[Méthode de segmentation semi-automatique fondée sur une base réduite]
Nous présentons ici une méthode de segmentation d’imagerie médicale semi-automatique minimisant l’intervention d’un expert. A l’aide d’une base réduite, nous connaissons a priori la forme de l’objet à identifier sur les images, comme un muscle sur un scanner. Il suffit d’identifier les coefficients associés à l’objet d’intérêt dans la base réduite, via la résolution d’un système linéaire prenant en entrée les coordonnées de quelques points sélectionnés sur l’image. Un exemple implémenté en 2D est proposé. Cette méthode est indépendante des niveaux de gris de l’image, et peut donc être appliquée sur tous objets et toutes imageries.
This note adresses the following segmentation problem in medical imaging: minimize expert intervention for semi-automatic segmentation process. Using a reduced basis, we have an a priori knowledge of the objet we want to identify on the images, like a muscle on a CT-Scan. We just have to identify the coefficients associated to the object of interest in the reduced basis, by solving a linear system taking as input the coordinates of some selected points in the image. An example implemented in 2D is shown. This method is independent of the grayscale of the image, and can therefore be applied to all objects and images.
Révisé le :
Accepté le :
Publié le :
Damiano Lombardi 1 ; Yvon Maday 2 ; Lydie Uro 3
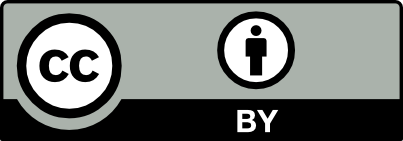
@article{CRMATH_2020__358_9-10_981_0, author = {Damiano Lombardi and Yvon Maday and Lydie Uro}, title = {Fast semi-automatic segmentation based on reduced basis}, journal = {Comptes Rendus. Math\'ematique}, pages = {981--987}, publisher = {Acad\'emie des sciences, Paris}, volume = {358}, number = {9-10}, year = {2020}, doi = {10.5802/crmath.89}, language = {en}, }
TY - JOUR AU - Damiano Lombardi AU - Yvon Maday AU - Lydie Uro TI - Fast semi-automatic segmentation based on reduced basis JO - Comptes Rendus. Mathématique PY - 2020 SP - 981 EP - 987 VL - 358 IS - 9-10 PB - Académie des sciences, Paris DO - 10.5802/crmath.89 LA - en ID - CRMATH_2020__358_9-10_981_0 ER -
Damiano Lombardi; Yvon Maday; Lydie Uro. Fast semi-automatic segmentation based on reduced basis. Comptes Rendus. Mathématique, Volume 358 (2020) no. 9-10, pp. 981-987. doi : 10.5802/crmath.89. https://comptes-rendus.academie-sciences.fr/mathematique/articles/10.5802/crmath.89/
[1] Multi-scale structured cnn with label consistency for brain mr image segmentation, Comput. Methods Biomech. Biomed. Eng. Imaging. Vis., Volume 6 (2018) no. 1, p. 113--117 | DOI
[2] Shape-aware surface reconstruction from sparse 3d point-clouds, Med. Image Anal., Volume 38 (2017), pp. 77-89 | DOI
[3] Deep neural networks for anatomical brain segmentation, Proceedings of the IEEE Conference on Computer Vision and Pattern Recognition Workshops (2015), pp. 20-28
[4] Statistical shape models for 3d medical image segmentation: a review, Med Image Anal, Volume 13 (2009) no. 4, pp. 543-563 | DOI
[5] Multi-atlas segmentation of biomedical images: a survey, Med Image Anal, Volume 24 (2015) no. 1, pp. 205-219 | DOI
[6] A new accurate and precise 3-d segmentation method for skeletal structures in volumetric ct data, IEEE transactions on medical imaging, Volume 22 (2003) no. 5, pp. 586-598 | DOI
[7] A survey on deep learning in medical image analysis, Med. Image Anal., Volume 42 (2017), pp. 60-88 | DOI
[8] Evaluation of methods for ridge and valley detection, IEEE Trans. Pattern Anal. Mach. Intell., Volume 21 (1999) no. 4, pp. 327-335 | DOI
[9] Current methods in medical image segmentation, Annu Rev Biomed Eng, Volume 2 (2000) no. 1, pp. 315-337 | DOI
[10] Current methods in medical image segmentation, Annu Rev Biomed Eng, Volume 2 (2000) no. 1, pp. 315-337 | DOI
[11] 3d bones segmentation based on ct images visualization, Biomedical Research, Volume 28 (2017) no. 8, pp. 3641-3644
[12] Thresholding based on histogram approximation, IEE Proceedings-Vision, Image and Signal Processing, Volume 142 (1995) no. 5, pp. 271-279 | DOI
[13] Automated medical image segmentation techniques, J. Med. Phys., Volume 35 (2010) no. 1, pp. 3-14 | DOI | Zbl
[14] Handbook of medical imaging, Academic Press, 2000
[15] Medical image segmentation: Methods and software, 2007 Joint Meeting of the 6th International Symposium on Noninvasive Functional Source Imaging of the Brain and Heart and the International Conference on Functional Biomedical Imaging, IEEE, 2007, pp. 140-143 | DOI
[16] Fast segmentation of bone in ct images using 3d adaptive thresholding, Computers in biology and medicine, Volume 40 (2010) no. 2, pp. 231-236 | DOI
Cité par Sources :
Commentaires - Politique