1. Introduction
A Ms7.0 earthquake occurred near Jiuzhaigou in the Sichuan Province of China on 8 August 2017. The focal mechanism of the earthquake indicates that it is a left-lateral strike-slip dislocation event with a nearly upright fault plane [Xie et al. 2018; Liang et al. 2018]. The seismogenic fault is the northern extension of the Huya fault [Xu et al. 2017; Ji et al. 2017]. This earthquake may be the result of the northeastward extrusion of the lower crust of the Qinghai Tibet Plateau [Li et al. 2017]. By analyzing both the Jiuzhaigou earthquake and the 2008 Wenchuan earthquake via the Coulomb stress method, previous studies proposed that the continuous action of the viscoelastic lower crust increased the Coulomb stress to the point where it eventually resulted in failure and rupture in the Jiuzhaigou area [Cheng et al. 2018]. An alternative theory for the cause of this earthquake involves the accumulation of stress and strain due to the ongoing eastward movement of low-strength material in the middle and lower crust near the Minjiang fault [Xie et al. 2018]. However, these macro-scale analyzes of the ambient tectonic conditions in this region do not examine the crustal movement or other stress and strain changes in the time leading up to the earthquake. Based on continuous seismic data recorded at stations located near the earthquake epicenter from April to December of 2017, we quantify the temporal and spatial characteristics of the crustal movement in this area and explore the formation, onset, and dynamic background of the Jiuzhaigou earthquake.
2. Data and data processing
While more standard regression analyzes can easily quantify the correlation between two sequences, it only provides information about the linear relationship between these two time series, which is not always suitable for the analysis of nonstationary signals. The mutual information technique measures the strength of both the linear and the nonlinear correlations between two time series, which provides a more comprehensive approach to understanding the relationship between these two data sets.
Shannon’s information theory provides a formula for the calculation of system information. For a signal S, the possible information sequences are {x(t)} (t = 1,2,…,n). The probability of occurrence of all this information is Px(x1), Px(x2),…,Px(xn). The average information amount of any S can be expressed as:
(1) |
(2) |
If we take S as the original data sequence {x(t)} (t = 1,2,…,n), Q is the data sequence of the time delay 𝜏 of S{x(t+𝜏)}, then the mutual information can be expressed as
(3) |
In the above formula, Psq(si,qj) is the joint distribution probability when S is equal to si and Q is equal to qj, and Ps(si), Pq(qj) is the marginal distribution probability, here, I(Q,S) can be called self-mutual information. A larger value means more overlapping information in S and Q [Fraser and Swinney 1986; Xu and Ge 2002]. In this study, the main calculation process of this study is shown in Figure 1.
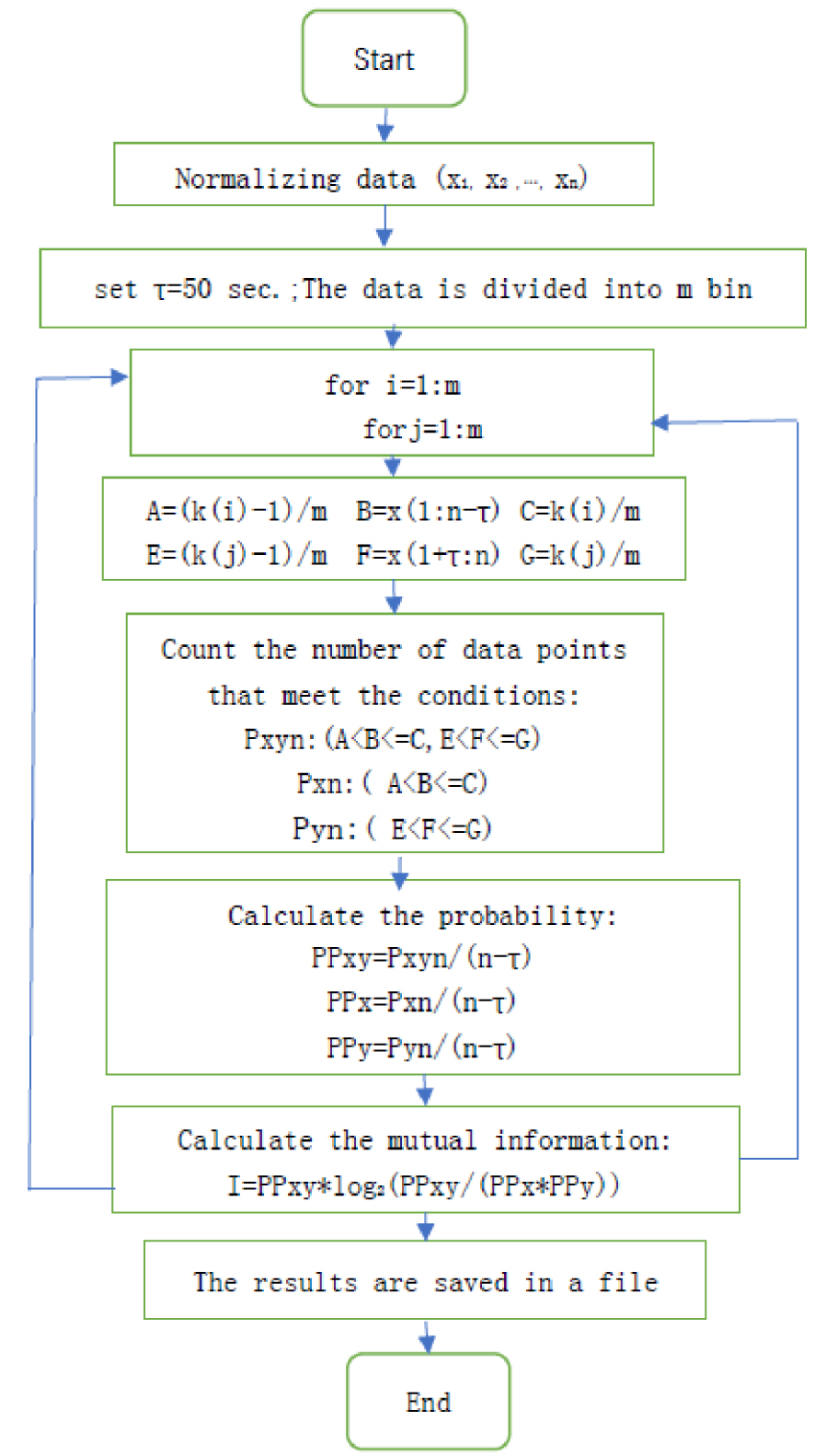
The calculation process of self-mutual information in this study.
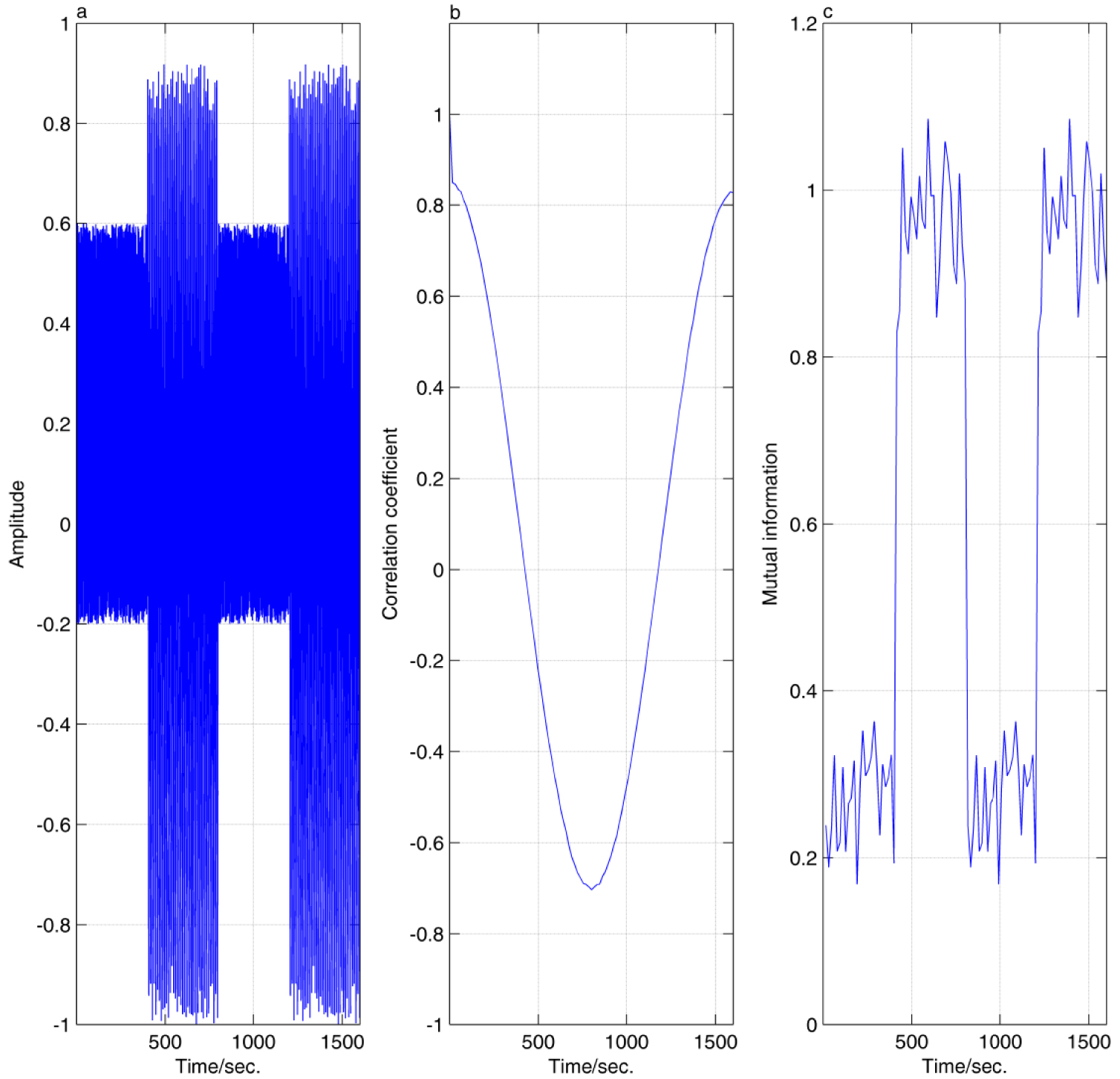
Comparison of using two methods for a simulate signal.
In order to investigate the effectiveness of the self-mutual information method, we use a simulate signal, which was processed by autocorrelation method and self-mutual information method, respectively. This simulate signal is composed of two sinusoidal signals with a period of 10 s (400–800 s, 1200–1600 s) superimposed on the random signal (Figure 2a). Figures 2b and c are the results of autocorrelation and self-mutual information processing, respectively. The comparison shows that the results of self-mutual information method better reflects the characteristics of the original signal, and it has good time resolution. Here, the time delay 𝜏 is 10 s.
A larger I value represents the persistence of a particular piece of information in a recorded signal. In this study, we analyze the self-mutual information of vertical ground motion. Our data set consists of continuous seismic data recorded from April to December 2017, at 12 seismic stations with a sampling rate of 100 samples per second. As shown in Figure 3, these stations are positioned at the intersection of the Bayan Har block and the South China block.
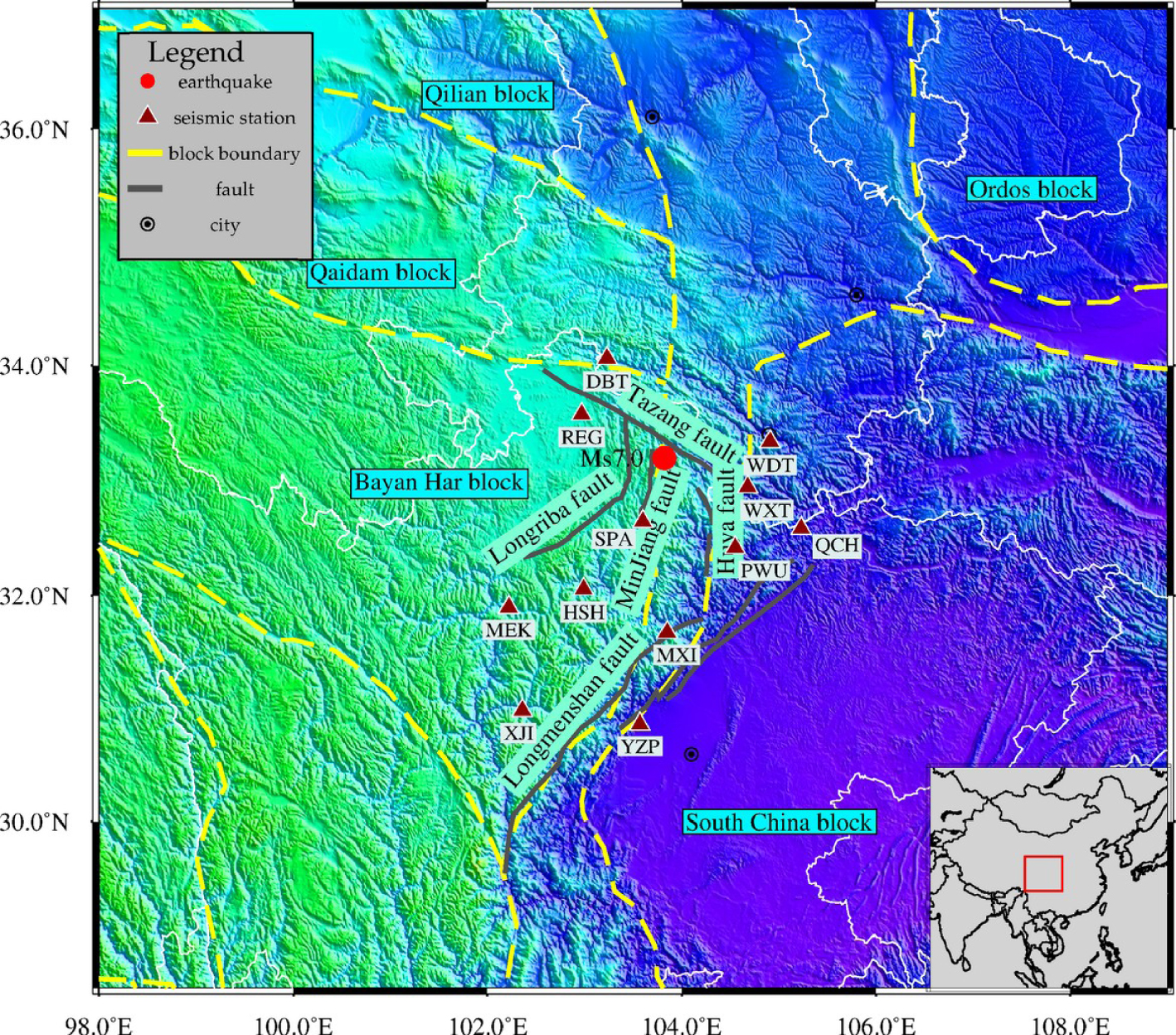
Map of our study area. The dark red triangles represent the seismic stations used in this study, and the red circle represents the location of the Ms7.0 Jiuzhaigou earthquake.
We divided the continuous records from each station into a sequence of six-minute-long time series. Considering that the maximum period of the instrument is 60 s, by applying a time delay 𝜏 of 50 s to every individual time series, we determined the signal memory of the data by calculating the self-mutual information, or the strength of the correlation, between the present signal and the past signal. A large self-mutual information value is indicative of a strong similarity before and after the signal, as well as the presence of minimal random noise in signal. In other words, a higher self-mutual information value means that the continuous vibration signal has a consistent source. We originally obtained 87,600 self-mutual information values. After smoothing our data to visualize the temporal variations in the self-mutual information values (Figure 4), we examined the spatial variation in self-mutual information values by calculating the monthly mean self-mutual information value at each station using the grid interpolation method (Figure 5).
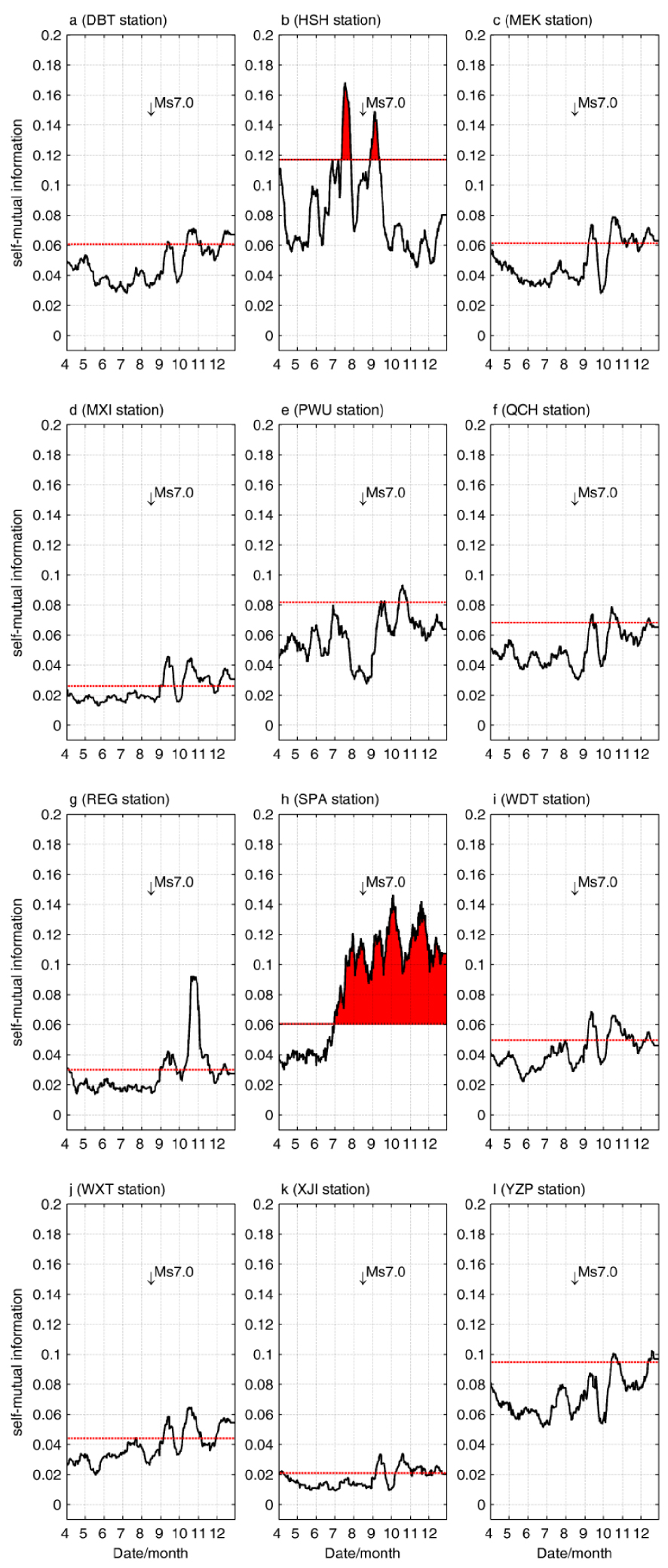
The temporal variation in the self-mutual information values for the vertical ground motion in our study area from April to August of 2017.
3. Results
3.1. Temporal variations in the self-mutual information data
To account for the expected variation in the background noise, we calculate the self-mutual information mean value of each station from April to June 2017, which is represented by 𝜎. We set an amplitude threshold of 1.8𝜎 at each station (red dotted lines in Figure 4); the self-mutual information values at stations HSH and SPA exceed these threshold values before August. The values at station HSH changed drastically in late June, with self-mutual information values reaching amplitudes twice as high as the threshold value. Similarly, the values at station SPA rose sharply in early August. The amplitudes of other stations also increased, but they were all after August.
3.2. Spatial variations in the self-mutual information data
Figure 5 shows the spatial distribution of the self-mutual information values on April, May, June, July, August and November of 2017, respectively. Warm colors indicate high values and cool colors indicate low values. We categorize the self-mutual information values as high (I > 0.1), medium (I = 0.05–0.1), or low (I < 0.05) values. In April (Figure 5a), medium values area appeared near station HSH, and the minimum values were found around station MXI. In May (Figure 5b), the value distribution is similar to Figure 5a. In June (Figure 5c), taking station HSH as the center, the self-mutual information values were increasing. In July (Figure 5d), the value of station HSH increases rapidly, and the high value area reaches station SPA northward and touches the south end of Longmenshan fault to the southeast. In August (Figure 5e), the highest mutual information values were centered between station HSH and station SPA. In November (Figure 5f), the high value area moved to the northeast, where it encompassed stations SPA, PWU, and DBT. A Ms7.0 Jiuzhaigou earthquake occurred on August 8, and its epicenter was located along the northeastern boundary of this high value area. We also noted that the high self-mutual information value area moved northward in July, which we believe coincides with the tectonic and structural events leading up to the Jiuzhaigou earthquake.
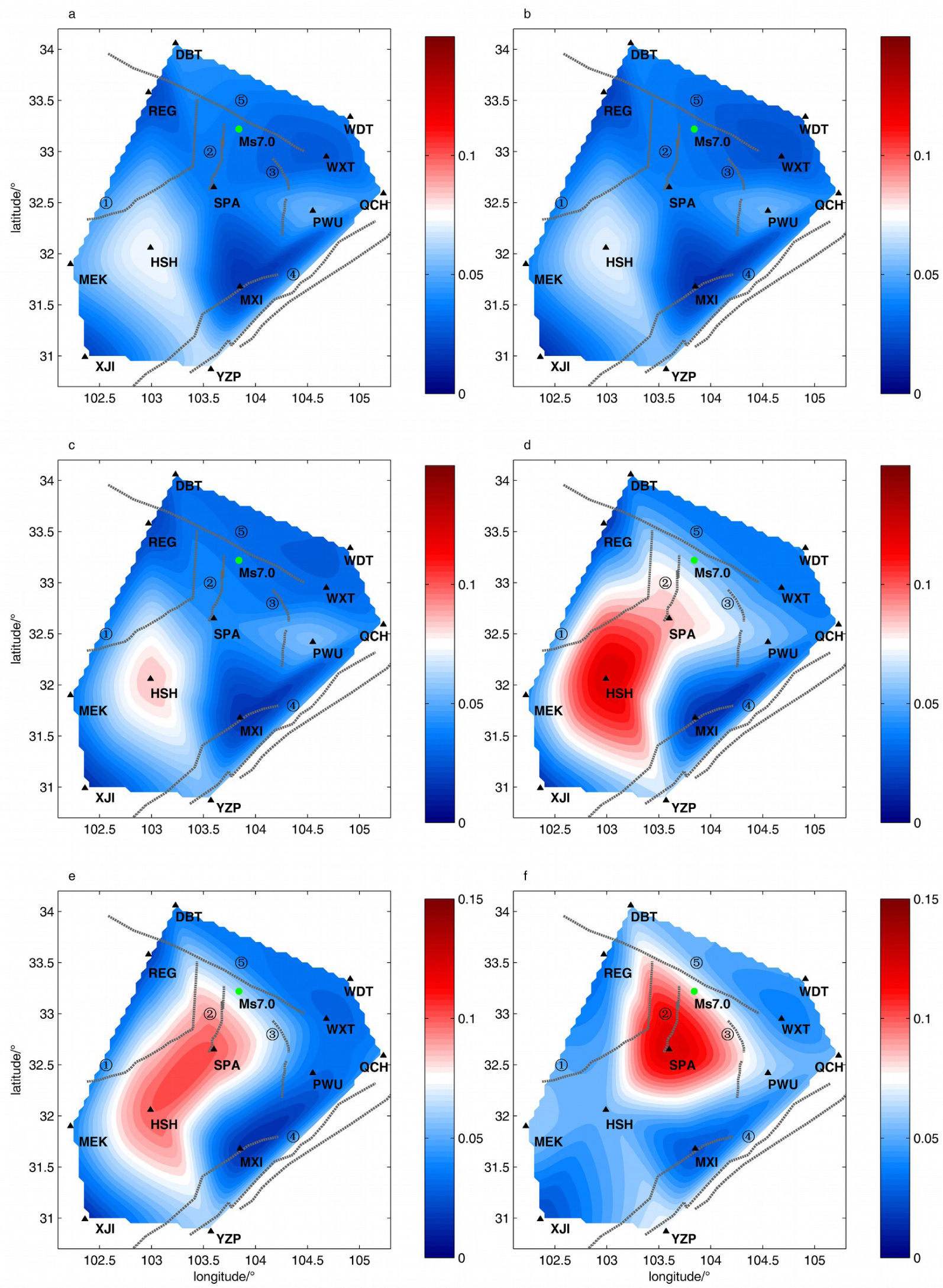
The spatial variation in the self-mutual information values for the vertical ground motion in our study area (2017). ① Longrina Fault, ② Minjiang Fault, ③ Huya Fault, ④ Longmenshan fault, and ⑤ Tazang Fault. (a) April, (b) May, (c) June, (d) July, (e) August, (f) November.
To further investigate the evolution of the self-mutual information values in the days before the earthquake, we show the spatial distribution of the daily mean self-mutual information values from August 1–8 in Figure 6a–h, respectively. During this period, the high value areas were located near station SPA. From August 1–6, there was a median value channel between station HSH and station YZP, and the value gradually increased with time (Figure 6a–f), to over 0.08 on August 7–8. On August 8, the Ms7.0 Jiuzhaigou earthquake occurred along the boundary of the remaining high self-mutual information value area. This means that the formation of this high value channel is closely related to the earthquake, or we can think that the movement of Longmenshan fault is one of the causes of the earthquake.
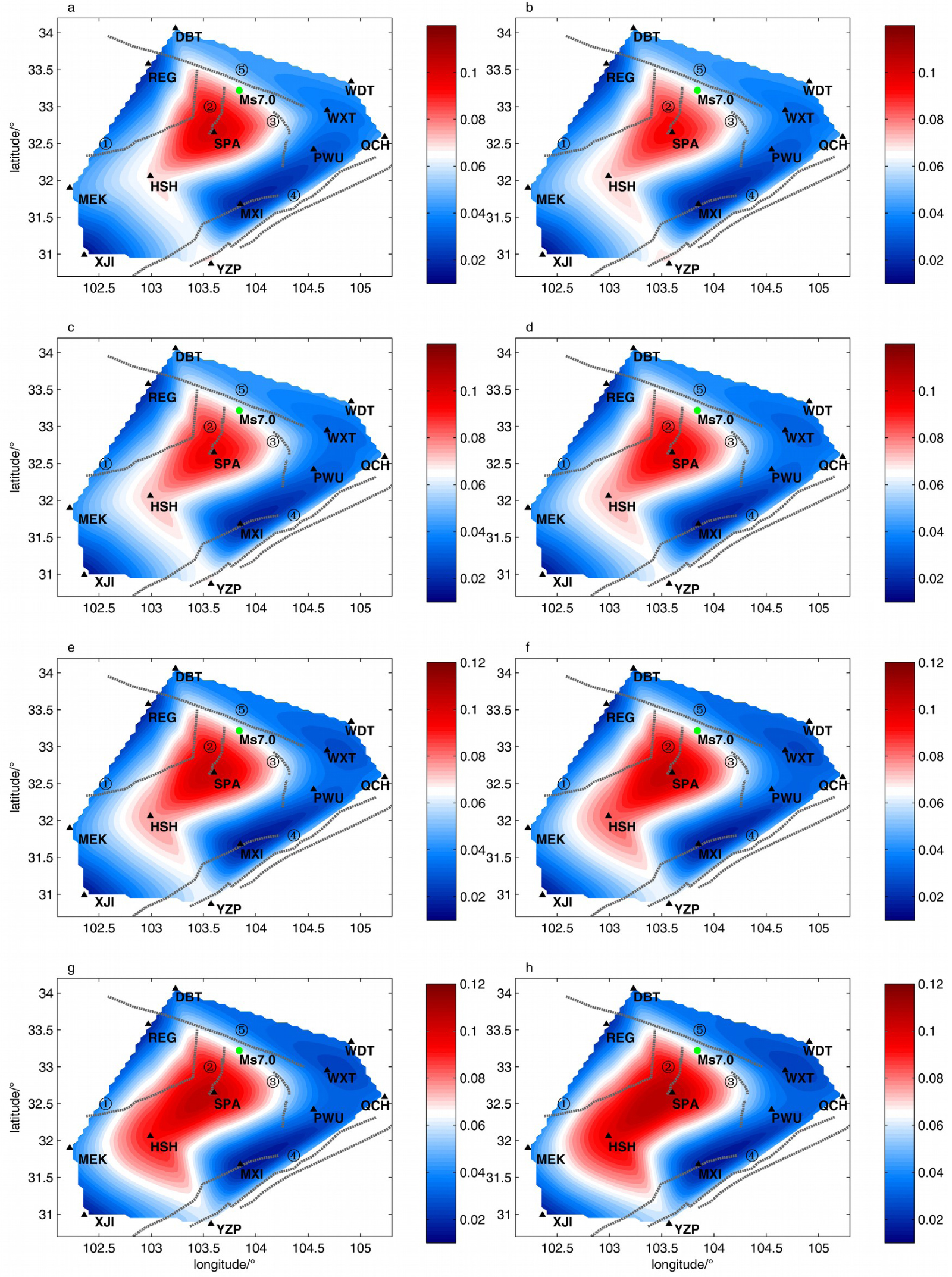
The spatial variations in the daily average self-mutual information values for the vertical ground motion in the days leading up to the Jiuzhaigou earthquake (August 1–8, 2017). ① Londrina Fault, ② Minjiang Fault, ③ Huya Fault, ④ Longmenshan fault, and ⑤ Tazang Fault. (a) August 1, (b) August 2, (c) August 3, (d) August 4, (e) August 5, (f) August 6, (g) August 7, (h) August 8.
3.3. Test of the result reliability
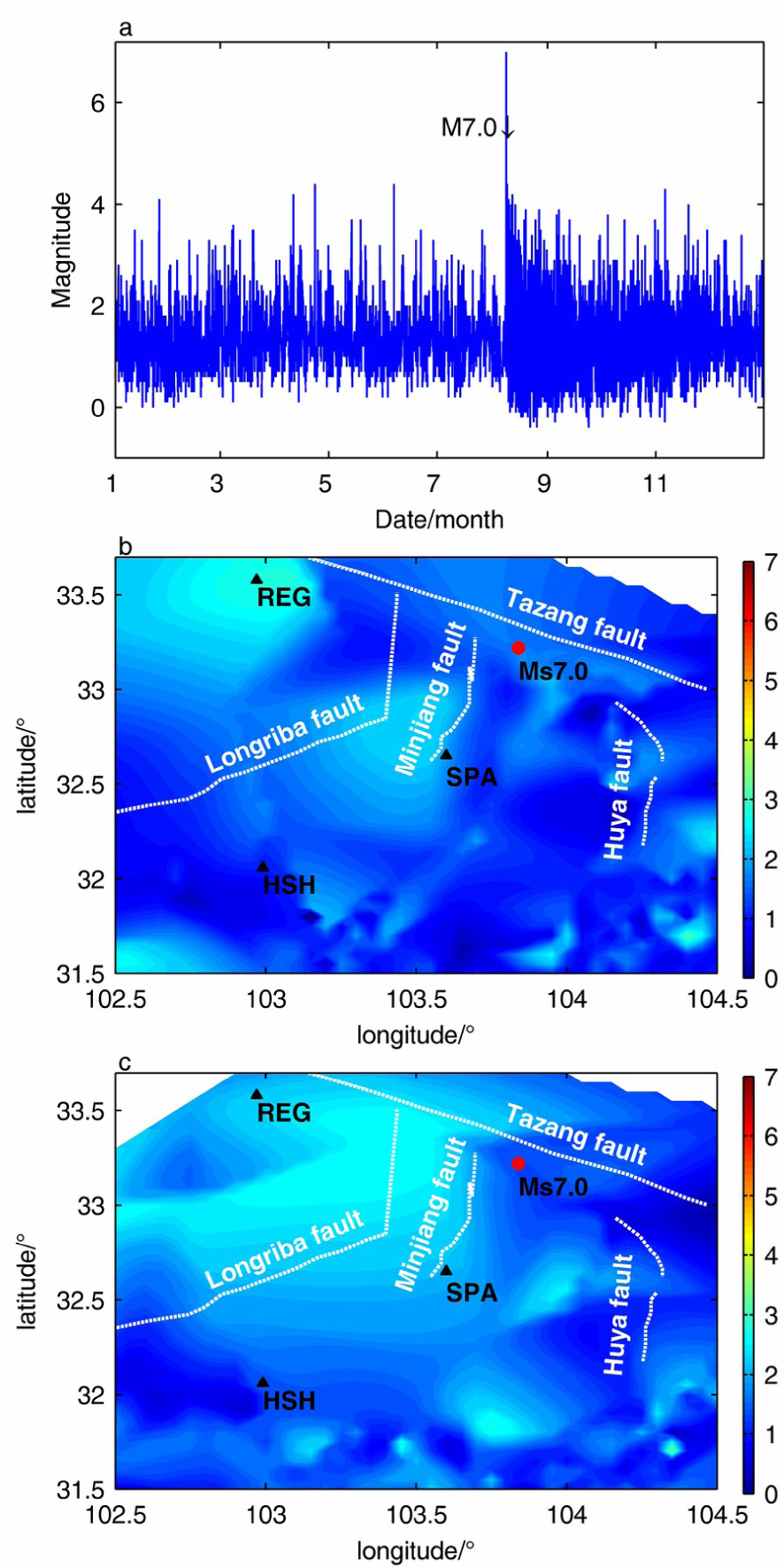
Magnitude distribution of earthquakes (2017). (a) Seismicity in this area (2017), (b) magnitude distribution of earthquakes from January to March 2017, (c) magnitude distribution of earthquakes from April to June 2017.
We examined the seismicity of 2017 in this area, and the sequence diagram of earthquakes is shown in Figure 7a (data from China Seismic Network Center http://10.5.160.18/uniteDayCatalog/index.action). We can see that there was no obvious earthquake swarm activity before August 2017. Figures 7b and c showed the magnitude distribution of earthquakes from January to March and from April to June in 2017, respectively. As shown in Figure 7b, during January to March 2017, earthquakes mainly occurred around REG station and to the west of SPA station; From April to June, earthquakes mainly occurred in the area between REG station and SPA station (Figure 7c). As can be seen from Figure 5, the high value of self-mutual information mainly changes between HSH station and SPA station. Thus, it can be considered that the results of this study have not been affected by the earthquake activity. In addition, we compared the self-mutual information characteristics at SPA from January to September 2017 and from January to September 2018. The results are shown in Figures 8a and d, respectively. It can be seen that the self-mutual information value continued to rise from July 2017, while in the same period of 2018, the self-mutual information value fluctuated within a certain range, but no similar variation characteristics were shown. Therefore, we believe that the rise process of the value in 2017 should not be the result of seasonal changes. In order to investigate the influence of the length of the data on the results, we take the data of SPA at April 2017 as the research object, and calculate the self-mutual information with the data length of 6 min and 10 min, respectively. The normalized results are given in Figures 8b and e. The mean values of the former and the latter is 0.1177 and 0.0948, respectively, and the standard deviations is 0.1167 and 0.1128, respectively. The different data lengths have little effect on the results. How do different time lags 𝜏 affect the results? We calculated the data of SPA in June 2017 with 𝜏 = 50 s and 𝜏 = 25 s, respectively. The normalized results are given in Figures 8c and f. The mean values of the former and the latter is 0.1385 and the standard deviation is 0.1593, which has little impact on the calculation results.
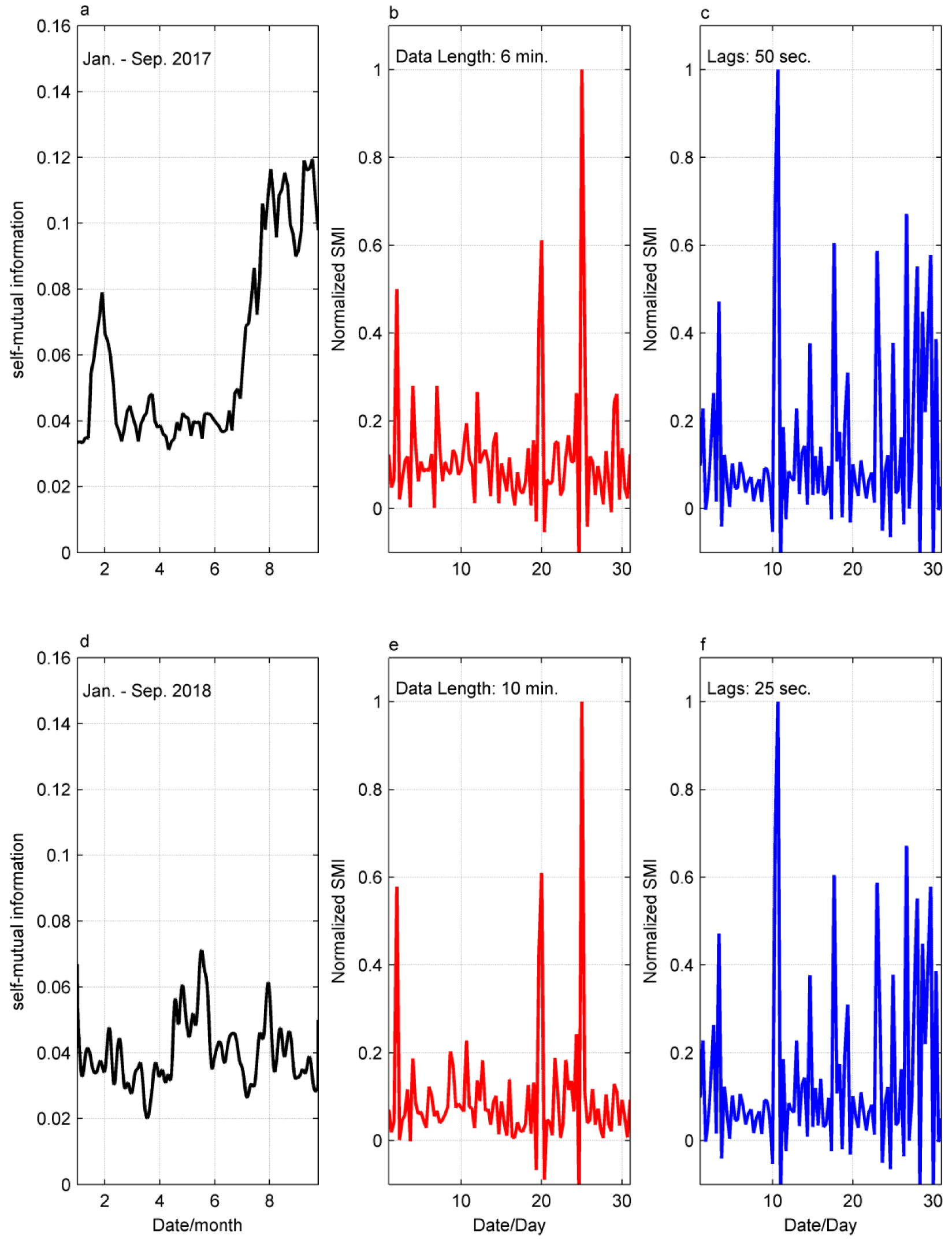
The self-mutual information test comparison of several cases. (a) The normalized self-mutual information at SPA from January to September 2017. (d) The normalized self-mutual information at SPA from January to September 2018. (b) The normalized self-mutual information with the data length of 6 min (April 2017). (e) The normalized self-mutual information with the data length of 10 min (April 2017). (c) The normalized self-mutual information with the time lags of 50 s (June 2017). (f) The normalized self-mutual information with the time lags of 25 s (June 2017).
4. Discussion
The increase of self-mutual information value means that there is continuous and homologous signal components in the signal. It should be the performance of underground material (energy) migration in a specific area, or we think it is the reflection of seismic energy convergence process, this is also consistent with the research results of other scholars.
The Ms7.0 Jiuzhaigou earthquake occurred in the collision and compression boundary zone between the Bayan Har block and the South China block. Based on our self-mutual information results, we infer that the migration of the vibration source from south to north represents the seismic energy gathering before the Ms7.0 Jiuzhaigou earthquake, and that the evolutionary trend of the vertical ground motion in this area reflects the obstruction of material in the middle and lower crust by hard material in the Sichuan Basin, a theory that is supported by the potential decoupling of the middle and lower crust from the upper crust in this region [Xiong and Teng 2002]. Based on the multi-scale wavelet inversion of the gravity field data in the Qinghai Tibet Plateau, Yang et al. identified an irregular low-density region at an equivalent depth of 19.52 km and a northeast-trending low-density region at the equivalent depth of 35.5 km. This low-density body represents a rheological layer in the crust [Yang et al. 2015]. Magnetotelluric data indicate that there is a highly conductive layer at a depth of 20–40 km in the Bayan Har block; this area may be a ductile low-viscosity layer [Zhao et al. 2012]. Some previous studies attribute the onset of the 2008 Wenchuan earthquake to the stress and energy accumulation that resulted from the obstruction of deep eastward-flowing material in the Qinghai Tibet Plateau by the rigid material of the Sichuan Basin [Teng et al. 2008; Lei et al. 2018]. Seismic observations such as the wave velocity distribution and Poisson’s ratio values along the eastern margin of the Qinghai Tibet Plateau indicate that there is a partially melted viscous fluid layer in the middle and lower crust of the Bayan Har block at a depth of 20–40 km [Zhu et al. 2017]. These results all point to the conclusion that a rheological layer exists in this region, that makes it possible for material to move.
A low self-mutual information value indicates that the signal is dominated by random noise, while high self-mutual information values indicate the presence of a consistent vibrational source in the signal. By combining our self-mutual information results with data from previously published works, we conclude that the high self-mutual information values in our study region represent the rapid eastward flow of material in the middle and lower crust of the Qinghai Tibet Plateau, which ultimately resulted in a Ms7.0 Jiuzhaigou earthquake. The spatial variation in the self-mutual information values shows that between late July and early August increased values appeared between station HSH and YZP. This evolving trend represents activity at the end of Longmenshan fault system, which contributed to or triggered the Ms7.0 Jiuzhaigou earthquake. From the spatial variations in our self-mutual information values from April to July, we infer that the obstructing effect of South China block was the main dynamic background of the energy accumulation of Jiuzhaigou earthquake, and the activity of the south end of Longmenshan fault was an inducement of the earthquake. Before the Jiuzhaigou earthquake, the study on the characteristics of amplitude spectrum of ground motion in this area showed that there was a phenomenon of violent ground motion from June to August 2017, which is consistent with the results of this study [Xu and Li 2020].
5. Conclusion
We extracted self-mutual information values from continuous vertical ground motion seismic data recorded in the months before and after the 2017 Ms7.0 Jiuzhaigou earthquake. The high self-mutual information values we observe in our study area are the result of the continuous action of a vibrational source that is the seismological manifestation of the energy accumulation and release that occurred before and during the Ms7.0 Jiuzhaigou earthquake.
By combining our results with those of other published works, we infer that the high self-mutual information values in our study area could be representative of the violent movement of the deep rheological material that ultimately caused the Ms7.0 Jiuzhaigou earthquake. The obstruction of the Qinghai Tibet Plateau by the Sichuan Basin, and the compression and upwelling in the Longmenshan area are possible triggers of the Jiuzhaigou Ms7.0 earthquake.
Conflicts of interest
There was no conflict of interest in this study.
Acknowledgments
The seismic data were obtained from the Sichuan Seismic Network and the Gansu Seismic Network. Some of the figures were prepared using the Generic Mapping Tools program (http://gmt.soest.hawaii.edu/projects/gmt/wiki/Download, last accessed June 2019). We express our thanks for this. Besides, we thank LetPub (www.letpub.com) for its linguistic assistance during the preparation of this manuscript. This study was supported by the Science and Technology Project of Gansu Province (21YF5FA031). The authors also thank the editors and reviewers for their comments on this manuscript.