1 Introduction
Climate change is affecting, and will continue to effect, biological and human systems in numerous and complex ways [43]. Scientific knowledge about climate change, and indeed the climate in general, creates the opportunity for decision-makers to make choices that will improve social welfare now and in the future [67]. First, governments can decide to mitigate the effects of climate change by placing restrictions on the emissions of greenhouse gasses. Second, government and private actors can decide to adapt their everyday lives, including the types of investment and consumption that support those daily lives, to anticipated or ongoing changes in climate. To support both types of decision-making, there exists a growing wealth of scientific literature, based on decades of empirical study, describing the workings of and interactions between the climate, biological, and human systems.
The goal of climate change assessment, as with all scientific assessment, is to provide this information and knowledge in a form that is accessible and useful to decision-makers [9,20]. This requires, as a first prerequisite, identifying the target audience of the assessment, and conducting the assessment in such a way that it fits with their cognitive capabilities, choice opportunities, and level of experience with the scientific information [21,49]. For international climate change assessments, such as the Intergovernmental Panel on Climate Change (IPCC), the target audience is very broad, including policy-makers at all levels of government, leaders of non-governmental organizations (NGOs), and decision-makers in the private sector [50]. Given this breadth, the IPCC and similar assessments can be most useful by presenting a picture of where scientific consensus exists, or perhaps more importantly the reasons why it fails to exist, across the full range of climate related knowledge, what has been called “Climate Affairs” [25].
A feature that climate change shares with most environmental problems is that the events to be assessed are highly uncertain [57] and the outcomes are poorly defined. First, given that the climate system, and the biological and human systems with which it interacts, are complex and in some cases complex adaptive systems, future outcomes are highly sensitive to small changes in current conditions, meaning that with any errors in measuring important data (and there are always errors in measuring important data), it is impossible precisely to predict future system states; any estimates will have to be probabilistic, at best [39]. Such probabilities can, in some cases, be arrived at through Monte Carlo analysis or similar techniques, running predictive models multiple times, varying the data within the range of likely measurement error [2,17,79,81]. Second, given the incomplete understanding of how all the relevant systems behave, there is always a certain degree of uncertainty as to whether the models used capture the essential structures of the system [44]. Scientists often have degrees of confidence in different models; while expert elicitation techniques [45,62] can often represent the confidence estimates from numerous scientists as probability distributions, it is essential to understand that they are highly subjective, based on the informed guesswork of the scientists [13,78].
For the purposes of climate change, it is useful to characterize three fundamentally different types of uncertainty; for each type of uncertainty, there may be one or more analytic techniques from which the analyst may choose [17]. Epistemic uncertainty originates from incomplete knowledge of processes that influence events, e.g., unknown values for climate sensitivity. This type of uncertainty can be quantified using Monte Carlo analysis or expert elicitation, as well as the examination of different model structures. Natural stochastic uncertainty relates to the chaotic nature of the climate system, which can be quantified (with limits) using multiple runs of the model with slightly different initial conditions. Human reflexive uncertainty exists in the case of climate change because society is part of the problem (through emission of greenhouse gases) and also part of the solution (through adaptation and mitigation). Various other nomenclatures have been provided to explain this uncertainty such as the self-referential system or observer-participant [16], the concept of agency [61], feedbacks [63], or reflexivity [17]. This type of uncertainty cannot be quantified in any meaningful sense so scenario analysis has to be applied. An important challenge for climate change assessment, therefore, is to represent these different types of uncertainty accurately and consistently [41].
Making this challenge difficult, however, is the heterogeneous ability of different actors to understand and interpret probabilistic information, and the observation that many of these people will either choose to ignore information that is too complicated for them, or will respond in ways that disproportionately makes use of some types of information over others, in a manner that the scientific knowledge itself does not justify. In this paper, we examine how international assessments of climate change, such as the IPCC, can best address this issue. First, we examine some of the known difficulties that people face in responding to probabilistic information, and common ways that have been used to overcome these challenges. Second, we examine the approach that the most recent IPCC assessment, the Third Assessment Report (TAR) used. We present results from two empirical studies, one conducted with lay-people, the other with climate change efforts, which can help us to evaluate the success of the IPCC strategy. Finally, we offer suggestions for future assessment.
2 Interpreting and communicating probabilities
The neo-classical economic theory of individual decision-making, which is the standard against which other theories of decision-making are judged, has taken as axiomatic that people work to maximize expected utility. Given reasonable assumptions about decreasing marginal utility (the wealthier one is, the less extra satisfaction and additional unit of money will buy), it is easy to show that people will be averse to taking risks, and prefer certain outcomes to uncertain ones of equal expected value. Central to this decision-theory, however, is that people compute utilities (or act as if they computed) associated with multiple possible states of the world, integrate those utilities along the probability distribution function, and choose the option with the highest value [64].
However, it is well-established and well-known that people make decisions under conditions of uncertainty that violate many normative axioms of choice, and are at times inconsistent and counterproductive [1,68]. For example, in decision-making experiments with well-described probabilities, changes of equal magnitude in assessed probabilities have very different effects: the change from 0.99 to 1.0, for example, often affects people's choices much more than a change from 0.50 to 0.60; likewise, many of the most serious risks are also underestimated [35]. People treat as more likely and more consequential those events that are more visible, such as overestimating the risk of death from an airplane crash or a terrorist attack compared to an automobile accident [14]. Policy-makers and others with technical training often perform little better than lay-decision-makers, at least when operating out of their precise area of specialization [26]. In this section, we review some of the findings that are most relevant to the issue of communicating climate change uncertainty.
2.1 Heuristics for decision-making under uncertainty
Researchers have described the task of choice as being comprised of a framing stage and an evaluation stage [72]. The framing phase is used to relate the decision-problem at hand to other similar problems, as a way of determining which of many decision-rules, or heuristics, ought to be used. In the evaluation phase, the individual draws on a wealth of these pre-existing decision-heuristics in order to come up with an answer or choice. For example, the framing stage is often used to determine whether the outcomes of the choice represent losses or gains relative to some base-line, or status quo; in the evaluation stage, individuals then often act first to reduce the probability of losses (often opting for a smaller probability of larger losses, and thus greater risk), and second to ensure a high probability of gains (a risk averse stance) [35]. The framing stage could also put the problem into a class according to whether the outcomes are particularly desired or feared [14]; in the evaluation phase, individuals will then adopt an extremely risk averse stance for feared outcomes, and a stance based more efficiency and maximizing expected values (‘waste not, want not’) for outcomes that are less feared [42].
Heuristics, the subconscious processes of evaluating information and making choices, are efficient (in terms of information and time requirements) means of generating satisfactory outcomes in situations the individual most often faces [24]. They can be viewed in evolutionary terms: individuals learn to apply the heuristics that generate better outcomes, but there is no guarantee that heuristics will necessarily generate the ‘best’ outcome, and it is possible for the individual to apply an inappropriate heuristic when facing a new or unfamiliar type of problem [54]. While the theory of heuristics developed out of the observation of choice anomalies – individuals making counterproductive or nonsensical choices – recent work suggests that in many ordinary situations, heuristics actually outperform conscious attempts at maximization, once the actual information and cognitive constraints are factored in [23].
People use heuristics, likewise, in order to estimate and describe probabilities. When asked to assign a likelihood of a one-time situation matching a class of outcomes, they evaluate how ‘representative’ the particular situation is of each class, i.e., whether there is a strong analogy between the broader class and the particular instance. For example, researchers asked participants to estimate relative chances that a particular woman (Linda) was engaged in different types of life work, with the choices including ‘bank teller’ and ‘bank teller who is active in the feminist movement’. Because the description of Linda had included the fact that she cared about social justice, people estimated higher likelihood for the latter class, even though it is obviously a subset of the former; Linda seemed better to represent a socially active bank teller than a mere average bank teller [71]. When asked to evaluate relative frequencies of different events, people appear to search through their memory for similar events, and judge as more likely the ones for which memories are more ‘available’. For example, people often rate airplane travel as more dangerous than car travel, since news of airplane accidents is more dramatic, and creates lasting impressions [71]. Consistent with the availability heuristic, individuals who hear of an event from a greater number of sources will rate the event as more likely than those who hear of it from fewer sources [70,76].
The availability heuristic appears to be sensitive to the base-rates of different events [77]. For example, when people are asked to assign a fixed numerical probability value to a forecast of ‘slight’ chance of rain in London, the number is higher than for a ‘slight chance’ of rain in Madrid [74]. But the sensitivity to base-rates can lead to errors when the new information already includes the base-rate information. In one experiment, people were told that they had a specific numerical chance of contracting a tropical disease at a particular travel destination, either Calcutta or Honolulu. Later, when asked to remember what that number was, the people for whom the travel destination was Calcutta, where tropical diseases are in general more frequent, remembered higher numbers than those for whom the destination was Honolulu [82]. Furthermore, when problems are framed differently, and people use the representativeness heuristic, people are often insensitive to different base-rates [71]. Thus, when asked to assign likelihood that a given person is engaged in a particular profession, people forget that there are some professions (e.g., astronaut) in which almost nobody is engaged in.
Because small events happen more frequently than large ones, people often use the magnitude of an event as a proxy for its base-rate [75]. For example, experiment subjects were asked to decide on the numeric probability they believe their doctor had in mind when describing the likelihood of different medical conditions of different severity, including warts, cancer, and ulcers. For each medical condition the doctor used same probability words, such as ‘slight chance’. Following the base-rate phenomenon, people often appeared to rate the more severe maladies, which occur less frequently, with a lower probability estimate [77]. But when they were given illnesses or similar base-rates, and told that the base-rates were in fact the same, people's answers appeared to correlate highly, and in an interesting manner, with the severity of the illness. For non life-threatening illnesses, the more serious maladies were assigned a higher probability estimate. As soon as the illness became life threatening, however, the people appeared to ‘decode’ the physician's communication, and assign it a lower probability estimate. This is unsurprising: when asked to describe, in words, the likelihood of different events, the language they use reflects not only the probability but also the magnitude of the different events [14]; people use more ominous sounding language (e.g., ‘very likely’, as opposed to ‘somewhat likely’) to describe the more dreaded outcomes.
As should already be evident, whether the task of estimating and responding to uncertainty is framed in stochastic (usually frequentist) or epistemic (often Bayesian) terms can strongly influence which heuristics people use, and likewise lead to different choice outcomes [23]. Framing in frequentist terms on the one hand promotes the availability heuristic, and on the other hand promotes the simple acts of multiplying, dividing, and counting. Framing in Bayesian terms, by contrast, promotes the representativeness heuristic, which is not well adapted to combining multiple pieces of information. In one experiment, people were given the problem of estimating the chances that a person has a rare disease, given a positive result from a test that sometimes generates false positives. When people were given the problem framed in terms of a single patient receiving the diagnostic test, and the base probabilities of the disease (e.g., 0.001) and the reliability of the test (e.g., 0.95), they significantly over-estimate the chances that the person has the disease (e.g., saying there is a 95% chance). But when people were given the same problem framed in terms of one thousand patients being tested, and the same probabilities for the disease and the test reliability, they resorted to counting patients, and typically arrived at the correct answer (in this case, about 2%). It has, indeed, been speculated that the gross errors at probability estimation, and indeed errors of logic, observed in the literature take place primarily when people are operating within the Bayesian probability framework, and that these disappear when people evaluate problems in frequentist terms [23,58].
The ability to make calculations based on assessed probabilities also appears to influence people's stance toward risk. Probability estimates based on relative frequencies of events, or on a well-defined and well-understood stochastic process (e.g., tossing a fair coin), generate far less risk aversion than do ambiguous probability estimates, where the exact probability distribution is unknown; just as individuals draw qualitative distinctions between problems involving certain versus uncertain outcomes, they tend to adopt a much more risk-averse decision stance when faced with ambiguity and poorly defined risk [6,11,65]. One explanation is that in the case of ambiguous probabilities, people feel less in control [29]; this could stimulate a negative emotional response toward the risk, and therefore greater feelings of discomfort taking the risk on [15]. Finally, it has also been shown that the language people use to describe this ambiguous, or epistemic, uncertainty is different from that used to describe frequentist information. When describing events of high frequency, people offer probability estimates along the full interval from zero to one; for epistemic uncertainty, people are much more likely to express an estimate of 0.50, as in “it's a fifty–fifty chance” [4]. All of this suggests, then, that there is a great deal of room for misunderstanding, and miscommunication, especially in situations where incomplete confidence in predictive models – epistemic or structural uncertainty – is one of the primary sources of the probability estimates. Likewise, the ways in which different types of uncertainty is framed – especially whether it is described in epistemic or stochastic terms – can greatly influence the choices that people make.
2.2 Approaches to probability communication
Effective communication and decision-support promotes sensible goal-oriented decision-making, while preserving the credibility and legitimacy of the information being communicated. Interestingly, these separate elements derive from two very different strands of social-science research. Indeed, it is only in the last decade or so that both elements have been recognized as important by a common group of people, and that communication practices have developed to incorporate both.
The field of risk communication grew out of economists' observations that both private decision-makers and public policy-makers were making irrational choices with respect to issues of health and safety [22,37]. Economists had long come to the conclusion that socially efficient outcomes would result when the world consisted of rational decision-makers making their own self-interested choices; any interference in the process of choice, by government or other third-parties, would lead to poor outcomes [84,85]. However, for many health risks, consumers appeared to make decisions that were not in their own self-interest, apparently out of common difficulties dealing with risks of extremely low probability [73]. Contemporaneously, the behavioral economists, using experimental methods, began to show that people making decisions under conditions of uncertainty exhibit predictable ‘biases’, departures from the normative model of rationality [69,71]. Moreover, it appeared that when individuals operated in groups – such as in the policy-making process – their individual biases could compound, leading to extremely distorted public decisions [36]. A study by the United States Environmental Protection Agency, for example, showed very little correlation between the societal resources used to minimize particular risks, and the actual magnitude of those risks [18]. When economists began to calculate the opportunity costs of risk regulation, they came to the conclusion that the pervasiveness of poor intuition in risk management and risk policy was actually making life worse for people, and perhaps more dangerous [3]. The implications seemed clear. First, where people could be predicted to make poor decisions, there was justification for government paternalism, moving the locus of decision-making from the individual to the trained government risk analysis [7,85]. Second, where democratic or social processes led to poor public decision-making, there was a need for greater bureaucratic autonomy, allowing the trained experts to be insulated from public opinion as they regulated people's risk behavior [3].
The second strand, science and technology studies, grew out of sociologists' observations that people were becoming increasingly skeptical and mistrusting of scientific advice and science as a basis for policy-making [19,34]. Numerous case studies showed that the effectiveness of science and technology advisors within government appeared to correlate with the institutional structure within which they operated [12,27,33]. Other case studies showed a similar pattern at the interface of science and private decision-making; when the communication process went seriously wrong, people actually preferred to make decisions under conditions of ignorance, rather than accepting the opinions of scientists [83]. The result was to suggest that certain communication practices – in particular a two-way flow of information – could assist in making scientific information credible and legitimate; more importantly, certain types of institutions are the ones that could best engage in these practices [28]. Case study research has shown that institutions with identifiable ties to both scientists and decision-makers – incentives to serve the interests of both parties – are more effective at promoting decision-making based on scientific knowledge [21]. Recent experimental results show this to operate at the individual level as well, in terms of heuristics that people use for establishing trust in expert advisors [56]. The implication is that the early approaches to risk communication, described in the last paragraph, can generate poor outcomes: when science advisors and decision-makers are insulated from public opinion, and at the same time tell people and policy-makers what to do, the people and the policy-makers will increasingly ignore their advice.
The most recent approach, incorporating both strands, incorporates an awareness of decision heuristics and framing into a participatory and distributed decision-support system [8–10], anticipating the potential for ‘cognitive conflicts’ between the communicators and the users of the information [66]. While scientists may well believe that their approach to using information will generate unambiguously better results, that is not necessarily the case: in many situations, there are other critical aspects to the decision, often involving issues of fairness, justice, and fear, that decision-makers consider valid, but that the experts, for lack of a good theory, have failed to incorporate into their methods [52]. Before scientists, or boundary organizations, can effectively communicate risk, they need to understand the ‘mental models’, essentially the framing and heuristics people use when making decisions on the basis of the information, as well as the actual decisions that could be taken and the objectives, where known [40]. Stakeholder participation is the vehicle through which this information moves from user to producer [10,31].
The assessment products – both the ongoing social process and any written documents – then need to address explicitly the framing and uses of heuristics that both the communicators and users hold, explore ways in which some of these may not be appropriate for using the information, and ultimately arrive at a set of mental models that ought to be acceptable to everyone. Since the stakeholders' decision and available choice sets determine what is an appropriate framing and heuristic, the best way to engage in this communication process is by using the information to solve problems, both real and hypothetical [60]. How the assessment frames the information is determined by the choices and goals of the users.
3 The current IPCC approach
That the IPCC, and environmental assessments in general, have made great strides in recent years to improve their practices of risk communication cannot be understated. In several studies, researchers have noted that early efforts to communicate seasonal climate forecasts to decision-makers explicitly avoided presenting the results in probabilistic terms at all, out of a belief that decision-makers lacked the ability to interpret uncertainty [48,53]. With numerous examples of a loss in credibility resulting from perceived forecasting errors [38,49], as well as evidence that even illiterate farmers can learn how to use probability estimates [51], practices have turned around entirely, with full disclosure of uncertainty deemed essential [30,67]. Likewise, a study of climate change assessments examining assessment reports published prior to 1997 – basically through the IPCC Second Assessment Report but no further – found some troubling biases [50]. The large-scale assessments that could be classified as intending to promote agreement within the policy community on the state of the science – reports such as those coming from the IPCC – were found to be more likely to fail to report low-probability high-magnitude events, compared to smaller scale and more advisory assessments. The author explained this bias by speculating that the large-scale assessments, deliberately or not, were acting strategically, aware that any attention they paid to extreme events would raise controversy, because of the choices they would inevitably have to make in framing the event and the type of uncertainty. Against this history, the efforts of the IPCC Third Assessment Report (TAR) to address the issue of uncertainty communication are impressive. In this section, we describe that approach, and examine empirical results showing potential further room for improvement.
3.1 IPCC TAR approach
The IPCC TAR, as with previous IPCC assessments, was divided into three working groups, with Working Group I (WGI) describing the basic science of climate change, WGII describing impacts and vulnerability to climate change, and WGIII describing mitigation option. The IPCC as a whole developed a methodology to describe climate change uncertainty, with the different working groups following the methodology to differing extents. The approach relied on a set of words and phrases, with each word or phrase referring to a fixed range of probability estimates, as shown in Table 1.
Definitions of the probability words and phrases used in the IPCC Third Assessment Report
Définitions des mots et des phrases utilisés pour caractériser les probabilités dans le troisième rapport d'évaluation du GIEC
Probability range | Descriptive term |
<1% | Extremely unlikely |
1–10% | Very unlikely |
10–33% | Unlikely |
33–66% | Medium likelihood |
66–90% | Likely |
90–99% | Very likely |
>99% | Virtually certain |
The decision to take this approach was not taken lightly. As one element of their investigation into the issue, WGII commissioned a background paper examining the issues surrounding probability communication [46]. That paper covered much of the same theory described in this paper, and made a number of recommendations, consistent with the latest thinking in risk communication. In particular, that paper recommended trying to help people use the probability estimates, by providing them in several forms (words and numbers), describing in detail the sources of the uncertainty (e.g., stochastic or structural), and offering examples of using the probability estimates to unravel policy problems. Perhaps because the guidance to lead authors was not entirely clear and straightforward, many authors decided not to adopt all of these recommendations. The decision to use words to describe probabilities was based on evidence that most people find words to be a more intuitive way of describing the likelihood of one-time events; by linking those words with a fixed scale, the authors could remove likely confusion about what the words actually meant [5].
In general, the approach of the IPCC TAR should be considered a step in the right direction, in that it was a deliberate effort to address the abilities and the needs of the assessment users, and not just following the descriptive practices of the scientific community [41,78]. Other cases where assessors have continued describe uncertainty in purely quantitative terms, rather than making an effort to describe information more intuitively, have come under criticism [59]. The United States National Research Council has issued a report highlighting the importance of making probability information user-friendlier, in the manner of the IPCC TAR [47]. There have been suggestions to take the approach of the TAR, and ground it in more legal contexts, such as the burden of proof in criminal trials [80]. In short, as the IPCC considers its approach for the fourth assessment report, it would be wise to build on, rather than discard, that taken in the third.
There is, however, room for improvement. Many of the recommendations of the TAR background paper were not adopted. In the Discussion section of this paper we suggest how those could now be implemented. Additionally, the approach that was taken, using words with fixed meaning, could have actually led to some additional confusion. One empirical study, already published, shows the potential for bias when untrained readers read the report [55]. A second study, examining trained policy-makers, shows that the use of the fixed scale may not have eliminated imprecision in the meanings of the probability words and phrases. In the following subsection, we describe these two studies.
3.2 Critical studies of the IPCC TAR approach
The literature that we have reviewed so far suggests that the IPCC TAR approach, while a step in the right direction, may still be insufficient to cure known biases among decision-makers. Two sets of experiments address this question directly, and their results are consistent with the literature so far reviewed.
3.2.1 Survey of science students at Boston University
Patt and Schrag describe a survey that they conducted in 2001 among science students in the United States [55]. Their objective was to see whether the use of language to describe risk and probability, as done in the IPCC TAR, could introduce bias into people's response to the assessment. Their hypothesis was that people would show a differential response to the probability language, based on the magnitude of the event being described, as the existing literature in behavioral economics suggests [5,74,77,82]. They were additionally interested in seeing if this magnitude effect was present in both types of translation: from numbers to words, and from words to numbers. To examine this, they randomly distributed four versions of a survey, differing on two dimensions. While all of the surveys described an unlikely weather event for the city of Boston in early September, half described an event of high magnitude (a catastrophic hurricane) while the other half described a low magnitude event (a light early season snowfall). For each weather event, half of the surveys asked the participants to answer as they would if they were a weather forecaster communicating to the public on television; this group had to translate the computer model estimate of 10% likelihood for the event into words, with the seven possible answers being those from the IPCC scale. The other half of the surveys asked participants to answer as they would if they were watching a weather forecast on television, in which the event was described as “unlikely, perhaps very unlikely”; they had to assign one of the seven probability ranges to the event.
Fig. 1 presents their results. In Fig. 1a, one sees that for the first group, the ‘weather forecasters’, the distribution of words used to describe the event was shifted to the right for the hurricane, relative to the snowfall. This means that people tended to use more serious sounding language to describe the higher magnitude event. In Fig. 1b, one sees that for the second group, the ‘television audience’, the distribution of probability ranges assigned to the words “unlikely, perhaps very unlikely” was shifted to the left for the hurricane. Although the direction of the shift is the opposite, the underlying effect is identical: for higher magnitude events, the more serious sounding words describe smaller ranges of numerical probability. If indeed this occurs, and to a similar extent, among both risk communicators and information users, then it is possible to communicate probabilistic information using words in a way that is without any bias: the audience would correct for any exaggeration on the part of the communicators. On the other hand, if the communicators use a fixed scale, but the audience does not, then the possibility exists for biased understanding on the part of the users. Given the same language to describe the likelihood of two different events, they will interpret that language to mean that the larger event is less likely than the smaller event. Since larger events, by definition, create more damage than smaller events, it is likely that their overall understanding will be biased towards underestimating the total expected damages. This would bias policy in the direction of under-responding to climate change.
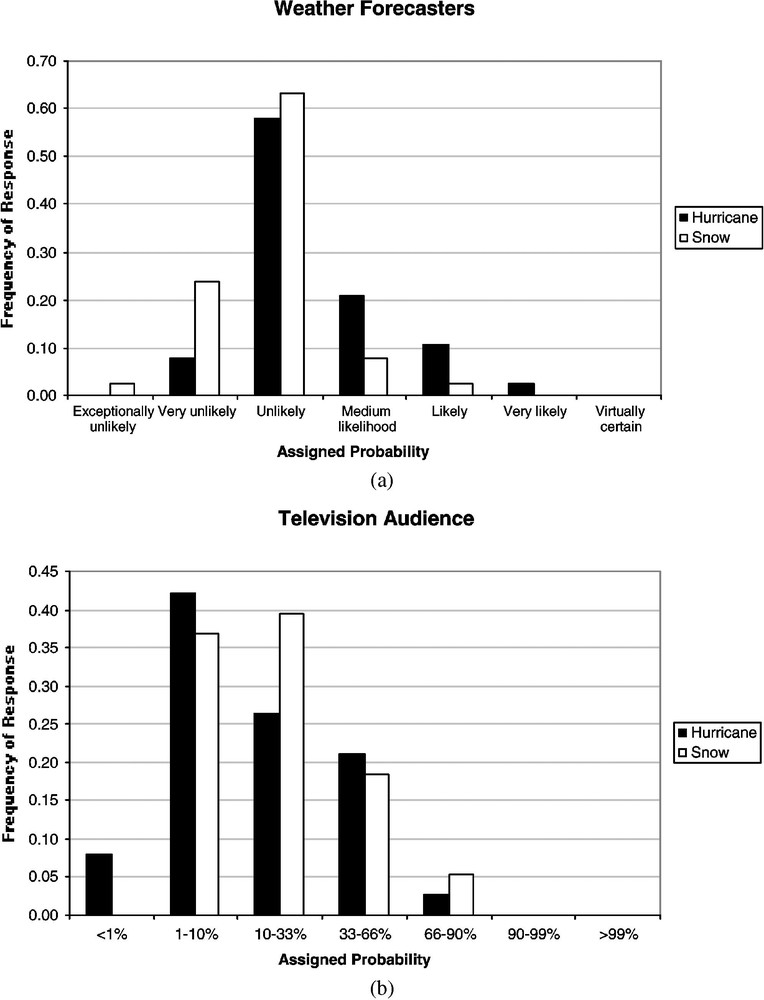
(a) Students in role of weather forecasters. Distribution of answers among participants translating 10% probability into words, for two events: hurricane, and snowfall. (b) Students in role of television audience. Distribution of answers among participants translating “unlikely, perhaps very unlikely” into numerical probability ranges, for two events: hurricane, and snowfall.
(a) Étudiants dans le rôle de spécialistes de la prévision du temps. Distribution des réponses des participants traduisant une probabilité de 10% en mots pour deux événements : une tornade et une chute de neige. (b) Distribution des réponses des participants traduisant « peu probable, sans doute très peu probable » en ordre de grandeur de la probabilité pour deux événements : une tornade et une chute de neige.
3.2.2 Survey at the Ninth Conference to the Parties in Milan, Italy
Fortunately, perhaps, university students are not the people reading the IPCC assessment and making climate change policy, and it was a criticism of the research that the survey audience was not representative [41]. We decided, therefore, to conduct a similar survey among a group of highly informed people, who would be representative of people reading and responding to the IPCC report. We conducted the survey at the Ninth Conference of the Parties (COP) of the United Nations Framework Convention on Climate Change, which took place in Milan, Italy, in December 2003. The COP events draw several hundred participants over the course of two weeks, including not only members of each country's official delegation, but also representatives from non-governmental organizations (NGOs), research institutions, business organizations, and the media. We interviewed 123 of these people at random. In addition to the survey question itself, we asked for people's country, institutional affiliation, and whether they had read all or part of the IPCC TAR WGI report.
The survey we used was similar in principle to that used among the university students, with two changes to make it appropriate to the COP audience. First, we described the roles not as weather forecaster and television audience, but as IPCC lead author and national policy maker. Second, we described two hypothetical impacts of climate change that would occur in the alpine area just north of Milan. The high magnitude event was an increase in the intensity of avalanches, which would make many alpine valleys effectively uninhabitable during the winter. The second was a spread of existing mosquito populations to higher elevations, with added annoyance but no serious adverse health effects. We present the language of the two survey versions in Table 2.
Text of the four survey versions used at COP 9 in Milan
IPCC authors | Policy makers | ||
High magnitude outcome | Low magnitude outcome | High magnitude outcome | Low magnitude outcome |
Imagine that you are a lead author for the IPCC, summarizing impacts for the alpine region in Europe. | Imagine that you are a policy-maker for an alpine region in Europe. | ||
One of the possible impacts is that more intense precipitation events would lead to snow depths far greater than those experienced in the last 500 years. This would overwhelm the capacity of existing passive avalanche control measures – forest areas, steel fences, and valley floor setbacks – and lead to the total destruction of several hundred alpine towns, villages, and ski resorts previously considered safe. | One of the possible impacts is that warmer nighttime temperatures in the summer could cross the threshold that would allow certain mosquito populations to spread from the lowlands, where they would thrive in the lush, mountain forests. While they would not pose any health risks, they would be a significant nuisance for residents and tourists, and many homeowners and hotel operators may decide to install window screens. | One of the possible impacts of climate change for your region is that more intense precipitation events would lead to snow depths far greater than those experienced in the last 500 years. This would overwhelm the capacity of existing passive avalanche control measures – forest areas, steel fences, and valley floor setbacks – and lead to the total destruction of several hundred alpine towns, villages, and ski resorts previously considered safe. | One of the possible impacts of climate change for your region is that warmer nighttime temperatures in the summer could cross the threshold that would allow certain mosquito populations to spread from the lowlands, where they would thrive in the lush, mountain forests. While they would not pose any health risks, they would be a significant nuisance for residents and tourists, and many homeowners and hotel operators may decide to install window screens. |
Through expert elicitation techniques, the scientific community has converged on a probability estimate of 10% for this impact occurring within the next 50 years, and you believe this to be a good estimate. In writing the summary for policymakers, which of the following language would you use to describe to your readers the chances of this happening? | The IPCC Summary for Policymakers, which you trust, is saying that it is unlikely, perhaps very unlikely, that this will actually happen within the next 50 years. Based on this forecast, what do you think the chances of this event happening actually are? | ||
a. Extremely unlikely | a. <1% | ||
b. Very unlikely | b. 1–10% | ||
c. Unlikely | c. 10–33% | ||
d. Medium likelihood | d. 33–66% | ||
e. Likely | e. 66–90% | ||
f. Very likely | f. 90–99% | ||
g. Virtually certain | g. > 99% |
Fig. 2 presents the results of this survey. Qualitatively, it shows the same results as the survey taken of university students. In Fig. 2a, the ‘IPCC Authors’, the distribution for the higher magnitude event is slightly to the right of that for the lower magnitude event, indicating a use of more serious sounding language. In Fig. 2b, the ‘Policy Makers’, the distribution for the higher magnitude event is slightly to the left of that for the lower magnitude event, although the main difference between the two distributions occurs in the tails. Compared to the results from the earlier study, the magnitude of the biases is less, and unlike the earlier study, they are not statistically significant. While these results indicate that the same bias observed among university students is possible, they do not provide convincing evidence that it necessarily exists.
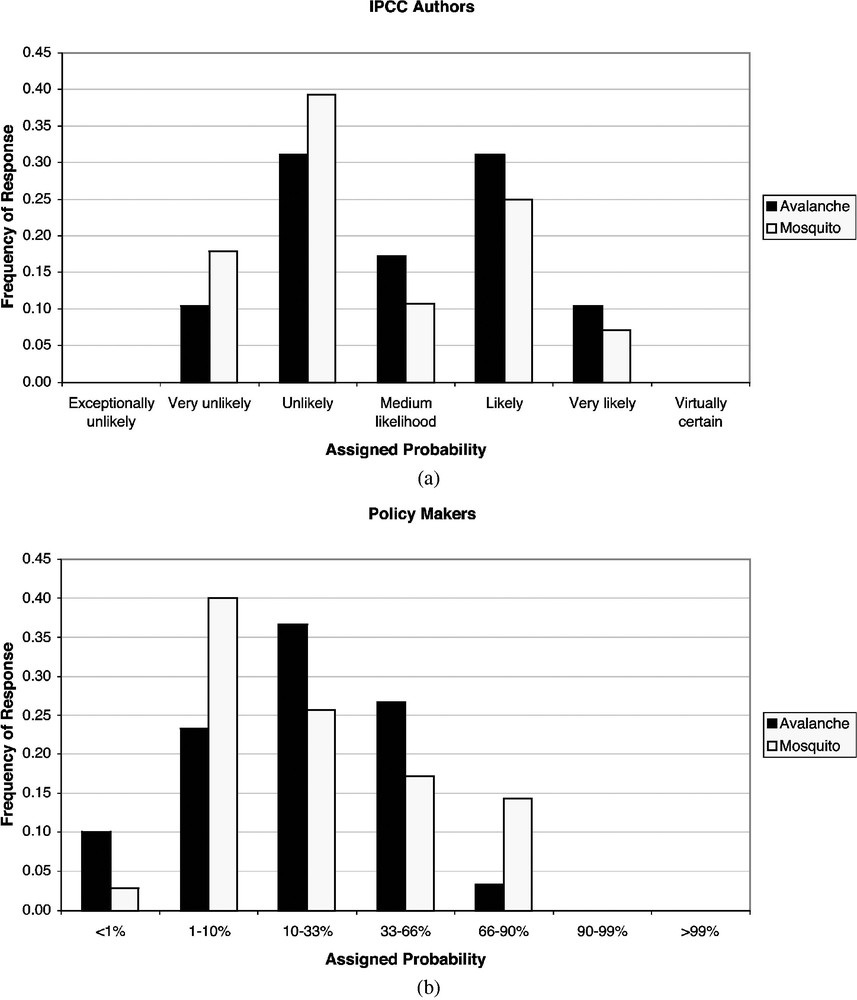
(a) COP participants in role of IPCC authors. Distribution of answers among participants translating 10% probability into words, for two events: more intense avalanches, and higher elevation mosquitoes. (b) COP participants in role of policy makers. Distribution of answers among participants translating “unlikely, perhaps very unlikely” into numerical probability ranges, for two events: more intense avalanches, and higher elevation mosquitoes.
(a) Participants à la Conférence des parties (COP) dans le rôle des auteurs des rapports GIEC. Distribution des réponses des participants traduisant une probabilité de 10% en mots pour deux événements : avalanches plus importantes et présence de moustiques à des altitudes plus élevées. (b) Distribution des réponses des participants traduisant « peu probable, sans doute très peu probable » en ordre de grandeur de la probabilité pour deux événements : avalanches plus importantes et présence de moustiques à des altitudes plus élevées. Masquer
(a) Participants à la Conférence des parties (COP) dans le rôle des auteurs des rapports GIEC. Distribution des réponses des participants traduisant une probabilité de 10% en mots pour deux événements : avalanches plus importantes et présence de moustiques à ... Lire la suite
3.2.3 Comparison of aggregate survey results
Two interesting points do result from the comparison of the responses of university students and the various COP participants when we aggregate results from all versions of the survey, i.e., when we do not examine differences between different survey versions, but rather between different groups of survey participants. First, the variance in replies among the COP participants is greater than that among the university students, and with that a higher proportion of answers in the higher probability ranges. To examine this, we can assign a value to each survey response, representing the middle percentage number for that range. Hence answer a, which is either ‘exceptionally unlikely’ or ‘<1%’ depending on the survey version, can be assigned a value of 0.5%, half way in between 0% and 1%. The two ‘correct’ responses, given the IPCC definitions, are b (‘very unlikely’ or ‘1–10%’) and c (‘unlikely’ or ‘10–33%’), which have mean values of 5.5% and 21.5%, respectively. If one were to observe half of the people choosing b, and half of the people choosing c, one would calculate a mean response of 13.5% and a standard deviation of about 8%. Among the university students, the mean response is 20.3% with a standard deviation of 24.8%, meaning that a great number of students gave responses that were too high, either in the choice of words or in the probability distributions. Among the COP participants, the mean response is 29.2% with a standard deviation of 35.7%. This difference in means is significant at the 90%, but not the 95% confidence level, using a parametric difference-in-difference test.
Second, how people responded to the survey does not depend on whether they reported reading the IPCC TAR WGI main report or Summary for Policy Makers (SPM). Of the 123 survey respondents at the COP, 75 reported having read all or part of the main report or SPM, while 48 reported not having read any part of either. Given that both the main report and the SPM define the connection between the probability words and phrases and the numerical ranges, to the extent that the people read either document carefully, and remembered what they had read, we would expect them to be more likely to give a ‘correct’ answer – either b or c – to the survey. Fig. 3 shows the responses among these two groups, as well as for the university students who had taken the earlier survey. In fact, the students were the most likely to give the “correct” answer, and the COP participants who had read the WGI report or SPM the least likely. The latter group was the most likely to give answers that were ‘too high’, either a numerical range or a verbal description that was larger than IPCC definition. None of the differences between the three groups of respondents shown in Fig. 3 is statistically significant.
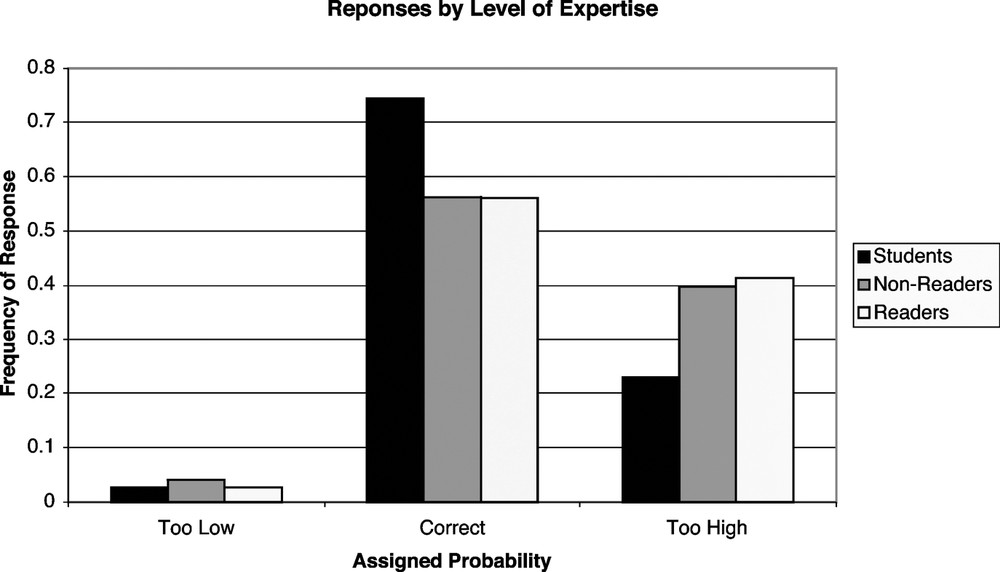
Distribution of answer types across university students, COP participants who reported not having read the IPCC TAR, and COP participants who reported having read the IPCC TAR. “Too low” indicates answer choice a on the survey. “Correct” indicates answer choice b or c on the survey. “Too high” indicates answer choice d, e, f, or g on the survey.
Distribution des types de réponse parmi les étudiants de l'université, les participants à la conférence des parties déclarant n'avoir pas lu le troisième rapport d'évaluation du GIEC et les participants à cette même conférence déclarant avoir lu ce rapport d'évaluation. « Too low » correspond à la réponse a de l'expérience. « Correct » indique aux réponses b ou c de l'expérience. « Too high » correspond aux choix d,e,f, ou g de l'expérience. Masquer
Distribution des types de réponse parmi les étudiants de l'université, les participants à la conférence des parties déclarant n'avoir pas lu le troisième rapport d'évaluation du GIEC et les participants à cette même conférence déclarant avoir lu ce rapport d'évaluation. ... Lire la suite
The results indicate that there are no significant differences in responses by the participants at the COP and the university students. In addition to the lack of significant difference within the COP survey by readership of the TAR WGI main report or SPM, there were no significant differences according to the participants' professional affiliation (national delegation, NGO, research organization, business organization, or media) or national origin. This suggests that most people, except perhaps for the actual authors of the IPCC WGI report, link probability language with numerical ranges using intuitive heuristics, rather than formal definitions. This could result in bias in terms of differential interpretation of small and large magnitude events, as the literature suggests [5,77]. For unlikely events, such as occurring with only a 10% probability, it also results in some over-response, also consistent with the idea of probability weighting from the behavioral economics literature [72].
4 Discussion
The challenge of communicating probabilistic information so that it will be used, and used appropriately, by decision-makers has been long recognized. People both interpret evidence of uncertainty and respond to their interpretations using a variety of heuristics, and while these heuristics have allowed people to survive and to lead productive lives, they can also lead to predictable errors of judgment. In some cases, the heuristics that people use are not well suited to the particular problem that they are solving or decision that they are making; this is especially likely for types of problems outside their normal experience. In such cases, the onus is on the communicators of the probabilistic information to help people find better ways of using the information, in such a manner that respects the users' autonomy, full set of concerns and goals, and cognitive perspective.
That these difficulties appear to be most pronounced when dealing with predictions of one-time events, where the probability estimates result from a lack of complete confidence in the predictive models. When people speak about such epistemic or structural uncertainty, they are far more likely to shun quantitative descriptions, and are far less likely to combine separate pieces of information in ways that are mathematically correct. Moreover, people perceive decisions that involve structural uncertainty as riskier, and will take decisions that are more risk averse. By contrast, when uncertainty results from well-understood stochastic processes, for which the probability estimate results from counting of relative frequencies, people are more likely to work effectively with multiple pieces of information, and to take decisions that are more risk neutral.
In many ways, the most recent approach of the IPCC WGI responds to these issues. Most of the uncertainties with respect to climate change science are in fact epistemic or structural, and the probability estimates of experts reflect degrees of confidence in the occurrence of one-time events, rather than measurement of relative frequencies in relevant data sets. Using probability language, rather than numerical ranges, matches people's cognitive framework, and will likely make the information both easier to understand, and more likely to be used. Moreover, defining the words in terms of specific numerical ranges ensures consistency within the report, and does allow comparison of multiple events, for which the uncertainty may derive from different sources.
We have already mentioned the importance of target audiences in communicating uncertainties, but this cannot be emphasized enough. The IPCC reports have a wide readership so a pluralistic approach is necessary. For example, because of its degree of sophistication, the water chapter could communicate uncertainties using numbers, whereas the regional chapters might use words and the adaptive capacity chapter could use narratives. “Careful design of communication and reporting should be done in order to avoid information divide, misunderstandings, and misinterpretations. The communication of uncertainty should be understandable by the audience. There should be clear guidelines to facilitate clear and consistent use of terms provided. Values should be made explicit in the reporting process” [32].
However, by writing the assessment in terms of people's intuitive framework, the IPCC authors need to understand that this intuitive framework carries with it several predictable biases. First, the language people use to describe uncertainty is ambiguous, with variance in definition across the population, and that ambiguity does not necessarily become less when people read that the words are being used in an non-ambiguous manner. Second, within people's intuitive use of language, there is a certain degree of probability weighting, pushing all likelihood in the direction of a 50:50 chance. Third, the meanings of the words are context dependent, sensitive to perceptions of base-rates, and the magnitudes of the events being described. As the study reported here suggests, those who had read the IPCC's TAR, or parts of it, exhibited the same biases as those who did not read it. Communicating effectively, so as to eliminate biases and allow the decision-makers themselves to arrive at wise judgments, is a difficult goal to attain.
The literature suggests, and the two experiments discussed here further confirm, that the approach of the IPCC leaves room for improvement. Further, as the literature suggests, there is no single solution for these potential problems, but there are communication practices that could help. First, when defining the probability words and phrases within the assessment report, it would be worthwhile briefly discussing that such a rigid framework does not necessarily match people's intuitive use of language. Such a “warning label” will not eliminate the potential for bias, but for conscientious readers could help to lessen it. Second, when using probability language during the text of the report, it would be helpful to continue to remind readers of the probability ranges the specific language represents. This would particularly appropriate for uncertain events where frequency data does exist, and many people would feel more comfortable adopting a numerical cognitive framework. Third, where appropriate, it may be useful to compare the probabilities of different risks, through a ranking table or similar device. This will help to remove the idea of probability from the realm of the abstract, and allow people to evaluate the relative likelihood of different events of different magnitudes. Furthermore, by comparing likelihood of different risks with different sources of uncertainty, it could force people to evaluate each using multiple cognitive frameworks.
Finally, the use of probability language, instead of numbers, addresses only some of the challenges in uncertainty communication that have been identified in the modern decision support literature. Most importantly, it is important in the communication process to address how the information can and should be used, using heuristics that are appropriate for the particular decisions. Since this requires a discussion of the decision and choice sets of the users of the information, such a discussion within an IPCC report, with its wide international audience, would not be able to include all potential decisions of all potential users. Indeed, much of this problem solving in assessments occurs not in the text of the final document, but in the work with stakeholders that takes place well prior to the writing of the assessment report. For the IPCC report, which is read by a much wider audience than those who participate in its analysis or drafting, there are still ways of addressing user needs. It could be possible, for example, to respond to some of the likely questions that many users will have about how to use the information. In addressing these questions, it would then be possible to discuss the sources of the uncertainty, how the uncertainty can be framed in different ways, and how many people choose, to their benefit and detriment, to respond to uncertainty when making choices. Obviously, there are limits to the length of the report, but within the balancing act of conciseness and clarity, greater attention to full dimensions of uncertainty could likely increase the chances that users will decide to take action on the basis of the new information.
Acknowledgments
Funding for this research came from the United States National Oceanic and Atmospheric Administration Environment Sustainability and Development Program, and the Potsdam Institute for Climate Impact Research, both of which funded Anthony Patt's contribution to this research. Suraje Dessai is supported by a grant (SFRH/BD/4901/2001) from Fundação para a Ciência e a Tecnologia, in Portugal. His attendance of the IPCC Workshop on Uncertainty and Risk in Dublin was possible through the support of the UK's Department for Environment, Food and Rural Affairs. We would like to thank Arthur Petersen, Richard Klein, and two anonymous reviewers for their helpful suggestions on an earlier draft of this manuscript. All remaining errors of fact or analysis are our own.