1 Introduction: The problem of multiple spatial and temporal scales
The papers in this issue of Comptes rendus Biologies provide overviews of many new approaches directed toward understanding the central nervous system and its disorders. As a brief examination of the table of contents makes clear, these approaches extend from the molecular to the systems level. They are based on the extraordinary achievements in science and technology in the last half century, which have been characterized by the development of powerful techniques for the acquisition, storage, and analysis of massive quantities of data. Perhaps nothing exemplifies this better than the mapping of the human genome with its base pairs arranged into about 30 000 genes [1–3]. We have witnessed similar achievements in neuroscience, especially in the last 20 years or so, with the proliferation of brain imaging devices, with the development of sophisticated electrophysiological hardware and software, and with utilization of powerful genetic, molecular and biochemical methods.
One thing that the papers in this issue make clear is that in neuroscience, as in almost all areas of biology, we now have the ability to acquire vast quantities of data at multiple spatial and temporal scales of investigation. The spatial scales range all the way from the brain as a whole to subcellular and molecular dimensions, and the temporal dimensions go from days, months, years to submillisecond intervals. Because there is no one method that transcends all the different levels, it is difficult for investigators to interpret their data in terms of a single, unified account relating specific behaviors to their underlying neural mechanisms. Therefore, in order for neuroscience to advance, it will be essential for us to develop systematic ways to bridge these levels – to integrate neuroscientific information across them so as to generate coherent and unified accounts of the phenomena that are being investigated.
Neurodegenerative diseases provide apt examples to illustrate some of these issues. Consider Parkinson's disease (PD), which has been extensively studied and for which we now know a great deal (see Samii et al. [4] for a recent review). It has long been recognized that the essential neuropathological basis for the disease is the degeneration of dopaminergic neurons in the substantia nigra pars compacta that project to the striatum. The resulting loss of this dopaminergic striatal innervation leads to a movement disorder characterized by tremor, bradykinesia and rigidity. Sometimes patients present with other non-motor symptoms, which can include cognitive deficiencies [5]. The motor symptoms often are alleviated by drugs that replace the lost dopamine [6], although this therapeutic approach may fail as the disease process continues. Treatment for advanced Parkinson's disease includes pallidotomy [7], and deep brain stimulation of either the globus pallidus or the sub-thalamic nucleus [8]. Because deep brain stimulation has proved to be more effective, it has essentially replaced unilateral pallidotomy [4]. The cause of the neurodegeneration of the nigral neurons that is the basis of Parkinson's disease is unknown, but has been attributed to a number of factors, including aging, environmental exposure and genetic susceptibility. In their review, Samii et al. [4] point out that studies of genetic markers associated with familial parkinsonism suggest that the common final pathway leading to neurodegeneration involves the ubiquitin–proteasome system.
As can be seen is this brief and simplified description of Parkinson's disease, scientific investigation concerned with understanding the neuroscientific aspects of the disease – the basis of the disease, the cellular and molecular mechanisms that lead to neurodegeneration, the ways in which the loss of nigral neurons result in movement abnormalities, how dopamine replacement therapy first brings relief, but ultimately leads to dyskinesias and refractory motor fluctuations (on-off phenomena), and how deep-brain stimulation (or pallidotomy) may alleviate tremor and dyskinesia – must address problems at multiple levels – the genetic and molecular, the synaptic and neuronal, the neural circuit and the neural system. Results obtained at one level (e.g., dopamine replacement therapy alleviates the motor symptoms of PD) must be integrated with other results (e.g., the development of motor fluctuations after long-term use of dopamine replacement therapy).
In the past, most neuroscientists were content with qualitative arguments for bridging the various levels. However, several facts suggest that quantitative techniques will be necessary for integrating our understanding of neural systems across many of the levels of analysis. First, many of the methods now used generate rich and complex data sets, and this very complexity will often require computational methods to manage and manipulate such data. Second, these data have features that differ from one another, making them difficult, if not impossible, to relate directly to one another. Finally, neural systems do not act in isolation, but rather are part of complex networks with both feedforward and feedback interactions. Such systems tend to possess nonlinear properties that only can be dealt with in the context of mathematical analysis. Thus, one important feature of the neurosciences of the future will be the increasing use of computational neuroscientific methods in conjunction with the rich and detailed data sets that will flow from experimental laboratories.
In the remainder of this article I want to expand upon these general remarks with some specific examples, illustrating some of the work that my colleagues and I have performed that attempt to develop methods that bridge different levels of analysis, and to use these methods to understand the neural basis of certain aspects of human cognition. Of course, nobody and no single method can bridge all the different levels simultaneously. Rather, different efforts are being made by different groups to cross a few levels. In our case, we are interested in relating neuronal activity to functional neuroimaging data to specific behavior. Although this approach is just getting under way, and our initial results are modest and tentative, I think these examples represent interesting illustrations of how neuroscience will likely proceed in the near future.
2 Investigating the neural basis of human cognition
Cognitive neuroscientists have used a number of different methods to understand the neural basis of human cognitive function [9]. For a long time, most knowledge concerning the neurobiological correlates of human cognition was derived from neuropsychological investigation of brain damaged patients, by electrical stimulation and recording of individuals undergoing neurosurgery, or by examining behavior in conjunction with genetic analysis or following psychopharmacological intervention. This information was further enhanced and clarified by the analysis in nonhuman primates and other mammals of their neuroanatomical connections, neurochemical architecture, performance changes produced by focal brain lesions, and electrophysiological microelectrode recordings acquired during specific behavioral tasks. More recently, techniques like transcranial magnetic stimulation, which can produce ‘virtual’ lesions, have become valuable tools for studying the brain basis of cognition. Of course, these methods still constitute a significant component of cognitive neuroscience. However, human functional neuroimaging methods [positron emission tomography (PET), functional magnetic resonance imaging (fMRI), electro(magneto)encephalography (EEG/MEG)], developed primarily within the last twenty-five years, have produced an extraordinary wealth of new data that have added considerable information about the functional neuroanatomy of specific cognitive functions (and dysfunctions).
Several features make functional brain imaging data so extraordinary: (1) they, of course, permit one to directly have a measure of brain functional activity that can be related to brain structure and to behavior; (2) they can be acquired non-invasively from healthy normal subjects, as well as from patients with brain disorders; (3) because these data are obtained simultaneously from much of the brain, they are quite unique for investigating not just what a single brain area does, but also how brain regions work together during the performance of individual cognitive tasks. This latter point is significant because the more traditional methods used to understand the neural basis of human cognition investigate one ‘object’ at a time (e.g., the ideal brain damaged patient has a single localized brain lesion; single unit recordings from primates are obtained from individual neurons in one brain region). The potential to assess how brain regions interact to implement specific cognitive functions has necessitated the development of network analysis methods. Thus, we are now witnessing what may be conceived as a conceptual revolution in cognitive neuroscience, in that one prevailing paradigm – that of each cognitive function corresponding to an encapsulated functional (and possibly neuroanatomical) module [10] – is giving way to a new paradigm which views cognitive functions being mediated by distributed interacting neural elements [11–14].
However, all the different neuroscience methods – whether neuroimaging or non-neuroimaging based – produce data possessing differing spatial and temporal features, as well as other distinctive properties. For example, the temporal resolution of fMRI is on the order of seconds, whereas that for MEG is in the millisecond range. In essence, one can say that the data produced by each method are incommensurate with one another; that is, there is no easy way to make sure that the interpretations produced by one method lead to conclusions that are consistent with those produced by the others.
For the remainder of this paper, the functional brain imaging data I will focus on come from PET and fMRI, which are methods that measure changes in hemodynamic and metabolic activity in the brain (see [15] for a brief overview of these methods). The idea is that, because neural activity requires energy, regional increases in neural activity lead to regional changes in oxidative metabolism, which are met by increasing the local cerebral blood flow. The different brain imaging methods aid in locating the neural populations engaged by particular cognitive operations by producing maps of changes in one or another of the indicators of metabolism. The exact relationship between neural activity and altered metabolism is not well understood, but it is a topic of active research [16–18], and is discussed in the article by Nikos Logothetis in this issue [19].
To understand the neural basis for cognitive function in humans, we have used a combined neural modeling-functional brain imaging strategy. There are two kinds of modeling that we employ [20]. The first seeks to identify the brain regions that comprise the functional network mediating a specific cognitive task, and to determine the functional strength of the connections between these brain regions [21–25].
The second modeling method, which is the one I will discuss in greater detail in this article, involves the construction of large-scale, biologically realistic neural models of the cognitive task of interest in which we can simulate both neural activity, and functional brain imaging data [26–28]. This is the modeling method that enables us to bridge different spatiotemporal scales. The simulated neural activity is compared to experimental values obtained in nonhuman primates, when available, and the simulated functional brain imaging data are compared to PET or fMRI data from human subjects. The model incorporates specific hypotheses about how we think the cognitive processes are mediated by different neural components. Note that we try to phrase our assumptions in neural terms, not cognitive terms. The goal will be to have the cognitive behaviors appear as emergent phenomena.
3 Visual and auditory object perception
To illustrate the large-scale neural modeling approach presented in the previous section, I will discuss my laboratory's research investigating the neural substrates of object perception. My group is interested in both visual objects and auditory objects (for a useful discussion of the nature of visual and auditory objects, see [29]). Although many nonhuman primates show great interest in visual objects, humans appear to be unique among primates in that they have a well-developed interest in several types of auditory objects. Like visual objects (such as tables, chairs, people), auditory objects can be thought of as perceptual entities susceptible to figure-ground separation [29]. Besides definable environmental sounds, humans are particularly interested in words and musical patterns, and thus the number of auditory objects human beings have the ability to distinguish is in the hundreds of thousands. Conversely, the corresponding number for nonhuman primates is likely to be several orders of magnitude smaller; such objects likely consist of some species-specific sounds as well as some important environment sounds (e.g., sounds associated with a predator [30]). Understanding the neural basis for how such auditory objects are processed is a major challenge.
Our starting point for thinking about this problem was to examine the similarities between auditory and visual object processing. Both start as signals at the receptor surface (retina for vision, cochlea for audition). Following Roy Patterson's notion [31], a great deal of lower brain level processing occurs, resulting in the construction of what Patterson terms an auditory (or visual) image – and what I will somewhat loosely call a percept – at the level of primary auditory (or visual) cortex. However, neural processing does not stop there – rather, higher cortical areas engage in integrating the percept with other aspects of the world, at least in primates; that is, the percept is transformed into a concept (Fig. 1). Understanding the processing of auditory objects at the higher levels is critical for understanding speech perception.
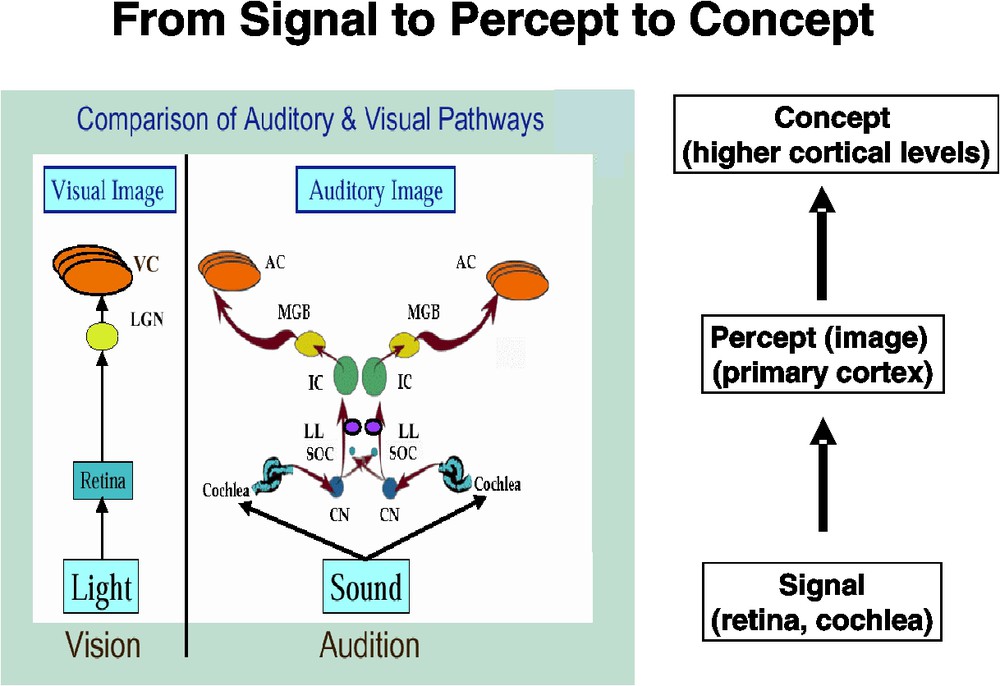
Figure showing a comparison of the auditory and visual pathways from receptor to primary cortex (left) and how this results in transforming an incoming ‘signal’ into a ‘percept’. Higher cortical levels (not shown), in turn, convert a ‘percept’ into a ‘concept’. The pathway comparison part of the figure is modified from one created by Roy Patterson. LGN – lateral geniculate nucleus; VC – (primary) visual cortex; CN – cochlear nucleus; SOC – superior olivary complex; LL – lateral lemniscus; IC – inferior colliculus; MGB – medial geniculate body; AC – (primary) auditory cortex.
It has been well known for over 20 years that there are multiple areas in the primate brain that respond to visual stimuli. The areas show both a parallel and hierarchical arrangement, forming primarily two pathways that start in primary visual cortex, as first proposed by Ungerleider and Mishkin [32]. One pathway includes regions in ventral occipital, temporal and frontal cortex and appears to be concerned with objects – processing features such as form and color. The other major pathway starts in occipital cortex and extends dorsally into parietal cortex and thence into dorsal frontal cortex. Neurons in these areas seem to be engaged in processing the location of objects in space.
Recent investigations in primates, including humans, have given rise to the hypothesis, proposed by Kaas et al. [33], Rauschecker [34] and others [35], that, like the visual system, the auditory areas in the cerebral cortex contain at least two primary processing pathways – a ventral stream running from primary auditory cortex anteriorly along the superior temporal gyrus that is associated with processing the features of auditory objects, and a dorsal stream that goes into the parietal lobe that is concerned with the spatial location of the auditory input. Unlike the visual system, this notion is more controversial and lacks strong experimental support. Nonetheless, it was the starting point for our work, which focused on the object processing pathway.
To understand the neural basis for object processing in humans, we used a combined neural modeling-functional brain imaging strategy. We constructed large-scale, biologically realistic neural models of the object processing pathways (all the way from primary cortex to the frontal lobe) in which we could simulate both neural activity and functional brain imaging data. The former was compared to experimental values obtained in nonhuman primates, when available, and the latter was compared to PET or fMRI data from human subjects. The models incorporated specific hypotheses about how we think objects are processed at the different cortical levels. We hope in the near future to expand our model to deal with speech, and to be able to incorporate and simulate EEG/MEG data.
We developed two models – one for visual object processing [27,36] and one for auditory object processing [37]. Both models perform a delayed match-to-sample (DMS) task, in which a stimulus is presented briefly, there is a delay period during which the stimulus is kept in short-term memory, a second stimulus is presented, and the model decides if the second stimulus is the same as the first. For the visual model, the stimuli consisted of simple geometric shapes (e.g., squares, tees), whereas for the auditory model, the stimuli consisted of simple tonal patterns (e.g., combinations of frequency sweeps).
The visual model [27] incorporates four major brain regions representing the ventral object processing stream [32]: (1) primary sensory cortex (V1/V2); (2) secondary sensory cortex (V4); (3) a perceptual integration region (inferior temporal (IT) cortex); and (4) prefrontal cortex (PFC), which plays a central role in short-term working memory. Every region consists of multiple excitatory-inhibitory units (modified Wilson–Cowan units) each of which represents a cortical column. Both feedforward and feedback connections link neighboring regions. There are different scales of spatial integration in the first 3 stages, with the primary sensory region having the smallest spatial receptive field and IT the largest. This is based on the experimental observation that the spatial receptive field of a neuron increases as one goes from primary visual cortex to higher-level areas [38]. Model parameters were chosen so that the excitatory elements have simulated neuronal activities resembling those found in electrophysiological recordings from monkeys performing similar tasks (e.g., [39]).
The model for auditory object processing was constructed in a manner analogous to the visual model (see Husain et al. [37] for details and parameter values). The modules we included were primary sensory cortex (Ai), secondary sensory cortex (Aii), a perceptual integration region (superior temporal cortex/sulcus, ST), and a prefrontal module (PFC) essentially identical to that used in the visual model. As with the visual model, there were feedforward and feedback connections between modules (and also specific lateral connections within some of the modules; this is different from that used in the visual model). Because auditory stimuli are perceived over time, rather than space, the neurons in the auditory model were posited to have temporal receptive fields that become larger as one goes from Ai to Aii to ST.
A functional neuroimaging study is simulated by presenting stimuli to an area of the model corresponding to the lateral geniculate nucleus (LGN) for the visual case or the medial geniculate nucleus (MGN) for the auditory case. The PET/fMRI response is simulated by temporally and spatially integrating the absolute value of the synaptic activity in each region over an appropriate time course. For simulating fMRI, these values are convolved with a function representing the hemodynamic delay [36].
There is also a biasing (or attention) signal that is used to tell the models which task to perform: the DMS task, or a sensory control task that requires only sensory processing but no retention in short-term memory. This biasing variable modulates a specific subset of prefrontal units via diffuse synaptic inputs, the functional strength of which controls whether the stimuli are to be retained in short-term memory or not [40]. Activity in each brain area, therefore, is some combination of feedforward activity determined in part by the presence of an input stimulus, feedback activity determined in part by the strength of the modulatory bias signal, and local activity within each region. Details about the parameters used in the two models, and a thorough discussion of all the assumptions employed, are given in Tagamets and Horwitz [27,40] and in Husain et al. [37].
In a typical simulation, following presentation of the initial stimulus, significant neural activity occurs in all brain regions of the model. During the delay interval, the period when the stimulus must be kept in short-term memory, activity in two prefrontal populations is relatively high, but low-level activity continues in all other neural populations. When the second stimulus occurs during the response portion of the task, neural activity again increases in all areas, and a subpopulation in PFC responds only if the second stimulus matches the first. There also is a control task, where “noise” inputs are used as inputs to the models, but no representations have to be maintained in short-term memory. Both the visual and auditory simulations demonstrate that these neural models can perform the DMS task and that the simulated electrical activities in each region are similar to those observed in nonhuman mammalian electrophysiological studies.
The simulated functional neuroimaging data for the visual model were compared to PET regional cerebral blood flow (rCBF) values for a short-term memory task for faces (the original data came from Haxby et al. [41]). The control tasks consisted of passive viewing of scrambled shapes for the model and a nonsense pattern for the experiment. When the simulated rCBF values (obtained as the temporal integration of the synaptic activity in each region over the 10 trials of the PET DMS task, which corresponds to a time interval of about 1 minute) of the two conditions were compared [27], the differences had values similar to those found in the experimental PET study of short-term memory for faces [41]. Specially, we found in V1/V2 a 3.1% change in simulated rCBF vs. a 2.7% change in the experimental data. In V4 the corresponding numbers were 5.2% (simulation) vs. 8.1% (experiment); in IT they were 2.5% (simulation) vs. 4.2% (experiment). Finally, in PFC we found a 3.5% change in the simulated data and a 4.1% change in the experimental values.
For the auditory simulation, identical stimuli (tonal contours, each consisting of two frequency sweeps, separated by a constant tone; each tonal contour was 350 ms in duration and the delay period in the DMS task was 1000 ms long) were used for both modeling and experiment [37]. Fig. 2 shows the percent signal changes (comparing the fMRI activity for tonal contours to that for pure tones) in each brain region for both the simulated and the experimental data. We chose experimental values in regions from both the left and right hemisphere to compare to the simulated data, since we had no a priori reason to restrict ourselves to either the right or the left. We also included modeling data from two separate simulations that differed from one another in the choice of the value of the attention bias parameter used during the DMS task for tones. Two main points emerge from Fig. 2. First, our simulated results in primary auditory cortex did not match the experimental value. A likely reason for this is that we included in our model only one type of neuron found in primary auditory cortex (selective for frequency sweeps), but there are many neuronal types selective for other features in the auditory input (e.g., loudness, on and off properties of the input) in the brain that we were not modeling. Moreover, there was a large amount of scanner noise during the experiment that would have had some effect on the experimental data that was not accounted for during the simulation. The second point is that for one choice of the attention parameter, we were able to get close quantitative agreement between simulated and experimental data in all the right hemisphere regions (except Ai).
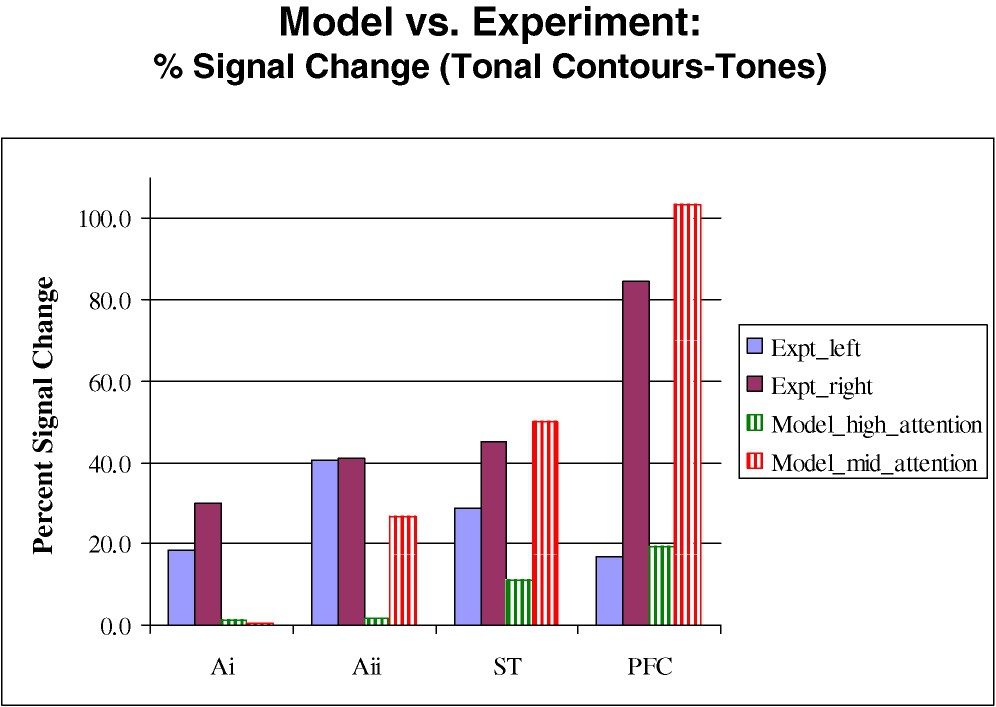
Experimental and simulated fMRI values in the different brain regions for the auditory model. Shown are percent signal change comparing the DMS tasks for tonal contours to that for pure tones. Experimental data correspond to the two solid bars on the left, and simulated data correspond to the two hatched bars on the right. The high and mid attention parameters in the model refer to the levels of attention during the tone task. See Husain et al. [37].
The results of both sets of simulations demonstrate that the neurobiologically realistic models we have constructed can generate both regional electrical activities that match experimental data from electrophysiological studies in nonhuman primates, and, at the same time, PET or fMRI data in multiple, interconnected brain regions that generally are in close quantitative correspondence with experimental values. This agreement between simulation and experimental data therefore supports the hypotheses that were used to construct the models, in particular different frontal neuronal populations interact during a DMS task, and the crucial role of top-down processing (via feedback connections) play in implementing correct performance. For the auditory model, where there is much less experimental information available concerning the neurophysiological and neuroanatomical properties of the neuronal populations involved with auditory processing, we had to make a number of assumptions concerning the response properties of model neurons. One important assumption we made, for which there was limited experimental support (e.g., [42]), was that the temporal receptive field of neurons in the auditory object pathway increased as one progressed from primary to secondary to higher-level cortex. The generally good agreement between experimental and simulated data, therefore, offers additional support for this assumption, and indicates that experimental studies in nonhuman animals testing this notion are worth performing.
4 Concluding remarks
The type of modeling that was presented in the previous section demonstrates that quantitative biological data can be used to test hypotheses concerning the relation between biological processes that cross different spatial and temporal scales. However, the models that we illustrated represent only the first steps in the direction that neuroscience will need to travel in order to provide integrated accounts across the many levels of investigation. As this type of modeling becomes more widely utilized, there are other types of data can that one can attempt to include. Currently, in our laboratory we are trying to incorporate EEG/MEG data into this framework, since we think these types of data will be essential for providing the temporal information associated with cognitive tasks that only humans can perform, such as those associated with language function. We also think that this approach will be very powerful for studying developmental and degenerative disorders. However, these types of studies are likely to require a better understanding of the role of neuroplasticity, which will need to be incorporated explicitly into our modeling framework. Finally, the neurons we currently use are relatively simple ones, so it will be imperative to incorporate neurons with more complex, nonlinear properties, including different types of receptors. When this occurs, PET studies using a variety of radiotracers and ligands to examine receptor properties will become key sources of data, and will allow one to investigate pharmacologic effects. Many of these experimental approaches will be discussed in other papers in this issue.
Finally, I would like to conclude with a general comment. As I stated at the beginning, the problems neuroscience faces for the future are really problems that many sciences, but especially biology, also face. As has been noted by many others, one of the key problems is converting what is known about local interactions at one level into an understanding of how a more global system behaves. Perhaps the simplest example is that although we may know the sequence of amino acids that comprise a specific protein, this information does not, as yet, enable one to know how this sequence folds up to produce a molecule with a specific structure and function. Scientists are getting better and better at determining the nature of the local interactions. In the neurosciences, we can record from single neurons, we can investigate with great detail the properties of individual receptor molecules, and so forth. A significant problem is to determine how all these local interactions become orchestrated into specific global behaviors. Our approach has been to investigate this problem using detailed and somewhat biologically realistic simulations. I believe that it is through these computational and simulation approaches that progress will be made in relating local to global behavior.
Acknowledgments
I would like to thank my collaborators who have been instrumental in developing the simulation method described in this paper, especially Drs Malle Tagamets and Fatima Husain. I also want to thank Dr Husain for reading and commenting on a preliminary version of this paper. I also am grateful to Dr Roy Patterson for permission to modify a figure of his for incorporation into Fig. 1.