Abridged version
1 Introduction
In a borehole environment, each depth can be characterised by a multidimensional vector corresponding to all measured logs. All these vectors are then treated in order to infer the lithology [19]. The difficulty is to determine the number, the thickness, and the facies of the various geological units. All the vectors corresponding to a particular electro-facies are very similar to each other, as regards the direction and the modulus. This is why the statistical methods of automatic data classification allow fulfilling this objective [9,16]. Neural networks are therefore well adapted to this particular processing [2,5,8,14,21].
2 Neural network method
A self-organising map [10,11] is a collection of n reference vectors organised in a neighbourhood network, which bear the same dimension as the data vectors. Usually, the neighbourhood function is given in terms of a two-dimensional neighbourhood matrix . On the map, each node has the same neighbourhood radius that decreases linearly to zero during the self-organising process. The conventional Euclidian distance is used to determine the best-matching unit (so-called ‘winner’) on a map for the input vector . The vector of the winner node and its neighbourhood were updated towards the input vector by a learning factor following Eqs. (1) and (2) [6,12]. The units outside the neighbourhood area were left intact. The learning process starts with a large neighbourhood radius and a large learning factor, which will diminish during the training step.
After the self-organising process is complete, the map approximates the density distribution probability function of the dataset. The location of a reference vector on the map corresponds to the location of the vector in the n-dimensional dataset.
3 Geological background
The Rhodanian Gard is located between the Massif Central border to the west and the Alpine domain to the east, beyond the Rhone valley (Fig. 1). It is part of an extended sedimentary basin that was located on the Tethys margin. On the Massif Central border, the end of the Urgonian marine sedimentation is associated with a deformation of the carbonate platform. The Gargasian calcareous marl series, which out crops further east, is absent below the MAR203 well. The platform does not sink before the Upper Albian: it gets immerse and is stacked with a silty shale series (the CSM), the largest thickness being located below Marcoule. Despite the important subsidence of the Rhone Valley, the basin remains shallow, as shown by the silty nature of the CSM and by its very bioturbed structure. This series gets thinner towards the north and the east [13].
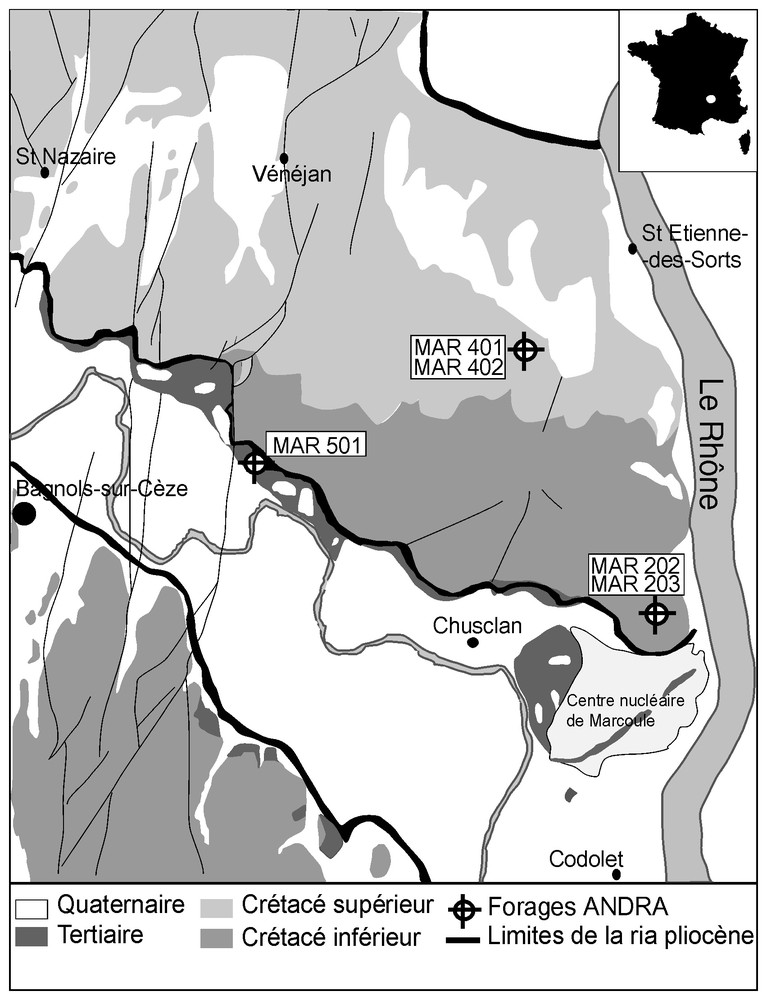
Carte géologique simplifiée et localisation des forages implantés sur le site du Gard rhodanien pour étudier la couche silteuse de Marcoule (CSM).
Schematic geological map and wells sites located on the Rhodanian Gard in order to study the CSM.
During the Upper Cenomanian, a clear regression follows up at the top of the CSM, with alternation of shaly and silty beds, then orbitolinid sandstone beds and finally, white sandstone with coal rakes (Fig. 2a).
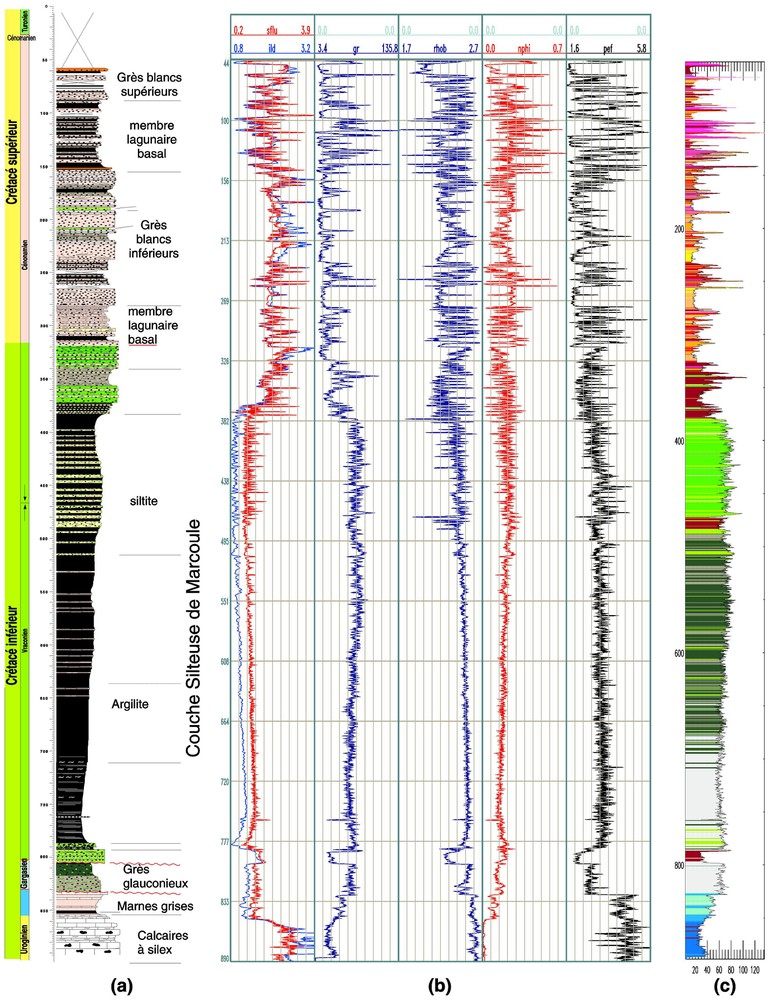
a. Log de référence résultant de l'observation des carottes. b. Traces des diagraphies utilisées pour l'entraı̂nement du réseau de neurones. c. Log reconstitué à l'aide du réseau de neurones auto-organisé mis en œuvre. Le pourcentage de reconstruction est de l'ordre de 90%. La CSM est reconstituée quasiment en continu.
a. Reference log resulting from core observations. b. Plots of the logs used to train de neural network. c. Reconstitution of the log with the neural network. The CSM is almost totally reconstituted. The data process clearly separates three major sub-units and describes a continuous mineralogical evolution from the bottom to the top of the series.
Thanks to his work, Ferry [4] updated the existing knowledge and proposed both a sedimentary and a palaeogeographical synthesis regarding the evolution of the basin during the Cretaceous. The author demonstrates that these sequences were deposited over four major transgression/ regression cycles, separated by tectonically induced regressions.
4 Results and discussion
Prior to data processing with neural networks, an orthogonalisation by PCA is implemented within the data space, in order to normalise the log data respect to each other (Fig. 2b). After having initialised a 15×15 node map, the network progressively gets self-organised. When the learning process is finished, each neuron automatically recognises a specific combination of log responses. Up to this point, each vector of the dataset could be sequentially processed to receive the arbitrary colour of the neurons they have stimulated: this is the classification stage [1]. Nevertheless, if an external geological knowledge is available, we are able to identify the specialised neural groups by giving them a colour (or an alphanumeric code) for a further geological interpretation (Fig. 3) [5,7]. As a result, the partially indexed self-organising map can be considered as a tool for automatic electro-facies recognition.
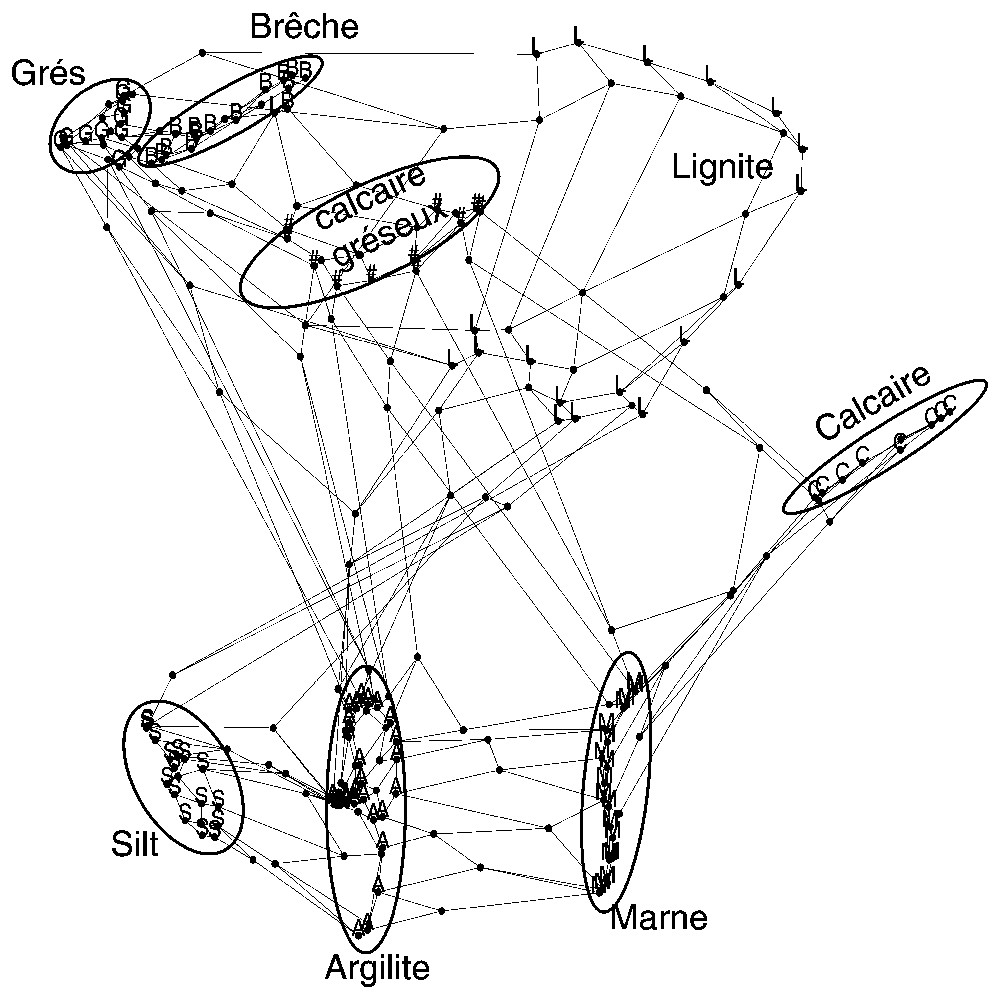
Carte 15×15 après auto-organisation et indexation visualisée grâce à une projection de Sammon [18]. Les nœ uds du réseau qui ont été excités par les individus du fichier d'indexation (Tableau 1) portent leurs codes alphanumériques.
15×15 map after self-organising and indexation processes on a Sammon's plot [18]. The network nodes identified by alphanumeric codes have been excited by each sample of the indexation file (Table 1). After this map indexation, we can check the continuous transition between silts, clays and then marls to reach these massive limestones has been confirmed.
Taking into account the neighbourhood relationships between the network nodes and the derived quantitative interpolation, the obtained recognition rate of about 90% is quite satisfactory (Fig. 2c). The CSM is clearly identified by the network and seems to be composed of two sub-units: the first silty one is located from −377 to −484 m; the second one, made of silty claystone, is located from −484 to −782 m (the deeper, the more marly the unit becomes).
The statistical data processing performed reconfirms the existence of a mineralogical gradient within the CSM This gradient was previously discovered through other approaches based on geochemical or mineralogical works [17,20]. Below the fully silty level, the silty claystone progressively turns to a more carbonate and marly component. Statistical data processing implemented using neural networks confirms this mineralogical evolution. It is also shown by the presence of a larger interstitial fraction of the carbonate phase. The CSM appears to be a real continuous transition between the clayey facies and the marly component.
5 Conclusion
The self-organising map (SOM) based on the neural network technology appears to be a reliable automatic method for the statistical classification of data: this approach is well adapted to the lithological interpretation of well log data. During the first processing phase (and based on the systematic exploration of the dataset), the topological map helps determine the number of facies as well as the continuous (or progressive) transitions between each one of the identified layers. During the indexation phase, the topological map is fed in with the injection of a geological knowledge (even limited or partial) resulting from core slabs observation, and allows to identify the lithological nature of the various studied layers and thus, to automatically generate a almost continuous log for the stratigraphic sequence.
1 Introduction
Dans un forage, chaque niveau en profondeur peut être caractérisé par le vecteur multidimensionnel des diagraphies enregistrées à son regard. La détermination de la lithologie est un des buts de leur traitement. Le problème consiste, dans ce cas, à déterminer le nombre, l'épaisseur et la nature faciologique des différentes unités géologiques. Parce que tous les vecteurs relatifs à un même électro-faciès [19] se ressemblent en direction et en module, les méthodes statistiques de classification automatique des données et d'analyse discriminante permettent d'atteindre l'objectif ainsi défini [9,16]. Les réseaux de neurones sont des outils bien adaptés à ce type de traitement [2,5,8,14,21].
2 Méthodologie statistique
Nous avons choisi de mettre en œuvre un réseau non supervisé, la carte auto-organisatrice proposée par Kohonen [10,11]. Le réseau puise dans le jeu de données les informations dont il a besoin pour son auto-structuration. Ses nœuds sont agencés en grille 1D, 2D ou 3D. À chacun d'eux est associé un vecteur (dit poids), de même dimension que les vecteurs des mesures. Lors de la phase d'initialisation, ces poids sont affectés aléatoirement. Un vecteur est tiré au hasard dans le jeu de données. Sur le critère de distance euclidienne minimum, l'algorithme détermine le vecteur poids qui lui est le plus ressemblant . C'est la phase de compétition. Le vecteur associé au neurone gagnant est adapté de façon à augmenter cette ressemblance. Cette adaptation, par rotation pondérée en fonction de l'éloignement au gagnant, est appliquée également aux vecteurs de tous les neurones situés dans le voisinage du gagnant. C'est la phase de coopération. La modification des poids obéit aux équations suivantes [6,12] :
La carte auto-organisatrice est une généralisation de la classification automatique des données par l'algorithme des nuées dynamiques [1]. Elle ne présuppose aucune connaissance a priori quant au nombre de groupes.
À ce stade, il suffirait d'affecter à chaque groupe de neurones un code arbitraire, pour générer une image de la séquence stratigraphique. Elle serait réduite à la détermination du nombre de faciès et à celle de l'épaisseur de chaque couche. En revanche, si une connaissance géologique externe est disponible, alors il est possible de codifier la carte en affectant à chaque neurone une couleur ou un symbole, géologiquement représentatif [5,7]. Quelques vecteurs de mesures correspondant à des niveaux géologiquement reconnus à partir de l'observation de carottes ou de cuttings, voire de déblais de forage, sont labellisés et présentés en entrée de la carte. Toujours sur le même critère de distance minimum, les vecteurs de la carte prennent le label du vecteur de données le plus ressemblant. Certains d'entre eux peuvent ne pas être indexés ; néanmoins, compte tenu de la pertinence intrinsèque des relations de proximité entre les neurones, il est légitime de codifier les nœuds du réseau non dûment indexés et de définir ainsi des faciès intermédiaires entre chacun des pôles purs reconnus. De ce point de vue, la carte auto-organisatrice généralise les méthodes de classification floue [3].
Lorsque la carte est ainsi auto-structurée et indexée, il suffit de présenter séquentiellement le jeu de données au réseau. Le niveau de mesure prend le code du neurone que son vecteur excite.
3 Le contexte géologique
Afin d'illustrer cette méthode statistique de traitement des diagraphies, nous l'avons appliquée aux mesures enregistrées dans le forage MAR203 réalisé par l'Andra dans le Gard rhodanien, en contexte silico-clastique du compartiment occidental du bassin sud-est de la France (Fig. 1) [15]. Outre les diagraphies enregistrées, ce forage MAR203 a fait l'objet d'un carottage en continu, ce qui permet de définir a priori un fichier d'indexation et de bénéficier a posteriori d'un contrôle précis de la lithologie.
Le forage a été arrêté à la cote −892 m dans la formation sommitale de la plate-forme carbonatée urgonnienne. Les séries sédimentaires crétacées sont caractérisées par la présence d'une épaisse couche de siltite, la CSM, jusqu'alors inconnue dans cette partie du bassin. Dans le forage, elle atteint 400 m d'épaisseur. Elle repose sur la dalle récifale urgonnienne par un niveau de marnes calcaires, marqueur de l'approfondissement de la marge thétysienne vers la fosse voconcienne [13] et de son ennoyage progressif. Ces marnes sont surmontées d'un banc de grès glauconieux à gravier, sur lesquels repose la CSM. En phase de régression, le cycle vraconnien–cénomanien, au cours duquel elle s'est déposée, se termine par l'alternance de grès blancs à orbitoline (faciès de plage), de faciès à lignite et de calcaires lacustres (Fig. 2a).
Les connaissances nouvelles acquises au cours de ces travaux conduisent Ferry [4] à proposer des synthèses sédimentaires et paléogéographiques réactualisées de l'évolution du bassin durant le Crétacé. Cet auteur montre que ces séries se sont déposées au cours de quatre cycles majeurs transgressions/régressions marines, séparés par des régressions forcées d'origine tectonique.
4 Résultats
Nous avons mis en œuvre le traitement neuronal sur les diagraphies suivantes, préférentiellement représentatives de la lithologie : la radioactivité gamma naturelle GR et les concentrations chimiques (K, U, Th) qui sont déduites de sa mesure spectrale, l'effet photo-électrique (PEF), la masse volumique (RHOB), diagraphies auxquelles nous avons adjoint la porosité neutron (NPHI), ainsi que deux résistivités électriques (ILD, SFLU), caractéristiques de la présence ou de l'absence de fluides, aqueux en l'occurrence (Fig. 2b). Préalablement aux traitements, nous avons normalisé et orthogonalisé les données par une analyse en composantes principales.
Compte tenu de la diversité des faciès entre les pôles marin, lacustre et terrigène, ainsi que de leur texture, des marnes aux grès grossiers, nous avons opté pour une carte 2D, traduisant ces deux paramètres faciologiques et texturaux. Sur l'exemple traité, les résultats sont peu dépendants de la dimension de la carte. Nous présentons ici ceux obtenus avec une grille carrée de 15×15 neurones, soit 225 nœuds pour classer et codifier 5587 vecteurs de mesure.
Les caractéristiques du fichier d'indexation que nous avons réalisé à partir de la description des carottes sont rassemblées dans le Tableau 1.
Fichier d'indexation de la carte de Kohonen. Association entre faciès, codes couleur et symboles alphanumériques.
Indexation file of the Kohonen map.
Faciès | Cote inférieure | Cote supérieure | Nombre de niveaux | Code couleur | Code alphabétique |
Lignite | 44,81 | 47,55 | 18 | noir | L |
Brèche | 195,83 | 199,95 | 27 | orange | B |
Calcaire gréseux | 207,57 | 209,70 | 14 | brun | # |
Grès | 225,55 | 229,36 | 25 | jaune | G |
Silt | 404,20 | 408,60 | 29 | vert | S |
Argilite | 600,00 | 606,70 | 44 | gris | A |
Marne | 842,00 | 846,00 | 26 | cyan | M |
Calcaire | 855,00 | 858,00 | 19 | bleu | C |
Après la phase d'auto-organisation, la structure du réseau peut être visualisée grâce à une projection bidimensionnelle non linéaire des vecteurs poids, suivant l'algorithme proposé par Sammon [18] (Fig. 3).
5 Discussion
La carte permet d'identifier le nombre de faciès composant la colonne sédimentaire. En effet, les nœuds du réseau se structurent en sept groupes bien individualisés. Une partie de la carte est toutefois moins fortement structurée. Son indexation permet d'identifier les différents faciès reconnu par l'auto-organisation. Chaque groupe prend un label unique. On peut vérifier alors la cohérence sédimentologique des relations de proximité interclasse. Dans la Fig. 3, les faciès marins occupent la partie inférieure de la carte. La transition entre les groupes est progressive entre le pôle calcaire et le pôle silt, en passant successivement par les marnes et les argilites. Les faciès à forte composante terrigène occupent la partie supérieure de la carte, diagonalement opposée au pôle calcaire. Au milieu de la grille, un faciès bréchique fait transition entre ces deux pôles extrêmes. Le lignite serpente entre un bord et le centre de la carte. Compte tenu de la faible épaisseur des bancs charbonneux, cette disposition éparse résulte du manque de résolution en épaisseur des mesures diagraphiques.
La carte étant ainsi structurée et codifiée, il suffit de présenter séquentiellement le jeu de données au réseau. Toujours sur la base du même critère géométrique, chaque niveau en profondeur prend le code du vecteur poids ressemblant le plus à son vecteur de mesures. Le résultat de cette détermination lithologique est représenté sur la Fig. 2c. Le taux de reconnaissance des différentes unités est satisfaisant, puisqu'il est de l'ordre de 90 % pour la totalité du forage. La CSM, quant à elle, est reconnue en continu à 95 %. Du fait de la pertinence des relations de proximité entre les nœuds du réseau, le traitement statistique mis en œuvre permet de confirmer l'existence en son sein d'un gradient minéralogique, également mis en évidence par d'autres approches, quelles soient géochimiques [20] ou minéralogiques [17]. En remontant dans la série, les marnes évoluent progressivement vers une composante, d'abord argileuse, puis de plus en plus silteuse (Fig. 2c). Ce gradient faciologique est le résultat d'une des phases transgressives majeures dans l'histoire crétacée du bassin [4].
6 Conclusion
Le réseau de neurones « carte auto-organisatrice » [10,11] se révèle être une méthode statistique de classification automatique des données, bien adaptée à l'interprétation lithologique des diagraphies. Au cours de la première phase du traitement, à partir de l'exploration systématique du jeu de données, la carte permet de déterminer le nombre de faciès ainsi que les transitions discontinues, ou au contraire progressives, entre chacun des bancs identifiés. Dans une seconde phase, l'indexation de la carte par l'apport d'une connaissance géologique, même très fragmentaire, résultant de l'observation de quelques portions de carottes, permet de déterminer la nature lithologique des différents bancs et donc de générer automatiquement un log (presque) continu de la séquence stratigraphique.
Moyennant quelques précautions et vérifications élémentaires (même contexte géologique, acquisitions des diagraphies à l'aide des mêmes sondes, au cours d'une même campagne de reconnaissance du sous-sol), l'outil statistique ainsi configuré et indexé peut être utilisé pour l'interprétation des mesures réalisées en forage destructif, sans autre contrôle a posteriori que l'observation des boues et de quelques cuttings.