1 Introduction
1.1 From genes to genomics
Although the word genomics is rather recent [1], its origin goes back to the beginning of the last century, when Johannsen introduced in 1909 the concept of the gene, the physical entity that corresponds to the genetic determinant of an inheritable trait in an organism. At the same time, he coined the terms genotype and phenotype. In 1920, Hans Winkler proposed the term genome to designate the complete genetic makeup of an organism.
It became rapidly essential to identify the physical entity, the so-called hereditary material that constituted the genes, in other words the genome that defines the genotype of an organism. It took another couple of decades from Winkler's genome to the demonstration that DNA was the actual hereditary material and an additional one to establish its three-dimensional structure. This latter milestone set by Watson and Crick is usually taken as the origin of the era of molecular biology.
The initial basics of molecular biology were simple, and were usually confirmed by subsequent experimental findings. These findings consisted mainly of the discovery of the molecules and machineries involved in the processes of genome replication (DNA polymerases), gene transcription (mRNA, RNA polymerases and transcription factors) and protein synthesis (tRNAs and the tRNA synthetases, ribosomes and translation factors). The first exception came with the discovery of reverse transcriptase in the early seventies. But, most early experimental results were essentially in agreement with the Central Dogma.
1.2 The first sequencing results
Technology was progressing and RNA sequencing became feasible in the sixties and first focused on transfer and ribosomal RNAs. The first sequencing results on protein coding genes were obtained from bacteriophage RNAs [2], in the late 1960s and early 1970s. They were clearly in line with the basis of molecular biology. Amino acids were encoded according to the established code and genes were framed by the expected start and stop signals. It took another six years to obtain the complete genome sequence from the RNA phage MS2 [3]. In the meantime, the techniques for DNA sequencing had been developed [4] and the sequence of the first viral DNA genome followed less than a year later [5].
The beginning of the gene-cloning era in the 1970s was accompanied by the sequencing of the first non-viral genes and was followed by entire genomic regions [6] and more ambitious undertakings on ever-larger viral genomes. Using the dideoxy sequencing procedure developed by Fred Sanger, sequencing of entire genomes from autonomous organisms, microorganisms first, was undertaken and quickly followed by projects on multicellular eukaryotes. The sequencing of cloned genes was undertaken for various reasons. First, it gave exceedingly easier access to the amino acid sequence, including the occasional mutations, than using protein-sequencing techniques. It also provided identification of the genomic context such as adjacent control regions and neighbouring genes.
2 Complete Prokaryote Genomes: the beginning of the era of genomics in the 1990s
In addition to the basic scientific merits of microbial genome sequencing, the continuous improvements of existing technologies and the growing interest in the Human Genome Project were the main driving forces of the sequencing of complete genomes of microorganisms. The first complete bacterial genome sequences came as a surprise. Whereas other microbial genomes were being sequenced with the help of physical genomic maps and sets of ordered overlapping clones, Venter and colleagues at TIGR were able to reassemble entire genome sequences based on whole genome shotgun sequencing (WGS). The first genome sequences were thus not from model organisms, but two pathogens including a non-virulent isolate [7,8]. This necessitated solving some basic issues, namely assembling the complete genome from thousands of sequence reads of cloned fragments, setting up a strategy to fill the remaining gaps and developing algorithms to identify open reading frames (ORFs) along the DNA sequence and finally annotating those ORFs that were considered as true coding sequences (CDSs).
The presence of gaps in the sequence assembly reflected the distribution bias of cloned sequences that could only be satisfactorily solved recently with the advent of single-molecule sequencing. However, the use of a variety of palliative procedures enabled investigators to obtain complete or nearly complete sequences. Completeness is important for several reasons, among which is the experimental proof of the presence or absence of a given function but also for comparison purposes. By the end of the 1990s, the accumulation of a number of bacterial genome sequences supported a series of fundamental and general findings.
Analysis and interpretation of the sequence of complete genomes can be seen from a variety of viewpoints. The inventory of complete sets of genes of organisms was among the major and initial goals of the whole genome sequencing projects and was the first step into a deeper insight into the organism's biology. Sequence analysis also provided a completely novel representation of the physical nature (structure) of a prokaryote genome in terms of organisation, topology, number of replicons, GC content, gene orientation and so on (Table 1). Wide variation in terms of size between bacterial species emerged as the rule.
A non-exhaustive list of genomic features that can be directly accessed by sequence analysis.
Main genomic features usually accessed by genome sequencing and analysis | |
Feature | Comment |
Size | Accurate, all replicons |
GC content | Accurate |
Origin of replication | Based on GC skewa |
Protein coding genes | Rather accurate |
Pseudogenes | Rather satisfactory |
Codon usage | Accurate |
rRNAs, tRNAs | Accurate |
Non-coding RNAS | Based on RNAseqb |
Gene orientation | Accurate |
Detection of IS, mobile elements, etc. | Accurate |
Gene clusters, Operons | |
Genomic Islands |
a GC skew is defined as the measure of (G − C)/(G + C) ratio of each strand which shows a bias towards G over C on the leading strand. It enables to detect leading and lagging strands and hence the origin of replication.
b Detection of non-coding RNA genes, such as antisense RNAs, is based on the additional use of transcriptome sequence data usually obtained from RNAseq experiments.
3 Eukaryotes and the human genome
The first bacterial genomes were quickly followed by the yeast genome sequence, based on a set of ordered cosmid clones and established by the effort of a network of “cottage factories” from standard academic laboratories [9]. Altogether, these achievements set the stage for larger projects although genomes of model organisms, such as the nematode Caenorhabditis elegans [10] and the plant model Arabidopsis thaliana [11] were already in progress. The success of whole genome shotgun (WGS) stimulated dreams about the human genome and regardless of the strategy (WGS versus map-based sequencing), it seemed possible to undertake such a gigantic project, which would propel biology into big science. Walter Gilbert estimated its cost at around 1$/base, an estimate that turned out to be in the range of reality. It is not possible even to outline the history and various episodes of this project. Because of its cost, the project had raised opposition as soon as the idea was floating around and induced a fierce debate. It may be of interest to recall a few of the main arguments of the opponents.
A first criticism came from the lack of interest in sequencing at all and in particular, in promoting such a monstrous undertaking, that was just the opposite of the criteria for elegant science. This brute force project was anything but a smart idea and one could hear the litany of criticisms such as “Is this a sound scientific objective? Haven’t we much wiser priorities? This is not a way to train young students and to help them to become autonomous scientists! This will take all the resources available for biology!” And so on.
Another main criticism was based on the lack of interest expressed by many biologists for sequencing introns and intergenic regions, the so-called junk DNA. Many of these biologists argued to restrict the project to expressed sequences. In retrospect, this seems incredibly short minded and it was mainly the attitude of biologists with poor or no literacy in genetics. However, once they could find the sequence of their favourite gene in databanks, many of the early opponents quickly realized the interest and utility of such a programme.
A third argument against was based on our incapacity to properly interpret and exploit the data, and this was largely true. In addition, humans were not an experimental species. But, this also indicated that a substantial part of the effort should address the issue of getting the meaning of the sequence and extending the project to models such as mouse and rat.
Now, how to get there? Venter was convinced that WGS, which was so successful for bacterial genomes, would be appropriate for genomes three orders of magnitude larger. The launch by Applied Biosystems of a new sequencing instrument based on Sanger sequencing using capillary gel electrophoresis and incorporating a number of other improvements, coincided purposely with C. Venter's announcement in May 1998 of the creation of a private company (Celera) to sequence the human genome by WGS. This announcement, which also coincided with the main international Genome meeting in Cold Spring Harbor, was accompanied by a fierce criticism of the public project that had just started at a very low pace and was still exploring and discussing a number of practical issues.
But the competition was launched and it received at lot of coverage from the public media. In spring 2000, to keep everyone happy, both projects announced victory jointly. If one considers that a genome with some 200,000 gaps is a satisfactory goal, this can be considered as an achievement. However, this was not the case for those working in human genetics and searching their favourite disease gene, and it took another three years for the public consortium to produce a human genome sequence assembly of high quality with less than 300 gaps [12].
Did the victory claim of Venter mean a success of the WGS? Probably not, as argued by the main leaders of the public project [13]. However, the WGS strategy clearly worked for Drosophila, sequenced ahead of the human project as a proof of concept, and even for the mouse. Why did it not work for human beings, even after adding the sequence coverage provided by the data of the public project? Venter and his colleagues probably underestimated the effect of heterozygosity in their assembly. They wanted to be “universal” by mixing sequence reads from several unrelated genomes from different ethnic origins, hence choosing the worst conditions for an optimal assembly. This is also the main reason why the inclusion of the public project data was rather an obstacle [14] to the improvement of the assembly. There was probably a smarter way to make use of the public sequence, e.g., adding the Celera sequence reads to the public draft assembly, but this would have been in contradiction to the WGS strategy and appeared as recognition of its limitation.
Genomes from multicellular eukaryotes, first focused on model organisms. Later choices were mainly based on practical or economic reasons, or their special interest in evolution. Except for the fruit fly, the sequence of the first eukaryote genomes was based on sequence ready maps made of ordered overlapping clones. However, the switch to WGS occurred rapidly. Despite some drawbacks to exhaustive coverage and assembly issues caused by structural variations, repeats and heterozygosity, WGS became generally adopted because of lower cost and no need for a sequence ready map. Conversely, finishing to actual completion became more and more neglected, limiting the practical use of many of these sequences to analyses mainly at the genome level.
Annotation of eukaryotic genomes raised a number of challenges [15] mainly due to the split character of protein coding genes, intergenic sequences of highly variable length and the presence of numerous repeated sequences. The use of additional sequences from transcripts and protein sequences as a gene-finding resource has become routine since very early on in the eukaryote genome annotation process.
4 The growth of bioinformatics
Bioinformatics predated genomics, but its activity remained modest, at the margins of the core of molecular biology, although everyone was convinced that a kind of revolution was ripening. Sequences had first to be manipulated with programmes designed for alignment, assembly, completion, quality checking, etc. To handle these huge amounts of data would have been impossible without the availability of computers and the spectacular advances in computer science. The development of adequate software was rapid, despite the dearth of computer literate biologists and qualified users.
The data had to be made accessible and the free and ready access, a challenge that the sequence data banks had to cope with, was a crucial step that helped to change the mind of many genome sceptics. In general complete, though unfinished for certain projects, sequence stretches were submitted to sequence data banks. When complete, these sequences were usually annotated in a way consisting first of defining open reading frames and identifying start and stop signals. This was based on a series of algorithms that performed with a rather satisfactory accuracy. These coordinates were then mapped on the DNA sequence. Prokaryote gene identification software making additional use of transcription data, now usually produced alongside genomic sequencing, has been implemented [16]. A major difficulty, from the beginning, was to distinguish between short non-coding ORFs and actual short protein coding genes. This problem is still not satisfactorily solved, and people just prefer to dismiss ORFs below 100 codons at the cost of numerous detection failures [17].
Using sequence alignments for comparisons, it was possible to identify genes encoding known proteins (see below), rRNA-coding genes, and other biologically relevant elements, such as control signals, insertion elements, other repeated sequences, etc. Later, this information grew in complexity with the data produced through other large-scale approaches such as the HapMap and ENCODE projects [18,19] and with the numerous findings on the various types of non-coding RNA (ncRNA). The representation of these latter elements and of the epigenetic changes in databanks is still a matter of debate complicated by the tissue specificity aspect. Similarly the mapping of the numerous and large structural variations remains an issue. Very rapidly, the data flood could no longer be controlled and sequences needed to be processed by suites of software that produced automated annotations with all the inconveniences that such procedures usually generate (see below).
Frequently, additional biological information from experimental or computational origin can usefully complement the constrained indications featured in the generalist sequence data banks. In a number of instances, such knowledge resulting from secondary data processing can be found in other data resources. Some of these, such as the protein databases, remain very general, whereas many other specialized databases focus on a limited set of objects or on a single species. In addition, many of these specialized resources have a limited life expectancy because of long-term funding difficulties. The total number of such individual initiatives is difficult to estimate; a description of many of the most popular can be found in an annual specialized issue of Nucleic Acids Research. The 2016 issue features about 150 such databases. Unfortunately, not all of these resources are well curated.
The crucial role taken by Bioinformatics had to be met by the permanent availability and the continuous upgrading of data analysis tools, computing and storage capacities, public access and imaginative representation of both raw and analysed data, creating a huge demand for additional human resources. And these needs have had to be adjusted ever since, to the compelling and overwhelming increase of data production. In addition, although these resources are totally transverse to biology, they have been periodically suffering from underfunding that was sparsely allocated, especially in Europe, by funding agencies advised by wet lab biologists not always well aware of the burning issues raised by genomic data.
5 Functions encoded by genomes
5.1 How complete is the parts list of functional annotations?
Although DNA codes for much more than just proteins, we will limit this discussion to proteins. In the early days of genetics, during the century following the establishment of Mendel's laws, the genotype was an abstract notion, in which the genes could just be defined by the traits they were specifying. Genomics has just reversed this view. Genes and their products are now known by their chemical structure, but the phenotype they specify can only be predicted in simple situations in which knowledge has accumulated sometimes over several decades. Making more sophisticated predictions usually remains a guess (see below).
Two decades ago, when the first whole genome sequences became available, a large number of proteins of known function, the genes of which had already been cloned and sequenced, had been collected over time in sequence data banks. It was thus straightforward, on the basis of sequence alignments and comparisons, to assign functions to a large fraction of the proteins encoded in fully sequenced genomes. This enabled scientists to reconstruct metabolic pathways, identify a large set of other functions (informational, physiological, etc.) and afforded a global view of the organism's properties. These opportunities initiated a rush into prokaryote genome sequencing and analysis, particularly for pathogens (see below). The availability of such global representations and listings paved the way to comparative genomics for functional and evolutionary purposes. It also provided an unprecedented amount of knowledge to apprehend pathogenicity and pinpointed potential targets for the development of new antibiotics. But 20 years later, the results fall far short of the hopes…
Practically, once identified on the sequence stretch, the whole set of protein coding genes was sorted into functional bins, each bin corresponding to a category of genes involved in a particular cellular process or group of processes The outcome of this sorting is often displayed as pie charts in which the relative size of each sector reflects the life style and environmental constraints faced by the organism.
A considerable fraction of CDSs had no match in sequence data banks. These CDSs were considered as bona fide protein coding genes and were classified as hypothetical genes encoding proteins of unknown function. With the increasing amount of sequences available, homologs of a subset of such hypothetical genes could be found again in unrelated newly sequenced genomes. This increased their likelihood of being true genes and their status evolved to conserved hypothetical. At present, the fraction of hypothetical genes (conserved and non-conserved) still represents about a quarter to a third of the genes identified in any sequenced bacterial genome and has almost remained stable over time, indicating that knowledge about their real function has made little progress as can be seen in Table 2. The discovery of new functions and their integration into the physiology of a cell or organism remains a very slow process requiring innovative and carefully planned experimental approaches.
Distribution of status of proteins encoded by the genome of Acinetobacter baylyi ADP1 in four successive annotation rounds between 2003 and 2015.
Evolution of status of protein coding genes | ||||
2003a n (%) |
2006 n (%) |
2009b n (%) |
2015 n (%) |
|
Proteins | ||||
Known functions | 1150 (36) | 1150 (36) | 1180 (37) | 1223 (38) |
Putative functions | 907 (28) | 925 (29) | 950 (30) | 977 (31) |
Conserved hypothetical | 686 (21) | 893 (28) | 857 (27) | 783 (24) |
Hypothetical | 458 (14) | 220 (7) | 219 (7) | 218 (7) |
Total | 3201 | 3207 | 3196 | 3201 |
a Barbe et al., Nucleic Acids Res. 2004.
b de Berardinis et al., Curr. Opin. Microbiol. 2009.
5.2 How accurate is the parts list? Errors in annotation
The production of such huge amounts of data and analyses was paralleled by the accumulation of errors in annotations. Ironically, this inaccuracy issue in annotations was already pointed out before genome annotation, but the advent of genomics has just massively worsened the problem.
As indicated above, accumulation of data also prompted the development of automated annotation pipelines. However, these suites of algorithms, when applied to the same genome sequence, produced, at a non-negligible level, discrepant gene predictions. A clear summary of this misannotation issue was given by Andorf et al. [20]:
“Most automated approaches to protein function annotation rely on transfer of annotations from previously annotated proteins, based on sequence or structural similarity. Such annotations are susceptible to several sources of error, including errors in the original annotations from which new annotations are inferred, errors in the algorithms, bugs in the programs or scripts used to process the data, clerical errors on the part of human curators, among others. The effect of such errors can be magnified because they can propagate from one set of annotated sequences to another through the widespread use of automated techniques for genome-wide functional annotation of proteins. Once introduced, such errors can go undetected for a long time.”
Other causes often involve over interpretations based on weak sequence identity or matches involving only parts of genes/proteins. Several ways to detect and curate annotation errors have been proposed. First, errors could be less frequent if analyses would make systematic use of genomic context, metabolic pathways, protein family analysis, evolutionary information or experimental data. Also, computational approaches to checking automatically inferred annotations against independent sources of evidence have been developed. They seem efficient in detecting potential annotation errors and may offer a valuable way to address the erroneous annotation issue. All data banks rely to some extent on manual curation and sequence data banks are no exception to this last but unfortunately not least remedy. There are even occasionally some optimistic calls for a more intensive involvement of expert biologists, but Wikipedia seems more successful in recruiting volunteers.
5.3 Can we predict a phenotype from the parts list (genotype)?
The availability of complete genome sequences and of other omics data initially raised the hope of predicting phenotypes and constructing models of living systems and led to the advent of systems biology. This hope was based on the analogy that a cell is a computer that permanently evaluates its state and that of its immediate environment and acts in a logical fashion after processing this information. It was surmised that through the collection of massive amounts of data it should be possible to infer the laws and principles governing the logic of the cellular behaviour. However, 15 years later, the achievements of systems biology remain at best modest, despite the massive effort to systematically collect data in all areas of omics. A simple explanation of this failure was proposed by Brenner [21]. It globally states that the inverse problem, i.e. deducing a model from the behaviour of a complex system, is impossible, because the number of potential models is far too large and cannot be constrained without a guiding theory.
Finding the role of hypothetical and particularly of conserved hypothetical genes is a prerequisite for further progress in phenotype predictions. For many model organisms, prokaryotes as well as eukaryotes, genome-wide collections of mutants have been established using various approaches. Each object of these collections corresponds to a strain with a different mutated gene. The entire collection comprises a mutant of each of the genes from a genome. Mutations from genes fulfilling indispensable functions (essential genes) are not viable and hence absent in these collections. The collections are very useful, since a mutant can be characterized by a phenotype that corresponds to a standardized description of its behaviour under various conditions. However, this approach has its limitations, since many mutants have no specific phenotype. For reasons that are only sometimes understood they behave like the wild-type strain. In our classical neo-Darwinian conception, one gene inactivation affects one or more biological processes, but this only applies to a subset of situations. Moreover, even when single gene mutants display a manifest phenotype, this rarely provides a molecular explanation of the biochemical role of the proteins at the cellular level. There is no systematic procedure or large-scale approach that offers a general solution to this issue, which remains a major challenge. Post-genomic large-scale approaches (the various omics) or bioinformatics may nevertheless offer clues to help progress in the conundrum of unknown functions, notably in regulatory aspects.
6 Evolution
The study of evolution is one of the major beneficiaries of genomics. Comparative genome analysis has dramatically changed our perception of the evolution of bacterial genomes. The classical view of evolution in which changes occur via the accumulation of point mutations has to be extended by the inclusion of additional mechanisms that allow for the rapid gain, loss, and rearrangement of significant portions of the genome. This large genome shuffling enables prokaryotes to evolve rapidly in response to environmental changes, accounting for their wide dissemination in the biosphere.
Gene content within a single prokaryote species can thus be quite variable and for many species is continuously subject to changes principally mediated by horizontal gene transfer (HGT) (see below), duplications and deletions that appear as the three main drivers reshuffling genomes of unicellular organisms. Whereas deletions and duplications, which may involve entire genomes, remain essential in eukaryotes [22–24], the latter seem much less subject to HGT, if one excepts the invasions by various types of transposable elements, an important mechanism of speciation [25].
The process of HGT was long hypothesized before it became clearly identified by genomic sequence analyses [26]. Various methods exist based on either phylogenetic or on compositional sequence analyses, providing different views on the phenomenon which is now widely recognized as a major force shaping genome evolution. Alignment of complete genome sequences visualised by dot-plot or line-plot representations has also provided a highly revealing view of the dynamics of genome rearrangements (Fig. 1).
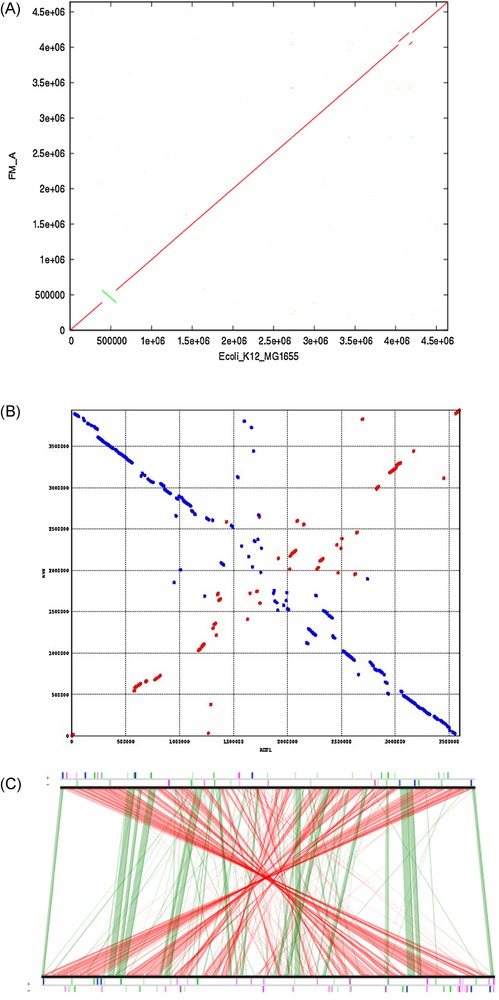
A. Dot-plot of alignment of an E. coli K12 MG1655 genome sequence along with the same genome after evolution during several months under unfavourable conditions. Each nucleotide match is represented by one dot. At the scale used, the succession of dots appears as a line. Matching nucleotides in the same orientation are in red dots, matching nucleotides in opposite orientations are in green (bottom left). Transposition of a segment results in shifts in alignments in the affected region and in interruptions of the main alignment (diagonal) (top right). B. Dot-plot of alignment of an Acinetobacter baylyi ADP1 sequence (abscissa) along with Acinetobacter baumannii AYE (ordinate). Each nucleotide match is represented by one dot as panel A. As opposed to panel A showing a very high sequence identity, the genomes of the two Acinetobacter species exhibit an important divergence shown by numerous interruptions and shifts of the diagonals. Numerous deletions, transpositions and inversions can be seen. The origins of replication are in opposite orientation on both genomes. C. Line-plot of alignment of an A. baylyi sequence (top) along with A. baumannii AYE (bottom) showing conserved syntenies. Alignment was computed by the MicroScope software by pairwise comparisons between corresponding protein sequences. Green lines join clusters of homologous genes (showing sequence conservation) in the same orientation (with respect to replication origin). Red lines join clusters of homologous genes in the opposite orientation. Linearized chromosomes are represented with their insertion sequences (in pink), rRNAs (in blue) and tRNAs (in green) genes on each DNA strand.
Genomes tend to expand and contract. It is largely admitted that reduction in size can occur in more stable and favourable environments, as seen in intracellular parasites and endosymbionts. Otherwise, niche change in free living organisms may necessitate or benefit from acquisition of novel functionalities accompanied by expansions that could secondarily be balanced by loss of genes that became superfluous in the new environment.
Contractions involve very preferentially genes that have no or very little effect on fitness under the conditions that prevail during and after the gene(s) loss. Expansions are probably more random. As a consequence of such alterations, important variations in gene content started to be frequently observed within a single prokaryote species a decade ago, although such variations did not occur to the same extent in different species [27]. This formed the basis of the concept of a pan-genome, in which the genome can be subdivided into subsets of:
- • stable genes seen in all strains from a species, the core genome;
- • dispensable genes seen in several strains;
- • strain-specific genes found in a single strain.
However, genes may move from one category to another as seen with the accumulation of sequenced genomes from additional strains.
The concept of the pan-genome was extended to higher taxonomic units and notably to the entire kingdom of bacteria in an analysis of a set of 573 genomes that resulted in the definition of three groups of genes:
- • a nearly universal core group of about 250 highly conserved gene families present in a vast majority of species (99%) and encoding essential functions;
- • a group of about 8000 gene families occurring at variable frequencies and determining metabolic specificities;
- • a large group of fast-evolving genes present at very low frequency suggesting a high turn-over rate.
The two latter groups represent the reservoir for the emergence of new biological functions.
One of the major consequences of HGT in prokaryotes is connected to the occurrence of genomic islands that were first observed in pathogenic bacteria. These genomic segments contain virulence factors largo sensu and were hence called pathogenicity islands [28]. Similarly, other genomic islands, often conferring increased fitness in certain niches, can be found in environmental bacterial genomes. Genomic islands are acquired by HGT, display usually specific features such as nucleotide composition and codon frequency distinct from the rest of the genome, are associated with mobile elements and are flanked by tRNA genes. These islands can convey associations of highly important factors impacting adaptability, fitness and competitiveness between extremely distant prokaryote organisms. Their role in evolution can hardly be overstated.
7 Perspectives: do we need more genome sequences?
7.1 Prokaryotes
Several clues indicate that we may have enough genome sequences as we are probably close to limits such as upper and lower size limits, extreme GC/AT content, saturation of the set of highly conserved genes, diminishing chances of discovering a new protein fold or a new protein superfamily.
Conversely, complete genome sequences will also be continuously needed just to guide experimental work and to detect missing genes and traits. However, the need for sequencing each person's favourite organism has led to a major bias in the representation of fully sequenced genomes as well as drafts. Bacteria of medical relevance have benefited from intensive work. Because they provide clues to virulence, host specificity, drug resistance and enable pathogen survey at planetary scale, including emerging pathogens, multiple strains have been sequenced for many pathogens. This resulted in a major overrepresentation of a few phyla, namely in Proteobacteria, Actinobacteria, and Firmicutes, and even in such divisions the sequencing effort has focused on a very limited set of species or genera.
Conversely, environmental genomics is lagging behind and the vast evolutionary diversity still awaits more intensive exploration. Some initiatives are trying to correct this unsatisfactory trend [29]. In particular, DOE has launched a programme entitled Genomic Encyclopaedia of Bacteria and Archaea aiming to cover the current lists of prokaryote type strains.
7.2 Eukaryotes
A representation bias as seen in prokaryotes is also present in eukaryotes. Whereas the cost of bacterial genome sequencing has become very modest with the advent of NGS, the bias seen in eukaryote genomes reflects largely the funding opportunities and results in an overrepresentation of genomes of economic interest. There are thus entire phyla of the eukaryotic phylogenetic tree that remain totally unexplored. Some trials to adapt metagenomics sequencing, such as the Tara Ocean project [30], have shown encouraging results and could possibly be extended to other environments. Similarly, the eukaryotic viral world remains very poorly explored and observations as unexpected as the discovery of giant viruses [31] remain possible.
7.3 Other directions
Applied to both environmental and medical purposes, metagenomics has produced a wealth of results and offered a first insight into the realm of non-cultivated organisms in various niches [30,32,33]. We mentioned above the possibilities afforded by metagenomics in the area of eukaryotic biodiversity. At the opposite side of the horizon, the progress made in single cell genomics, especially with the recent availability of long sequence reads, will usefully complement the embryonic picture given by metagenomic analyses to date.
Genomics is also becoming a tool for new applications, such as the identification of pathogens and the search for both acquired and inherited mutations in human diseases. Furthermore, no one could foresee that genomics was also going to revolutionize anthropology and human archaeology, and we are just at the beginning of this fascinating renewal of the saga of mankind. These applications that appeared with NGS are just mentioned to illustrate how far reaching the advent of genomics and its outcome have been.
8 Beyond genomics
Genome sequences have become an essential and obligatory tool and not only for biologists. Genomics affords a molecular description of the main actors of life, the proteins, and provides insight into all aspects of biology: metabolism, physiology, cellular biology, pathology including infectious diseases, evolution, and so on.
Thanks to genome sequences, it became also possible to identify a new world of molecules, the non-coding RNAs (ncRNAS) some of which have a proven and clear function in cells. It is not the scope of this paper to describe and discuss these new biological actors. Initial results tend to show that their role may be very diverse. Sometimes it seems already well documented as for the microRNAs. But in general the roles of ncRNAs, if any, are less clear. To complicate matters, sequence conservation is highly variable [34] and some cases of knockout with no resulting phenotype have been reported. However, high hopes are placed in experiments of surgical removal of regions encoding ncRNAs mediated by the CRISPR/Cas9 system.
Genomes do not just encode the various operational molecules, they are also part of the structural elements exerting functions of the cellular system, as we know it since the conception of the lactose operon model. Also genomes take part in their own replication. Characterization of the genomic sequence of these elements along with the interacting molecules that contribute to these multiple roles is underway and represents the objective of genome biology. ncRNAs probably play multiple roles in genome biology. Initial large-scale endeavours have already produced massive amounts of data [18,19] and should contribute to progress in this new discipline.
In some ways, genomics is the culmination of molecular biology. It put us at the very heart of the information that specifies and controls biological processes and systems. While going further perhaps should we keep in mind the warning of Brenner [21]:
“What most people have forgotten in their easy dismissal of molecular biology is that it introduced the notion of information into biology and showed that it had a material basis in the form of nucleic acid sequences. It forces us to think of biological systems as molecular information processing systems rather than systems involved merely in the molecular processes of energy transactions and chemical transformations.”
Whereas in computers humans have separated hardware and software, life has always kept their tasks closely intricate and often performed by the same molecule. This will not facilitate disentangling the complexity of biological systems. But challenges are just what we need.
Disclosure of interest
The author declares that he has no competing interest.
Acknowledgements
I am indebted to Susan Cure, Claudine Médigue, and Patrick Wincker for proposing numerous and thoughtful improvements to this manuscript and to Véronique de Berardinis, Stéphane Cruveiller, and David Vallenet for their help in preparing the tables and the figure.Funding: FACAD.