1 Introduction
During the recent years, the rapid development of the telemedicine (which consists in remote consultation, diagnosis and therapy using telecommunications procedures) pushes direct exchanges between the patient and the medical provider located in classical care structures (GP, hospital physician...). Medical monitoring thus becomes more frequent and effective, costs are reduced, diagnosis is faster, and patients in remote areas are covered. This is especially crucial for elderly patients living at home. After first research works at the beginning of the 90’s 〚1〛, a home tele-monitoring system (the ‘Health Integrated Smart Home Information System’, HIS2) 〚2–10〛 has been recently developed at the Faculty of Medicine of Grenoble. The system has been conceived to respect ethical constraints 〚11–14〛 and to be acceptable by the patient 〚15〛. It contains smart sensors linked to a domestic CAN (Controller Area Network) 〚16〛, and acquires physiological data (blood pressure, heart rate, weight, SaO2, etc.). An ambulatory actimetry sensor continuously detects physical activity such as posture, body vibrations and falls 〚2〛. (Actimetry is widely used in sleep research. Actimeters are used to measure fine limb movements, usually of the wrist, which are indicative of sleep disturbance; they are small, relatively inexpensive devices, worn like a wristwatch, and easily used in the home without supervision. They log and store data for many nights – editor’s note.) These data are transmitted to a personal computer (the ‘Homecare Station’, HS) using the Microsoft Windows operating system. Monitoring software is used based on LabVIEW™ instrumentation software. It downloads and saves the data from the different sensors in an interoperable XML file.
We will detail in the following the general concept of Health Smart Home (HSH), then present the sensors at the three, generic, vegetative and specific, levels. After, we will show alarm triggering procedures and give some examples of new medical knowledge we can extract from the long-time observation of patients at home.
2 The concept of Health Smart Home (HSH)
One of the first causes of hospitalisation in chronic or degenerative diseases is a major disorder at the individual or social activity level, like frequent falls or impossibility to have a social life because of incontinency, important memory loss or aggressiveness. The concept of Health Smart Home (HSH) has been developed in order to permit the return at home (after a sojourn in hospital or medical institution) or the stay at home (avoiding hospitalisation) by ensuring a transparent watching the general state of the patient and/or specific symptoms of particular chronic diseases 〚17〛. The sensors set needed for such a watching has to be acceptable (both in the fixed version and in the portable one accompanying the person during its displacements), needs to reassure helping people at home (family, paramedics, social home help.) and must authorise information restitution in an easy and clear way on usual devices (like numerical TV screen, watch or in case of saturation or degeneracy of certain sensorial ways, not ordinary stimulations, e.g. through skin or tongue). In order to be transparent, but useful, information communications have to be as often as possible wireless 〚18〛 (HF transmission) and miniaturised, especially in the portable version. The present equipment has been passed with success the phases 1 to 3 of the instrumentation life cycle (CAD – Computer-Aided Design – step, animal (if needed) and healthy voluntary tests) and will enter in the mono- then multi-site clinical evaluation. The industrialisation of an eventual final product will occur at the end of the present research process, first for the elderly people 〚19, 20〛 (people aged of more of 60 will represent the quarter of the French population within 12 years) and after for dedicated (especially renal and pulmonary) chronic patients. A HSH is made of a generic and dedicated intelligent sensors set 〚21〛 that we will describe below, a classical PC (Personal Computer) collecting the information and triggering alarms, if after data fusion certain thresholds are passed over. Finally, the alarms are transmitted to either a ‘hospital at home’ service, dispatching the information to the right medical or paramedical people for a rapid intervention, or to a ‘nurse at home’ association for a long-term action.
3 From generic to specific sensors
Clinical observations show that human daily activities periodically fluctuate (and are therefore predictable) according to an approximate 24 h-period 〚22〛, called circadian rhythm. It is qualified as nycthemeral when its period is exactly equal to 24 h. Imperative schedules (sleep, wake-up, meals, leisure, etc.) are based on the earth’s rotation and day-night alternation that calibrate our circadian rhythms. Our body temperature, weight, muscular force and many physiological functions follow these biological rhythms: certain variables, like temperature, have a strict endogenous control and are not sensitive to external changes and other, like heart, rate are more sensitive to an exogenous ‘Zeitgeber’ (an environmental cue, as the length of daylight or the degree of temperature, that helps to regulate the cycles of an organism’s biological clock – editors’ note) and can be entrained at periods different than 24 h. During a nycthemeral phase, the circadian variables, separated into different groups of weak or strong amplitudes 〚23〛, are defined by the following characteristics 〚24〛:
- • period: the lapse of time separating the occurrence of two identical phenomena;
- • acrophase (the occurrence time of the maximum amplitude value);
- • bathyphase (the occurrence time of the minimum amplitude value);
- • phase (the reference instant in the period interval).
These dynamical features enable the organism to respect a global homeostasis. Consequently, the essential information revealed by the biological rhythms cannot be ignored when making a medical diagnosis. For instance, chronobiological analysis based on the circadian amplitude and acrophase, in addition to the 24 h mean value of the hourly incidence of a ventricular arrhythmia in 24 h ECG records, succeeds in separating groups of patients who die or are still alive five years after a myocardial infarction 〚25〛. In order to follow the patient activity, we measure its nycthemeral variability using location sensors (infrared sensors and magnetic contacts in doors) in the HIS2. The continuous monitoring of the patient’s activity within his own environment allows the system to create a computerised application that subdivides the activity phases into hourly slices. At the end of a sufficient lapse of time, we consider that the statistical calculation of the mean value upon rapid and small rhythmic fluctuations while an hour well describes hourly biological data. Their juxtaposition on 24 h reveals the nycthemeral rhythm. Thus, data analysis is performed each hour, with a comparison between the actual hourly cycle and the usual hourly cycle according to thresholds based on the result of statistical calculations. Differences between both actual and usual cycles show deviations in the patient’s behaviour. The objective of this approach is to alert the medical provider of changes in activity or of unusual behaviour. With the elderly, for example, this system could indicate when a decrease of activity appears. The system also indicates to the provider the number of diurnal or nocturnal alerts made during the day along with their levels of importance. Tracking these variations allows close monitoring of certain disease symptoms. The sensors can be classified into three types.
3.1 Generic sensors
We can see in Fig. 1 infrared sensors giving information about the presence of the watched person in a specific room. Coupled with an accelerometer located on the body of the patient and with magnetic contacts on the room doors, they are able to detect a fall or an abnormally long or frequent presence in the toilet for example.
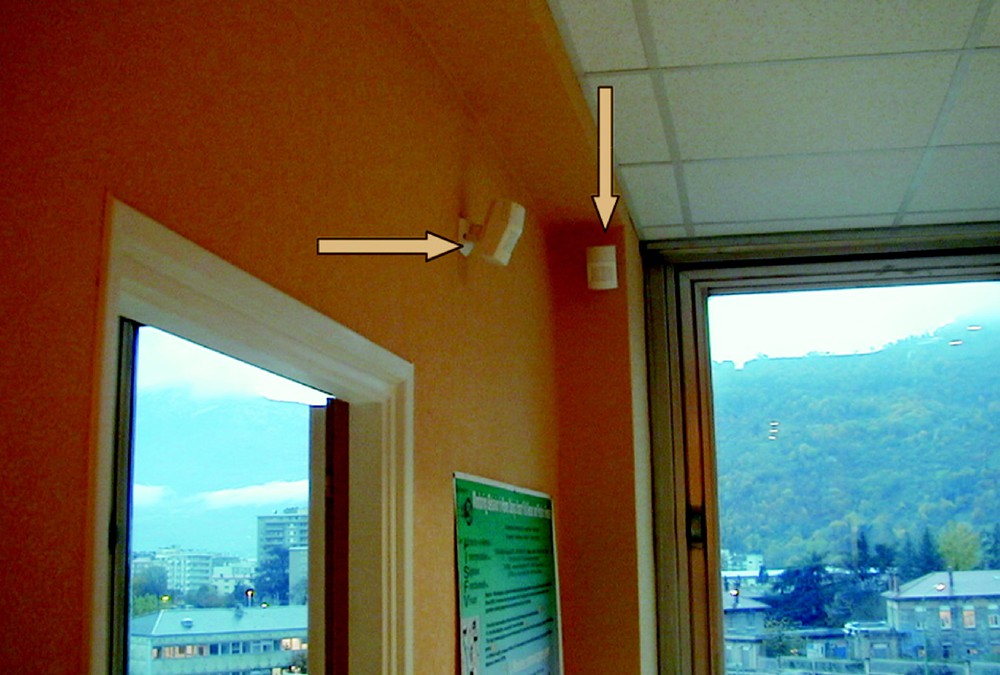
Activity generic sensors (infrared volumetric sensors indicated by arrows).
3.2 Vegetative integrity sensors
On the monitoring screen located for example at the Hospital at Home service in the closest vicinity hospital, we can watch separately the respiratory rhythm by using a portable plethysmograph giving the inspiratory air flow signal, and the most important cardiac parameters like the heart rate and the pulse amplitude (see Fig. 2 below). We will see in the following that a new knowledge can be provided by the synchronous recording of these physiological variables and that a new variable characterising the relationship between respiratory and cardiac functions permits to control the vegetative bulbar integrity.
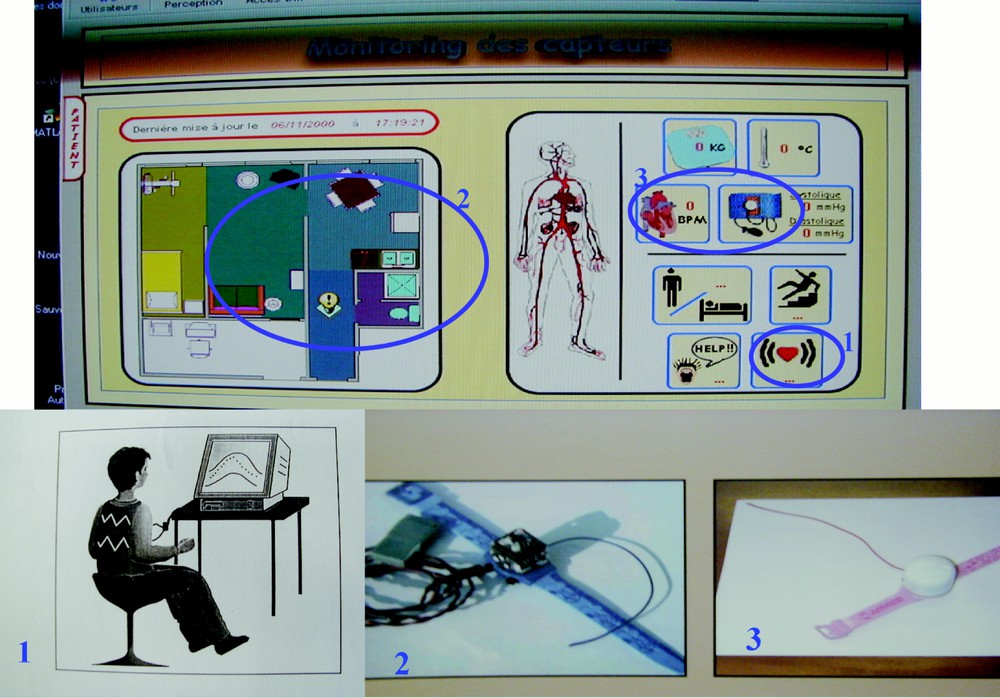
Monitoring screen of sensors above and below the respiratory plethysmograph (1), the accelerometer (2), and the heart rate and arterial pressure recorder (2).
3.3 Specific disorders sensors
An example of specific sensor is the dedicated recorder monitoring in situ the capillaro-alveolar permeability. Any inflammation of the capillaro-alveolar membrane is a sign of an increase of its permeability that leads to pulmonary oedema. It can be a symptom of adult respiratory distress syndrome or asthma, which are major concerns in public health. In classical clinical exploration, a fluorescent marker (dextran-like) is injected in the patient’s blood. The marker diffuses towards pulmonary alveoli and remains in the Epithelium Lining Fluid that covers the walls. A broncho-alveolar lavage is then performed by the physician and at regular periods some fluid is extracted for later analysis in a distant laboratory. The typical analysis consists in a fluorescence spectrometry that enables measuring the concentration of dextran contained by the broncho-alveolar fluid. The specific sensor that we propose 〚26〛 allows direct in situ recording of the dextran (or of any protein characteristic of the oedema) concentration, providing, short term, the kinetics of the marker diffusion from blood to alveoli. The corresponding device is a micro-system based on silicon micro-machining and a bio-receptor membrane made of a Mach–Zehnder micro-interferometer. A sensitive window is designed by adding a super-stratum layer on one arm of the interferometer. This super-stratum layer interacts with the evanescent part of the wave in such a way that it modifies the effective refractive index of this part of the guide. Any variation of the refractive index of one arm of the interferometer induces interferences ΔΦ, so that:
I being the intensity, λ the wavelength and L the length of the sensitive window. A sensitivity of 10–4 to 10–6 is targeted for detecting changes in effective refractive index Δneff. In order to detect the molecules of dextran, a bio-molecular film is deposited onto the sensitive window, as shown in Fig. 3. When dextran is fixed by the receptors, the effective refractiveness of the sensitive window is changed, thus generating interferences.
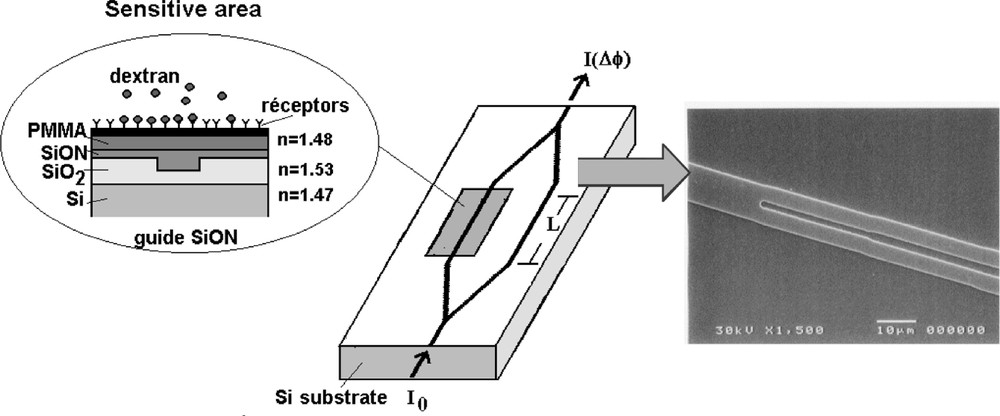
Micro-interferometer for in situ measurement of capillaro-alveolar permeability via dosage of a marker and the Y junction of the micro-interferometer wave-guide obtained by reactive ion etching (RIE) of the SiO2 upper layer on Si substrate.
4 Examples of alarm triggering
4.1 The fall
The fall is detected by combining the infrared volumetric sensors, the magnetic door contacts and the accelerometer: the corresponding data fusion 〚27, 28〛 leads to a score (whose weights can be obtained from a classical factorial analysis): we improve progressively its specificity and sensitivity by reducing in a Receiver Operating Characteristic (ROC) analysis the number of both false positive and negative triggering. Then the alarm is symbolised by the icon of a people on the ground on the monitoring screen of the Hospital at Home proximity service (cf. Fig. 4).
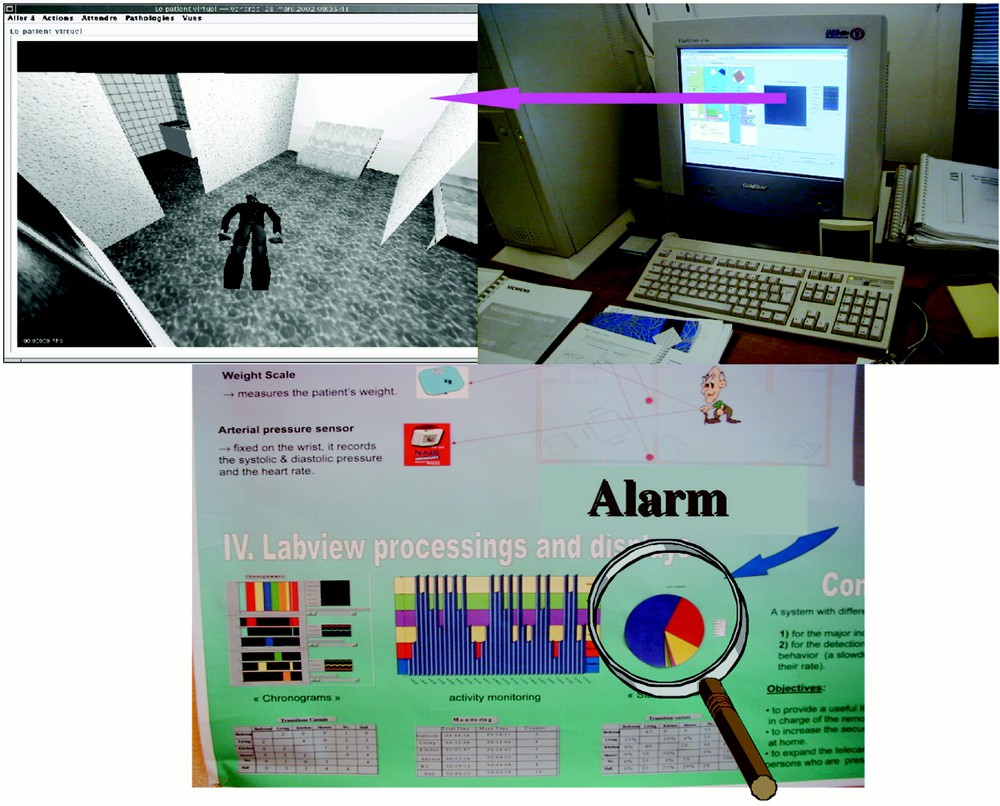
Fall detection (above) and abnormal activity compared to the canonical one (below).
4.2 The pollakiuria
From the calculations of the mean value m and of the standard deviation s of the distribution of the score of activity obtained by the data fusion described above, we define four symmetrical thresholds S1, S1* and S2, S2* with parameters μ1 and μ2:
〚S1*, S1〛 = 〚m – μ1 s, m – μ1 s〛, with μ1 a ‘benign’ parameter;
〚S2*, S2〛 = 〚m – μ2 s, m – μ2 s〛, with μ2 a ‘critical’ parameter.
The behaviour outside the 〚S1*, S1〛 interval is considered as unusual. Outside the 〚S2*, S2〛 interval, the behaviour is considered as even more critical. The threshold levels are represented by information depending on the score distribution. The results in terms of rate and profile of both occupation and information calculations are represented with the typical values μ1 = 1.5 and μ2 = 2. Although it has not yet been definitively proved through the experimentation, the distribution is probably of Gaussian type. In this case, the ‘μ1 = 1.5’ value gives the confidence interval 〚m – 1.5 s, m + 1.5 s〛 where 87% of the observations are included. A minor alert is triggered if the benign threshold is exceeded. The ‘μ2 = 2’ value triggers a major alert. The limit interval is 〚m – 2 s, m + 2s〛 and corresponds to a probability of 5% of being outside this interval. The comparison of the actual occupation rate of a room with the canonical statistical occupation profile (calculated from the normal activity periods) leads to the triggering of an eventual alert each hour. As soon as the rate exceeds one of the limit thresholds, luminous indicators (traffic lights), synonymous with (sup or sub)-presences, are in consequence triggered, depending on the importance of the alert: the pollakiuria will then been detected from significantly too frequent short stays in the toilet.
4.3 The arterial hypertension
The discrepancy measure between the normal pressure (obtained from a long period of stability) and the pathologic one permits to trigger alarms, the threshold (cf. Fig. 5) being improved by optimising the point chosen in a ROC analysis.
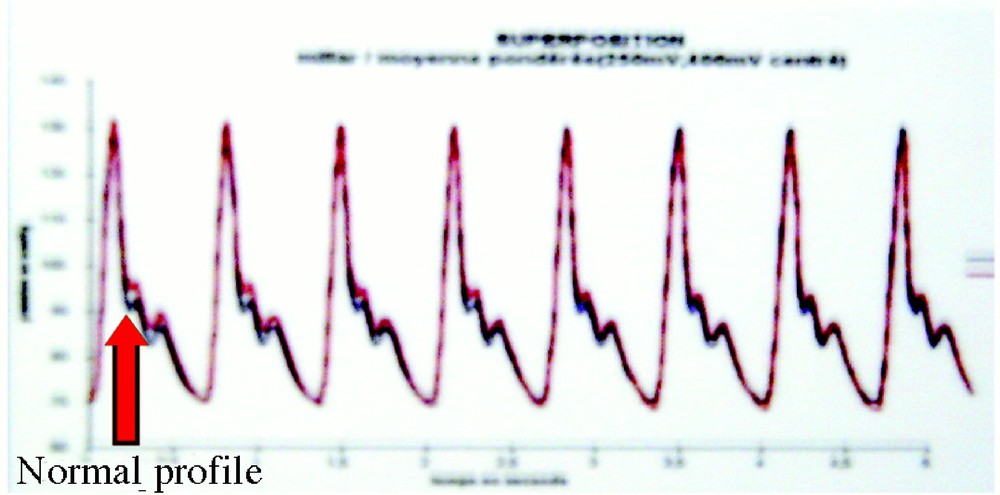
Detecting an arterial hypertension crisis by measuring the normal (red) versus actual pathologic pressure (blue) discrepancy.
5 Creating a new knowledge: from elderly people watching to the patient-centred self-information system
By observing large samples of patients we will be able to constitute standards of actimetric or vegetative values. We can then extract a physiological knowledge from these data, e.g. concerning the integration in the vegetative system of the respiratory and cardiac controls. By analysing the nycthemeral curves of a patient equipped by holter specific sensors, we observe that the cardiac instantaneous period (just the lapse of time between two cardiac beats) is anti-correlated with the time in the inspiration the actual cardiac cycle occurs (cf. Fig. 6 below).
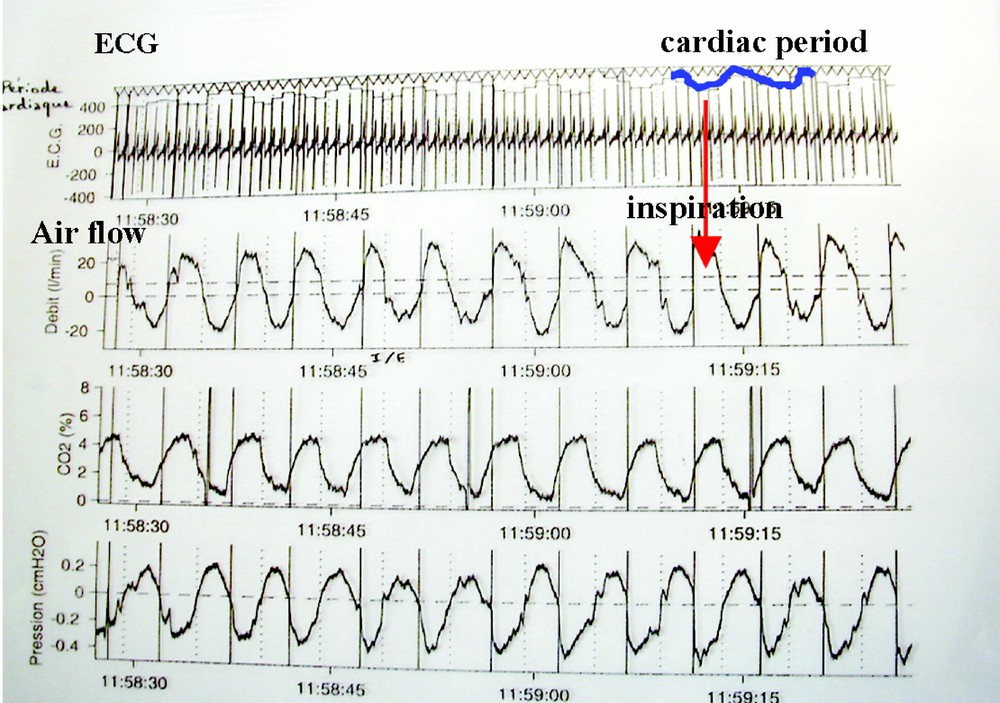
Respiratory sinusal arrhythmia detection.
Then we can calculate the intensity of the coupling (in the bulb) between the two (respiratory and cardiac) pacemakers (Fig. 7). We consider that these pacemakers can be modelled by van der Pol oscillators like two regulons (the simplest regulation systems having one positive and one negative loop 〚29〛) in interaction. Indeed, for the sake of simplicity, if I is a set of inspiratory neurons (firing synchronously with the phrenic nerve) containing auto-activatory loops interacting with E, a set of expiratory neurons (firing during the phrenic silence): the expiratory centre E is activated by the inspiratory centre I (via the pleural stretch receptors) and E inhibits I (through intra-bulbar connections, cf. Fig. 8). We neglect in this simplified description the other classical groups of neurons coming from the Richter classification, but taking into account them leads to a system of higher dimension having the same qualitative dynamical properties, in particular the entrainment ability 〚30〛. In the same spirit, by neglecting the peripheral Aschow–Tawara node, we can consider the cardiac control system as made of two groups of excitable cells, one located in the bulb, composed of neurons and called the cardio-moderator centre CM, and the other located in the heart septum and called the sinusal node S (cf. Fig. 8).
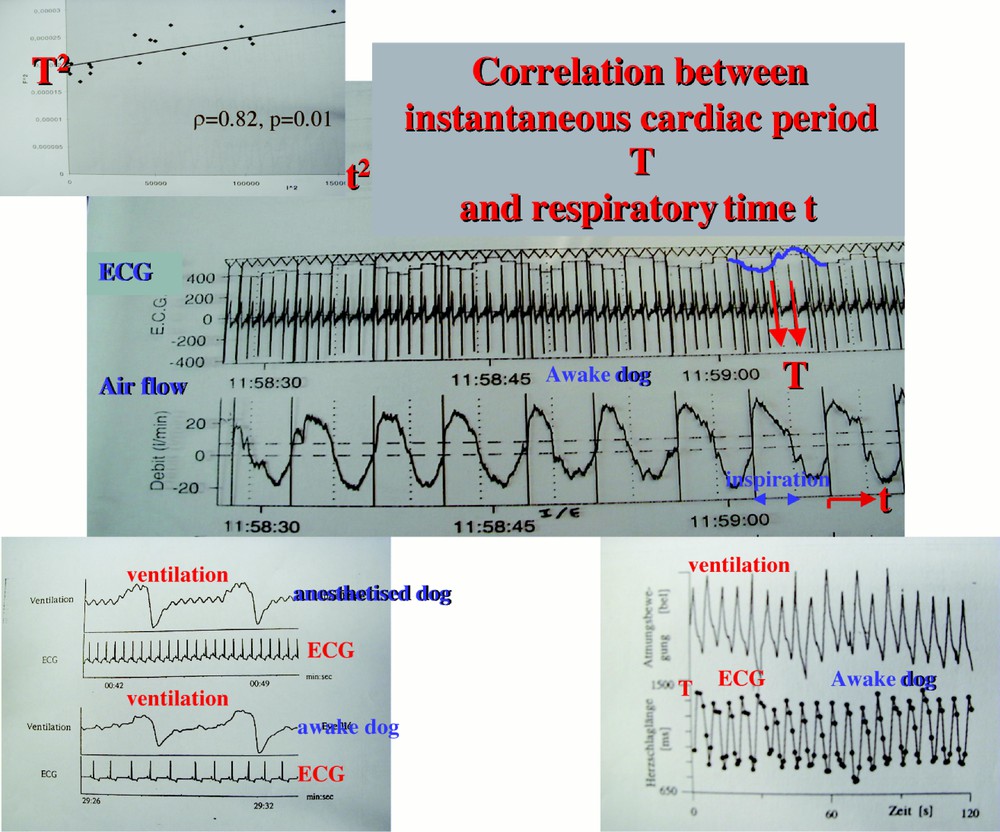
Calculation of the correlation coefficient ρ between the cardiac instantaneous period T and the actual respiratory time t.
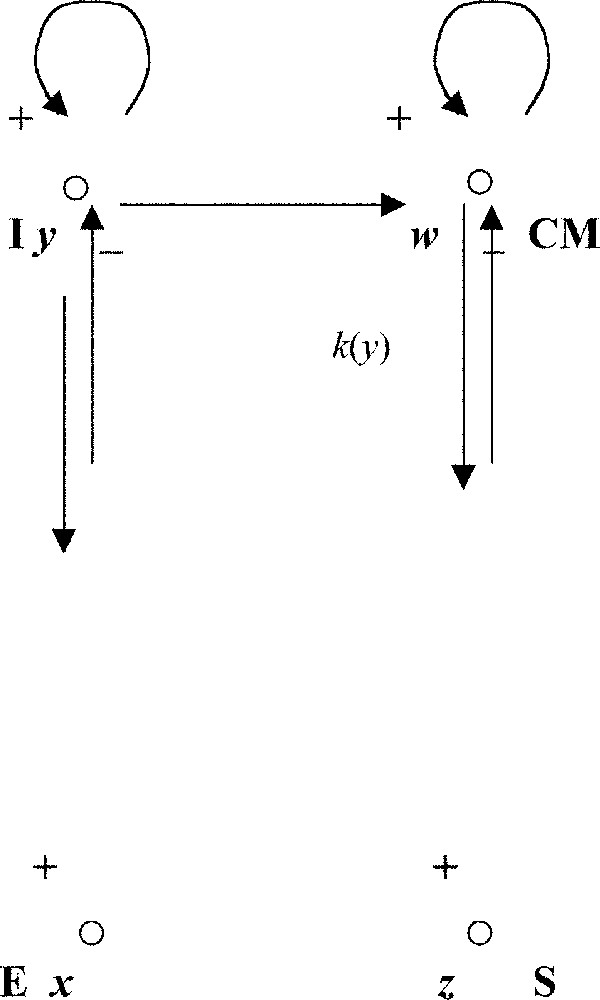
Coupling between the respiratory oscillator (left) and the cardio-moderator (right).
The van der Pol system representing the rhythmic respiratory activity reads:
where ϵ represents the anharmonic parameter of the oscillator, withy a free run (proper period) τ, equal (near the bifurcation of the van der Pol limit cycle obtained for ϵ = 0) to the ratio τ = 2π/i, where i = (2 – ϵ2/2)1/2 is the imaginary part of the eigenvalues of the Jacobian matrix of the system:
The van der Pol system representing the rhythmic cardiac activity reads:
where η is the anharmonic parameter and k(y) is the coupling intensity between I and CM. The entrained period of the cardiac oscillator is equal to:
The values of ϵ et η are fixed by the proper periods of the respiratory (4s) and cardiac (1s) oscillators, then k(y) can be obtained by measuring the instantaneous cardiac period T (which is just the inter-beats duration) and by calculating the slope of the regression line between T and the respiratory activity y (represented by the actual inspiratory time t where occurs the cardiac beat of period T). This slope is directly related to the correlation coefficient between T and t (cf. Fig. 7, showing the periodical evolution of T, which proves the coupling between the two oscillators). The integrity of the coupling allows the bulbar vegetative system to adapt to the effort: first the breathing is entrained by a muscular activity and secondarily entrains the heart. Such a capacity of adaptation disappears in degenerative diseases like the Parkinson or the diabetes. Watching a parameter like ρ is then particularly interesting in the elderly people and the HIS2 will permit the emergence of a new knowledge about the vegetative regulation.
This new knowledge allows a better restitution of information: Fig. 9 shows that we can restitute a high-level information to the patient at home by giving its respiratory rhythm (on the screen of its own numerical TV) in a ‘tube’ of admissible trajectories 〚31, 32〛 corresponding to the succession in time of the threshold alarm intervals described above. In a bio-feedback procedure, he will learn to respect the frontiers of this tube during rehabilitation sessions he can freely choose. Obtaining such tubes needs the constitution of large data basis 〚33–35〛 on different classes of patients (normal, elderly, asthmatic...).
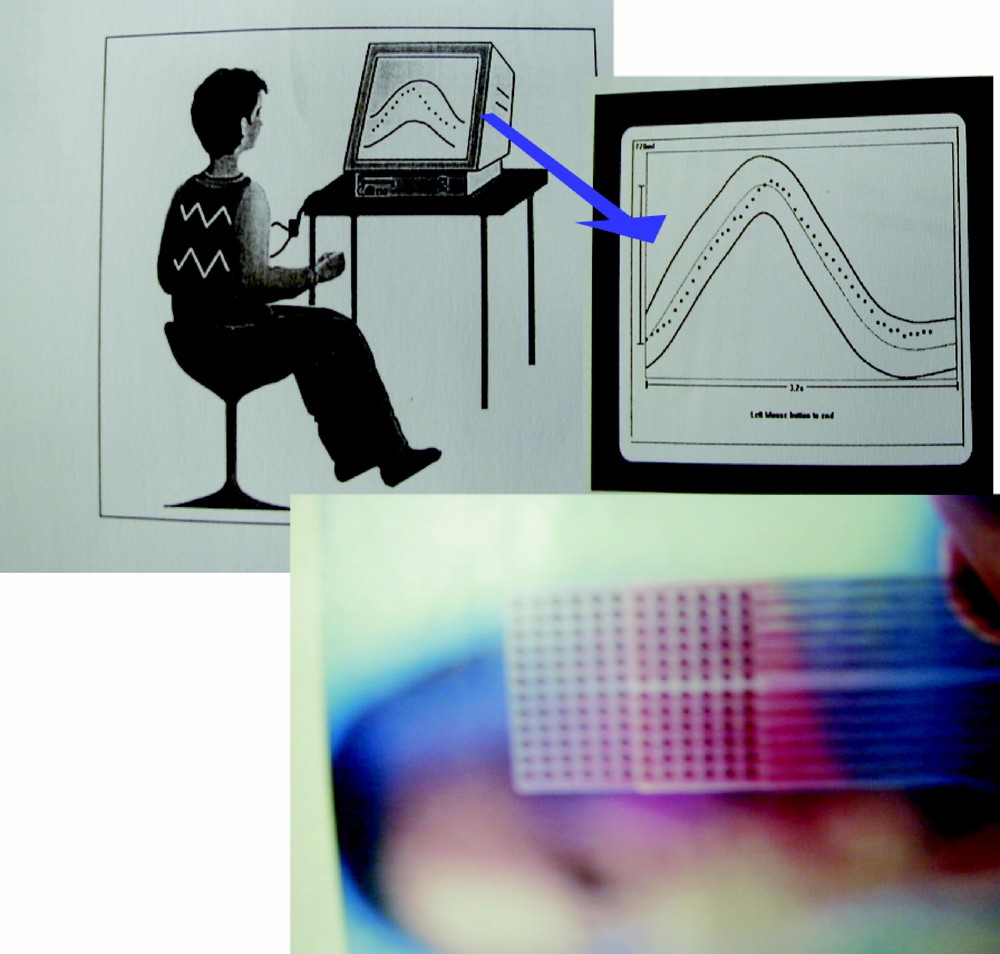
Information restitution on a screen (above) and on the tongue (below) for biofeedback learning.
The information restitution can also if the classical sensory ways are saturated or absent (macular or cochlear degeneracy in elderly people) take other sensory canals like the skin or the tongue (see Fig. 9 above): an application is for example the real time information about the friction zones (on the bed or rolling chair) recorded by specific sensors: skin or tongue sensation can lead to a direct ‘reflex arc’ correction by the patient (having for example partially lost its sensitivity).
6 Conclusion
The different phases of the person’s activity during several days have been assimilated as a succession of 24 hourly biological rhythms. We defined different confidence zones where the behaviour of the patient can be considered as ‘normal’ or ‘abnormal’. The unpredictable divergences taken into account for analysing in real time the circadian rhythm are therefore localised and interpreted as fatigue, agitation or stress. When a new patient moves into the HIS2, a new automatic learning period is required, because of each patient’s individual behaviour and activity. The correlation between the rigorous information produced by the system and the clinical reality is then performed by a physician. He will be able to certify the events occurring in the HIS2 and then to decide a return at home or a stay at home, avoiding not necessary hospitalisations and hence reducing the cost of the elderly people or chronic patients watching at home 〚36–38〛.
Acknowledgements
We have done this work thanks to the support of the National Network for Technology Research RNTS ‘Technologies for Health’ from the French Ministry of Research. We thank L. Schwartz for encouraging us and paying attention to our work during the congress ‘Sénescence’ at the French Academy of Sciences in October 2001.