The present work aims at analyzing issues related to the data manifold dimensionality. The interest of the study is twofold: (i) first, when too many measurable variables are considered, manifold learning is expected to extract useless variables; (ii) second, and more important, the same technique, manifold learning, could be utilized for identifying the necessity of employing latent extra variables able to recover single-valued outputs. Both aspects are discussed in the modeling of materials and structural systems by using unsupervised manifold learning strategies.
Révisé le :
Accepté le :
Première publication :
Publié le :
Ruben Ibanez 1 ; Pierre Gilormini 1 ; Elias Cueto 2 ; Francisco Chinesta 1
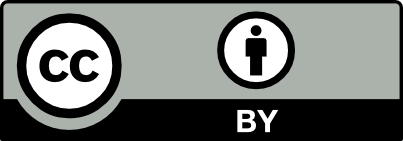
@article{CRMECA_2020__348_10-11_937_0, author = {Ruben Ibanez and Pierre Gilormini and Elias Cueto and Francisco Chinesta}, title = {Numerical experiments on unsupervised manifold learning applied to mechanical modeling of materials and structures}, journal = {Comptes Rendus. M\'ecanique}, pages = {937--958}, publisher = {Acad\'emie des sciences, Paris}, volume = {348}, number = {10-11}, year = {2020}, doi = {10.5802/crmeca.53}, language = {en}, }
TY - JOUR AU - Ruben Ibanez AU - Pierre Gilormini AU - Elias Cueto AU - Francisco Chinesta TI - Numerical experiments on unsupervised manifold learning applied to mechanical modeling of materials and structures JO - Comptes Rendus. Mécanique PY - 2020 SP - 937 EP - 958 VL - 348 IS - 10-11 PB - Académie des sciences, Paris DO - 10.5802/crmeca.53 LA - en ID - CRMECA_2020__348_10-11_937_0 ER -
%0 Journal Article %A Ruben Ibanez %A Pierre Gilormini %A Elias Cueto %A Francisco Chinesta %T Numerical experiments on unsupervised manifold learning applied to mechanical modeling of materials and structures %J Comptes Rendus. Mécanique %D 2020 %P 937-958 %V 348 %N 10-11 %I Académie des sciences, Paris %R 10.5802/crmeca.53 %G en %F CRMECA_2020__348_10-11_937_0
Ruben Ibanez; Pierre Gilormini; Elias Cueto; Francisco Chinesta. Numerical experiments on unsupervised manifold learning applied to mechanical modeling of materials and structures. Comptes Rendus. Mécanique, Contributions in mechanics of materials, Volume 348 (2020) no. 10-11, pp. 937-958. doi : 10.5802/crmeca.53. https://comptes-rendus.academie-sciences.fr/mecanique/articles/10.5802/crmeca.53/
[1] Data-driven computational mechanics, Comput. Methods Appl. Mech. Eng., Volume 304 (2016), pp. 81-101 | DOI | MR | Zbl
[2] A framework for data-driven analysis of materials under uncertainty: countering the curse of dimensionality, Comput. Methods Appl. Mech. Eng., Volume 320 (2017), pp. 633-667 | DOI | MR | Zbl
[3] Microstructural material database for self-consistent clustering analysis of elastoplastic strain softening materials, Comput. Methods Appl. Mech. Eng., Volume 330 (2018), pp. 547-577 | DOI | MR | Zbl
[4] Thermodynamically consistent data-driven computational mechanics, Contin. Mech. Thermodyn., Volume 31 (2019), pp. 239-253 | DOI | MR
[5] A manifold learning approach to data-driven computational elasticity and inelasticity, Arch. Comput. Methods Eng., Volume 25 (2018) no. 1, pp. 47-57 | DOI | MR | Zbl
[6] What-you-prescribe-is-what-you-get orthotropic hyperelasticity, Comput. Mech., Volume 53 (2014) no. 6, pp. 1279-1298 | DOI | MR | Zbl
[7] Data-driven computation for history-dependent materials, C. R. Méc., Volume 347 (2019) no. 11, pp. 831-844 | DOI
[8] Nonlinear Dimensionality Reduction, Springer, New York, 2007 | Zbl
[9] Visualizing data using t-SNE, J. Mach. Learn Res., Volume 9 (2008), pp. 2579-2605 | Zbl
[10] Nonlinear dimensionality reduction by locally linear embedding, Science, Volume 290 (2000) no. 5500, pp. 2323-2326 | DOI
[11] Dimension reduction by local principal component analysis, Neural Comput., Volume 9 (1997) no. 7, pp. 1493-1516 | DOI
[12] Principal manifolds and nonlinear dimensionality reduction via tangent space alignment, SIAM J. Sci. Comput., Volume 26 (2005) no. 1, pp. 313-338 | DOI | MR | Zbl
[13] An augmented reality platform for interactive aerodynamic design and analysis, Int. J. Numer. Methods Eng., Volume 120 (2019) no. 1, pp. 125-138 | DOI | MR
[14] kPCA-based parametric solutions within the PGD framework, Arch. Comput. Methods Eng., Volume 25 (2018) no. 1, pp. 69-86 | DOI | MR | Zbl
[15] A manifold learning approach for integrated computational materials engineering, Arch. Comput. Methods Eng., Volume 25 (2018) no. 1, pp. 59-68 | DOI | MR | Zbl
[16] On the effect of phase transition on the manifold dimensionality: application to the Ising model, Math. Mech. Complex Syst., Volume 6 (2018) no. 3, pp. 251-265 | DOI | MR | Zbl
- Conciliating accuracy and efficiency to empower engineering based on performance: a short journey, Comptes Rendus. Mécanique, Volume 351 (2024) no. S3, p. 121 | DOI:10.5802/crmeca.188
- Machine Learning in Computer Aided Engineering, Machine Learning in Modeling and Simulation (2023), p. 1 | DOI:10.1007/978-3-031-36644-4_1
- Empowering engineering with data, machine learning and artificial intelligence: a short introductive review, Advanced Modeling and Simulation in Engineering Sciences, Volume 9 (2022) no. 1 | DOI:10.1186/s40323-022-00234-8
- Data-driven elasto-(visco)-plasticity involving hidden state variables, Computer Methods in Applied Mechanics and Engineering, Volume 402 (2022), p. 115394 | DOI:10.1016/j.cma.2022.115394
- Material Forming Digital Twins: The Alliance between Physics-Based and Data-Driven Models, Key Engineering Materials, Volume 926 (2022), p. 3 | DOI:10.4028/p-234d4y
Cité par 5 documents. Sources : Crossref
Commentaires - Politique
Vous devez vous connecter pour continuer.
S'authentifier