1 Introduction
We approach here the difficult problem of querying data generated by diverse experimental approaches such as EST, SAGE and microarray. Expression data is stored in distinct locations using different platforms and with different conceptual organisation, and there is also frequent ambiguity in the meaning of basic terms used to describe the biological source of the material used for the experiment. A unification effort is underway and to assess the progress of this effort we apply eVOC within Ensembl (http://www.ensembl.org/Homo_sapiens/martview) to provide a standard for linking expression phenotype information with the genome sequence. A commercial application that links eVOC with other gene expression analysis tools is available from http://www.egenetics.com. In this paper we discuss the structure of eVOC so that we can describe how it can be accessed in an integrated view via EnsMart.
Biological ontologies aim to overcome the semantic heterogeneity commonly encountered in molecular biology databases, and to provide a common terminology for the description of a focussed aspect of biology. One such resource, TAMBIS [1] implements ontologies for both bioinformatics tasks and molecular biology to provide users with transparent access to multiple heterogeneous bioinformatics resources. Other ontologies focussing on specific aspects of biology include the Gene Ontology Consortium [2], which provides vocabularies that can be used to describe gene products in any organism, the EcoCyc ontology [3] which represents important metabolic and signal-transduction events in E. coli, and the MetaCyc [4] and KEGG [5] ontologies which describe aspects of the relationships between the chemical reactants, catalysts, substrates and products.
The annotation has been achieved by the assignment of terms from each of the four ontologies to the libraries. Initial assignment of terms to libraries was performed computationally, with curators who are domain experts performing assessment of annotation quality and further manual assignment. Where information was lacking in the library record, the original submitters were contacted where possible to provide more extensive information. Both the vocabularies and the vocabulary-annotated libraries can be retrieved from http://www.sanbi.ac.za/evoc/.
2 Methods and discussion
2.1 Development of a data structure for expression ontologies
The expression ontologies have been developed in four orthogonal knowledge domains: Anatomical System, Cell Type, Developmental Stage and Pathology. Anatomical System and Cell Type describe where a gene is expressed, Developmental Stage describes the timing of gene expression during development, and Pathology describes the disease state in which the gene is expressed. These four ontologies represent the vast majority of the expression data currently under classification.
The expression ontologies are independent pure hierarchies (or trees). In a pure hierarchy, each node has only one parent but may have multiple children. Each node is associated with one or more synonymous terms. For example, the terms ‘nasal’ and ‘nose’ are synonyms attached to a single node in the pathology ontology.
There is only a single type of relationship between the nodes in each hierarchy. For each ontology, the nature of the expression domain imposes an implicit type on the relationship between the nodes. For instance, in the ‘Anatomical System’ ontology, the relationships are of the ‘part-of’ type. In the ‘Cell Type’ and ‘Pathology’ ontologies, they are of the ‘subclass’ type, and in the ‘Developmental Stage’ ontology, the relationships are of the ‘is-a’ variety.
Pure hierarchies have a number of advantages over the more complex data structures often used to represent ontologies [6]. They are easy to maintain and expand and they can be visualised easily. Moreover, it is possible to construct a simple yet extremely powerful and flexible mechanism to query data across multiple hierarchies.
In cases where terms appear to have more than one parent, two options are available; migration to a directed acyclic graph (DAG), or untangling of the hierarchy to yield a pure hierarchy. In order to handle multi-parent terms and different parent-child relationships, the GO project [7] has implemented a DAG structure. During the development of the eVOC ontologies, and based on the available cDNA and SAGE libraries, we have found that where it appears that there is a need to represent multiple relationship types in one hierarchy, it is possible to untangle the hierarchy further by splitting it into separate hierarchies with more narrowly defined relationship types.
2.2 Development of the four expression ontologies
2.2.1 Anatomical System ontology
The controlled vocabulary for the description of the anatomical system or organ in which a gene is expressed is based on the controlled vocabulary used in the Computational Biology and Informatics Laboratory's (CBIL) databases (http://www.cbil.upenn.edu/anatomy.php3) but with modifications including the removal of all references to tissue type, cell type or developmental stage. Organisation of the Anatomical System hierarchies is systems-based. Examples of broad Anatomical Systems are ‘digestive system’ or ‘nervous system’, with more specific anatomical terms within these systems being ‘pancreatic islets’ or ‘retina’.
Future developments of eVOC will include the creation of an Anatomical Site ontology which will extend the current Anatomical System ontology by dividing anatomical parts according to their spatial position, rather than according to the system to which they belong. This is of particular value in describing libraries from spatially distinct anatomical sites containing multi-system anatomical sites. For example, ‘head’ is a distinct anatomical site, but includes both nervous and circulatory systems. The Anatomical System ontology contains 372 terms.
2.2.2 Cell Type ontology
The Cell Type ontology provides a fine-grained description of where a gene is expressed. It is a listing of human cell types extracted from Gray's Anatomy [8]. The Cell Type ontology includes 153 different cell types.
2.2.3 Developmental Stage ontology
The Developmental Stage ontology provides an ordered timeline of human development for the description of gene expression in temporal space. Examples of terms in the current hierarchy include ‘embryo’ and ‘adult’. Embryogenesis is further divided into the standard Carnegie stages (http://www.ana.ed.ac.uk/anatomy/database/humat/), which define the first two months of human development. Each of the major stages of development is further divided into appropriate weekly and yearly categories. The Developmental Stage ontology contains 132 distinct terms.
2.2.4 Pathology ontology
The Pathology ontology is loosely based on the World Health Organisation's ICD-9-CM (http://www.mcis.duke.edu/standards/termcode/icd9/1tabular.html). ICD-9-CM is designed for the classification of morbidity and mortality information for statistical purposes and for the indexing of hospital records by disease and surgical operations. We have implemented a modified version of the first two levels of this hierarchy, and have incorporated terms that are widely used in sample description, but which are not present in ICD-9-CM, e.g., Wilm's tumor. We have also removed terms which refer to systems, organs, tissues and cell types as these are already included in the Anatomical System and Cell Type ontologies. The Pathology ontology contains 141 terms.
2.3 Curation of the eVOC ontologies
Through a commercial-public partnership between Electric Genetics PTY Ltd (http://www.egenetics.com) and the Centre for Computational Biology at SANBI, we maintain a central, versioned database of eVOC ontologies which are updated, modified and released publicly by domain-experts. Commercial support addresses the needs of commercial research efforts through Electric Genetics.
Groups that choose to modify the ontologies for their own purposes are encouraged to contribute their modifications and corrections to the curators for inclusion. A mailing list: evoc@sanbi.ac.za has been established for this purpose.
2.4 Annotation of cDNA and SAGE libraries using eVOC
The expression data are independent of the ontologies presented here which are used to annotate them. We have annotated publicly available cDNA and SAGE libraries using these expression ontologies and made these available at http://www.sanbi.ac.za/vocab and http://www.ensembl.org/EnsMart/Integration/explorer.html. The eVOC ontologies are highly appropriate for the annotation of labelled target cDNAs for microarray experiments.
We have annotated 13144 human cDNA, 104 human SAGE libraries and 47 array expression states with the eVOC expression ontologies. These represent all the human cDNA and SAGE libraries that were publicly available in August 2003. The amount of information provided for each library varies widely. In some cases extensive information about the anatomical system, developmental stage and pathological state of the sample source is provided, while in other cases only a subset of this information is provided. The majority of the cDNA libraries (94.8%) have the information required for classification in the Anatomical System ontology, and most have information required for annotation with Pathology and Developmental Stage terms. Where the library could not be annotated, this was because the library information provided by submitters did not capture the relevant information. As a result of the fact that cDNA and SAGE libraries are largely derived from whole organs and tissues rather than from individual cell types, the majority of the libraries (94.2%) could not be annotated using the Cell Type ontology (data not shown).
2.5 Applications of the eVOC ontologies
2.5.1 Querying
A query for a particular term returns the node with which that term is associated, as well as all the nodes in the entire sub tree (branch) rooted at that node. For instance, a query for the term ‘neoplasia’ returns a particular node in the Pathology ontology, as well as all of its children, recursively. The next step in building a useful querying system lies in utilising the mappings from nodes to public databases (for example, cDNA libraries). In this way, a query for a particular term is translated first to a node, then expanded to a set of nodes, and then translated to a set of cDNA libraries. The set of libraries includes all the libraries associated with all the nodes in the branch rooted at the node which was originally associated with this node.
This simplistic query methodology can be the basis of an enormously powerful query infrastructure if the ability to perform basic set algebra (union and intersection) operations on the returned sets of cDNA libraries is used.
Consider, for instance, the query ‘liver AND neoplasia’. A query on ‘liver’ resolves to a node in the Anatomical System ontology, which in turn results in a set of cDNA libraries (all the libraries associated with the ‘liver’ node and all its sub nodes). Similarly, a query on ‘neoplasia’ returns the set of cDNA libraries associated with a sub tree of the Pathology ontology. The combined query – ‘liver AND neoplasia’ – returns the intersection of these two sets of cDNA libraries. In other words, it will return only libraries which were constructed from neoplastic liver samples.
2.5.2 Application of eVOC in ENSEMBL
EnsMart is a data retrieval tool that provides the ability to build queries on genome sequence and annotation present in the ENSEMBL genome database. Since ESTs have been mapped to the genome by ENSEMBL, eVOC terms can be linked transitively (via their parent clone library which is mapped to the eVOC ontologies) to the genomic sequence. As a result, users can perform expression-based queries in the context of genomic data and can extract transcripts and genes based on the location, state and timing of their expression. Similar mappings of array-based information have been performed using eVOC and are presented within EnsMart.
Using the EnsMart interface (http://www.ensembl.org/EnsMart/) a region (1–10 Mb) of chromosome 1 is queried for ‘liver AND neoplasia’ (Fig. 1). Of the 106 known genes listed in that region, 3 genes (ENSG 00000162568: unknown function, ENSG 00000157911: a peroxisome assembly protein, and ENSG 00000171603: a calsyntenin precursor) have both ‘liver’ and ‘neoplasia’ as terms. This does not mean that they are the only ones which are neoplastic in expression in the liver, but it does mean that only these genes have ESTs associated that have libraries that provide this information. A total of 86 other expression terms are also returned for the genes in question (Fig. 2). Other EnsMart filters allow a broad range of additional queries to modulate the list of possible genes.
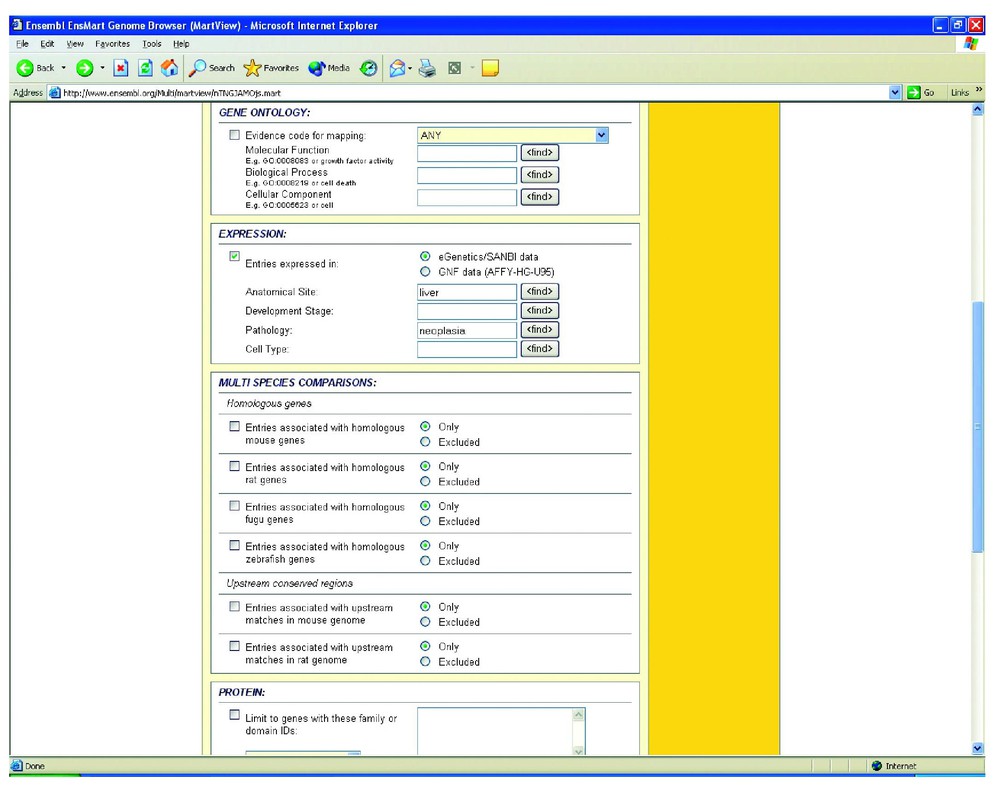
Selection of anatomical terms from the EnsMart pop-up view of eVOC terms.

Expression terms returned for liver AND Neoplasia query.
2.6 Worked application of eVOC within EnsMart
The application of this system (http://www.ensembl.org/Homo_sapiens/martview) has obvious implications in the determination/exclusion of candidates for genes in both complex and single locus diseases. The key for success is the availability of mapped ESTs and expression array information. Comprehensive EST to genome mappings have been available at ENSEMBL since March 2003.
A simple test of the implementation using known information on the expression of RP1, a gene that causes retinitis pigmentosa, demonstrates that a geneticist working with a region of the genome restricted to 8q11.23–8q12.1 (known mapped region of RP1 prior to its locus discovery) can return 10 genes in the region that are expressed in retina, providing a focus for further investigation [9].
The user can select the ‘type’ of gene that is desired from a list of genes. In reality this means that the user can select how well he/she believes the annotation. Genes range from those limited by ID's such as AFFY, LocusLink, HUGO, etc. We selected LocusLink for our example. We then selected the expression domain of interest. This is where eVOC had an impact, as both tissue array type data (such as that provided by the Genomics Institute of the Novartis Foundation: GNF) and EST data are mapped using the same vocabularies. However, the distribution of libraries can affect the utility of eVOC. GNF data for retina are not available. The user can select between these, and choose an expression from the drop down lists that appear when the relevant ‘find’ button for each ontology is selected. In this simple case, we select ‘retina’ under the anatomy ontology on SANBI/EG data. Presently, SNP and Interpro domains, and several other quantifiers could also be selected to further narrow the search.
The next page that appears provides the user with a ‘feature’ list from which to pick terms (Fig. 3). After a choice of relevant features and output format such as HTML, the next page is generated as a table. As we selected LocusLink genes in our exclusion process, only one gene is returned that has retinal expression ENSG00000120992 (LYSOPHOSPHOLIPASE I). For genetic research purposes, it is desirable to have a far broader net for identification of potential genes expressed in the diseased tissue. We therefore return through the menus and select a broader definition of gene types to include ‘Known Genes’. The subsequent search using eVOC gene expression categories for EST data reveals 10 genes. Interestingly the RP1 gene is included, and two library descriptions are returned with eVOC information. There are 10 genes out of the 40 genes in the region that have some form of retinal expression. What is of interest however, is that only two library descriptions were returned, one of which was from a cancer of breast tissue reflecting possible abnormal expression. No other gene in the 10 filtered genes returned had such restricted expression by tissue type. ENSEMBL accepts only ESTs for mapping that are of a certain ‘quality’ in terms of similarity cut-off. The standard thresholds are 90% for coverage and 97% for identity. A measure of the degree to which ESTs are mapped to a single locus is reflected in the cut-off of similarity required before an EST mapping can be accepted. This may exclude certain ESTs. All ESTs and mappings do exist however through eVOC as a resource, which is available separately as listed. Querying Unigene for expression of the RP1 genes shows that a total of 20 ESTs are listed, of which one is from breast cancer, one from melanoma and 4 are from muscle. The remainder are found in retina. ENSEMBL maps 1 507 628 unique human ESTs in the release current as of 9 August 2003. Updates to eVOC are to be released in parallel with ENSEMBL updates and so future mapping efforts should more closely reflect the actual availability of ESTs.
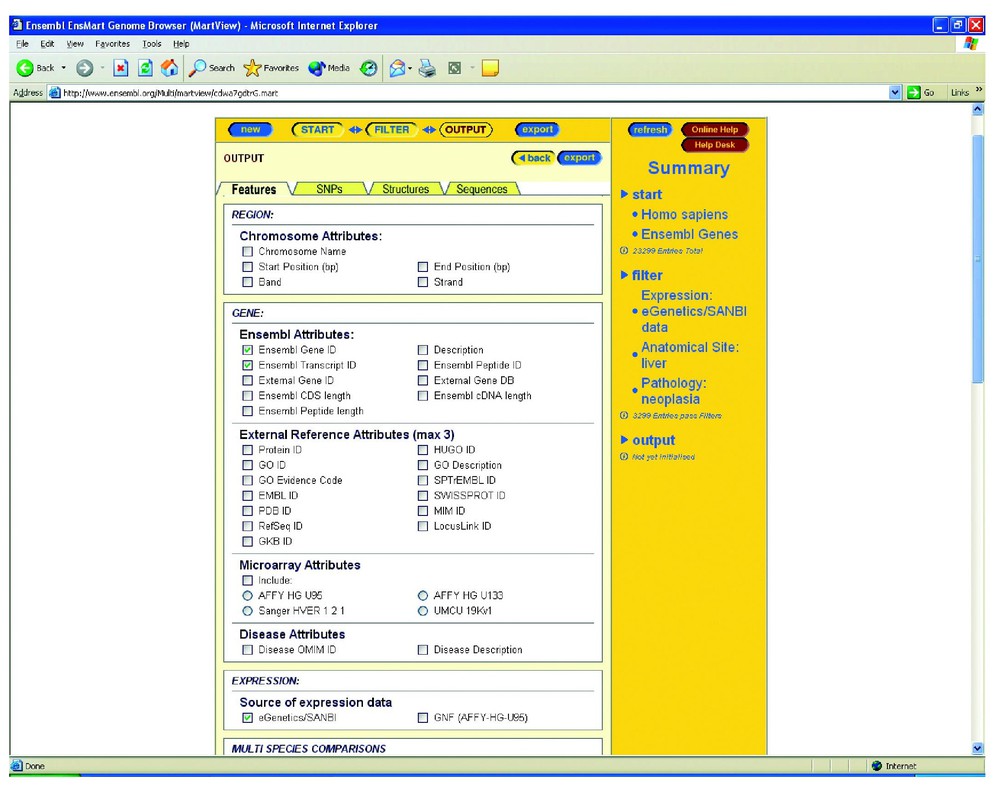
Features selection view for EnsMart selection of genes.
The principal limitation of eVOC is that currently the majority of its data is based upon EST libraries. The power of eVOC comes to the fore when data from SAGE, microarray and ESTs are combined. Such mappings are underway now for other broadly used datasets such as GNF Array data (AFFY-HG-U95), which is also mapped to the chromosomal information found in EnsMart. Only 47 expression states have so far been made available through the GNF project. However, as more array data is deposited, eVOC will curate these data, and provide the means to standardise terms between data sources.
2.7 Availability and interfaces for editing and graphical browsing
eVOC is provided under a BSD-style license and is available for download free of charge from http://www.sanbi.ac.za/evoc/ and can be used and modified without restriction. From the website users are also able to download the annotated datasets, join the Expression Vocabulary Consortium and sign-up to use the eVOC mailing list.
A graphical interface and integration system for eVOC has been developed by Electric Genetics and is available from info@egenetics.com. It provides users with the ability to view the ontologies, browse the hierarchical trees and to perform set operations on the annotated cDNA library data. Using this interface it is possible to obtain the list of cDNA libraries or ESTs returned by a query, or to provide a list of libraries or EST accessions and obtain the associated expression profile. The interface will be extended to include curation facilities, simplifying the users ability to modify the existing eVOC ontologies or create de novo ontologies of their own. In addition Electric Genetics has developed an API which provides the ability to develop custom software to interface eVOC with external data repositories, and to perform complex ontological queries on that data.
3 Conclusion
We have presented here application of a set of ontologies for the description of gene expression data, and have provided a database of the mappings between these ontologies and public cDNA and SAGE libraries under the EnsMart browser. These have been applied successfully in retrieving expression information about ESTs from ENSEMBL databases.
Acknowledgements
This work had financial support from the South African Government through the Department Science, and Technology initiated Innovation Fund Program, grant 32146 (W.H.) and the South African National Research Foundation (J.K.) and Wellcome Trust Collaborative Research Initiative Grant 006294/Z/01/Z (W.H. and M.M.) and the South African National Bioinformatics Network (W.H. and J.K.).