1 Introduction
The necessity to solve complex problems has led research in biological sciences to turn to mathematical and computational approaches, which allow rigorous testing of hypotheses. Modelling can help coping with the wealth of data from complex gene networks and the many environmental factors involved. Such flexible tool can be used to test simple hypothesis as well as, when the regulation pathways become increasingly investigated, their further refinements with novel experimental data and hypothesis. Some mechanistic models of plant development incorporate genetic regulatory networks, such as those controlling flower morphogenesis and root hair development in Arabidopsis (Arabidopsis thaliana) [1–4]. There are also examples of qualitative genetic networks that have been converted into quantitative predictive tools, such as that of Welch et al. [5] which predicts flowering time under the control of seven genes and temperature in Arabidopsis.
In plant breeding, developing a new cultivar with improved yields under field conditions is a long and difficult process, particularly due to strong genotype × environment interactions. A systems biology approach can utilize the broad amount of data at different scales to optimize the breeding strategy (e.g. [6]). Here, we present the example of using modelling approaches as a tool for the development of new winter pea cultivars.
Pea (Pisum sativum), the most widely grown European grain legume, both a crop and a model species already characterized by large amounts of experimental data, represents a good candidate for new breeding strategies derived from systems biology approaches. As a crop, most pea cultivars currently grown in Europe are spring varieties, which are sown after winter frosts. These varieties were selected for traits such as early flowering, one-axis architecture and high grain yield. However, spring pea varieties are characterized by late maturity and less effective radiation used in comparison to a winter crop (Fig. 1). This can result in unstable yields between years and sites [7].
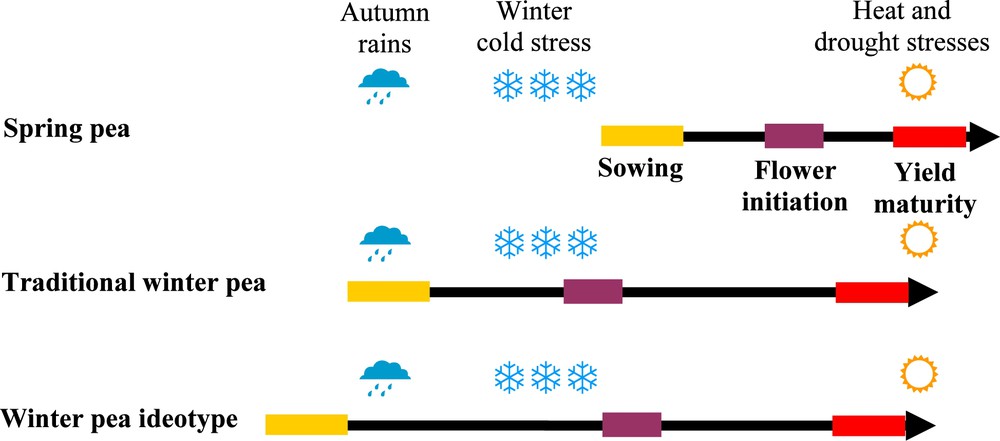
The winter pea strategy: manipulating the dates of floral initiation and flowering in order to escape winter frosts as well as drought and heat stresses in late spring.
Breeding strategies ongoing at Institut national de la recherche agronomique (INRA) aim to increase and stabilize yields for pea crops. This could be achieved through the release of winter cultivars, as has been done, for example, with wheat and canola. Escaping the harmful effects of drought and heat stresses in the spring throughout an earlier flowering period, together with a better use of enlightenment during grain filling, would increase the reliability and productivity of such winter grown pea cultivars [7]. Fall-sown peas are however limited by frost together with other stresses associated with hard winter climatic conditions, including wind dehydration, photoinhibition and diseases [8]. For the last 30 years, winter dry pea cultivars have been bred progressively for better frost tolerance, but so far have never reached the level of tolerance expressed by some forage lines. Given that frost sensitivity increases after floral initiation [9], previous observations have shown that such frost tolerant forage peas are able to escape the main winter freezing periods by delaying their floral initiation under short days [10].
For the winter pea crop, the manipulation of flowering regulatory genes appears to be a promising way to control key developmental stages, like the dates of floral initiation and flowering. One of the most crucial developmental transitions in flowering plant is the switch from vegetative to reproductive development. The process involves the quantitative integration of signalling responses to environmental cues with an endogenous developmental program [11,12]. Correct timing of this transition is critical to maximize reproductive success and thus has a major impact on crop performance. The ideal winter pea should initiate its flower primordia late enough to avoid winter frosts, but flower early enough to escape drought and heat stresses in late spring. A good combination of alleles should allow accurate control of flowering time in the ideal winter pea candidates. The breeding strategy chosen by INRA is currently based on two key flowering genes in pea, namely HIGH RESPONSE TO PHOTOPERIOD (HR) and LATE FLOWERING (LF). The strategy is to combine the dominant HR allele which confers high response to short days (SDs) [10] with an appropriate allele of LF which governs the plants' “inherent lateness” [13,14].
Integrating the knowledge of the genetic control mechanisms governing flowering gained in model species such as Arabidopsis with what is already known on these genes in pea should help to better examine and manipulate flowering genes for the development of a successful winter pea strategy. More precisely, a comprehensive understanding of the genetic and physiological basis of the interaction between flowering genes and the environment is essential to the appropriate selection of varieties finely adapted to their specific environments. For flowering time in pea, such models will provide robust support to test hypotheses derived from experimental data together with accurate predictive tools for breeding strategies.
As a model species for developmental studies and a crop, pea has been used to investigate both genetic and environmental regulation of flowering time. These studies resulted in many available experimental data on flowering in pea, such as the node of flower initiation (NFI) for different genotypes under various environmental conditions and the flowering behaviour of pea cultivars under a wide range of temperatures and photoperiods. These data led to the development of different models for flowering time in pea including conceptual models for the genetic regulation of flowering time [15–17] and ecophysiological models predicting the time of flowering according to environmental conditions [18,19]. A systems biology project is currently carried out by INRA to develop a model that would integrate the hypotheses that best represent our current understanding of the genetic and physiological regulation of flowering time in pea.
In this paper, we introduce the first computational model for flowering time in pea, which was recently published [20] and corresponds to the first step of this systems biology project. Furthermore, we present the perspectives for this systems biology project in light of the recent molecular understanding of flowering control in pea.
2 Development of the first computational model for the genetic control of flowering in pea
The genetic regulation of flowering time has been extensively studied in pea for decades, using physiological and genetic approaches on flowering mutants. More than a dozen major flowering loci were previously identified, mapped and characterized and these analyses led to the development of a conceptual, qualitative model, herein referred to as the classic model for the control of flowering in pea [17]. This classic model was based on the concept that two graft-transmissible signals are synthesized in the leaves and move towards the apex to regulate flowering (Fig. 2a). These signals were a flowering inhibitor produced by a system of three genes SN [14,21,22], DNE (DIE NEUTRALIS) [23] and PPD (PHOTOPERIOD) [24], and a flowering stimulus produced by the gene GI (GIGAS) [25] (Fig. 2a). Pea is a facultative long-day (LD) plant and photoperiod was hypothesised to act by maintaining a high level of flowering inhibitor under LDs, hence delaying flower initiation under unfavourable conditions (Fig. 2a) [22,26]. In addition, HR was thought to have little effect in LDs but dramatically delays flowering time in SDs [27] by acting in the inhibitor pathway (Fig. 2a) [26,27]. Results suggested that flower initiation was triggered when the flowering signal, defined as the ratio of the stimulus to the inhibitor, exceeded a certain critical threshold at the apex (Fig. 2a) [13,14]. This threshold was thought to be determined by LF [14,28].
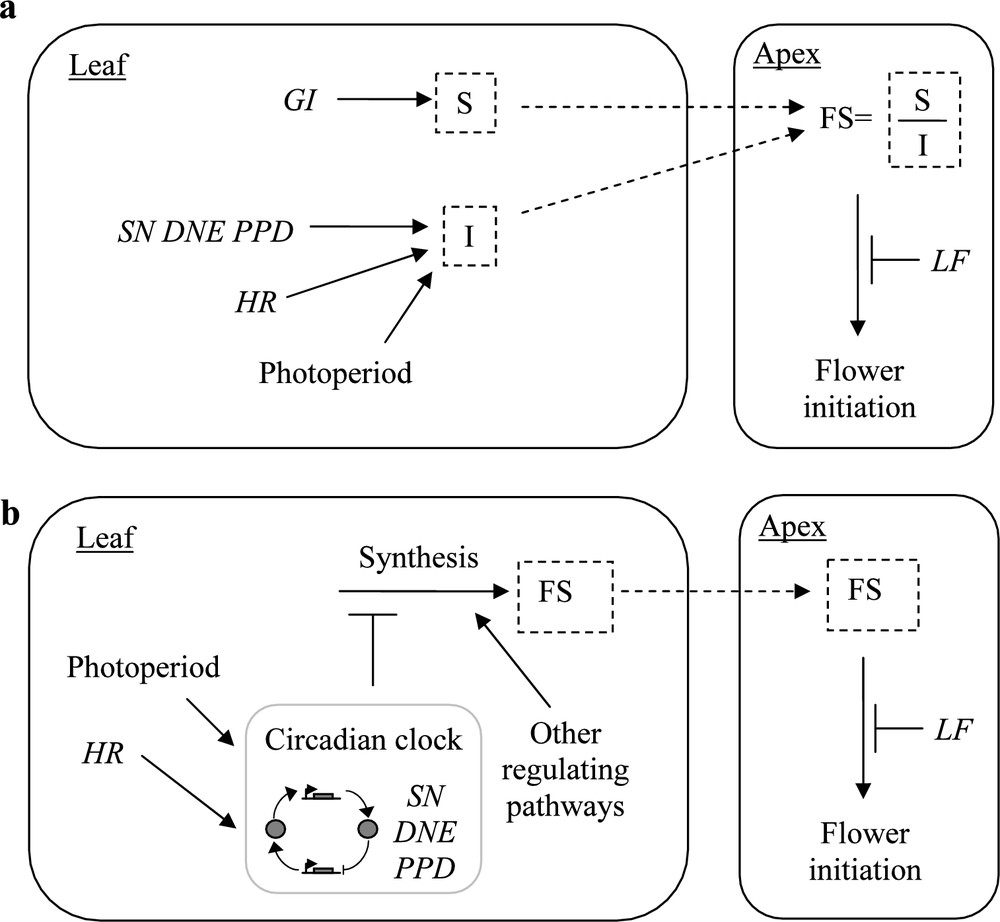
Models for regulation of flowering in pea. (a) Schematic representation of the main hypotheses of the classic model for the control of flowering in pea: a mobile flowering inhibitor (I) and mobile flowering stimulus (S) are integrated as a flowering signal (FS) which is equal to the ratio S/I. Signals and processes are under genetic and photoperiod control (mobile signals are indicated in dash lines, and genes in italic). Arrowheads indicate a promotion, while flat-ended lines represent repression. Based on classic hypotheses, the model simulates; (b) new conceptual model for regulation of flowering in pea. The synthesis of a putative mobile flowering signal (FS) is under genetic and photoperiodic control via the circadian clock. GI: GIGAS; LF: LATE FLOWERING; SN: STERILE NODES; DNE: DIE NEUTRALIS; PPD: PHOTOPERIOD; HR: HIGH RESPONSE TO PHOTOPERIOD.
As such, the classic model for the regulation of NFI in pea, derived from experimental results, was conceptual. A first computational model, recently published, was developed to test whether the classic model for flowering regulation in pea, based on the wide set of genetic and phenotypic data, quantitatively accounts for all NFI data [20]. Results indicated that new hypotheses, in addition to those already described for the classic model, were required that explicitly described the signals. In particular, the key flowering gene HR was hypothesised to interact with the photoperiod pathway to control flowering. The computational model was tested against biological data used to optimize the parameters set and accurately predicted the first node of flower initiation for a wide range of genotypes and photoperiods [20].
This first computational model for flowering in pea provided a preliminary scheme for more complex models derived from new data. In particular, recent molecular data indicate that new hypotheses on the photoperiod control of flowering in pea will renew our vision of the classic inhibitor and stimulus pathways [29,30].
3 Perspective: improving the pea flowering model
In the past, despite the accumulation of considerable physiological data on flowering mutants in pea, the lack of molecular information on the genes involved held back further study. More recently, molecular analysis of flowering in pea was accelerated by the extensive work done on flowering in model species including Arabidopsis, in addition to the developing sequence databases for model legumes and the well documented synteny between pea and Medicago (Medicago truncatula) [31,32]. These ongoing results will allow improving the model for the genetic control of flowering in pea, in particular with a better precision in the mechanisms involved and the possibility of signal quantification.
3.1 Quantification of the flowering threshold
In the classic model for flowering control in pea, it was hypothesised that flower initiation is triggered when the flowering signal reaches a critical flowering threshold at the apex. Experimental data suggested that this flowering threshold is determined by the different alleles of LF [13,28]. Four main naturally occurring alleles of LF have been defined: lf-a, lf, Lf, Lf-d, each with increasing dominance conferring increasing NFI and hence delaying the time of flowering [33,34]. Even though LF is a key gene in the control of flowering time in pea, its participation in mechanisms controlling flower transition are still unknown but recent data allowed a better understanding of its action. The LF locus, however, is notable as the first of the classical pea flowering gene to have been identified at the molecular level as a homolog of the Arabidopsis flowering inhibitor TFL1 (TERMINAL FLOWER1) [35]. Molecular analyses indicated that NFI was correlated with LF expression level in pea [35], where very early flowering genotypes displayed no expression and the late flowering genotypes were characterized by a high level of expression. These results suggested that LF alleles, and therefore the flowering threshold, were determined by the amount of LF transcripts. Consequently, further experiments were carried out to study LF expression and its response to development and environmental conditions as a marker for the flowering threshold. Consistently with the classic model hypotheses [20], preliminary results suggest that LF does not show any marked developmental or environmental regulation (V. Hecht, J. Weller, B. Wenden, unpublished). Consequently, quantitative molecular data for LF expression levels will allow a more precise estimation of the model parameters for the flowering threshold.
3.2 A precise understanding of the photoperiod pathway
As has been shown for LF [35] and LATE1 [36], cloning flowering key genes in pea allows insights into the molecular mechanism underlying the regulation of flowering. Data obtained in the LD plant Arabidopsis suggest that the majority of early-flowering photoperiod-insensitive mutants have primary defects in the circadian clock. More recently, many different Arabidopsis flowering gene homologs were isolated in pea [37] and their functions are being analyzed using forward and reverse genetics and expression analysis [30,36]. Consistently with what was shown in Arabidopsis, recent results allowed identification of pea flowering mutants with defects in rhythmic expression of circadian clock genes involved in the control of flowering, including the sn, dne and ppd mutants and a novel pea flowering mutant, late1 [30]. In particular, LATE1 gene was found to be the pea ortholog of Arabidopsis GI (GIGANTEA) [36], which is involved in the circadian clock and photoperiodic flowering [38]. Together, these recent results suggest that circadian clock is a key player in the regulation of flowering in pea. Taking into account circadian clock data will allow improving the computational model for flowering time control in pea. In addition, for the mechanisms that are not yet understood in pea, the model can be complemented by hypotheses derived from the extensive work carried out on Arabidopsis.
As a facultative LD plant, Arabidopsis flowers earlier under LD conditions than under SD conditions. CO (CONSTANS) is considered as the central component of the photoperiod pathway in Arabidopsis, because it is essential in discriminating between LD and SD conditions due to its regulation at different levels. First, the clock acts to establish a rhythm of the CO gene expression such that maximum transcript levels occur during the night under SD conditions and coincide with light under LD conditions [39–43]. Second, photoreceptors act to control the stability of CO protein, leading to its degradation in the dark. The combination of these regulatory mechanisms results in accumulation of CO protein specifically under LDs, when it activates the transcription of flowering promoter, including FT (FLOWERING LOCUS T) that acts as an integrator of various flowering pathways [43–46].
Whereas an equivalent circadian clock output component, which would behave like the Arabidopsis CO has not yet been identified in pea, several FT homologs were identified in pea [36,37]. Therefore, the model for flowering in pea can be improved based on the hypothesis that flowering time response to photoperiod in pea is controlled by circadian clock (Fig. 2a) and a similar mechanism to what has been proposed for Arabidopsis (Fig. 3). The pea flowering genes SN, DNE, PPD and HR are known to be involved in the photoperiodic control of flowering and to be related to the circadian mechanism. Consequently, in the improved model, they are hypothesised to be part of the circadian clock which controls the rhythmic expression of a putative output gene (Fig. 3). Similarly to the Arabidopsis CO, the output gene would encode a putative output protein that is degraded at dark, but when produced at light activates the production of a flowering signal.

New perspectives for the computational model for flowering in pea. Circadian clock, including SN, DNE, PPD and HR genes, controls the rhythmic expression of a putative output gene that, at light, activates the production of a flowering signal FS. In the computational model, area under the output gene expression curve in coincidence with light is daily integrated for the production of FS. FS accumulates, proportionally to the coincidence between output gene expression level and light, until it reaches a threshold, determined by LF allele, and triggers flower initiation. Depending on the modelling objective, two parts can be identified in the model: (I) develop a fine molecular modelling approach to provide a flexible tool that will allow testing ongoing hypotheses, (II) develop a predictive model as a tool for breeding strategies. SD: short-day conditions; LD: long-day conditions; LF: LATE FLOWERING; SN: STERILE NODES; DNE: DIE NEUTRALIS; PPD: PHOTOPERIOD; HR: HIGH RESPONSE TO PHOTOPERIOD.
The flowering signal (FS) accumulates proportionally to daily integration of the area under the output gene expression curve when it coincides with light. Under SD conditions, the peak of expression coincides with dark, so FS accumulation is slow, whereas under LD conditions the peak of expression coincides with light and FS accumulates faster (Fig. 3). Flower initiation is triggered when FS reaches a flowering threshold, which is hypothesized to be constant and independent of environmental conditions. The level of this threshold is defined like in the classic model by a constant determined by LF allele (Figs. 2 and 3).
Furthermore, such external coincidence model allows integration of fluctuant photoperiod, and thus application to field data. New molecular data on the regulation of flowering in pea will lead to the improvement of this model, including the addition of a circadian clock module, similar to what has recently been developed in Arabidopsis [47–50], together with new data on HR gene effect and the identification of the flowering signal FS. This simple integration model will provide a flexible tool that will allow testing new hypotheses on the regulation of flowering in pea from the molecular level – the circadian clock – to the physiological level – flower initiation time. Identification and quantification of the flowering signal, however, is the next necessary step that would lead to the development of more complete models.
4 Perspective: integrating field data and ecophysiological models
An accurate timing of the transition to flowering is essential for plants to initiate their first flowers when both development and environmental conditions are favourable. As a result, flowering time has a major impact on yield in crops and has also been extensively studied in pea for agronomic purpose. In particular, much effort was put to develop tools that would accurately simulate flowering response to environmental conditions. Different models predicting the date of beginning of flowering in pea were compared by Roche et al. [18]. Several attempts were conducted to develop the most accurate model to predict the time of flower initiation, mainly based on environmental conditions. In particular, Truong and Duthion [19] developed a preliminary model based on the decomposition of flowering time into two variables: the leaf appearance rate and the node of first open flower. The node of first flower was described as dependant on NFI which was related to mean photoperiod and temperature [19]. The leaf appearance rate could be estimated using days or degree-days: models in days are very useful for crop management, whereas models in degree-days are well-adapted for crop modelling. The model with photoperiod and mean temperature as explicative variables did not give the most reliable results in field conditions whereas the best fit was obtained by combining photoperiod, the date of sowing and the latitude in the model [18]. As expected, particularly in regard to the number of parameters, mechanistic models are not always the best predictive tools. However, limited number of cultivars, only one in Roche et al. [18] and two in Truong and Duthion [19], were used to develop the models. Therefore, extrapolation of these results to other genotypes is limited, but would be possible when associating such ecophysiological models with genetic data.
Several attempts to combine ecophysiological and genetic models for flowering in pea, however, are noticeable. Berry and Aitken [51] studied different genotypes under combinations of four constant photoperiods with four constant temperatures, and developed a photothermal model to quantify the differential responses to environmental conditions by the flowering genes. This work was later completed by Alcalde et al. [52,53], who confirmed that the major flowering genes have quantitative effects on flowering time. Varietal differences in these processes have been modelled by the introduction of genetic coefficient, so named because they quantify effects assumed to be of genetic origin. However, underlying mechanisms that could explain the genetic control of flowering time are not included in the previous models, hence limiting their flexibility as well as their improvement in regards to future discoveries on the molecular regulation of flowering in pea.
Consequently and as suggested by Hammer et al., improved representation of process controls could allow more accurate crop modelling [54]. In pea, based on preliminary work introduced by Truong and Duthion [19], such an integrative approach is proposed by combining a genetic model for the time or node of flowering initiation, as introduced earlier, with a predictive model for the leaf appearance rate, expressed in thermal or chronological time. This complete model will allow an accurate prediction of both the time and the node of flower initiation and will provide an integrative tool that will be useful for various purposes, including crop models and physiological research. Hence this model could facilitate the prediction of the flowering behaviours of different pea genotypes under chosen environmental conditions. This could be done for virtual candidate genotypes under a range of different environmental conditions, allowing breeders to know the best allele combinations with the expected flowering time and environmental responses. For the winter pea, such a model might allow designing an ideal allele combination for flowering genes, especially HR and LF, that could lead to a phenotype as proposed in Fig. 1. Finally, these combinations will have to be tested in several genetic backgrounds to control any negative correlation between alleles and test for other important agronomical traits such as yield.
5 Conclusion
With current advances in mathematical modelling approaches, it is necessary to focus on the key knowledge we want to include in our model. The essential question is ‘what do we want to model and predict?’ The model output determines the level of details we are allowed in the models. For flowering in pea, the modelling approach is dual: (i) develop a fine molecular modelling approach to provide a flexible tool that will allow testing our ongoing hypotheses; (ii) develop a predictive model as a tool for breeding strategies. The approach proposed herein, as shown in Fig. 3, might be used for both purposes. For fine molecular modelling, we will focus on the circadian clock model and we will particularly test different hypotheses on the flowering signal regulation. For breeding strategies, we will use a simple rhythmic pattern for the output gene expression and focus on the interactions with environmental conditions that result in accumulation of the flowering signal and thus flower initiation.
Since the effect of environmental conditions on flowering and leaf appearance rate has been studied by ecophysiologists, and the identification of major genes involved in the regulation of flowering in pea has been carried out by geneticists and physiologists, a complete set of data does not exist. Such data would include flowering traits as time and node of flowering, together with the number of initiated nodes at the apex, and precise records for environmental conditions. More generally, as integrative approaches become critical for plant research, and even more so in the future, experts from various fields have to contribute their skills to accurately feed the modelling processes. Reciprocally, systems biology might become the Rosetta Stone of research and should foster in the future dialogue between disciplines working from the molecular level to the crop model.
Acknowledgements
This work was supported by INRA.