1 Introduction
Field seismic data contain a significant amount of noise which has a strong interference on the signal for seismic exploration. Noise elimination is a very important step in seismic data processing (Zhang and Klemperer, 2005). Unlike coherent noise, random noise is unpredictable and incoherent in space and time. Abundant methods coming from different fields have been proposed for seismic random noise attenuation, such as median filtering (Duncan and Beresford, 1995), frequency-spatial prediction (Wang, 1999, 2002), wavelet transform (Cao and Chen, 2005), polynomial approximation (Lu et al., 2006), singular value decomposition (Lu, 2006) and so on. However, most of the existing methods have a negative effect on denoising in the case of low signal-to-noise ratio (SNR). It may cause attenuation of useful signals and therefore hinder the seismic data processing. Hence, one of the tasks in the seismic data processing is to design a useful denoising method to eliminate the random noise as much as possible, but at the same time able to preserve or recover the important features in the cases of low SNR.
Time-frequency peak filtering (TFPF) can give an unbiased estimation of the signal in cases where it is linear in time and contaminated by stationary white Gaussian noise (Barkat and Boashash, 1999; Boashash and Mesbah, 2004; Zahir and Hussain, 2000), and is a technique successfully applied to seismic signal processing (Lin et al., 2007; Wu et al., 2011). Conventional TFPF is generally biased when the pseudo Wigner–Ville distribution (PWVD) is used to estimate instantaneous frequency of the analytic signal. The smoothing effect in the frequency domain results in a poor time-frequency concentration of PWVD. That means a deficient energy concentration on the curve of instantaneous frequency. Therefore, conventional TFPF based on PWVD cannot accomplish a very clean recovery of the original signal when SNR is indeed low.
In this paper, we focus on finding an ideal time-frequency distribution to enhance the energy concentration on the curve of the instantaneous frequency. In this way, we can reduce the bias in TFPF and achieve a greater denoising performance. We introduce a new joint time-frequency distribution (JTFD) to be applied to TFPF. This new distribution can be briefly named by its acronym JTFD. JTFD presents a double advantage: it gives rise to a higher concentration of the signal around its IF in time-frequency, and encloses a better ability to remove undesirable noise interferences. TFPF based on JTFD may further reduce random noise affecting the seismic data than conventional TFPF.
2 Time-frequency peak filtering
TFPF allows the attenuation of seismic noise by encoding the noisy seismic signal as the instantaneous frequency (IF) of the analytic signal. Assuming that the time-frequency distribution energy of the analytic signal is concentrated around the IF, we can estimate the instantaneous frequency on the analytic signal by means of the peak of the time-frequency distribution to recover the desired signal.
A seismic trace can be modeled by the following equation:
(1) |
(2) |
(3) |
(4) |
In the conventional TFPF above, we use the PWVD for IF estimation. However, the time-frequency energy of the PWVD in the frequency direction is divergent and it is sensitive to noise interferences that mask the borderline between signal and noise in the time-frequency analysis. In other words, the interferences are the cause by which the peak of PWVD deviates from IF. Hence, this requires the appropriate adjustments in the time-frequency domain to enhance the energy concentration around the IF. To this end, we use the joint time-frequency distribution for filtering, which not only makes the energy of the signal become concentrated on the IF of each component, but also removes the interferences and enables the IF curve be readily identified.
3 Smoothed and filtered PWVD
The smoothed PWVD, hereafter denoted by SPWVD, is a time-frequency distribution that allows us to distinguish the borderline between signal and noise in time-frequency analysis, in such a way that the IF curve can be identified with clarity. The definition of SPWVD is (Auger and Flandrin, 1995):
(5) |
As an example, we start from the Ricker wavelet:
(6) |
The PWVD and SPWVD of a Ricker wavelet highly contaminated by additive white Gaussian noise (SNR = –10 dB) are shown in Fig. 1. The peak of PWVD deviates from IF because of the noise interferences. It is difficult to identify the IF curve in the PWVD as shown in Fig. 1a. As can be seen in Fig. 1b, the energy of the IF curve in the SPWVD is divergent. However, the IF curve can be recognized after smoothing in time and frequency. Hence, we plan to make use of SPWVD to get a broad distribution area of the IF curve.
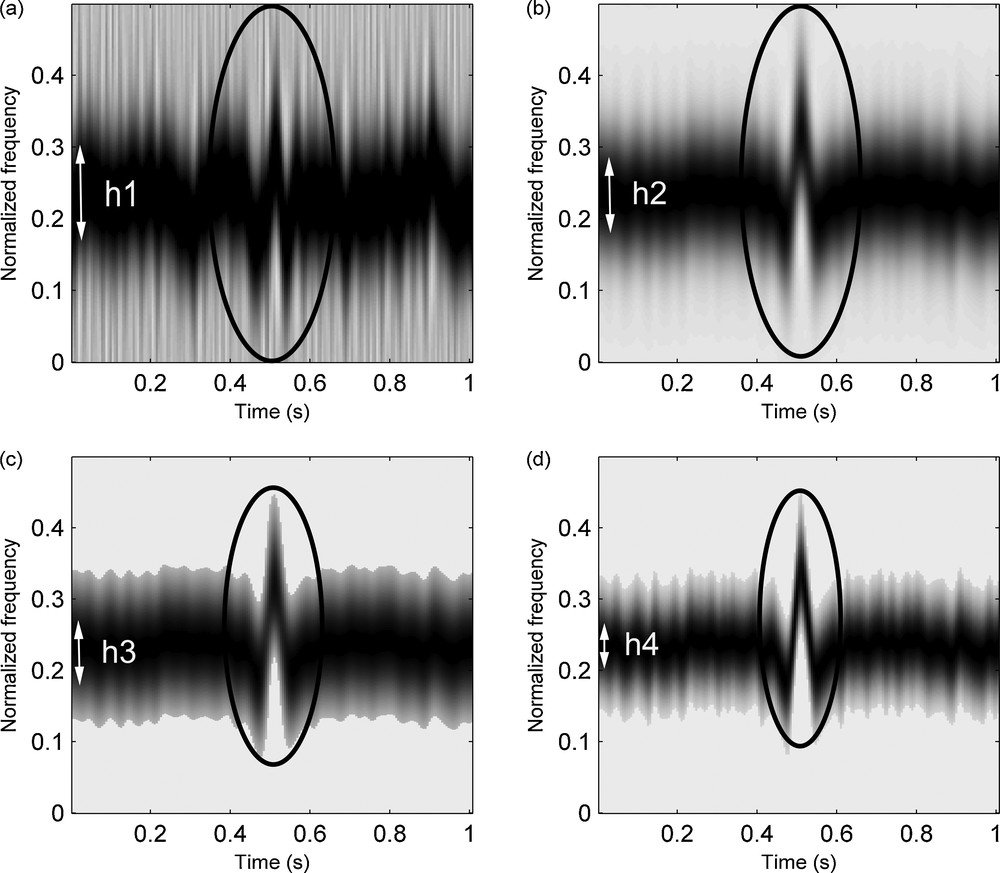
Comparison of different time-frequency distributions obtained from a Ricker wavelet contaminated by additive white Gaussian noise (SNR = –10 dB): (a) pseudo Wigner–Ville distribution (PWVD); (b) SPWVD smoothed distribution (after a double windowing in time and frequency); (c) SPWVD filtered distribution (processed by a step function); (d) JTFD weighted distribution (PWVD after being finally weighted by the previous filtered distribution). The notation h1,…, h4 indicates the width of each function (concentration of energy), while the ellipses enclose waveforms (evident accumulation of energy) in each case.
Due to the smoothing effect in the time-frequency domain, the time-frequency resolution of SPWVD decreases (Ghias et al., 2007). In order to remove divergent time-frequency points and make the IF curve clearer, the matrix SPWVD (t, f) is filtered with a step function. This non-linear filter has an input-output relation of the form:
(7) |
(8) |
The of the Ricker wavelet is shown in Fig. 1c. We can see that the time-frequency area of the signal is more effective and the noise interference is further suppressed. In this distribution matrix, the value of the IF curve is much higher than the noise interference. So we use to weight the PWVD of the signal in order to reduce the effects of the interferences and enhance the time-frequency concentration of the signal around IF.
4 Joint time-frequency distribution JTFD
The joint time-frequency distribution, hereafter denoted by JTFD, is a combination of PWVD and SPWVD. The whole weighting procedure can be expressed as follows:
(9) |
To further demonstrate the advantages of JTFD, we take again the Ricker wavelet. The dominant frequency of the wavelet is 25 Hz. Then we add white Gaussian noise (SNR = 0 dB) to the original wavelet. The amplitude spectra of PWVD, SPWVD and JTFD are shown in Fig. 2. The PWVD curve still reveals the energy fluctuations in the non-instantaneous frequency because of the noise interference, which results in some energy divergence, while the JTFD curve shows maximum amplitude in the instantaneous frequency and the energy here is higher than in the other two distributions. The JTFD amplitude for other frequencies is near to zero, so that JTFD makes the signal energy concentrated in the vicinity of the instantaneous frequency. In this way, we achieve a more accurate estimation of the instantaneous frequency by increasing the energy concentration in time-frequency and eliminating the interferences.
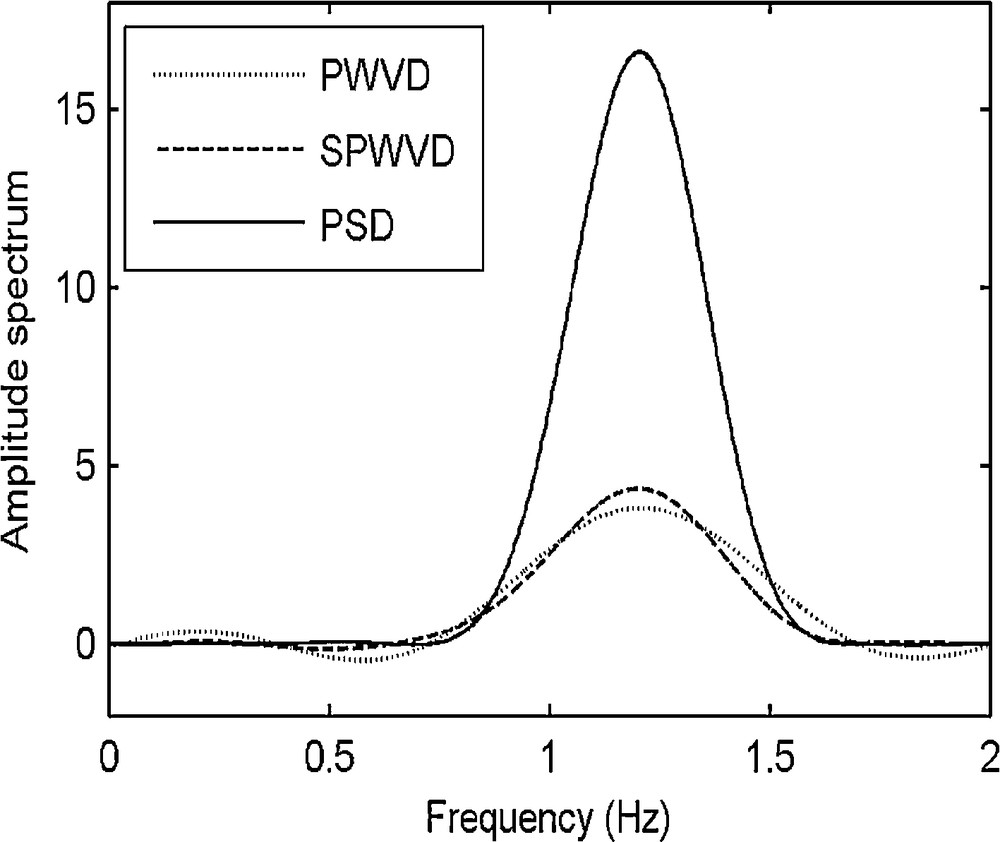
Starting from a Ricker wavelet of dominant frequency 25 Hz, with added white Gaussian noise (SNR = 0 dB), amplitude spectra of PWVD, SPWVD and JTFD.
5 Denoising with synthetic seismic data
JTFD is used for IF estimation to improve the drawbacks in conventional TFPF. To check the effectiveness of the modified TFPF, we present the following examples with synthetic seismic data. The first case is a seismic model with two cross-events, as shown in Fig. 3a. The dominant frequencies of the two events are 20 and 25 Hz. The source wavelet that we applied to simulate the waveform is a Ricker wavelet. In order to deal with more realistic synthetic data, we have added white Gauss noise to our synthetic data. Fig. 3b is a noisy version (SNR = –10 dB) of the noise-free representation in Fig. 3a. Modified TFPF is compared with conventional TFPF. Fig. 3c, d show the results obtained by both methods. Qualitatively, at a glance, we can get an idea of the achieved performance. The conventional TFPF can suppress some amount of random noise, but the effect is not fairly, while with the modified TFPF a larger amount of noise has been removed and the events are more clearly recovered.
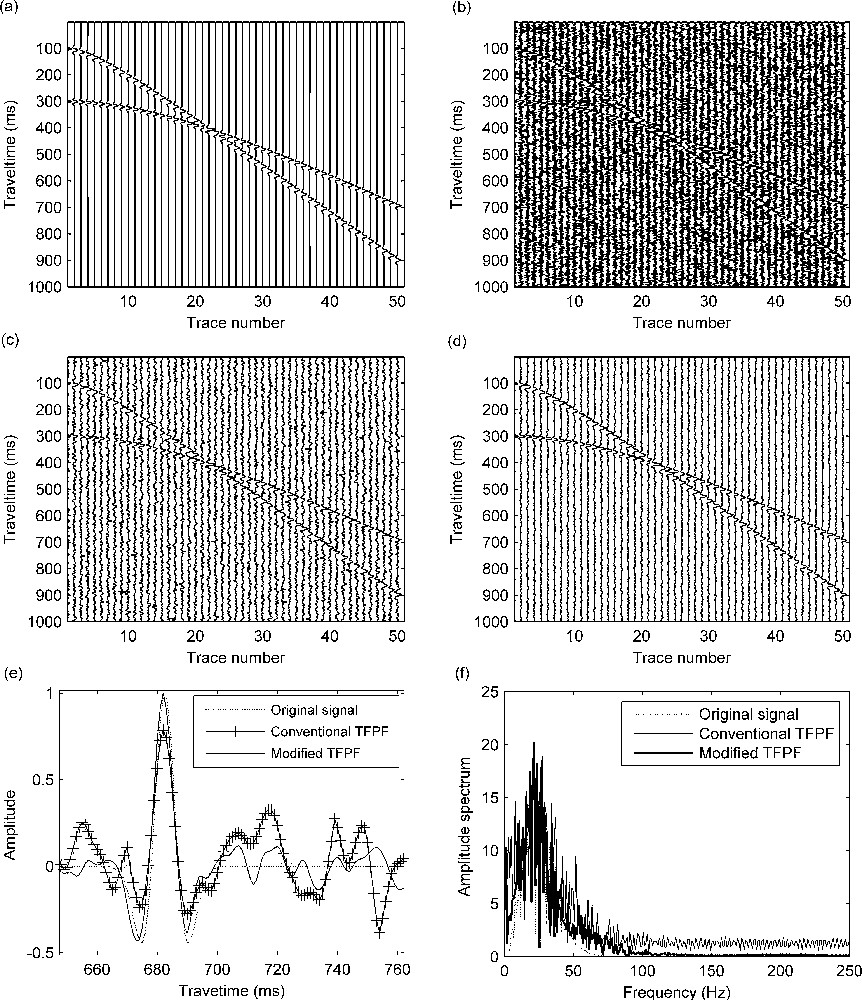
Denoising with synthetic seismic data: (a) Synthetic seismic data. Two Ricker wavelets of dominant frequencies 20 Hz and 25 Hz were considered to simulate synthetic waveforms as natural events; (b) noisy seismic data (SNR = –10 dB). The synthetic data were contaminated with white Gaussian noise; (c) result after applying conventional TFPF; (d) result after applying modified TFPF; (e) comparison of results after processing a single synthetic trace (18th trace) with both filtering types for noise suppression; (f) amplitude spectra of the original signal and the two filtered signals.
We can achieve a more detailed knowledge by analyzing a single trace and its amplitude spectrum. To this end, we extract the 18th trace of the synthetic records. The amplitudes of the waveforms in the time and frequency domains are shown in Fig. 3e, f. Fig. 3e exhibits the denoising result of seismic wavelet from the 18th trace of the synthetic record in time domain. Notice that the filtering result of modified TFPF is closer to the original signal and the amplitude value of noise on both sides of the signal is significantly lower than conventional TFPF does. The comparison in frequency domain is shown in Fig. 3f. We can see that the filtered signal spectrum of the modified method is more close to that of the original signal, and the amplitude spectrum of the signal in high frequency components beyond 100 Hz is approximately zero. So the random noise is better suppressed.
In the following, we check the effectiveness of the modified TFPF on SNR (in dB) and MSE after adding different levels of white Gaussian noise. The signal-to-noise ratio SNR and the mean square error MSE are calculated as follows:
(10) |
(11) |
The resulting SNR and MSE for synthetic seismic data (Fig. 3a) are given in Tables 1 and 2. The larger the SNR value and the lower the MSE value, the better the filtering effects. Figs. 4 and 5 represent curves of SNR and MSE drawn from Tables 1 and 2. As can be seen in Figs. 4 and 5, the modified TFPF has obvious advantages when the SNR is below –10 dB. The SNRs after filtering by modified TFPF are larger and the MSEs are smaller than when applying conventional TFPF. The modified TFPF leads to a noticeably cleaner signal and more accurate estimations.
Signal-to-noise ratio (SNR) after applying conventional and modified Time-frequency peak filtering (TFPF) with different noise levels.
SNR (in dB) computed from the original signal | SNR (in dB) computed from the processed signal by conventional TFPF | SNR (in dB) computed from the processed signal by modified TFPF |
–20 | –13.36 | –10.60 |
–16 | –9.45 | –6.61 |
–12 | –5.41 | –2.67 |
–8 | –1.26 | 1.09 |
–4 | 2.34 | 4.64 |
0 | 5.56 | 7.62 |
4 | 8.05 | 9.82 |
8 | 9.73 | 11.24 |
MSE after applying conventional and modified Time-frequency peak filtering (TFPF) with different noise levels.
SNR (in dB) computed from the original signal | MSE computed from the processed signal by conventional TFPF | MSE computed from the processed signal by modified TFPF |
–20 | 0.5384 | 0.3915 |
–16 | 0.3407 | 0.2520 |
–12 | 0.2135 | 0.1617 |
–8 | 0.1359 | 0.1045 |
–4 | 0.0878 | 0.0699 |
0 | 0.0603 | 0.0488 |
4 | 0.0454 | 0.0373 |
8 | 0.0379 | 0.0315 |
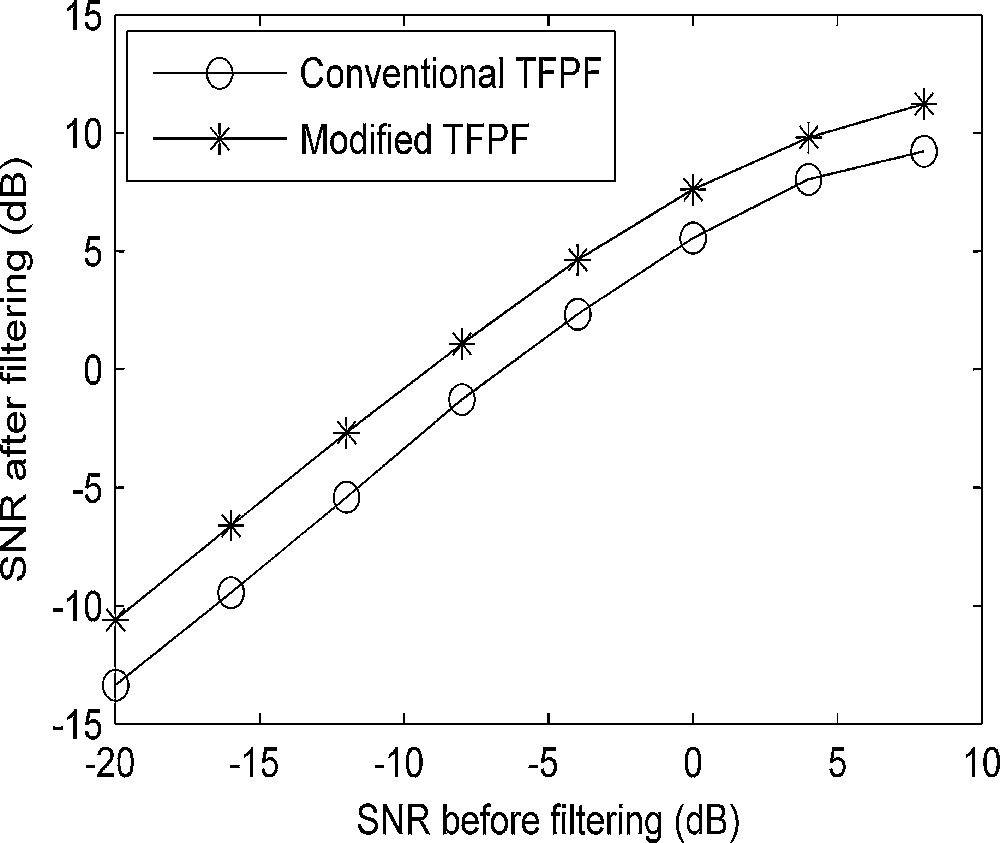
SNR curves obtained from the two denoising methods (Table 1) versus SNR before filtering. The values correspond to different noise levels.
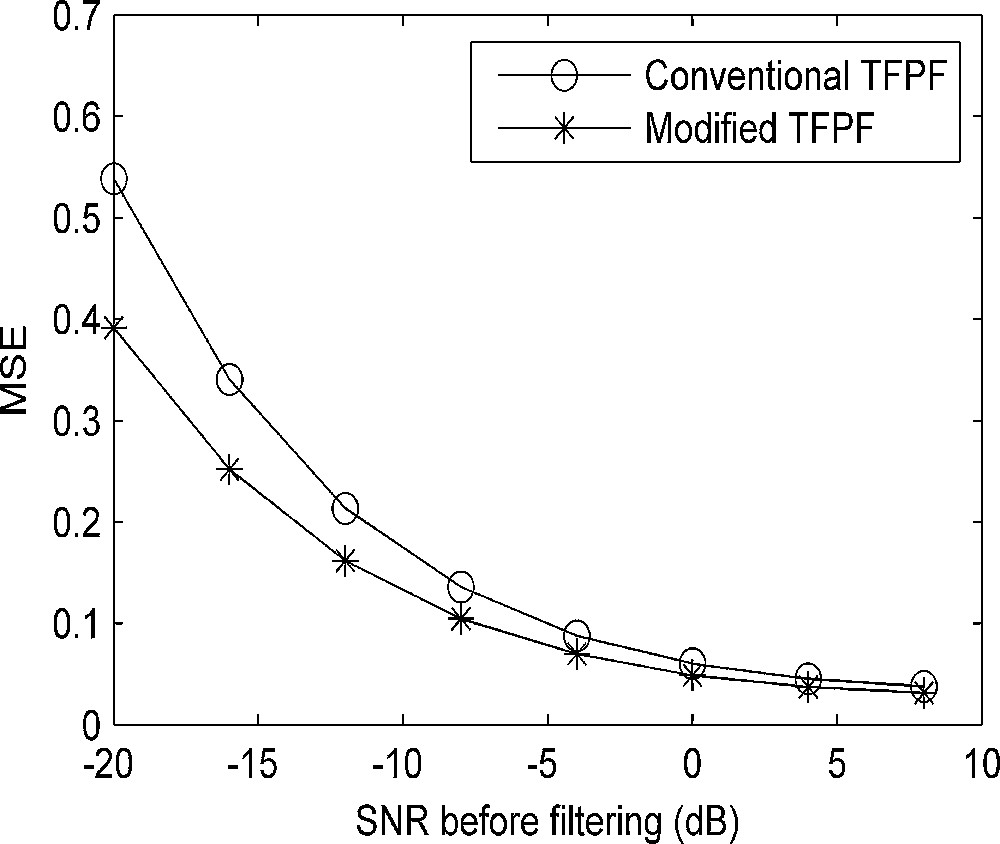
MSE curves obtained from the two denoising methods (Table 2) versus SNR before filtering. The values correspond to different noise levels.
The second example consists of a more complex model with four hyperbolic reflection events, as shown in Fig. 6a. Four Ricker wavelets of dominant frequencies 25 Hz, 30 Hz, 35 Hz and 40 Hz were used to simulate synthetic waveforms as natural events. As before, we have added white Gauss noise to the synthetic model. The noisy record with SNR = –15 dB is shown in Fig. 6b; in this illustration, the events are almost completely submerged by the strong noise. In this example, the modified TFPF is compared with conventional TFPF and the well-known wavelet-transform filtering method. Fig. 6c–e show the denoising results obtained by the wavelet-transform filtering method, conventional TFPF and modified TFPF, respectively. It is to be noted that the events in Fig. 6c, d can be hardly identified, especially the deeper event, while they are all recovered in Fig. 6e. Thus our modified TFPF method proves to be a very effective tool to clean seismic data contaminated with high noise levels.
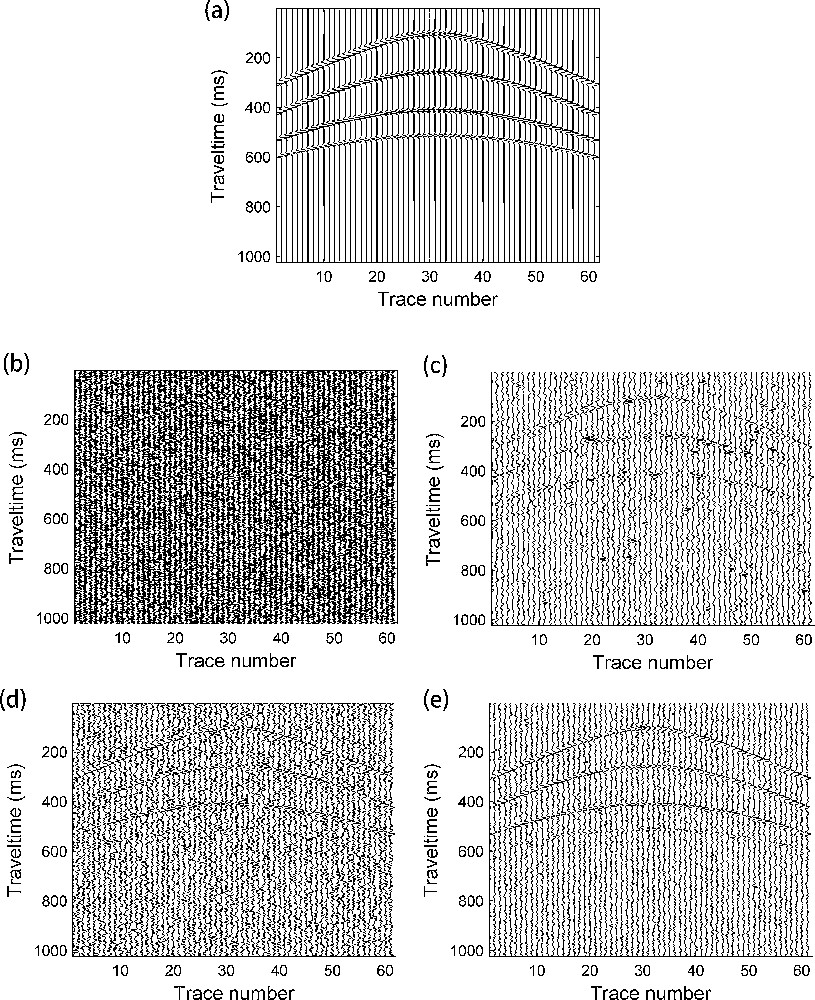
Results after filtering synthetic seismic data with low SNR: (a) four Ricker wavelets of dominant frequencies 25 Hz, 30 Hz, 35 Hz and 40 Hz were used to simulate synthetic waveforms as natural events; (b) noisy seismic data (SNR = –15 dB) contaminated with white Gaussian noise; (c) result after using the wavelet-transform filtering method; (d) result after using conventional TFPF; (e) result after using modified TFPF.
6 Denoising with field seismic data
To verify the versatility of the proposed method, we applied it to field seismic data. We have considered a common shot point seismic gather for testing, as shown in Fig. 7a. The spatial gap between geophones is 30 m and the traces are sampled with a frequency of 1000 Hz. Again, we have contrasted the results obtained through modified TFPF with those obtained with conventional TFPF and the wavelet-transform filtering method. As can be seen, when comparing the results to each other, the latter method and the conventional TFPF method do not reduce the random noise (Fig. 7b, c, respectively) with the same effectiveness that the modified TFPF (Fig. 7d), such as is highlighted within the rectangles. To visually assessing the quality of the results, we show an enlarged view between traces 6–69 and double time 701–1724 ms in Fig. 8a. The reflection events processed through modified TFPF (Fig. 8d) are more clearly recovered, as well as the continuity of the energy arrivals (see in the interior of the ellipses) than with the other two methods (Fig. 8b, c). According to these experimental results, the proposed seismic denoising method has a greater advantage for eliminating random noise and preserving the reflection events more efficiently if we compare it with the other two methods.
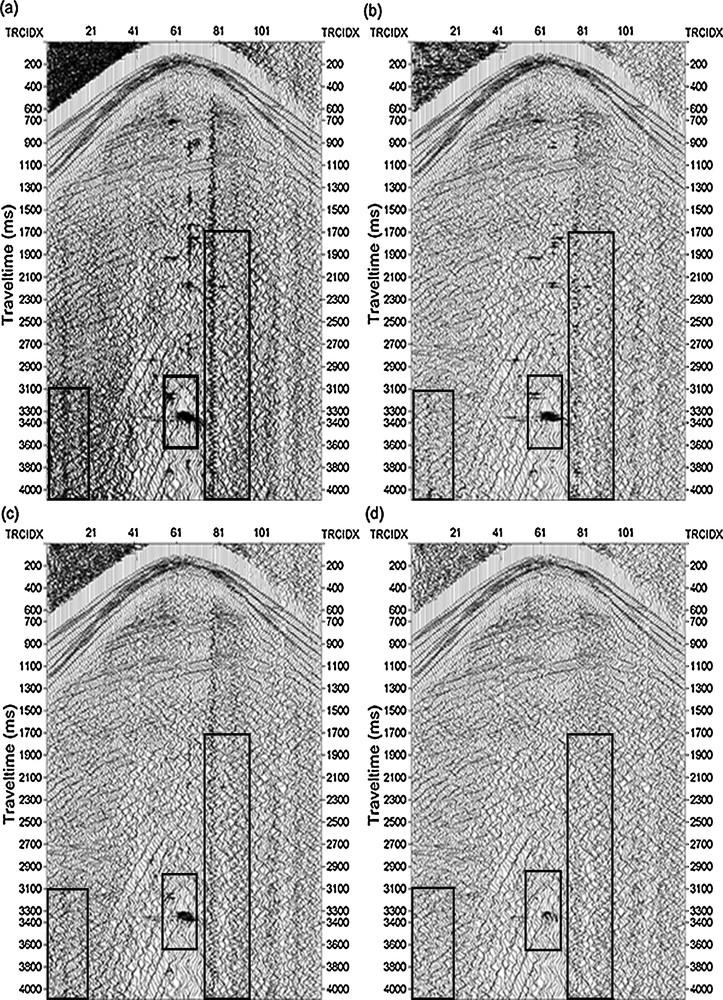
Results obtained with field seismic data after using conventional and modified time-frequency peak filtering: (a) field data (common shot point seismic data); (b) after applying the wavelet-transform filtering method; (c) by using conventional TFPF; (d) by using modified TFPF. Rectangles delimit those areas where we can better observe the progressive efficiency of the filtering.
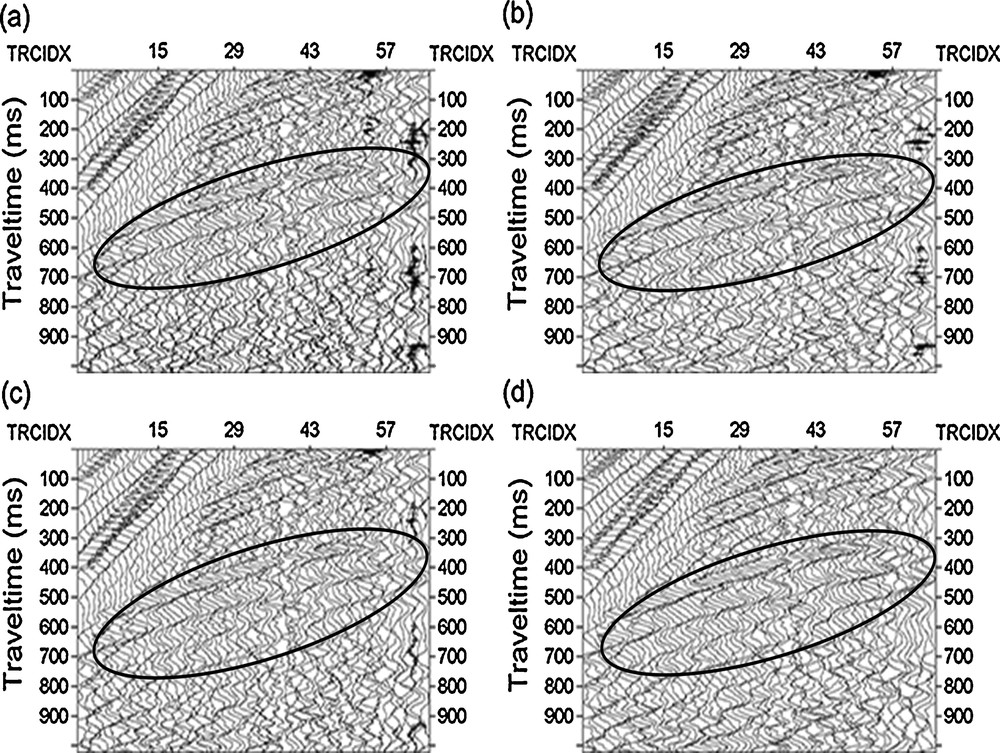
An enlarged view allowing seeing the quality of the filtering between the traces 6 to 69 and double travel times 701 to 1724 ms: (a) unfiltered seismic parts; (b) filtered seismic sections using wavelet transform filter; (c) filtered seismic sections using conventional TFPF; (d) filtered seismic sections using modified TFPF.
7 Conclusions
We have developed an effective time-frequency distribution to improve the denoising performance of TFPF on noisy seismic signals. The distribution follows a four-step working scheme, namely: (a) calculation of the pseudo Wigner–Ville distribution PWVD; (b) smoothing in time and frequency by means of a double window to obtain SPWVD; (c) use of a step function to remove divergent time-frequency points and to obtain ; (d) computation of the joint time-frequency distribution JTFD from PWVD weighted by . The usefulness of the modified time-frequency peak filtering based on JTFD has been checked with synthetic and real seismic data, and also contrasted with conventional TFPF and the wavelet-transform filtering method. The results demonstrate that modified TFPF gives smaller MSEs and higher quality signals recovered from noisy signals. The proposed method has a clear effect on suppressing random noise and can recover seismic events with a greater effectiveness. Therefore, the method presented here has wide applicability, especially in the case of noisy seismic signals with low signal-to-noise ratio.
Acknowledgments
We would like to thank the two reviewers for their constructive comments that helped us to greatly improve the early version of the manuscript. The National Natural Science Foundation of China (grants Nos. 41130421 and 41274118), the Science and Technology Development Program of Jilin Province of China (grant No. 20110362) and the Development and Reform Commission of Jilin Province (grants No. 2011006-2, JF2012C013-2) financially supported this research.