1 Introduction
The disease of human immunodeficiency virus (HIV) infection has become a worldwide pandemic [1,2]. UNAIDS reports about an estimated number of 34–46 million people living with HIV worldwide by the end of 2003, and 5 million new infections in 2003 show that it is still growing rapidly. The disease is a major problem first of all in the developing countries of Sub-Saharan Africa. This region accounts for 25.0–28.2 million people infected with HIV by the end of 2003 and 4.2–5.8 million new infections in 2003 (source: UNAIDS http://www.unaids.org/).
Very effective treatments for HIV infected individuals have been developed, and drug prices dropped significantly the last years according to UNAIDS (http://www.unaids.org/). Therefore, they may be widely distributed, not only in the industrial, but also in the developing countries. Thus, it is worth studying the effects that the wide use of antiretroviral can have on the spread of the disease.
HIV predominantly infects CDT cells that are crucial for the functioning of the human immune system. In the course of the disease of HIV infection, an increasing amount of viruses in the blood of the patients leads to a decrease in the CDT cell count, and finally to the destruction of the immune system [3].
Several groups of anti-HIV drugs have been developed that interfere with several processes in the replication cycle of HIV. The most important ones among those are reverse transcriptase inhibitors and protease inhibitors, antiretroviral drugs that inhibit the process of reverse transcription and the viral maturation process, respectively. To interfere with the integration process, integrase is used as another target for the development of anti-HIV drugs. Multiple coreceptors for different types of HIV strains have been identified that probably play an important role in the process of binding to the host cell. These coreceptors are also promising targets for anti-HIV drugs. Starting with highly active antiretroviral therapy (HAART), an anti-HIV combination therapy that includes one protease inhibitor and two reverse transcriptase inhibitors, combination therapy has been used to reduce resistance effects. In many cases, combination therapy leads to a delayed progression of HIV disease. If initiating therapy is used and if dose reductions and inconsistent therapy are avoided, a long-term suppression of the virus below the limit of detection is possible. However, recent studies have shown that HIV persists in a replication-competent form in resting CDT cells, despite aggressive antiretroviral therapy. Therapy-induced decreases in viral load and an increasing number of CDT cells were the most significant prognostic indicators of positive clinical treatment benefit [4,5]. As a result, treated individuals can live longer free of HIV-related symptoms.
The influence that antiretroviral therapy has on the battle between the human immunodeficiency virus and the human immune system has widely been analysed, see e.g. [6–12]. The spread of HIV/AIDS amongst a population or among interacting communities without treatment has also been investigated [13–19]. Some mathematical and statistical models deal with the spread of HIV/AIDS amongst a population including treatment and/or change of behaviour [8,17,20–25]. For more details on the mathematical modelling of the dynamics of infectious diseases, see [15,26–30].
In [31], the authors formulated and analysed a model for HIV transmission for a homosexual population of varying size with recruitment into the susceptible class proportional to the active population size and with stages of progression to AIDS in [31]. In [32–34], the authors modified this ordinary differential-equation model by incorporating treatment effects. The underlying assumption in [32,33] is that of a worst-case scenario, where infected individuals – no matter if they are treated or not – do not change their behaviour despite their knowledge of the risks. In [32], a general staged progression model with n infectious stages, including anti-HIV drug treatment, was formulated and analysed in detail. A similar model with staged progression based on clinical stages was discussed in detail and simulations were shown in [23].
Although there are lots of research going on in the field of modelling the disease of HIV infection, many effects that antiretroviral treatment has on the spread of HIV infection in a population remain unknown. First of all, it has not been investigated, how the delayed onset of positive treatment effects influences the spread of the disease. Secondly, it is worth studying the possible effects of the latency period, although it is assumed to be very short in the case of HIV (in the range of a few days, see [5]).
In the following, we will study the dynamics of the spread of the disease of HIV infection in a population depending on this treatment-induced delay. We will present an ordinary differential-equation model based on clinical stages as given in [32,33] and the main results in Section 2. In Section 3, we will then modify it by introducing two time delays λ and τ to model the delayed onset of treatment effects (τ) and the latency period (λ). As there is no data on the size of τ, we will show simulations for different scenarios according to the size of this treatment-induced delay. A detailed discussion of the results is given in Section 4.
2 ODE model with two infectious stages: model formulation and main results
In the following transmission via sexual contact is assumed. Thus, we consider a sexually active population of varying size N. AIDS patients are assumed to be too sick to be sexually active. Thus, the total population can be divided into a susceptible class of size S and an infectious class before the onset of AIDS. As the infectious period is very long (⩾10 years), it is further divided into several stages. The widely-used CDC-classification suggests 2–6 stages of infection before AIDS with the classes defined by either viral load or CDT cell count, and clinical symptoms (see [5]). In our model, we use two stages according to clinical stages, that is the asymptomatic and the symptomatic phases as defined in [18]. The numbers of individuals in the infectious classes are given by and , respectively. All individuals entering the population are assumed to be susceptible, because vertical transmission can be almost excluded by means of antiretroviral treatment [5]. The number of individuals entering the population per unit of time is bN with birth-rate constant b, where ‘birth’ means that an individual becomes sexually active. Similarly, d is the natural death rate constant for all subpopulations. For simplicity reasons, the total population size is assumed to grow exponentially with rate constant in the absence of the disease. It is further assumed that infected individuals do not change their behaviour, no matter if they are treated or not. Thus, the average number of contacts of an individual per unit of time is a constant c for all subpopulations. The disease progression rate constant from stage j to stage or the AIDS-stage is given by for , respectively. To model treatment effects as described in Section 1, it is assumed that an infected individual in the second stage can move back to the first stage by means of successful drug treatment. The corresponding transfer-rate constant is denoted by α. By β and we denote the probability of disease transmission during one contact with an infected individual in stages 1 and 2, respectively.
See Table 1 for an overview of the parameters and variables used in the formulation of the model equations. Here also the parameter values used in the simulations below are given. The values for the disease progression rates are taken from [18]. The reasons for using these quite old estimates are obvious: as today antiretroviral treatments are widely used, new estimates on these transfer rates always include treatment effects. The main goal of our work was to study the effects of treatment on the population growth and the spread of the epidemic. This is why we have to use these very old estimates for the that hopefully represent the spread of the epidemic in an untreated population. In case , an epidemic of HIV infection can only speed up extinction of the population, whereas in case , the disease of HIV infection can slow down the population growth or even reverse growth to decay. As the latter case is much more interesting, the simulations shown in this paper use parameter values b and d chosen such that the population grows in the absence of the disease. According to [5], the probability of disease transmission per contact is between 11% and 50%. It is highest right after infection and in the last stage before AIDS, thus we take and . In the simulations we analyse two treatment levels – medium level and high level treatment with and , respectively. It has to be pointed out that there is no data available for the size of parameter α, thus our values are only reasonable estimates for different treatment levels. Parameter c reflects the level of risky behaviour in the population.
Parameters, variables and parameter values
S | number, proportion of susceptibles |
for j=1,2 | number, proportion of infectives in stage j |
, | initial numbers of infectives (in thousands) |
total active population size | |
initial population size (in thousands) | |
A | number of AIDS cases |
b=0.001 | ‘birth’ rate constant (number of people becoming sexually active per unit of time) |
d=0.0006 | natural death rate constant |
c=0.3 | average number of contacts of an individual per unit of time |
β=0.5 | probability of disease transmission per contact by an infective in the first stage |
a β=0.6 β | probability of disease transmission per contact by an infective in the second stage |
transfer rate constant and , respectively (disease progression) | |
α=0.1 or 0.2 | transfer rate constant (successful treatment: medium or high level treatment) |
with | average number of new infections per unit of time (standard incidence) |
Let denote the derivative of X with respect to time. The model equations are then given by:
(1) |
The treatment reproduction number R is used to define a threshold condition for the existence and stability of equilibrium points. It can be interpreted as the average number of infections a typical infective causes in a completely susceptible population. In [32], a formula for R was derived for a very general model with n infectious stages. The complexity of this formula increases significantly with increasing n. In the case of two infectious compartments, it yields the following expression for R:
(2) |
(3) |
(4) |
(5) |
To discuss the local stability of the endemic steady state , we consider the linearized system of (1) at . The Jacobian at is given by:
(6) |
(7) |
(8) |
(9) |
(10) |
(11) |
(12) |
By using the Routh–Hurwitz criterion [35], it follows that all eigenvalues of the characteristic equation (9) have negative real parts if and only if the following condition is satisfied:
(13) |
The endemic population steady stateexists if and only ifand is asymptotically stable if the inequalities in (13) are satisfied.Theorem 1
For the parameter values as given in Table 1 and as defined in (6)–(8), we get:
(14) |
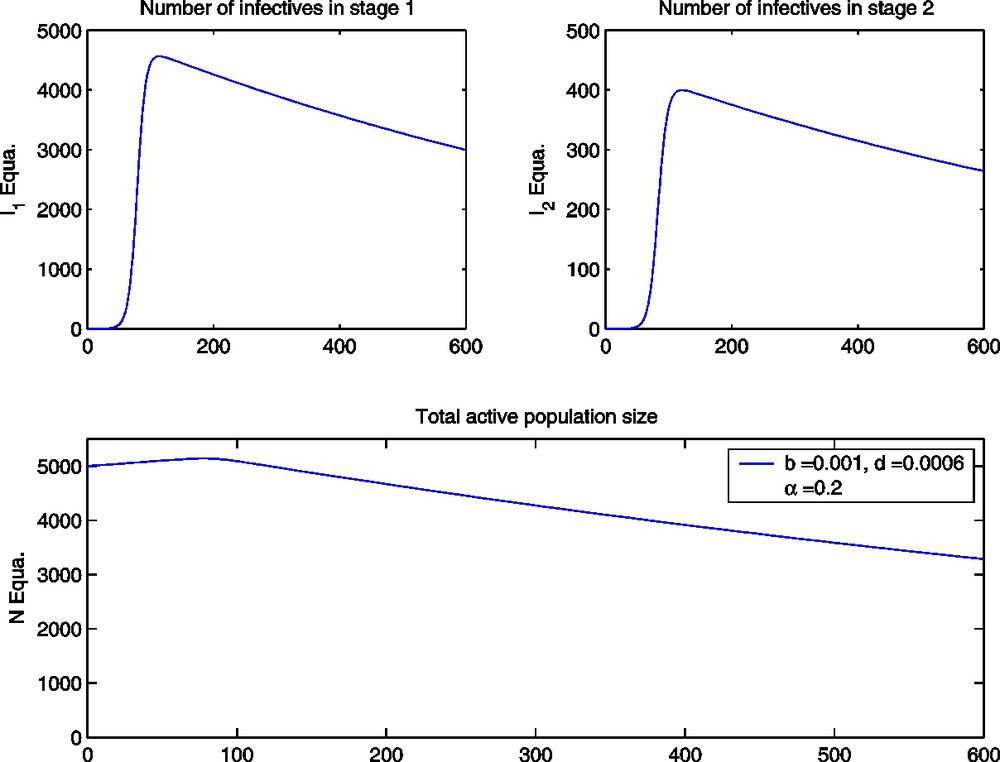
The ODE model with high level treatment (α=0.2) and all parameter values as given in Table 1.
In [32], it was shown in detail that is asymptotically stable for n infectious stages whenever it exists.
3 Models with time delay: latency period and treatment induced delay
Let constant τ (in months) be the time delay from the start of treatment in the symptomatic stage until treatment effects become visible. As with every virus infection, one has to distinguish between infected individuals having the virus in their bloodstream and infectious individuals, who also can infect others. When a patient is infected with HIV, he typically can transmit the disease not at once, but after a short timespan (in the range of a few days, see [5]) called the latency period. In the following the latency period will be modelled by a time-delay constant λ (in months).
We incorporate these two delays into our ordinary differential-equation model (1) and use the same parameters as in Table 1. We then get the following delay differential-equation model:
(15) |
(16) |
The characteristic equation of (16) is given by:
(17) |
The steady state of system (15) is called absolutely stable (i.e. asymptotically stable independently of delay) if it is asymptotically stable for all delays λ and τ. is called conditionally stable (i.e. asymptotically stable depending on the delays) if it is asymptotically stable for λ and τ in some intervals, but not necessarily for all delays λ and τ.Definition 1
The characteristic equation (17) of the linearised system at the endemic steady state can be written as a third-degree polynomial equation of the following form:
Here we have (again with , , as given in (6)–(8)):
We have , and , where are defined in Eqs. (10)–(12).Remark 1
The location of the roots of Eq. (17) has been studied by many authors (see [38–46]). The following result, giving necessary and sufficient conditions for the absolute stability of (16), was proved by Hale et al. in [37,47].
The delay differential-equation system(16)is absolutely stable if and only if: Lemma 1
To study the delay case, we consider the ordinary differential-equation model (1) first (with for model (15)), and assume that condition (13) for the asymptotic stability of is satisfied. Thus, all the roots of (1) have negative real parts, and assumption (1) in Lemma 1 is fulfilled. Assumption (2) in Lemma 1 means that iω, is not a root of Eq. (17). Then by using Lemma 1, the delay system is asymptotically stable if and only if the ODE system is asymptotically stable and the characteristic equation (17) has no purely imaginary roots.
Let , with , be the eigenvalues of the characteristic equation (17), where and depend on the delays τ and λ.
If, on the other hand, the characteristic equation (17) has a pair of purely imaginary roots iω, , then the delay system (15) is not absolutely stable, but could be conditionally stable. For example in the case of one delay, say for τ, and we suppose that is given, for some value . Hence, when , the real parts of all roots of the characteristic equation (17) still remain negative and the system is conditionally stable. When , the characteristic equation (17) has a purely imaginary root iω, , and the system (15) loses its stability. By using Rouché's theorem (Dieudonné [48], Theorem 9.17.4) and continuity in τ, and if the transversality condition holds () at , then the characteristic equation (17) will have at least one root with positive real part when and becomes unstable.
To be able to find a condition for all eigenvalues to have negative real parts, we will determine if (17) has purely imaginary roots.
Since the positive equilibrium of the ODE model is stable, we have when . By continuity, if , are sufficiently small, we still have and still remains stable. If for certain values and , the steady state loses its stability and becomes unstable when . On the other hand, if such an does not exist for all delays, that is the characteristic equation (17) does not have purely imaginary roots, the system will always be stable.
If , is a root of the characteristic equation (17), then we have:
(18) |
(19) |
The resulting differential-equation model including delayed onset of treatment effects but no latency period is formulated as follows:
(20) |
(21) |
(22) |
(23) |
If and , then Eq. (23) has no positive real roots.Remark 2
Notice that in the case of a population with high-level treatment () and all parameter values as given in Table 1, we have: and . Thus, the equation has a positive root . Hence the characteristic equation (17) with has a purely imaginary root .
Again, let be the eigenvalues of Eq. (17) – with – such that . From (21) and (22), we have
(24) |
(25) |
Then the real part of becomes positive when , and the steady state becomes unstable (see Fig. 4). From that, it follows that is a bifurcation value, and a Hopf bifurcation (see [49]) occurs when τ passes through the critical value in Fig. 3. We can see in Fig. 2 that the steady state still is stable for delay , allthough the delay causes transient oscillations. Thus, we have the following theorem.
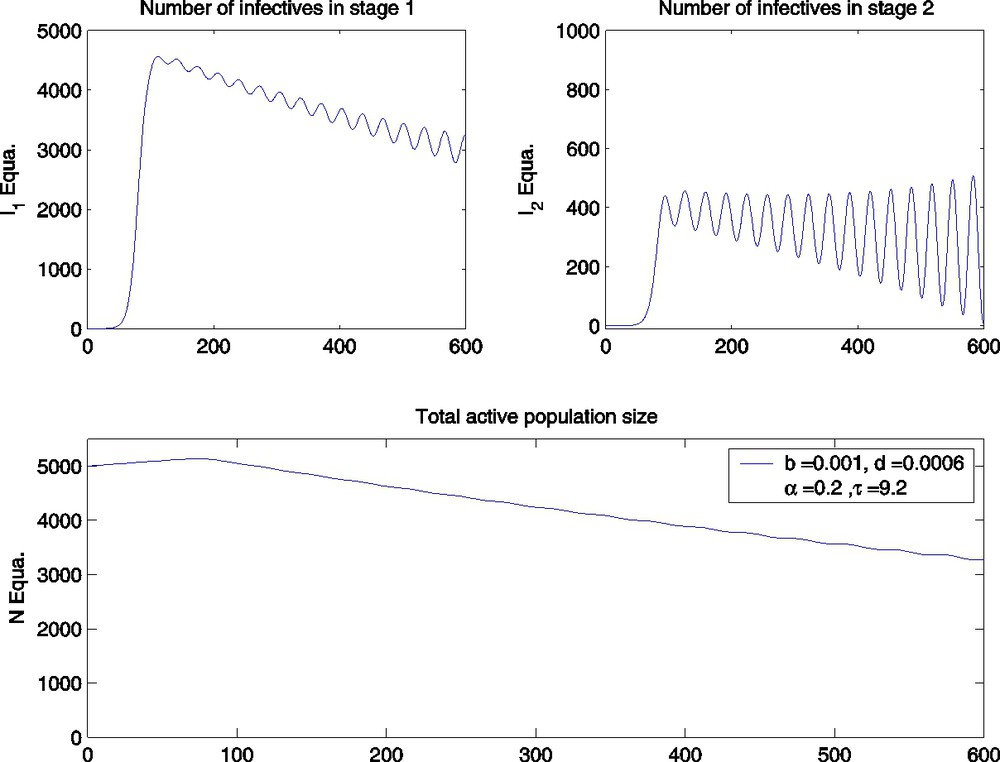
The DDE model with α=0.2,τ=9.2 and parameter values as given in Table 1.
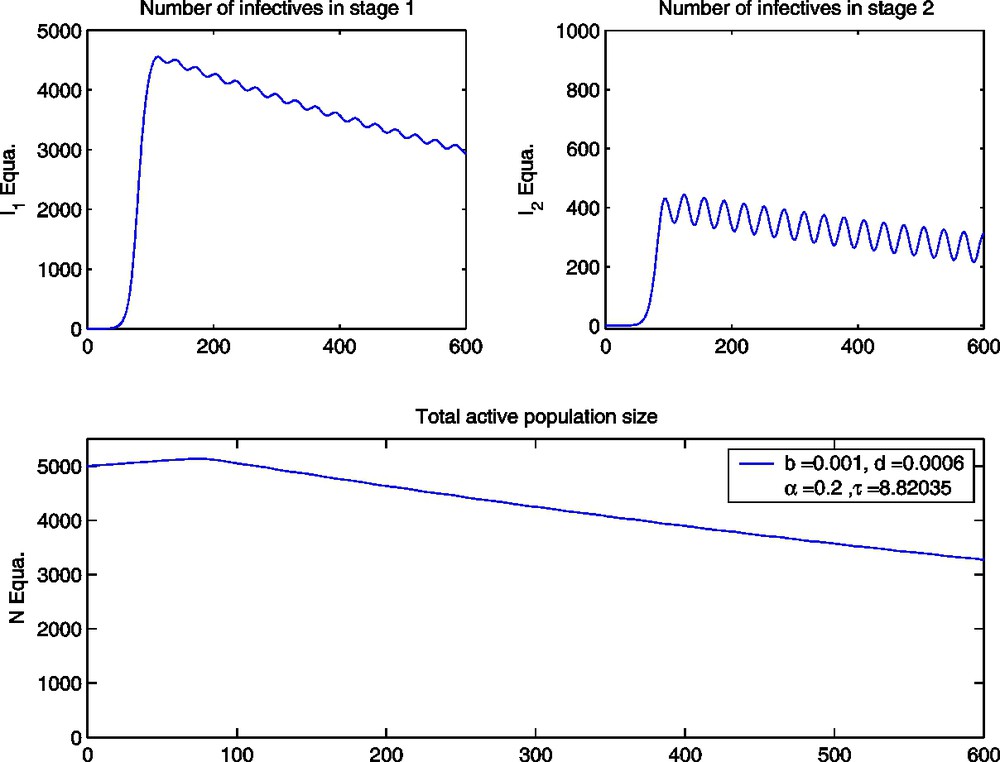
The DDE model with α=0.2,τ≅8.82035 and parameter values as given in Table 1.
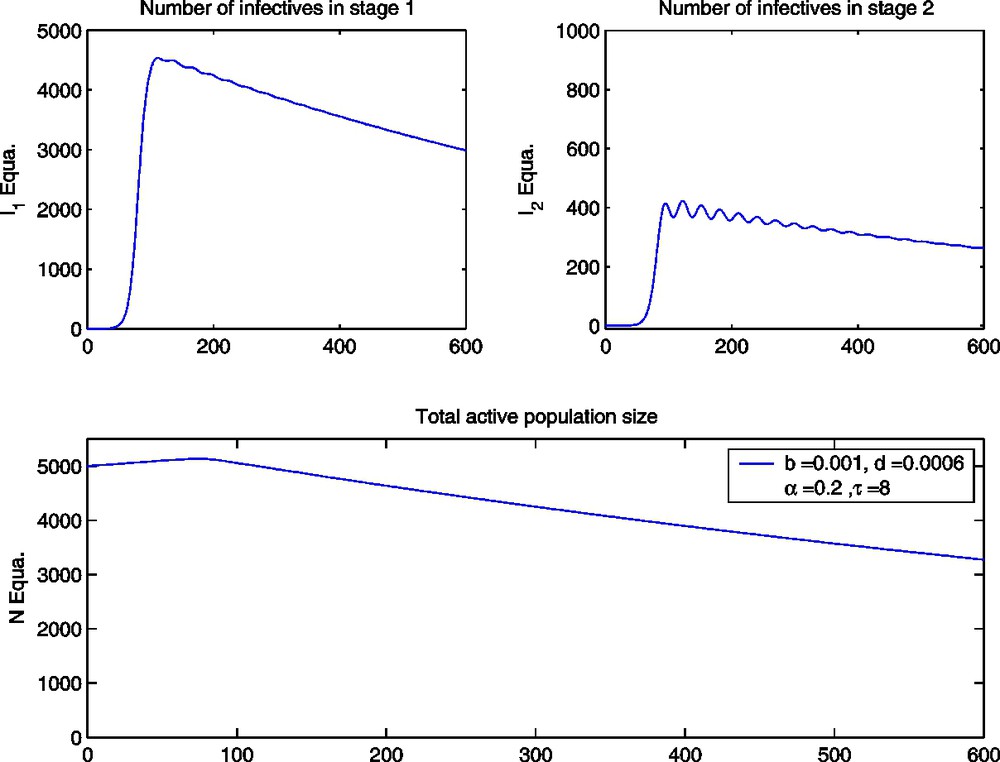
The ODE model with α=0.2,τ=8 and parameter values as given in Table 1.
The infected steady state
of the delay model
(20)
is asymptotically stable when
and unstable when
, where
Theorem 2
and
are given by
(24)
and
(25)
.
If , a Hopf bifurcation occurs, that is, a family of periodic solution bifurcates from as τ passes through the critical value .
Biologically, this means that there is a critical value for the treatment-induced delay , that determines the stability of the endemic equilibrium point . With the parameter values given in Table 1, the endemic equilibrium point is asymptotically stable when antiretroviral drugs in average show positive effects in the patients within less than months. In this case (see Fig. 2) the population will reach the infected steady state as given in Eqs. (3)–(5). As soon as it takes more than months for the patients to feel better, the infected steady state loses its stability (see Fig. 4).
4 Discussion
The underlying assumption in the present paper is that people who are infected with HIV do not change their behaviour despite the knowledge of the risks. In [50], S. Blower states that incidence rates of HIV will fall as more HIV-positive individuals gain access to treatment (HAART), but that this public health benefit will only occur if the levels of risky behaviour do not increase. Computer simulations based on a simple ordinary differential-equation model for an HIV epidemic (see [33]), on the contrary, show that treatment without reduction of risky behaviour may even increase the proportion of infected individuals. This effects can also be seen in the delay differential-equation models presented in the present paper. The simulations for the ODE model in [33] show that the combination of reduction of risky behaviour together with antiretroviral drug treatment is a very promising strategy in fighting the epidemic of HIV infection. This has to be taken into account, when anti-HIV regimens are distributed in the poorest countries of the world, where behaviour change is hard to achieve because of low educational levels or cultural reasons.
In our delay differential-equation models we do have two threshold parameters: The treatment reproduction number R serves as a threshold for the existence of an endemic equilibrium point, and the size of the treatment-induced delay τ has enormous effects on the dynamics of the spread of the disease of HIV infection in the population. This treatment-induced delay τ can be seen as the minimum time span that patients have to be under medical treatment until positive treatment effects occur. If and only if the treatment reproduction number R is above one, there is a positive steady state. If in this case, the treatment induced delay τ is below a critical value, the total sexually active population will reach the infected equilibrium point (see Fig. 2). Note that at this positive steady state there are still people that have not been infected with HIV. The proportion of these susceptibles is given by . As soon as τ increases and reaches the critical value, a Hopf bifurcation occurs (see Fig. 3), and, finally, the endemic equilibrium point loses its stability above the critical value (see Fig. 4).
The present work wants to be understood as a starting point for further research on analysing treatment effects including time delay on the spread of an HIV epidemic. The next step will be to analyse the general model with n infected stages. We also have to point out that we assumed exponential growth for the total population in the absence of the disease, what is a very restrictive assumption. The simulations with logistic growth for the ODE model given in [32] show that the disease of HIV infection also could lower the carrying capacity or give rise to a second positive steady state below the carrying capacity. It remains to analyse the delay differential-equation model with other than exponential population growth and the role of treatment and behaviour change under these circumstances.
Acknowledgments
The first author was supported by the Fonds zur Förderung der wissenschaftliche Forschung under SFB F003, ‘Optimierung und Kontrolle’, and by EC programme Centers of Excellence of State in phase of pre-accession, No. ICA1-CT-2000-70024.