentile, the whiskers extend out to these two thresholds and outliers are then identified with small circles. Insert: same as above, but using only models shared by the ALL and NAT ensembles and with the NAT MME mean added. All insert time series have been filtered with a 21-year running mean. Small-size crosses indicate years where the ALL and NAT ensemble means are significantly different at the 5% level (paired t-test).
As for the 21st century, France is shown to warm significantly under the scenario RCP8.5. The CMIP5 MME simulates a median France warming of about 2.1 and 4.5 K for the near future and end of 21st century, respectively. The full range of projected changes is [0.9–2.8 K] for the near future and [2.7–6.1 K] for the end of the 21st century. These results suggest that the rate of warming may increase by a factor 2 to 3 during the 21st century (half of the models already simulate a warming larger than 2 K by 2035).
Fig. 2 shows SAT seasonal changes for the historical period and the 21st century under three different GHG scenarios. Observed summer and winter SAT 20th century changes are within the multi-model distribution suggesting that the CMIP5 MME is able to generate interannual anomalies consistent with observed ones. This applies also to extreme seasonal events such as the 1963 winter or even the 2003 summer (a few simulated years show warming very close to these events’ observed values). CMIP5 models also reproduce observed stronger SAT interannual variability in winter compared to summer. Observed SAT multi-decadal changes differ between summer and winter. While winter SAT exhibits a cool phase in the 1930s and 1940s followed by a slow and regular warming, summer SAT exhibits a warming-cooling-warming pattern close to that of annual mean temperature. Century-long (1982–2011 versus 1900–1929) observed SAT changes are larger in summer (1.37 K) than in winter (0.66 K), while SAT simulated changes have the same value (0.66 K) in both seasons. This suggests that, in addition to anthropogenic forcings, natural internal variability might have contributed to the recent summer warming. While summer SAT simulated changes are significantly correlated (0.95, significant at the 1% level using a random-phase sampling test, P-value 0.01) to the observed SAT evolution, the winter ones (showing a monotonic weak warming) differ from the observed evolution (cold phase marked by a series of cold winters) during decades around the mid-20th century (correlation of 0.72, significant at the 10% level, P-value 0.08). Furthermore, observed winter SAT does not exhibit the cooling seen in summer from the 1950s to the 1970s. A fraction of European SAT decline between 1950 and 1980 has been attributed to tropospheric aerosol forcing (Folini and Wild, 2011; Wild, 2009). Whether aerosol forcing and/or natural internal variability can account for France observed SAT changes contrast between summer and winter remains unclear and requires additional work. This also suggests that observed winter SAT could have been significantly influenced by natural internal variability during the 1930–1970 period (it might also point out to common model biases in the forced response to external forcings or inadequacies in the forcing itself).
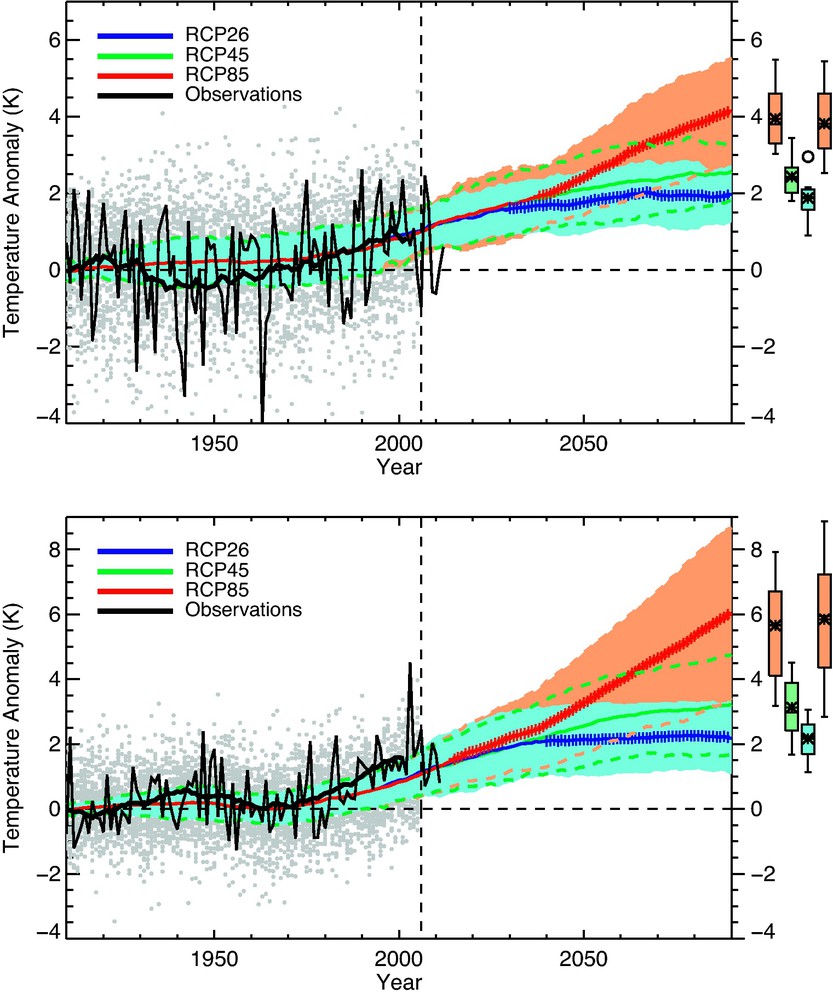
Time evolution of France seasonal mean surface air temperature anomalies (K) relatively to the 1900–1929 climatology: observations (thin black line) and the ALL multi-model ensemble mean (red) merged with those of the RCP2.6 (blue), RCP4.5 (green) and RCP8.5 (red line) ensembles. Surface air temperature time series have been low-pass filtered with a simple 21-year running mean (thick lines). Shading and dashed lines give the envelope defined by the [5–95%] model range (for each ensemble) and grey dots indicate individual years from individual historical simulations. The colored histograms on the right give the model distribution [min, 25%, 50%, 75%, max, and mean given by the asterisk symbol] of the changes averaged over the near future [2020–2049] and end of 21st century [2070–2099]. The two orange ones refer to RCP8.5 using 10 (left, shared by all radiative concentration pathways) and 25 (right) models. Small-size crosses indicate years where the radiative concentration pathway ensemble means are significantly different at the 5% level (paired t-test): red line for the RCP [8.5–4.5] pair, blue line for the radiative concentration pathway [4.5–2.6] pair. Upper panel: winter mean defined as December–January–February (DJF) average. Lower panel: summer mean defined as June–July–August (JJA) average.
Under the RCP8.5 scenario (and based on the 25-model MME), the projected summer SAT changes for the end of the 21st century are on the order of 6 K (median value), with a full range given by [2.8–8.9 K]. Winter changes are smaller and reach 3.7 K [2.5–5.5 K]. The summer projection range is larger by a factor 2 than its winter counterpart, which is likely due to larger epistemic uncertainty in summer related to land–atmosphere and cloud–temperature interactions (Boé and Terray, 2008a; see also section 5). Note that the summer spread increase with time for the RCP8.5 scenario may arise from a variety of interacting processes (Boé and Terray, 2013). The two other GHG scenarios project changes of much reduced amplitude. Based on a ten-member ensemble, RCP2.6 exhibits summer and winter changes around 2 K (2.2 K and 1.9 K) with even stabilization during the last decades of the 21st century. RCP4.5 is slightly warmer (3 K in summer, 2.4 K in winter) and shows still increasing (albeit at a slower rate than RCP8.5) temperatures at the end of the 21st century. Both scenarios exhibit reduced spread in both summer and winter seasons compared to RCP8.5 (for instance, RCP4.5 summer projection spread is 1.7–4.5 K). Note that the time of emergence of significant differences between the different scenarios vary with seasons. In winter, no significant difference is found until the 2030–2040 decade, while in summer, RCP8.5 is already significantly different with regard to RCP2.6 and RCP4.5 (the latter two are different from 2040 onwards) from 2015 onwards.
3.2 Precipitation
For PR, we only show seasonal mean changes and not the annual mean, the latter being very often the residual of large changes with opposite signs. While France observed summer mean PR has been overall stable during the 20th century, winter PR has slowly increased with larger decadal fluctuations in the last 40 years (Fig. 3). Note however the smallness of the trend and multi-decadal observed changes compared to the very large interannual variations. This suggests that detection of an emerging trend and/or multi-decadal variations is much more difficult than with SAT. However, these averaged diagnostics hide spatial differences. Over the 1901–2000 period, the contrast between the two seasons is noticeable with a winter increase over the whole country (with significant values only along a central north–south line) and roughly the opposite pattern for summer with reduced PR, particularly over southern France (Moisselin et al., 2002). As shown by Boé and Terray (2008b), winter variability is mainly due to changes in the occurrence of dominant circulation regimes. To a lesser extent, this is also true in summer, although the links between the large-scale circulation and precipitation are weaker than in winter due to the mostly convective nature of rainfall events.
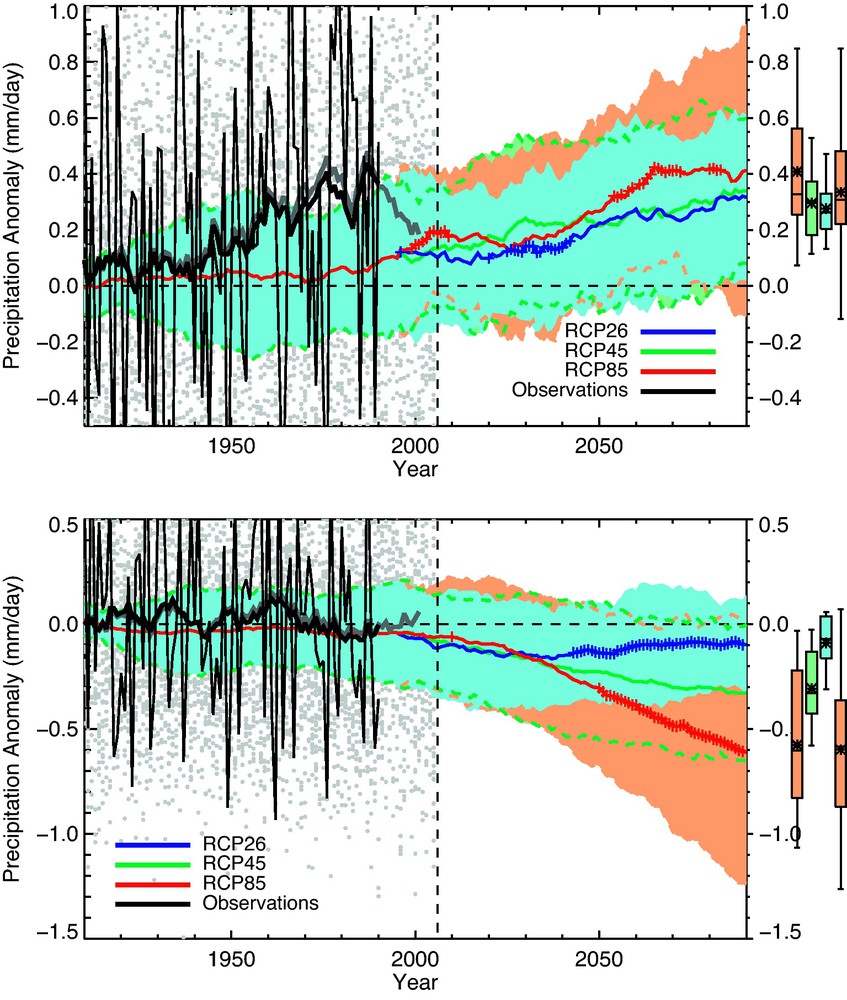
Same as Fig. 2, but for France precipitation (mm/day). Note that the full range of individual modeled and observed years is not displayed, in order to have a clear view of the 21st-century changes. Black and grey lines indicate observed data from the Météo France and Global Precipitation Climatology Center datasets, respectively. Top panel: winter; bottom panel: summer.
Mean France PR 21st century projected changes exhibit a contrasted seasonal behaviour (Fig. 3). Under RCP8.5, winter PR projected changes show an increase (up to 0.4 mm/day for the last 30 years) with decadal variation of the inter-model range. Summer projected PR changes exhibit a strong quasi-linear decrease (with a mean reduction at the end of the 21st century of −0.6 mm/day [0.07; −1.26 mm/day]) superimposed with a linear increase of the inter-model range with time. The large values of the inter-model range compared to the mean projected change suggest that the amplitudes of France mean summer and winter PR projected changes are rather uncertain. The other RCP scenarios are not significantly different from RCP8.5 in winter, even at the end of the 21st century (periods when they are different, do not persist, and illustrate strong multi-decadal internal variability and/or large model spread). In summer, all scenarios differ from each other by 2050. Under RCP4.5, projected PR changes are −0.3 mm/day [0; −0.6 mm/day] at the end of the 21st century, while RCP2.6 PR changes are close to zero with a [0.04; −0.3 mm/day] inter-model range. It is also interesting to point out that PR summer changes are much larger than the winter ones when looking at relative values (in percentage of the 1900–1929 climatology). We further discuss possible reasons explaining why inter-RCP PR changes differ more in summer than winter in the uncertainty section.
4 21st-century temperature and precipitation spatial changes under the RCP8.5 scenario
Spatial patterns of future France (and Europe) seasonal mean SAT changes show large differences with season under the RCP8.5 GHG scenario (Fig. 4). While winter SAT changes exhibit mostly a tilted zonal gradient with largest values over north-eastern Europe decreasing westward, summer changes are increasing from north to south over France and western Europe. While France near-future changes are characterized by small geographical differences (0.5 K), the end of 21st-century France SAT change gradients reach 1 K in winter and 2 K in summer. Summer SAT changes approach 7 K in southern France, while winter ones exceed 4 K over eastern France at the end of the 21st century. These regional pattern changes are part of a larger and spatially coherent European pattern. These different patterns arise from different mechanisms accounting for the seasonal temperature changes over France and Europe. In summer, the core of maximum warming associated with drier conditions and related evapotranspiration changes is found over the Mediterranean and southern Europe, including the South of France, while the maximum winter warming due to temperature-albedo feedbacks related to snow and sea ice melting is concentrated over north-eastern Europe. Advection of oceanic air masses by the westerly flow and a modified SAT land-sea ratio likely contributes to the winter minimum in western Europe. In agreement with previous work, CMIP5 models do show an increase of the SAT land-sea ratio along the coasts of western and southern Europe. Atlantic and Mediterranean SAT changes can reach 3 K in winter and even larger (4 to 5 K) values in summer.
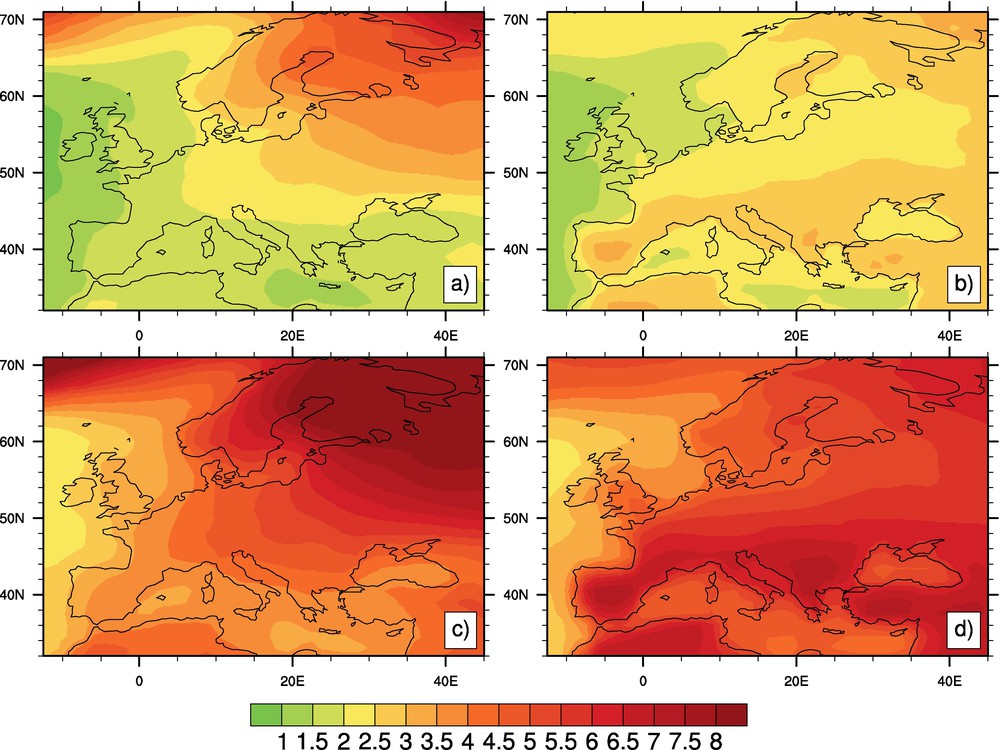
Projected surface air temperature changes (K) relatively to the 1900–1929 period. Near future: a) winter; b) summer. Late 21st century: c) winter; d) summer.
A latitudinal dipolar pattern is also present for European PR simulated changes, with a transition zone being located along 55°N and 45°N in summer and winter, respectively (Fig. 5). While summer precipitation over the entire France exhibits a strong decrease (with maximum values over the South extending all the way down to North Africa), there is an increase in winter only over the most northern part of France (extending to northern Europe) and a decrease over the Mediterranean and North Africa. Even if the patterns are already present for the near-future period, their amplitude is much weaker. While end of the-21st-century France PR change signs are coherent in summer among CMIP5 models, it is not the case in winter for the most part of France (not shown). This suggests that large uncertainty remains with regard to winter hydrological changes over France. Recent analysis of CMIP3 and CMIP5 model winter circulation changes suggest that large uncertainty remains as to the mean model response. It is also unclear if the projected changes do project strongly on an existing circulation regime. While there is more agreement in term of the sign of summer PR changes, there is still significant uncertainty as to their amplitude. Boé et al. (2008) have shown that a significant fraction of the future summer precipitation changes over England and western France is due to changes in circulation regimes (decrease in precipitation for these areas is strongly related to the increase of blocking occurrence which greatly varies in amplitude among CMIP3 and CMIP5 models).
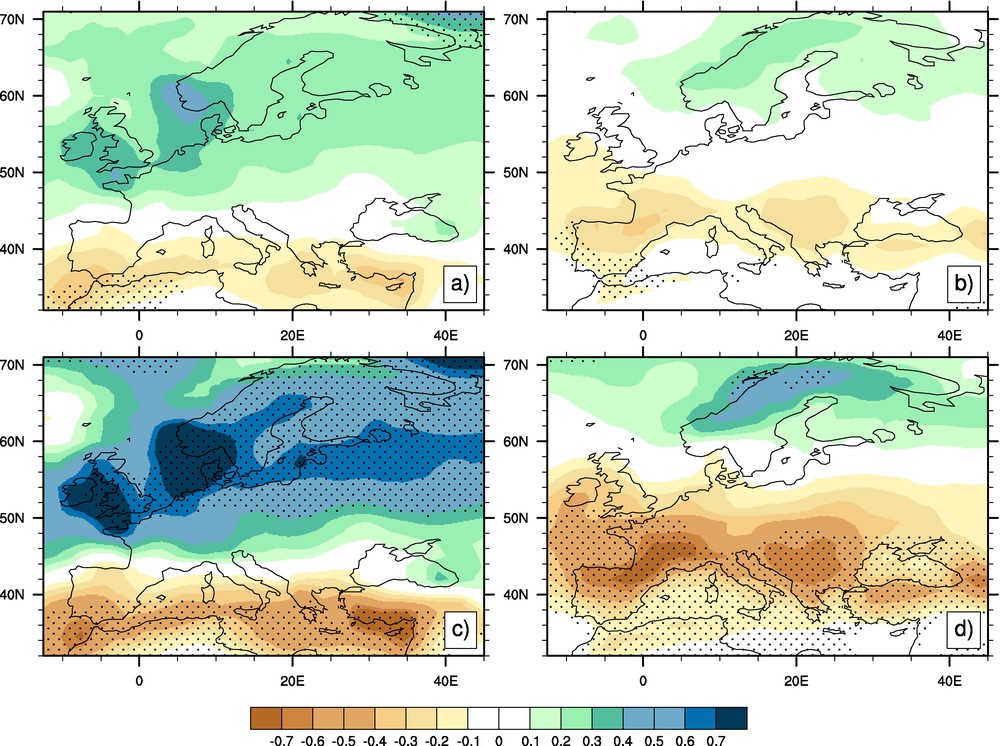
Same as Fig. 4 for precipitation projected changes (mm/day). Stippling indicates regions where absolute relative change is larger than 20%.
5 The different sources of uncertainty related to France climate 21st-century projections
In the previous sections, we have described the simulated forced changes under the RCP8.5 GHG scenario. We now focus on the quantification of the various sources of uncertainty associated with the projections. Many studies have recently tried to better understand and quantify the different sources of uncertainties in global and regional climate projections for the 21st century. Different typologies have been proposed. We concentrate here on the three standard sources of uncertainty related to global climate model projections, which all require a specific approach. The first one is the epistemic uncertainty and is related to the lack of knowledge in the model representation of physical and dynamical processes. It also includes missing processes and/or interactions within climate models. It is often named structural uncertainty or model uncertainty/error. Reduction of epistemic uncertainty is a noteworthy long-term objective. The second one is the aleatoric uncertainty and is related to the fact that the climate (defined here as a 30-year mean) is partially chaotic due to unpredictable internal variability. It is important to note that this source of uncertainty is likely irreducible at lead times greater than a few decades. While initialized decadal predictions can in principle be used to reduce aleatoric uncertainty, current prospect shows that the added value does not extend much beyond one decade for ocean variables and less for continental ones. Beyond this time scale, the objective is to find its best estimate with a conservative approach, meaning that we would prefer over- rather than under-estimate aleatoric uncertainty (meaning that we do not want to exclude possible climate change due to a possible multi-decadal internal variability superimposed on the forced changes). The third one is the reflexive uncertainty and is linked to the fact that the 21st GHG emission trajectory is unknown and involves unpredictable climate–society feedbacks as well as non-climate related (e.g., political and economic) factors (also not predictable).
We assess these two uncertainty sources, epistemic and aleatoric, by using only the RCP8.5 ensemble. We then select models having an ensemble size with at least three members (Nine models). We simply use the spread of CMIP5 MME ensemble means to characterize the epistemic uncertainty and the spread of the concatenated intra-ensemble changes (meaning we subtract the ensemble mean to each member for each model separately) for the aleatoric part. Finally, we take a fairly crude approach to assess reflexive uncertainty by defining it as the difference between the two extreme model-averaged RCP ensemble means. Again, we do not pretend that the three RCP storylines describe the full range of possible futures. They are nevertheless different enough (outside the 10–90% range of the all scenario range) to grasp the magnitude of the sensitivity to sizable differences in emission scenario (contrast between the RCP8.5 and 2.6, see discussion below).
We first show the estimated [5–95%] range (simply taken here as ± 1.64 standard deviation of the relevant distribution) of aleatoric and epistemic uncertainty related to projected SAT changes for both periods and seasons (Figs. 6 and 7). While both uncertainty sources are comparable in winter for the near future (± 0.51 and ± 0.69 K, France average), epistemic uncertainty is twice as large (± 1.23 K) as the unchanged aleatoric value at the end of the 21st century. In summer, epistemic uncertainty dominates, as it is already almost twice as large as the aleatoric part for the near future (± 0.96 K versus ± 0.52 K). It then doubles by the end of the 21st century (± 2.3 versus ± 0.54 K). While aleatoric uncertainty remains the same between the two periods, epistemic uncertainty strongly increases (even more so in summer). While epistemic uncertainty is not stationary, the aleatoric one seems rather stable, suggesting that external forcing does not strongly impact internal variability during the 21st century. Furthermore, summer SAT epistemic uncertainty is larger in France than anywhere else in western Europe (Boé and Terray, 2008a, 2013).

1.64 standard deviation (σ) of the given uncertainty source for winter surface air temperature projected changes (in K). Near future: a) aleatoric; b) epistemic. Late 21st century: c) aleatoric d) epistemic. Note that the 5–95% uncertainty range is given by the mean projected change ± 1.64 σ.
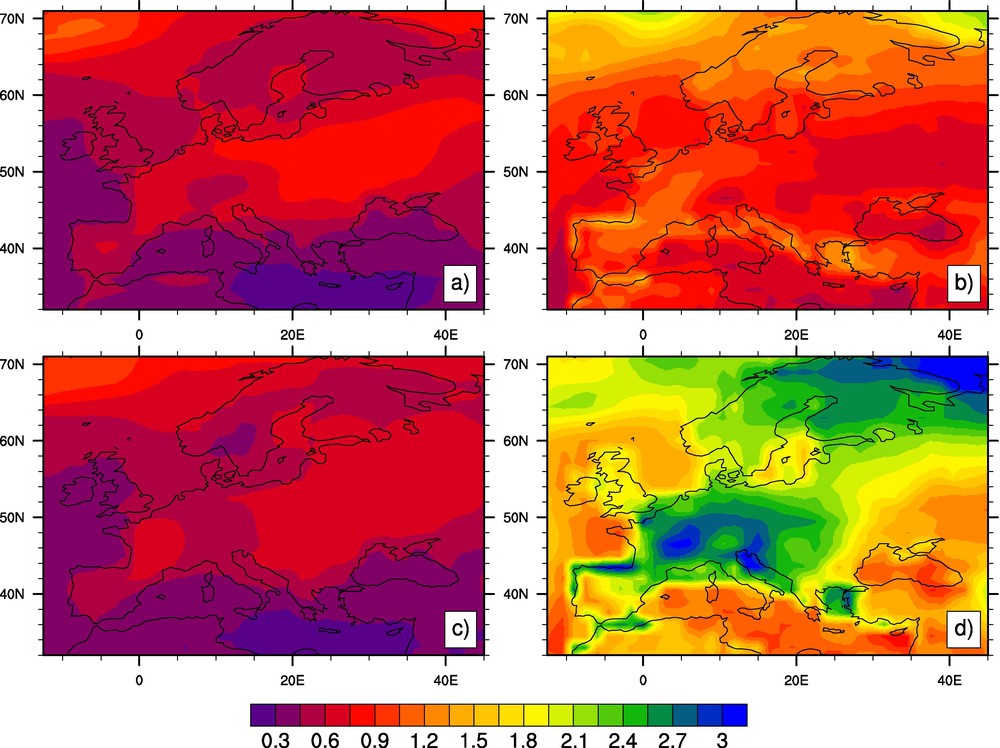
Same as Fig. 6 for summer surface air temperature changes (K).
The same analysis applied to PR shows (Figs. 8 and 9) that aleatoric uncertainty is rather similar over the two future periods (around ± 0.33 and ± 0.25 mm/day, winter and summer, France average). Spatial seasonal patterns over Europe are very similar between the two periods. Epistemic uncertainty roughly doubles from ± 0.19 to ± 0.35 mm/day and ± 0.29 to ± 0.47 mm/day in winter and summer, respectively. Central Europe (including eastern France) stands out as the region with the strongest increase. Note that these uncertainties have almost similar amplitudes as the projected changes in France, in particular in winter. Given that aleatoric uncertainty has large amplitude, particularly for the near future in winter, caution is needed on the possibility of significantly reducing the range of winter PR projections. Note also that epistemic uncertainty is by far the dominant source in summer, suggesting that it could in principle be reduced (see last section).
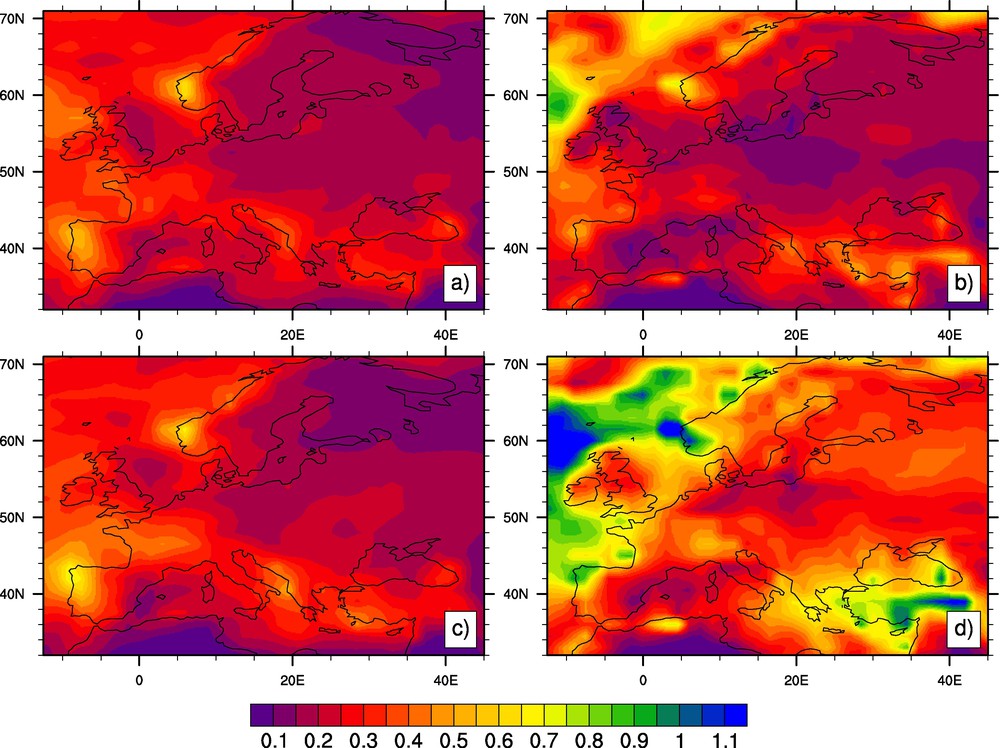
Same as Fig. 6 for winter precipitation changes (mm/day).
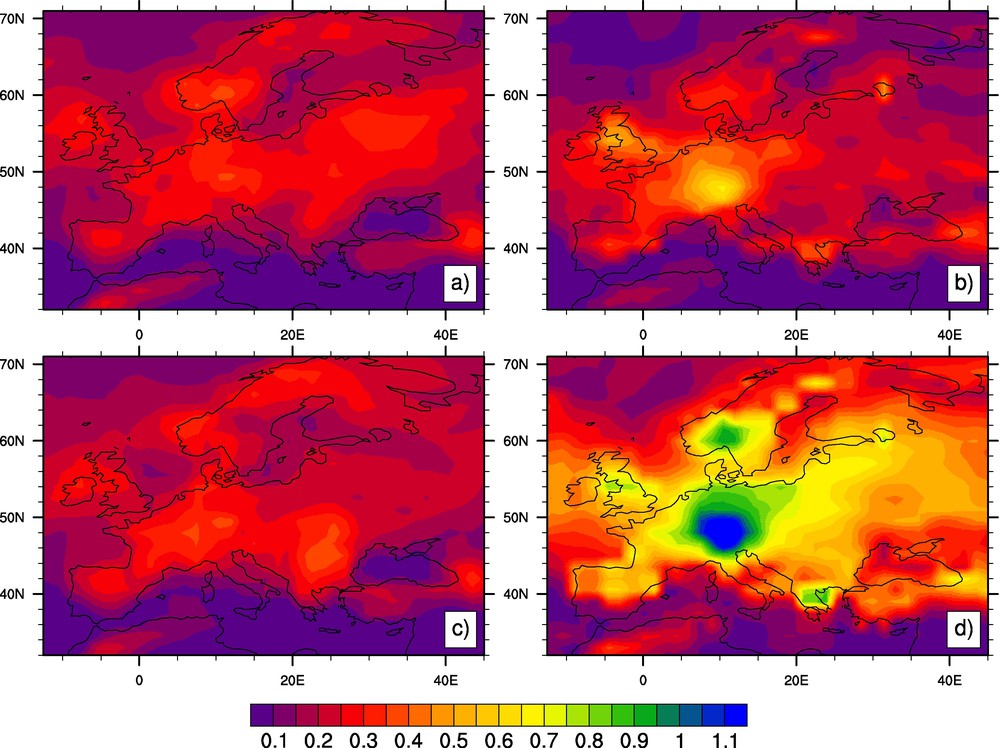
Same as Fig. 6 for summer precipitation changes (mm/day).
The reflexive uncertainty range is simply defined by the difference between RCP8.5 and RCP2.6 projected changes averaged over France. As mentioned before, the RCP2.6 emission and concentration pathway is representative of the emission scenarios aiming to limit the increase of global mean temperature to 2 K (van Vuuren et al., 2011b). Emissions would need to decline very substantially in order to reach a level of 2.6 W m−2 by the end of the century. Global emissions would need to peak by 2020 and then decrease at a 4% (of 2000 emissions annually) rate over several decades (van Vuuren et al., 2011b). Even if it is based on very optimistic assumptions on mitigation potential, it is still technically feasible and appears as a good candidate to represent the low-end scenarios. On the other hand, RCP8.5 is characterized by increasing greenhouse gas emissions over time, and is representative of high-end scenarios that lead to very high greenhouse gas concentration levels at the end of the 21st century. Note that these two scenarios are both plausible and internally consistent (van Vuuren et al., 2011a). Here, we only use the ten-model ensemble that have been used for the three scenarios (RCP8.5 SAT and PR used values are thus slightly different from those given previously, see Figs. 2 and 3). The SAT reflexive uncertainty range is thus [3.8–1.9 = 1.9 K] in winter and [5.7–2.2 = 3.5 K] in summer at the end of the 21st century. Its amplitude is thus comparable, albeit smaller, to that of epistemic uncertainty [2.6 and 4.6 K]. The near-future reflexive uncertainty range is notably smaller [winter: 1.8–1.6 = 0.2 K and summer: 2.4–1.8 = 0.6 K] than the two others, except for summer, where reflexive and aleatoric uncertainties have similar amplitude.
The winter PR reflexive uncertainty range (at the end of the 21st century) is barely different from zero [0.05 mm/day], while the summer value has almost the same magnitude [0.55 mm/day] as the projected PR decrease under RCP8.5, meaning that PR projected changes under RCP2.6 are close to zero. The near-future range shows indistinguishable changes among the three RCPs. Note that reflexive and epistemic have similar amplitude for the 21st-century projected summer PR changes and that the aleatoric source cannot be neglected, in particular in winter.
We now propose some physical processes that could be used to define process-based metrics aiming at reducing epistemic uncertainty. Note that “reducing uncertainty” may cover changes to both the projected MME change and spread. A process-based metric relies on the existence of a direct link between the model representation of a physical process in the current climate and the scatter of climate projections. We have previously shown that mechanisms controlling summer evapotranspiration could be used to constrain future European climate change (Boé and Terray, 2008a). Here, we suggest a slightly different approach based on the European summer cloud–temperature relationship (Tang et al., 2012). Europe and in particular southern Europe is one of the regions where the largest decrease in summer CC is projected during the 21st century, albeit with a large inter-model range. As cloud changes can strongly impact the surface energy budget, they likely play an important role in the summer SAT epistemic uncertainty range. It appears that the CC of models characterized by stronger present-day interannual anti-correlation between CC and land SAT (computed over the 1961–2000 period for each model and then averaged over France) tends to decrease more in the future climate (Boé and Terray, 2013). While all cloud CC-SAT model present-day correlations are negative, substantial spread exists on the amplitude (particularly over France and Spain). Therefore, we partition the models (ALL+ RCP8.5 25-model ensemble) in three groups (with eight, nine and eight models) corresponding to weak, median and strong (anti-) correlation amplitude. Fig. 10 shows that the difference in summer SAT projected changes for the two extreme groups is statistically significant by 2020 and reaches 2.5 K at the end of the 21st century. It represents 40% of the projected SAT change under RCP8.5. Note also the spread change between the two groups. Knowledge of the observed correlation value could then suggest an unrealistic cloud cover–SAT present climate relationship (and thereby projected changes) for one of the group and lead to a different mean projected change and range of epistemic uncertainty. Work is ongoing to make a detailed assessment of available cloud cover and temperature observations over France and Europe in order to identify the observed correlation and its uncertainty range. Note that an accurate representation of the latter is a complex issue. First, different types of cloud observation have to be compared with their respective measurement and data processing uncertainties. Second, as the cloud observational record covers at most a few decades, low-frequency internal variability of the metric cannot be directly assessed from observations. If its amplitude is large, it can have a strong influence upon any weighting model scheme (Weigel et al., 2010). Analysis of CMIP5 models aleatoric spread suggests that it can be significant (± 0.07 estimated over the 1961–2000 period). Finally, a strongly negative observed correlation value would suggest that models with strongly negative correlations are more realistic, which then would lead to enhanced warming and reduced epistemic uncertainty compared to the full MME.
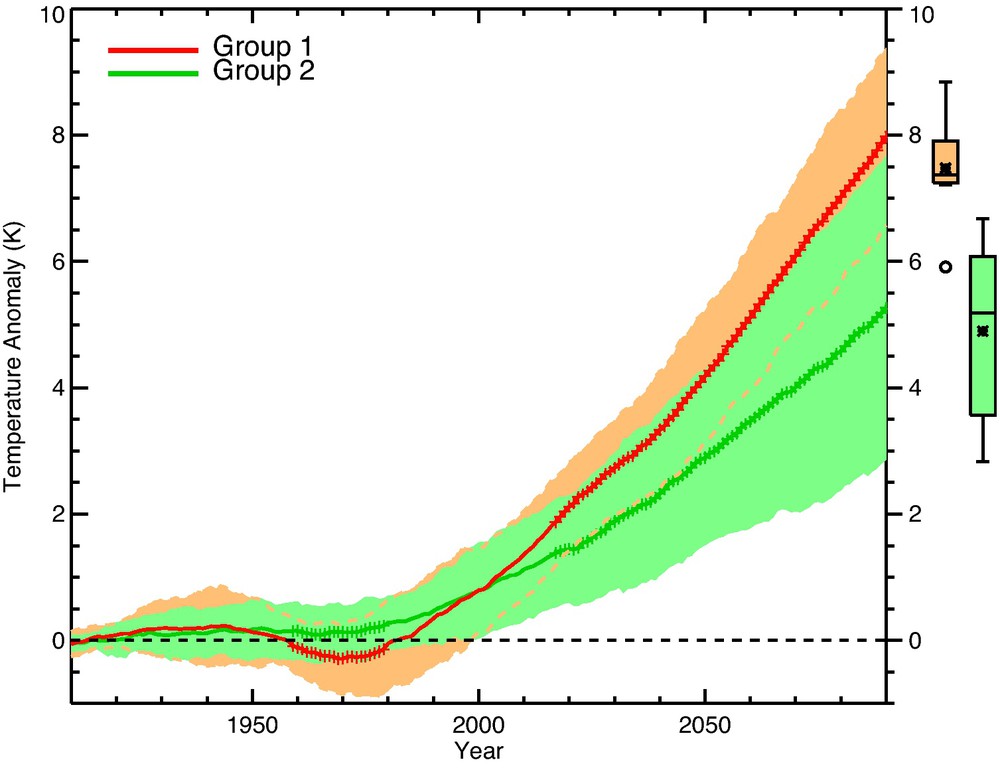
Time evolution of France summer mean surface air temperature anomalies (K) relatively to the 1900–1929 climatology for the means of two groups of models (ALL merged with RCP8.5). Group 1: models with strongly negative present-day summer surface air temperature-cloud cover correlations (red line). Group 2: models with weakly negative ones (green line). Surface air temperature time series have been low-pass filtered with a simple 21-year running mean. Shading and dashed lines give the envelope defined by the [5–95%] model range for each group of models.
6 The 2-K threshold and regional implications
The United Nations Framework Convention on Climate Change (UNFCCC) has proposed that reducing GHG emissions to keep the global mean SAT increase below 2 K (since pre-industrial levels) would greatly help to prevent dangerous anthropogenic impacts on the climate system. We therefore investigate the two following questions: “based on CMIP5 models and RCP emission pathways, will the global mean 2-K threshold be exceeded and, if yes, when?” and its corollary “What does the global mean 2-K threshold mean to France climate change?” Note that here we consider the 2-K threshold relatively to the early 20th century. The crossing year is defined in the legend of Fig. 11. The crossing period is defined as the period centred on the crossing year.
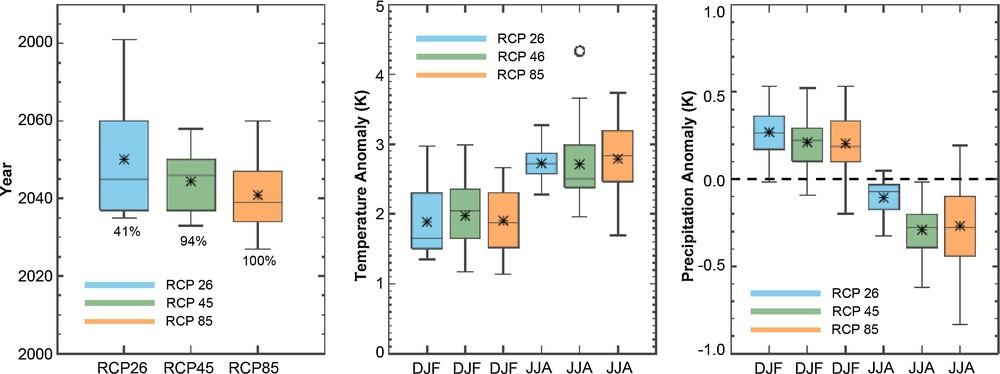
(Left) Central year of the first 31-year period during which time-averaged global mean surface air temperature increase reaches 2 K for the first time (for each simulation and grouped by radiative concentration pathway scenarios). The numbers below the boxes give the percentage of simulations for each scenario in which annual global surface air temperature increase averaged over 31-year periods reaches 2 K. Corresponding climate anomalies over France in winter and summer averaged over those 31-year periods: (Center) surface air temperature (K). (Right) precipitation (mm/day). The histograms show: [min, 25%, 50%, 75%, max, and mean given by the asterisk].
These questions are important as lowering future emissions might delay the crossing of temperature thresholds and ease the adaptation planning (Joshi et al., 2011). For instance, Fig. 11 shows that under RCP8.5, the 2-K threshold will be crossed by all model simulations during the 21st century. The median crossing year is around 2040 with some climate simulations crossing the threshold as early as the late 2020s and some others as late as 2060s. However, the bulk of climate simulations crossing year is between 2035 and 2045. Similar behaviour is seen for the RCP4.5 with a median crossing year occurring five years later. Under the RCP2.6 emission scenario, a majority of climate simulations (∼ 60%) do not reach the 2 K threshold during the 21st century. Among those that do reach the threshold, the median crossing year is 2050 with no crossing before 2035. These results indicate that unless very aggressive mitigation scenarios (e.g. RCP2.6) are implemented, the likelihood to exceed the global 2 K threshold by 2050 is very high and almost certain by the end of the 21st century, according to CMIP5 projections. Furthermore, the regional SAT impact over France of a 2-K global warming does not strongly depend on the RCP emission pathway. Expected France warming (median value of SAT changes averaged over the crossing period) would be less than 2 K in winter and 2.8 K in summer. Indeed, under RCP2.6, France climate would then remain relatively stable while warming will continue to increase under the other RCPs. Furthermore, the median summer PR decrease for the crossing period is much reduced under RCP2.6, while it reaches −0.3 mm/day in the case of other RCPs. In any case and even if one manages to keep the global mean SAT below 2 K, an additional 1–2-K France warming (beyond the current observed change) is still to be expected and will require appropriate adaptation measures.
7 Summary and perspective
Using observations and results from the recent CMIP5 historical simulations, we have first compared observed and simulated climate changes for France over the 20th century. Analysis suggests that the only natural external forcings cannot explain the observed increased warming since 1980 and that the latter is only consistent with climate simulations including the anthropogenic forcing. A seasonal analysis suggests that natural internal variability also contributed to the winter and summer SAT observed multi-decadal fluctuations. Observed PR trends have much smaller amplitude than the very large PR interannual variability, which prevents robust detection of any low-frequency trend. Analysis of climate projections under the RCP8.5 scenario suggests for France a 4.5-K annual mean warming at the end of the 21st century. Projected summer and winter SAT increases are 6 K and 3.7 K, respectively. Summer PR is projected to decrease by 0.6 mm/day, contrasting with the winter increase by 0.3 mm/day. The inter-model range is huge, indicating large uncertainty as to the amplitude changes. Uncertainty quantification indicates that SAT reflexive uncertainty has almost the same magnitude as epistemic uncertainty at the end of the 21st century. SAT aleatoric uncertainty is comparable to the epistemic one for the near future and remains stable along the full 21st century, in contrast with the two other sources that largely increase. PR aleatoric and epistemic uncertainties are similar for the near future, while the reflexive source is not detectable. PR epistemic uncertainty is almost of the same magnitude as the PR projected changes at the end of the 21st century. While end of the-21st-century winter PR reflexive uncertainty is very weak, the summer one is as important as the epistemic source. We also suggest that process-based metrics are a useful way to constrain epistemic uncertainty and projected changes. We illustrate it with SAT summer changes and their links with the interannual SAT–cloud cover relationship in the current climate. We conclude by an estimation of a global 2-K warming threshold crossing time according to the different RCPs and France related climate change. 21st-century global mean (as well as mean France) warming is expected to exceed the 2-K threshold by 2050 under either RCP8.5 or RCP4.5. Enforcing strong mitigation measures (RCP2.6 pathway) would allow us to remain under, or just slightly exceed, the threshold. Under RCP2.6, France winter and summer warming at the end of the 21st century would be around 2 K, respectively.
Future work will focus on further reducing epistemic uncertainty using process-based metrics to better constrain France climate projections. Work is also ongoing to estimate whether one can reduce aleatoric uncertainty for the next decades. The strategy is based upon constraining near-term climate predictions using observed initial ocean, atmosphere and land states (Meehl et al., 2009). Finally, our results have also implications with regard to regional modelling. Caution is needed when selecting the few global model drivers in order to sample epistemic and aleatoric uncertainty and avoiding an underestimation of the range of projected regional climate changes. While regional models might provide a more accurate representation of extreme events, land–sea and land–orographic effects, they indeed add another layer of uncertainty (in addition to that due to global climate projections) to the regional and local projections of climate variables needed for impact studies.
Acknowledgements
This work was supported by the BNP-Paribas foundation via the PRECLIDE project under the CNRS research convention agreement No. 30023488. It has also been partially supported by the French National Research Agency (ANR) in the framework of its JCJC program (ECHO, decision no ANR 2011 JS56 014 01). The authors thank the two reviewers, Hugues Goosse and Matthew Collins, for their insightful comments, which greatly helped to improve the manuscript. Discussion with Aurélien Ribes (CNRM/Météo France), Anne-Laure Gibelin and Brigitte Dubuisson (DCLIM/Météo France) provided valuable input. The authors also acknowledge the World Climate Research Programme's Working Group on Coupled Modelling, which is responsible for CMIP, and thanks are also due to the climate modelling groups for producing and making available their model output. For CMIP the US Department of Energy's Program for Climate Model Diagnosis and Inter-comparison provides coordinating support and led development of software infrastructure in partnership with the Global Organization for Earth System Science Portals. The authors also acknowledge the Division of Climatology at Météo France, who provided the datasets of observed temperature and precipitation over France, and Jean-Claude André for insightful comments.