1 Introduction
Richard Lewontin's [1] review of the recent book by Fodor and Piattelli-Palmarini [2] neatly summarizes the predominant evolutionary paradigm, the ‘Modern Synthesis’.
“The modern skeletal formulation of evolution by natural selection consists of [several] principles that provide a purely mechanical basis for evolutionary change, stripped of its metaphorical elements:
- (1) The principle of variation: among individuals in a population there is variation in form, physiology, and behavior.
- (2) The principle of heredity: offspring resemble their parents more than they resemble unrelated individuals.
- (3) The principle of differential reproduction: in a given environment, some forms are more likely to survive and produce more offspring than other forms…
- (4) The principle of mutation: new heritable variation is constantly occurring.”
Tellingly, Lewontin asserts:
“The trouble with this outline is that… [t]here is an immense amount of biology that is missing.”
The synthesis itself, minus that immense amount of biology, has been formalized, and hence frozen, into the elaborate apparatus of mathematical population genetics that some find quite elegant [3]. But mathematical fashion – elegance, after all, is in the eye of the beholder – is not quite the same as science.
The omission of the role of embedding environment in the development of organisms (e.g., epigenetic effects such as heritable stress-induced gene methylation) and the omission of other interactions between organism and embedding environment (e.g., niche construction sensu Odling-Smee et al. [4]) severely limits the biological relevance of that synthesis. Here, following [5–7], we will describe genes, environment, and gene expression, in terms of information sources that interact and affect each other through a broadly coevolutionary crosstalk having quasi-stable ‘resilience’ modes in the sense of Holling [8,9].
This implies, among other things, that internal dynamics, for example the ‘large deviations’ described in [10], can trigger ecosystem shifts that, in turn, create selection pressure on organisms. The aerobic transition seems a most telling example. External factors may also trigger punctuated ecosystem shifts that can entrain organisms: volcanism, meteor strikes, ice ages, and the like.
But the story does not end with niche construction, epigenetics, or catastrophe.
Recently Sun and Caetano-Anolles [11] claimed evidence for deep evolutionary patterns embedded in tRNA phylogenies, calculated from trees reconstructed from analyses of data from several hundred tRNA molecules. They argue that an observed lack of correlation between ancestries of amino acid charging and encoding indicates the separate discoveries of these functions and reflects independent histories of recruitment. These histories were, in their view, probably curbed by co-options and important take-overs during early diversification of the living world. That is, disjoint evolutionary patterns were associated with evolution of amino acid specificity and codon identity, indicating that co-options and take-overs embedded perhaps in horizontal gene transfer affected differently the amino acid charging and codon identity functions. These results, they claim, support a strand symmetric ancient world in which tRNA had both a genetic and a functional role [12].
Clearly, ‘co-options’ and ‘take-overs’ are, perhaps, most easily explained as products of a prebiotic serial endosymbiosis, instantiated by a Red Queen between significantly, perhaps radically, different precursor chemical systems.
Witzany [13] also takes a broadly similar ‘language’ approach to the transfer of heritage information between different kinds of proto-organisms. In that paper he reviews a massive literature, arguing that not only rRNA, but also tRNA and the processing of the primary transcript into the pre-mRNA and the mature mRNA seem to be remnants of viral infection events that did not kill their host, but transferred phenotypic competences to their host and changed both the genetic identity of the host organism and the identity of the former infectious viral swarms. His ‘biocommunication’ viewpoint investigates both communication within and among cells, tissues, organs and organisms as sign-mediated interactions, and nucleotide sequences as code, that is, language-like text. Thus editing genetic text sequences requires, similar to the signaling codes between cells, tissues, and organs, biotic agents that are competent in correct sign use. Otherwise, neither communication processes nor nucleotide sequence generation or recombination can function. From his perspective, DNA is not only an information storing archive, but a life habitat for nucleic acid language-using RNA agents of viral or subviral descent able to carry out almost error-free editing of nucleotide sequences according to systematic rules of grammar and syntax.
Koonin et al. [14] and Vetsigian et al. [15] take a roughly similar tack, without, however, invoking biocommunication: Koonin et al. postulate a Virus World that has coexisted with cellular organisms from deep evolutionary time, and Vetsigian et al. suggest a long period of vesicle crosstalk symbiosis driving standardization of genetic codes across competing populations, leading to a ‘Darwinian transition’ representing path dependent lock-in of genetic codes.
In particular, before the lock-in of the precursor of the current genetic code [16–18], vesicle structure may have been rather more plastic than today, permitting analogs to gene transfer between quite different prebiotic organisms.
Synthesizing these considerations, we introduce a fifth Principle:
- (5) “The principle of environmental interaction: individuals and groups engage in powerful, often punctuated, dynamic mutual relations with their embedding environments that may include the exchange of heritage material between markedly different organisms.”
We begin with the reexpression of some familiar biological phenomena as information sources, leading to a formal mathematical structure that expresses these extensions in terms of familiar coevolutionary models.
2 Ecosystems as information sources
We first consider a simplistic (and distinctly unrealistic, since populations can become negative) picture of an elementary predator/prey ecosystem. Let X represents the appropriately scaled number of ‘predators’, Y the scaled number of ‘prey’, t the time, and ω a parameter defining their interaction. The model assumes that the ecologically dominant relation is an interaction between predator and prey, so that dX/dt = ωY and dY/dt = − ωX.
Thus the predator populations grows proportionately to the prey population, and the prey declines proportionately to the predator population.
After differentiating the first and using the second equation, we obtain the simple relation d2X/dt2 + ω2X = 0 having the solution ; . Thus
In the two dimensional phase space defined by X(t) and Y(t), the system traces out an endless, circular trajectory in time, representing the out-of-phase sinusoidal oscillations of the ‘predator’ and ‘prey’ populations.
Divide the X − Y phase space into two components – the simplest coarse-graining – calling the halfplane to the left of the vertical Y-axis A and that to the right B. This system, over units of the period 1/(2πω) traces out a stream of A's and B's having a single very precise grammar and syntax: ABABABAB ⋯
Many other such statements might be conceivable, e.g.,
More complex dynamical system models, incorporating diffusional drift around deterministic solutions, or even very elaborate systems of complicated stochastic differential equations, having various domains of attraction, that is, different sets of grammars, can be described by analogous symbolic dynamics ([19], Ch. 3).
Rather than taking symbolic dynamics as a simplification of more exact analytic or stochastic approaches, we generalize symbolic dynamics to a more comprehensive information dynamics. Ecosystems may not have identifiable sets of stochastic dynamic equations like noisy, nonlinear mechanical clocks, but, under appropriate coarse-graining, they may still have recognizable sets of grammar and syntax over the long-term: the turn-of-the seasons in a temperate climate, for many natural communities, looks remarkably the same year after year: the ice melts, the migrating birds return, the trees bud, the grass grows, plants and animals reproduce, high summer arrives, the foliage turns, the birds leave, frost, snow, the rivers freeze, and so on.
Suppose it possible to empirically characterize an ecosystem at a given time by observations of both habitat parameters such as temperature and rainfall, and numbers of various plant and animal species.
Traditionally, one can then calculate a cross-sectional species diversity index using a standard information or entropy metric [20]. This is not the approach to be taken here. Quite the contrary, in fact. Suppose it possible to coarse grain the ecosystem at time t according to some appropriate partition of the phase space in which each division Aj represents a particular range of numbers of each possible species in the ecosystem, along with associated parameters such as temperature, rainfall, and the like. What is of particular interest to our development is not cross-sectional structure, but rather longitudinal paths, that is, ecosystem statements of the form defined in terms of some natural time unit of the system. Thus n corresponds to an again appropriate characteristic time unit T, so that t = T, 2T,…,nT.
To reiterate, unlike the traditional use of information theory in ecology, the central interest is in the serial correlations along paths, and not at all in the cross-sectional entropy calculated for of a single element of a path.
Let N(n) be the number of possible paths of length n that are consistent with the underlying grammar and syntax of the appropriately coarsegrained ecosystem: spring leads to summer, autumn, winter, back to spring, etc., but never something of the form spring to autumn to summer to winter in a temperate ecosystem.
The fundamental assumptions are that – for this chosen coarse-graining – N(n), the number of possible grammatical paths, is much smaller than the total number of paths possible, and that, in the limit of (relatively) large n,
(1) |
This is a critical foundation to, and limitation on, the modeling strategy and its range of strict applicability, but is, in a sense, fairly general since it is independent of the details of the serial correlations along a path.
Again, these conditions are the essence of the parallel with parametric statistics. Systems for which the assumptions are not true will require special nonparametric approaches. We are inclined to believe, however, that, as for parametric statistical inference, the methodology will prove robust in that many systems will sufficiently fulfill the essential criteria.
This being said, not all possible ecosystem coarse-grainings are likely to work, and different such divisions, even when appropriate, might well lead to different descriptive quasi-languages for the ecosystem of interest. The example of Markov models is relevant. The essential Markov assumption is that the probability of a transition from one state at time T to another at time T + ΔT depends only on the state at T, and not at all on the history by which that state was reached. If changes within the interval of length ΔT are plastic, or path-dependent, then attempts to model the system as a Markov process within the natural interval ΔT will fail, even though the model works quite well for phenomena separated by natural intervals.
Thus empirical identification of relevant coarse-grainings for which this body of theory will work is clearly not trivial, and may, in fact, constitute the hard scientific core of the matter.
This is not, however, a new difficulty in ecosystem theory. Holling [9], for example, explores the linkage of ecosystems across scales, finding that mesoscale structures – what might correspond to the neighborhood in a human community – are ecological keystones in space, time, and population, which drive process and pattern at both smaller and larger scales and levels of organization.
Levin [21] argues that there is no single correct scale of observation: the insights from any investigation are contingent on the choice of scales. Pattern is neither a property of the system alone nor of the observer, but of an interaction between them. Pattern exists at all levels and at all scales, and recognition of this multiplicity of scales is fundamental to describing and understanding ecosystems. In his view there can be no ‘correct’ level of aggregation: we must recognize explicitly the multiplicity of scales within ecosystems, and develop a perspective that looks across scales and that builds on a multiplicity of models rather than seeking the single ‘correct’ one.
Given an appropriately chosen coarse-graining, whose selection in many cases will be the difficult and central trick of scientific art, suppose it possible to define joint and conditional probabilities for different ecosystem paths, having the form , such that appropriate joint and conditional Shannon uncertainties can be defined on them. For paths of length two these would be of the form
(2) |
The essential content of the Shannon-McMillan Theorem is that, for a large class of systems characterized as information sources, a kind of law-of-large numbers exists in the limit of very long paths, so that
(3) |
Taking the definitions of Shannon uncertainties as above, and arguing backwards from the latter two equations, it is indeed possible to recover the first, and divide the set of all possible temporal paths of our ecosystem into two subsets, one very small, containing the grammatically correct, and hence highly probable paths, that we will call ‘meaningful’, and a much larger set of vanishingly low probability [22].
Basic material on information theory can be found in any number of texts [22–24].
3 Genetic heritage as an information source
Adami et al. [25] make a case for reinterpreting the Darwinian transmission of genetic heritage in terms of a formal information process. They assert that genomic complexity can be identified with the amount of information a sequence stores about its environment: genetic complexity can be defined in a consistent information-theoretic manner. In their view, information cannot exist in a vacuum and must be instantiated. For biological systems information is instantiated, in part, by DNA. To some extent it is the blueprint of an organism and thus information about its own structure. More specifically, it is a blueprint of how to build an organism that can best survive in its native environment, and pass on that information to its progeny. Adami et al. assert that an organism's DNA thus is not only a ‘book’ about the organism, but also a book about the environment it lives in, including the species with which it co-evolves. They identify the complexity of genomes by the amount of information they encode about the world in which they have evolved.
Ofria et al. [26] continue in the same direction and argue that genomic complexity can be defined rigorously within standard information theory as the information the genome of an organism contains about its environment. From the point of view of information theory, it is convenient to view Darwinian evolution on the molecular level as a collection of information transmission channels, subject to a number of constraints. In these channels, they state, the organism's genome codes for the information (a message) to be transmitted from progenitor to offspring, subject to noise from an imperfect replication process and multiple sources of contingency. Information theory is concerned with analyzing the properties of such channels, how much information can be transmitted and how the rate of perfect information transmission of such a channel can be maximized.
Adami and Cerf [27] argue, using simple models of genetic structure, that the information content, or complexity, of a genomic string by itself (without referring to an environment) is a meaningless concept and a change in environment (catastrophic or otherwise) generally leads to a pathological reduction in complexity.
The transmission of genetic information is thus a contextual matter involving operation of an information source that, according to this perspective, must interact with embedding (ecosystem) structures. Such interaction is, as we show below, often highly punctuated, modulated by mesoscale ecosystem transitions via a generalization of the Baldwin effect akin to stochastic resonance, i.e., a ‘mesoscale resonance’ [5,6].
4 Gene expression as an information source
Wallace and Wallace [5,6], following the footsteps of [28,29], argue at some formal length that a ‘cognitive paradigm’ is needed to understand gene expression, much as Atlan and Cohen [30] invoke a cognitive paradigm for the immune system.
Cohen and Harel [28] assert that gene expression is a reactive system that calls our attention to its emergent properties, i.e., behaviors that, taken as a whole, are not expressed by any one of the lower scale components that comprise it. The essential point is that cellular processes react to both internal and external signals to produce diverse tissues internally, and diverse general phenotypes across various scales of space, time, and population, all from a single set or relatively narrow distribution of genes.
Chapter 1 of [7] provides detailed justification of a cognitive paradigm for gene expression that we will not repeat here.
The essential point, from the perspective of this paper, is that a broad class of cognitive phenomena can be characterized in terms of a dual information source that can interact with other such sources: Atlan and Cohen [30] argue that the essence of cognition is comparison of a perceived external signal with an internal, learned picture of the world, and then, upon that comparison, the choice of one response from a much larger repertoire of possible responses. Such reduction in uncertainty inherently carries information, and it is possible to make a very general model of this process as an information source [31].
Cognitive pattern recognition-and-selected response, as conceived here, proceeds by convoluting an incoming external ‘sensory’ signal with an internal ‘ongoing activity’ – which includes, but is not limited to, the learned picture of the world – and, at some point, triggering an appropriate action based on a decision that the pattern of sensory activity requires a response. It is not necessary to specify how the pattern recognition system is trained, and hence possible to adopt a weak model, regardless of learning paradigm, which can itself be more formally described by the Rate Distortion Theorem. Fulfilling Atlan and Cohen's criterion of meaning-from-response, we define a language's contextual meaning entirely in terms of system output.
The model, a simplification of the standard neural network, is as follows.
A pattern of ‘sensory’ input – incorporating feedback from the external world – is expressed as an ordered sequence y0, y1,…. This is mixed in a systematic (but unspecified) algorithmic manner with internal ‘ongoing’ activity, a sequence w0, w1,…, to create a path of composite signals x = a0, a1,…,an,…, where aj = f(yj,wj) for some function f. This path is then fed into a highly nonlinear, but otherwise similarly unspecified, decision oscillator generating an output h(x) that is an element of one of two (presumably) disjoint sets B0 and B1. We take .
Thus we permit a graded response, supposing that if h(x) ∈ B0 the pattern is not recognized, and if h(x) ∈ B1 the pattern is recognized and some action bj, k + 1 ≤ j ≤ m takes place.
This approach is broadly analogous to, but simpler than, the Hopfield/Hebb stochastic neuron in which series of inputs from m nearby neurons at time j is convoluted with ‘weights’ , using an inner product
In the terminology of this section, the m values constitute ‘sensory activity’ and the m weights the ‘ongoing activity’ at time j, with aj = yj · wj and x = a0, a1, …, an, …. A little more work leads to a fairly standard neural network model in which the network is trained by appropriately varying the w through least squares or other error minimization feedback.
The principal focus of the simpler model presented here is the composite paths x that trigger pattern recognition-and-response. That is, given a fixed initial state a0, such that , we examine all possible subsequent paths x beginning with a0 and leading to the event . Thus for all 0 ≤ j < m, but . Remember, the yj, the ‘sensory’ input convoluted with the internal wj, contains feedback from the external world, i.e., how well h matches intent with need.
For each positive integer n let N(n) be the number of grammatical and syntactic high probability paths of length n which begin with some particular a0 having and lead to the condition . We shall call such paths meaningful and assume N(n) to be considerably less than the number of all possible paths of length n – pattern recognition-and-response is comparatively rare. We – again – assume that the longitudinal finite limit both exists and is independent of the path x. We will – not surprisingly – call such a cognitive process ergodic.
Note that disjoint partition of state space may be possible according to sets of states which can be connected by meaningful paths from a particular base point, leading to a natural coset algebra of the system defining a groupoid. This is a matter of some importance pursued at length in [7].
It is thus possible to define an ergodic information source X associated with stochastic variates Xj having joint and conditional probabilities and such that appropriate joint and conditional Shannon uncertainties may be defined which satisfy the relations above.
This information source is taken as dual to the ergodic cognitive process.
Again, the Shannon-McMillan Theorem and its variants provide ‘laws of large numbers’ that permit definition of the Shannon uncertainties in terms of cross-sectional sums of the form , where the Pk constitutes a probability distribution.
Different quasi-languages will be defined by different divisions of the total universe of possible responses into various pairs of sets B0 and B1. Like the use of different distortion measures in the Rate Distortion Theorem, however, it seems obvious that the underlying dynamics will all be qualitatively similar.
Nonetheless, dividing the full set of possible responses into the sets B0 and B1 may itself require higher order cognitive decisions by another module or modules, suggesting the necessity of choice within a more or less broad set of possible quasi-languages. This would directly reflect the need to shift gears according to the different challenges faced by the organism or organic subsystem. A critical problem then becomes the choice of a normal zero-mode language among a very large set of possible languages representing accessible excited states. This is a fundamental matter that mirrors, for isolated cognitive systems, the resilience arguments applicable to more conventional ecosystems, that is, the possibility of more than one zero state to a cognitive system. Identification of an excited state as the zero mode becomes, then, a kind of generalized autoimmune disorder that can be triggered by linkage with external ecological information sources representing various kinds of structured stress.
In sum, meaningful paths – creating an inherent grammar and syntax – have been defined entirely in terms of system response, as Atlan and Cohen [30] propose.
5 Interacting information sources
Here we model the interaction of these information sources: embedding environment, genetic heritage (possibly across different organisms), and cognitive gene expression, using a formalism equivalent to that invoked both for nonequilibrium thermodynamics and traditional studies of coevolution [32]. That is, this is a straightforward coevolution model in which the interacting ‘populations’ are the information sources representing environment, genes, and cognitive gene expression.
Consider a set of information sources representing these three phenomena. There may be many different interacting sources of each kind.
Use inverse measures ℋj ≡ 1/Hj, j ≠ m as parameters for each of the other sources, writing
The dynamics of such a system is defined by a standard recursive network of stochastic differential equations, quite similar to those used to study many other highly parallel dynamic structures [33].
Letting the Kj and ℋm all be represented as parameters Qj, (with the caveat that Hm not depend on ℋm), one can define a ‘disorder’ measure analogous to entropy in nonequilibrium thermodynamics, following the arguments of [5–7], to obtain the standard recursive system of phenomenological ‘Onsager relations’ stochastic differential equations,
(4) |
The index m ranges over the interacting information sources and we could allow different kinds of ‘noise’ , having particular forms of quadratic variation which may, in fact, represent a projection of environmental factors under something like a rate distortion manifold [34].
This equation requires some further explanation. It's origin lies in the formal similarity between the expression for free energy density and information source uncertainty, explored in more detail in [5–7]. The argument is as follows:
Let F(K) be the free energy density of a physical system, K the normalized inverse temperature, V the volume and Z(K,V) the partition function defined from the Hamiltonian characterizing energy states Ei. Then
If a nonequilibrium physical system is parameterized by a set of variables {Qi}, then the empirical Onsager equations are defined in terms of the gradient of the entropy S ≡ F − ∑ jQjdF/dQj as
It is important to note that information systems are not locally reversible, so there is no analog to the ‘Onsager reciprocal relations’ Li,j = Lj,i.
There are several obvious possible dynamic patterns for the system of Eq. (4):
- 1. setting Eq. (4) equal to zero and solving for stationary points gives attractor states since the noise terms preclude unstable equilibria;
- 2. this system may converge to limit cycle or pseudorandom ‘strange attractor’ behaviors in which the system seems to chase its tail endlessly within a limited venue – the traditional Red Queen;
- 3. what is converged to in both cases is not a simple state or limit cycle of states. Rather it is an equivalence class, or set of them, of highly dynamic information sources coupled by mutual interaction through crosstalk. Thus ‘stability’ in this structure represents particular patterns of ongoing dynamics rather than some identifiable static configuration.
Here we become deeply enmeshed in a system of highly recursive phenomenological stochastic differential equations [35], but in a dynamic rather than static manner. The objects of this dynamical system are equivalence classes of information sources, rather than simple ‘stationary states’ of a dynamical or reactive chemical system. Imposition of necessary conditions from the asymptotic limit theorems of communication theory has beaten the mathematical thicket back one full layer.
These results are essentially similar to the work of Diekmann and Law [32], who invoke evolutionary game dynamics to obtain a first order canonical equation for coevolutionary systems having the form
(5) |
The si, with i = 1,…,N denote adaptive trait values in a community comprising N species. The are measures of fitness of individuals with trait values in the environment determined by the resident trait values s, and the Ki(s) are non-negative coefficients, possibly distinct for each species, that scale the rate of evolutionary change. Adaptive dynamics of this kind have frequently been postulated, based either on the notion of a hill-climbing process on an adaptive landscape or some other sort of plausibility argument.
When this equation is set equal to zero, so there is no time dependence, one obtains what are characterized as ‘evolutionary singularities’ or stationary points.
Diekmann and Law contend that their formal derivation of this equation satisfies four critical requirements:
- 1. the evolutionary process needs to be considered in a coevolutionary context;
- 2. a proper mathematical theory of evolution should be dynamical;
- 3. the coevolutionary dynamics ought to be underpinned by a microscopic theory;
- 4. the evolutionary process has important stochastic elements.
Equation (4) above is exactly similar, although focused on information sources representing environment, genetic heritage and cognitive gene expression, allowing elaborate patterns of phase transition punctuation in a natural manner [7].
Champagnat et al. [36], in fact, derive a higher order canonical approximation extending equation (5) that is closer to Eq. (4), i.e., a stochastic differential equation describing coevolutionary dynamics. Champagnat et al. go even further, using a large deviations argument to analyze dynamical coevolutionary paths, not merely evolutionary singularities. They contend that in general, the issue of evolutionary dynamics drifting away from trajectories predicted by the canonical equation can be investigated by considering the asymptotic of the probability of ‘rare events’ for the sample paths of the diffusion.
By ‘rare events’ they mean diffusion paths drifting far away from the canonical equation. The probability of such rare events is governed by a large deviation principle: when a critical parameter (designated ɛ) goes to zero, the probability that the sample path of the diffusion is close to a given rare path ϕ decreases exponentially to 0 with rate I(ϕ), where the ‘rate function’ I can be expressed in terms of the parameters of the diffusion. This result, in their view, can be used to study long-time behavior of the diffusion process when there are multiple attractive evolutionary singularities. Under proper conditions the most likely path followed by the diffusion when exiting a basin of attraction is the one minimizing the rate function I over all the appropriate trajectories. The time needed to exit the basin is of the order exp(H/ɛ) where H is a quasi-potential representing the minimum of the rate function I over all possible trajectories.
An essential fact of large deviations theory is that the rate function I which Champagnat et al. invoke can almost always be expressed as a kind of entropy, that is, in the form
These considerations lead very much in the direction of Eq. (4) above, but now seen as subject to internally-driven large deviations that are themselves described as information sources, providing ℋ parameters that can trigger punctuated shifts between quasi-stable modes, in addition to resilience transitions driven by ‘catastrophic’ external events or the exchange of heritage information between different classes of organisms.
Equation (4) provides a very general statistical model that combines Principle (5) – in concert with the possibility of large deviations – with earlier theory.
Indeed, the direct inclusion of large deviations regularities within the context of the statistical model of Eq. (4) suggests that other factors that can be characterized in terms of information sources may be directly included within the formalism. Section 6.1 of [7], for example, explores the impact of culture, taken as a generalized language, on the evolution of human pathogens. The methodology thus provides a straightforward means of incorporating the evolutionary effects of animal traditions, as described by Avital and Jablonka [38].
The basic statistical model is illustrated by Fig. 1, for a ‘fast’ time, ‘small’ scale process. Here, two quasi-equilibria are characterized by diffusive drift about their singularities in a two-dimensional system, but are coupled by a highly structured large deviation connecting them. The pattern most obviously encompasses the punctuated equilibrium of Eldredge and Gould [39,40].
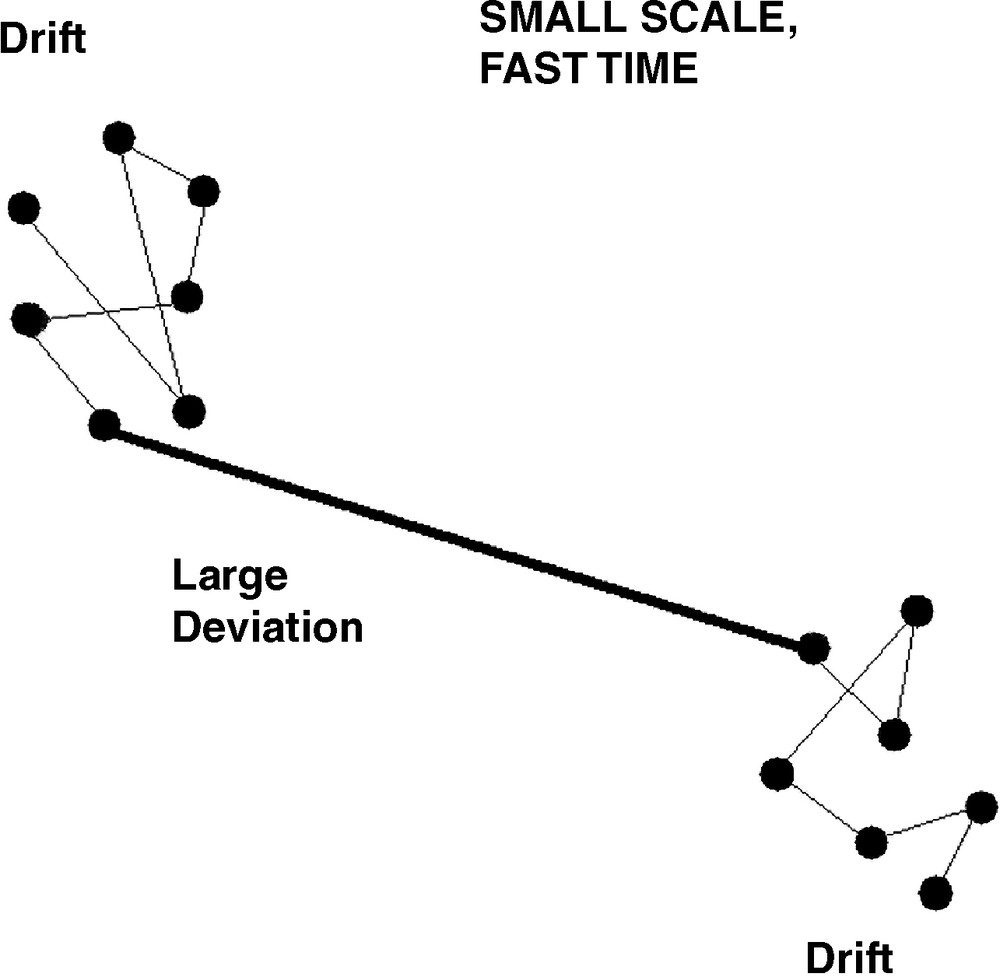
‘Small’ scale, ‘fast’ time behavior of the system obtained by setting Eq. (4) to zero. Diffusive drift about a quasi-equilibrium is interrupted by a highly structured large deviation leading to another quasi-equilibrium, in the pattern of punctuated equilibrium of Eldredge and Gould.
Fig. 2 expands the system of Fig. 1 to ‘slow’ time, ‘large’ scale, so that the large deviations of Fig. 1 are seen as, in essence, variation about a single ‘larger’ singularity. This latter structure could account for the observations of Gomez et al. [41] who show that, as with other niche components, ecological interactions are evolutionarily conserved, suggesting a shared pattern in the organization of biological systems through evolutionary time that is particularly mediated by marked conservation of ecological interactions among taxa.
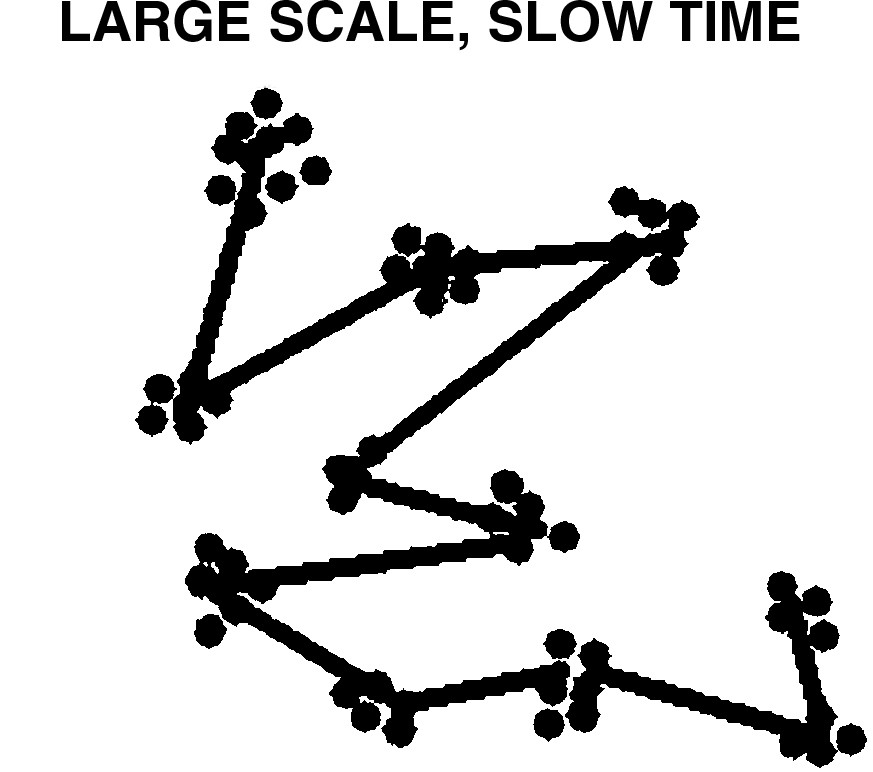
‘Large’ scale, ‘slow’ time enlargement of Fig. 1, showing the conservation of ecological interactions across deep evolutionary time as variation about a single, larger, singularity.
6 Conclusions
We have reexpressed ecosystem dynamics, genetic heritage, and (cognitive) gene expression producing phenotypes that interact with the embedding ecosystem, all in terms of interacting information sources. This instantiates Principle (5) of the Introduction, producing a system of stochastic differential equations closely analogous to those used to describe more traditional coevolutionary phenomena, subject to punctuated resilience shifts driven both by internal large deviations and large-scale external perturbations.
That is, environments affect living things, and living things affect their environments: Cyanobacteria created the aerobic transition, greatly changing the very atmosphere of the planet. Organisms can, more locally, engage in niche construction that changes the local environment as profoundly. Environments select phenotypes that, in a sense, select environments. Genes record the result, as does the embedding landscape. The system co-evolves as a unit, with sudden, complicated transitions between the quasi-equilibria of Eq. (4).
To reiterate, these transitions can be driven by internal ‘large deviation’ dynamics, as the aerobic transition, or by external events, volcanic eruptions or meteor strikes, and so on. Ecosystem resilience shifts entrain the evolution of individual organisms that, in turn, drive ecosystem resilience transitions.
Enlargement of scale, however, can produce a model of the conservation of ecological interactions across the tree of life.
Thus the introduction of Principle (5) to the Modern Synthesis generates the complex system of Eq. (4), perhaps best characterized by the term ‘evolution of ecosystems’. The essential point is that the Modern Synthesis now requires modernizing, recognizing the importance and ubiquity of a mutual interaction with the embedding ecosystem that includes the possibility of the exchange of heritage information between different classes of organisms.
Here we have, in the arguments leading to Eq. (4), outlined a ‘natural’ means for implementing such a program, based on the asymptotic limit theorems of communication theory that provides necessary conditions constraining the dynamics of all systems producing or exchanging information, in the same sense that the Central Limit Theorem provides constraints on systems that involve sums of stochastic variates. That is, we provide the basis for a new set of statistical tools useful in the study of ecological and evolutionary phenomena. Statistics, however, is not science, and the fundamental problems of data acquisition, ordination, and interpretation remain.
Acknowledgments
The author thanks a reviewer for perceptive comments useful in revision.