1 Introduction
1.1 Endocrine disruption research: a multidisciplinary field
Although research on substances now identified as endocrine disruptors (EDs) already existed in the mid-20th Century, the terminology “endocrine disruptors” was first used in the scientific literature in 1993 [1]. EDs are defined by the World Health Organisation as “an exogenous substance or mixture that alters function(s) of the endocrine system and consequently causes adverse health effects in an intact organism, or its progeny, or (sub) populations” [2]. Compared to other dangers for health, a specificity of this definition is the combination of the existence of adverse effects and of information on the mechanism of induction of these effects. Other dangers are generally either defined in terms of adverse effects (e.g., carcinogens) or in terms of mechanism (e.g., mutagenic substances, but not carcinogens). This specificity highlights the need for research on EDs to work both at the scale of organisms (or populations), which is most convenient to highlight adverse health effects (what toxicology, ecotoxicology, ecology, demography and epidemiology typically do) and at finer scales, which is usually required to identify biological mechanisms. Note that (eco)toxicology and epidemiology are now increasingly able to help identifying biological mechanisms [3,4].
1.2 What molecular and (eco)toxicological studies can tell us about EDs
Historically, ED research developed from a variety of disciplines including ecotoxicology, toxicology, molecular biology, epidemiology, and clinical research. Studies relying on molecular, cellular, organ and animal models as well as wildlife observations brought crucial findings about EDs. (i) At the molecular and cellular levels, structural and cell-based assays identified exogenous substances with affinity for key molecular components of the endocrine system such as nuclear receptors and enzymes implied in hormone metabolism or synthesis [5]. (ii) At the level of organs, studies finely documented the impact and mechanisms of action of chemicals on the endocrine system [6]. (iii) At the level of organisms, toxicological experiments described the toxicokinetics of the suspected EDs and their biological and health effects (including development, fecundity, metabolic disorders and behaviour) over large ranges of doses, alone or in mixtures, during specific developmental windows, over up to several generations [7–9]. (iv) At the ecosystem level, ecotoxicological research has identified EDs in the environment and described their impact on wildlife (e.g., the decrease in bird populations in the US Great lakes due to organochlorine exposures [10], or imposex in sea molluscs because of tributyltin (TBT) exposure [11]).
1.3 What molecular and (eco)toxicological studies cannot address efficiently
Although the above-mentioned issues are central, specific questions important for research on EDs, for risk assessment and to inform risk management cannot be efficiently tackled with the above-mentioned approaches. These include the actual exposure patterns and levels in humans [12]; the shape and slope of dose-response functions in humans [13]; the health impact (risk, or disease cases attributable to exposure to EDs) and the related economic cost for society [14]; the existence of possible synergy between EDs and specific lifestyle factors; the efficiency of specific prevention measures on human populations (e.g., informing the public, modifying one's diet…) [15].
These questions can be specifically addressed by (human) population-based approaches such as those of epidemiology and closely-related disciplines. Historically, for example, these approaches have been central to document the diethylstilboestrol [16], and Minamata [17] crises. Other important questions, related, e.g., to the decision-making processes regarding substances with endocrine-disrupting properties, are within the scope of social sciences and will not be discussed here.
1.4 The epidemiological approach
Epidemiology can be defined as a science aiming at studying patterns and causes of diseases in human populations, and at identifying approaches to limit disease incidence. We make no distinction between epidemiology and clinical research on patients, which we consider to be the application of epidemiology to a specific population and possibly specific “exposures”, such as drugs. Although generally of observational nature, epidemiologists have for a long time also used experimental and quasi-experimental approaches (see 2 below).
In this article, we will discuss some of the main challenges of epidemiological research, focusing on control for confounding bias (section 2), study design (section 3), and exposure misclassification (section 4), illustrating to which extent epidemiological research on EDs raises specific issues in each of these areas.
2 Confounding: nature, consequences and control
2.1 Confounding: Nature and consequences
Substances acting on the EDs are suspected to influence the risk of various diseases such as decreased fecundity, congenital anomalies, overweight, type II diabetes, specific cancers [2]… All of these conditions are multifactorial at the population level, which means that risk factors other than the EDs under study also exist. In an observational setting such as that of most epidemiological studies, the distribution of some of these disease risk factors may differ across the categories of exposure to the considered ED; for example, the proportion of subjects with a “poor” diet may be higher in the highly exposed population, compared to the less exposed one. Consequently, the variations in disease risk across exposure groups can differ compared to what would be observed if the exposure groups were a priori identical in terms of distribution of disease risk factors—what is termed confounding, or confounding bias. Confounding can be seen as resulting from the compared populations not being exchangeable in terms of disease risk factors other than the exposure of interest. This is different in a toxicological study, in which exposure is randomized (and in which high homogeneity of the test animals also minimizes the potential for confounding, compared to human studies), or in randomized control trials. In these approaches, in expectation, the distribution of risk factors is similar across exposure groups because exposure is drawn at random among study subjects.
Strictly speaking, confounding is defined in epidemiology as the distortion of an association between two parameters (typically, the exposure of interest and a health outcome) by an extraneous factor. Extraneous is used here to exclude the role of factors belonging to the causal chain between the exposure and the health outcome, which are termed intermediate factors (Fig. 1). Confounding can in theory induce a spurious statistical association between exposure and disease, modify the magnitude, or direction of any association, or mask an existing (causal) association.

The epidemiological approach. Epidemiological studies typically aim at assessing the possible effect of an exposure on a given biological parameter or disease, possibly considering the role of specific intermediate mechanisms and controlling for confounders.
In the specific case of EDs, for many compounds, exposure is ubiquitous and occurs through cosmetics (e.g., phthalates, and parabens), food (phthalates, bisphenols, metals, and persistent organic pollutants), so that confounding by dietary and behavioural factors needs to be considered. For example, in a study of the effects of in-utero exposure to perfluorinated compounds on body weight in childhood, participants with the highest in-utero exposure (perfluorinated compounds assessed from maternal blood) may include a larger proportion of families in which the mother has a high intake of packaged processed foods such as fast food (which are sometimes wrapped in paper coated by perfluorinated compounds) than participants with lower exposure. Their children may in the following years also more frequently consume more processed foods than the least exposed children, a behaviour that may increase the risk of obesity for dietary reasons such as higher fat in processed foods. Consequently, children most exposed to perfluorinated compounds in utero would more often be overweight later on for a reason due to a diet higher in processed foods and not because of a causal effect of perfluorinated compounds (Fig. 2). In other situations, confounding could mask an existing causal association; for example, mercury exposure can impact the central nervous system of children, but an important source of mercury in Western populations is fish consumption, which is also a source of poly-unsaturated fatty acids, which are beneficial for the developing brain, thus attenuating any effect of ED exposure.
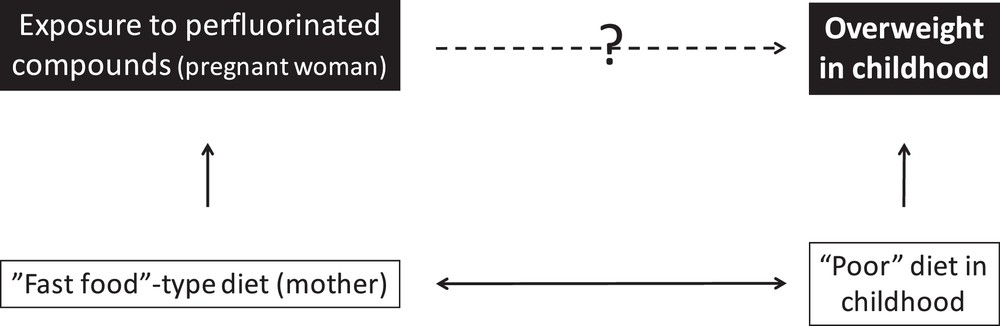
A theoretical situation leading to confounding: in an observational study of effects of perfluorinated compounds (PFC) in-utero exposure on overweight in childhood, the association may be confounded by the maternal and child diets (high intake of fast food, a source of exposure to PFCs which are sometimes used to coat wrapping paper), which needs to be controlled for.
We will now discuss how such bias can be corrected.
2.2 Approaches used to correct for confounding at the stage of study design
The possible impact of potential confounding factors can be limited at the stage of the study design. Generally, the approaches used at this stage can be grouped as either limiting the variability in the potential confounder or as avoiding/cancelling any association between exposure and the confounder.
The first situation (which is also what happens in toxicological studies, in which very homogeneous strains of animals with similar behaviours are generally used) can be obtained by restricting to a subgroup of the population in which the potential confounder (e.g., smoking, socio-economic status, or body weight) varies little or does not vary at all. For example, one could only include non-smokers or persons with a body mass index in a specific range. Several birth cohorts implying a high participation burden are de facto restricted to highly educated populations. By implying to focus on a population more homogeneous in terms of dietary behaviours or possible occupational exposures, restriction limits the potential for confounding. Restriction will also minimize representativeness, but representativeness is not a requirement in etiologic studies [18]. A possible drawback is that the variability in exposure will consequently be reduced, which is a price to pay for the limitation of the confounding bias—this is a manifestation of the bias-variance trade-off [19].
In time series analyses (and more generally before/after within-community comparisons), short-term variations in disease occurrence (e.g., hospital admissions for a specific cause) are related to short-term variations in exposures; the unit of observation is the community on a given day, which is compared to the same community on another (close) day; since many behavioural (e.g., dietary habits), health-related (e.g., obesity rate) or genetic factors are unlikely to change markedly at the population level within such short time periods, confounding by such factors is avoided by design. Only acutely varying factors, such as epidemics or meteorological conditions, remain a potential source of confounding, and need to be controlled for statistically at the analysis stage, e.g., by adjustment in regression models.
The second situation, that of avoidance of any association between a potential confounder and the exposure, can be obtained in experimental (or quasi-experimental) approaches. In this setting, the exposure is not expected to be associated with any other factor (including lifestyle, environmental or genetic characteristics), making confounding by any factor unlikely. Note that prior identification or measurement of the potential confounders is not required. Indeed, the randomization of exposure guarantees that, in expectation, the exposure is independent of any other factor. Another way to get rid of any association between exposure and individual characteristics is to perform within-person comparisons (that is, to observe the health parameter of interest in each subject under at least two exposure conditions), which corresponds to having the same person to be his/her own control. Note that this approach only makes sense if the exposure has short-term effects. This has been for example done in the case of Mesalamine And Reproductive health Study (MARS), a prospective crossover-crossback study, which aimed at determining whether high exposure to dibutyl phthalate (DBP) from coatings in medications impacts measures of male reproductive health. Some mesalamine medications used to treat inflammatory bowel disease have DBP in their coating, whereas other mesalamine formulations do not. Taking advantage of differences in mesalamine formulations, men taking non-DBP containing mesalamine at baseline (corresponding to background DBP exposure) crossed-over for four months to high-DBP mesalamine medications, and then crossed-back for four months to their non-DBP mesalamine, and vice versa for men taking high-DBP mesalamine at baseline. Men provided up to six semen and blood samples. This allowed exploring the effects of DBP from mesalamine, by design controlling for fixed (non-time varying) within-person confounders, both measured and unmeasured, and thus avoiding the random variability and confounding that would have occurred in a purely cross-sectional analysis comparing men taking a high-DBP medication to other men taking non-DBP medication [20]. If in a crossover study the order in which each exposure level (e.g., exposed versus unexposed) is assigned is drawn at random, then this corresponds to a randomized crossover design. As an example, a randomized crossover study reported an increase in blood pressure following bisphenol A exposure from drinking canned beverages [21]. Such experimental approaches may in some circumstances and population not be judged ethically acceptable in humans for potentially harmful exposures; an option to avoid this issue is to attempt randomizing a decrease in exposure, starting from a group of “naturally” exposed subjects.
Many other approaches, including Mendelian randomization and the reliance on instrumental variables, are also possible [22]; in the rest of this section, we will only present statistical approaches.
2.3 Approaches used to correct for confounding at the stage of study analysis
Various statistical approaches have been developed to limit confounding. Simple (and efficient) approaches include statistical restriction to a subgroup in which the potential confounder does not vary (e.g., non-smokers), stratification (according to the different values of the confounder, e.g., non-smokers versus smokers), or standardisation on the potential confounder. These approaches are generally limited by the number of confounders that can be simultaneously handled, a limitation that is waived to some extent by statistical adjustment in regression models. Regression models allow us to adjust for a large number of covariates (typically, up to about one tenth of the number of observations, in the case of linear regression), and are very flexible in terms of assumptions on the shape of the dose-response function (e.g., using spline models [23]) and of statistical interactions between exposures, or between exposures and individual characteristics.
In the case when a confounder is assessed but poorly measured or coded in an inappropriate way in the statistical model, bias may remain (a situation termed residual confounding [23]). Propensity-score-based methods constitute an increasingly used alternative (or complement) to adjustment. These consist in building a score based on covariates (e.g., sociodemographic or behavioural factors) associated with the level (or probability for binary exposures, hence the word propensity) of exposure. This score can then be used to reweight the population, by assigning to each person a weight roughly inversely proportional to his probability of being exposed, thus rebuilding a fictitious population in which the observed potential confounders have similar distributions in the exposed and non-exposed persons (or across exposure levels). Propensity-score weighting can be seen as a generalisation of standardisation allowing one to account for many potential confounders simultaneously. This approach has been used for example in a study of the effects of analgesic use during pregnancy on the risk of undescended testes at birth, to make women not using analgesics similar to those using analgesics with respect to potential risk factors of undescended testes [24]. Under the assumption of lack of unidentified confounders, propensity-score weighting provides a causal estimate of the association of exposure with the risk of occurrence of the health outcome, that is, an estimate of the risk difference between an exposed population and the same population without exposure [25].
In spite of their great flexibility, statistical adjustment and propensity scores are limited by the underlying assumptions of absence of unmeasured confounders, and also of lack of misclassification in the assessment of covariates. While there are approaches to tackle the second issue, the former cannot be handled directly. It should however be noted that the fact that the validity of the study results is conditional upon the existing current knowledge and a priori hypotheses also generally holds for many other scientific disciplines. For example, the validity of results from a cellular model aiming at testing the effect of a specific ED relies on the existing knowledge about how this particular ED is metabolized in intact organisms, so that the toxicologically-relevant by-products of the considered ED can be tested.
2.4 “Correlation is not causation”
The expression “correlation is not causation” is sometimes used in relation to epidemiological and other types of observational approaches, to guard against inferring causality from such observational studies. This calls for several comments: First, since a coefficient of correlation only implies two factors, the word “correlation” tends to imply that there is no control for extraneous factors possibly influencing the disease risk. This does not correspond to the standard epidemiological practice: epidemiological studies do not rely on correlations, but generally on the estimation of adjusted associations derived from models and approaches considering tens or hundreds of factors simultaneously, correcting for potential confounding by design-related and statistical approaches, as described above. These models are usually developed with strong a priori biological and toxicological hypotheses. Stating that epidemiology relies on “correlations”, in addition of being wrong statistically, overemphasizes the statistical component of environmental epidemiology, ignoring its biological and toxicological basis, and is just as simplistic as considering that toxicology is based on Chi2 tests because authors report the differences in the proportions of sick animals between the treated and control groups. Statistics are just one of the tools used by either discipline; epidemiology is, because of its observational design, more prone to confounding bias and relies heavily on a statistical control for confounding, while toxicology mainly relies on the selection of a homogeneous population and randomization to remove confounding, and is more prone to issues related to the validity of the model and species chosen, and to the complexity of rigorously establishing the relevance of the findings for the human species.
Second, there is now a whole branch of epidemiology and biostatistics (and methodologically related disciplines, such as economics) that rely on specific statistical approaches (such as the above-mentioned propensity score weighting) to estimate causal effects, understood as the difference (or ratio) in disease risk between an exposed population, and the risk that would be observed if this very same population had not been exposed at all, all other extraneous factors remaining identical [25].
Third, causation is not attainable by a single epidemiological study without additional assumptions, if only because of random fluctuations; but such is also the case for a toxicological study (if one is interested in effects in humans). Some scientists and philosophers of science, such as Russel, even argue that there is no scientific concept of causation [26]. Indeed, in a Popperian perspective, science is not about establishing causes, but about building refutable theories; it can thus be argued that causality (or, at least, an evaluation of the “level of proof”) is not so much the work of science as that of expert groups. One could consider that it is at the stage of literature synthesis that experts can appraise the level of evidence by considering and, if possible, integrating the results from molecular biology, toxicology, epidemiology and all other relevant sources. Stating about epidemiological studies that ``correlation is not causation'' is thus both missing the complex nature of the epidemiological approach, which integrates knowledge from biology, toxicology, data sciences… and is not a simple correlation analysis, and also ignoring the way theories are developed in the field of environmental health sciences.
Overall, each discipline has its own limitations and strengths as regards the construction of theories in the field of environmental health; each one has developed approaches to fight these limitations, and we see no scientific rationale for considering that one would be superior to other disciplines in terms of level of evidence it brings; rather, close disciplines such as toxicology and epidemiology should be seen as co-constructing the theories of the environmental health field.
3 Main types of epidemiological approaches
One convenient way to classify epidemiological studies in terms of design is to distinguish them according to whether the exposure is controlled (as in randomized trials) or not, and according to the unit of observation. The unit of observation can either be a person (cohorts, case-controls and related designs), a community (ecological studies, relying either on temporal contrasts in exposure, as is the case for times-series analyses, or on spatial contrasts), or a study (literature reviews, meta-analyses, Fig. 3). One could also include in this latter category health impact assessment studies. We will here only briefly present cohort and health impact assessment studies. Examples of experimental or quasi-experimental studies [20,21] have been given above in 2.2.
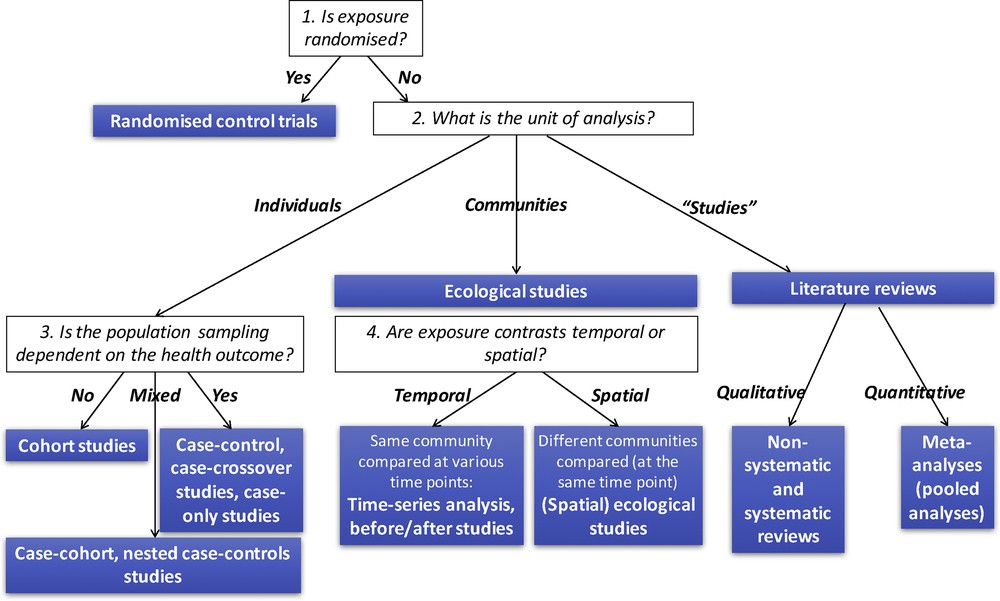
Overview of the main epidemiological study designs.
3.1 Cohort studies
Cohorts are defined by a follow-up of persons initially without the health event of interest in whom exposure is characterized at inclusion, and possibly later on. This follow-up could be prospective or retrospective, although most often study participants are identified and followed up prospectively. Randomized control trials can be seen as cohorts in which exposure is randomized. The wordings “panel study” and “longitudinal study” are generally used as synonymous for cohort. A particularly important feature of cohorts is the characterization of exposure at inclusion, which can permit us to characterize exposure in the toxicologically-relevant time window. We will here focus on cohorts allowing characterization of early-life exposures, so-called “birth” or pregnancy cohorts, which are of particular relevance in the context of EDs research.
Historically, the first birth cohorts were characterized by a recruitment at birth or in childhood, and some (or possibly no) collection of postnatal biospecimens, precluding a detailed study of prenatal exposures, at least for non-persistent exposures. These studies, an example of which being ALSPAC cohort (Bristol) [27], can be termed the first generation of birth cohorts, and have typically been used in the 1990s or earlier. Later, a second generation of birth cohorts have been conducted with earlier recruitment (typically in the second trimester of pregnancy), with some collection of (prenatal) biospecimens; examples include EDEN (France) [28], Inma (Spain) [29], the Center for Children's Environmental Health (CCEH, New-York) cohorts [30], or the Child Health and Development Studies pregnancy cohort, started in the 1960s (California), which allowed highlighting a deleterious effect of perinatal exposure to DDT on breast cancer risk in the first 50 years of life [31]. Some studies have even been able to recruit couples before the conception of the index pregnancy, such as LIFE cohort (USA), which considered many environmental exposures [32] or EARTH, a Massachusetts study of couples resorting to assisted reproduction technologies [33]. The largest recent birth cohorts with interest for environmental contaminants are MoBA cohort in Norway, with about 110,000 children recruited prenatally from 1999, and the JECS (Japan Environment and Children Study) cohort, which enrolled about 100,000 pregnant women and their child in 2011–2014.
Large cohorts are particularly relevant to study rare diseases, provided that the increase in sample size is not done at the cost of increased exposure misclassification. When exposure is assessed through (possibly costly) exposure biomarkers, the cost of the assessment of exposure can become prohibitively high in such large studies. Instead of using “cheaper” approaches to assess exposures (such as, e.g., questionnaires), which might entail a larger amount of exposure misclassification, a solution is to focus on the disease cases that occurred within the cohort during follow-up and only a subgroup of persons who had not developed the disease at a specific time during follow-up, and assessing exposure in the biospecimens collected at inclusion in these two subgroups instead of the whole cohort. This approach, corresponding to nested case-control, case-cohort and related designs [34], allows one to benefit from the prospective collection of biospecimens typical of the cohort approach while analysing a number of biospecimens corresponding to just a few times the number of cases, as in case-control studies; thus, in a way, one takes the best of both the worlds of cohort and of case-control designs. This design has for example been used in the already mentioned study of the effects of early-life DDT exposure on breast cancer [31].
3.2 Health impact and cost assessment studies
Cohorts, case-controls and other etiological designs allow characterizing the dose-response function, under the form of multiplicative (e.g., relative risks) or additive measures of association between the exposure and the health parameter of interest. These measures of association are not totally meaningful in a public health perspective, since, for example, an exposure with a steep dose-response function can have a low public health impact if exposure is very rare (which does not mean, of course, that there is no health issue for the–few–exposed subjects); symmetrically, an exposure with a shallow dose-response function can have a huge public health impact if the exposure is very prevalent in the general population, as is, e.g., the case for fine particulate matter exposure in many parts of the world [35]. Health impact assessment studies consist in combining information on the distribution of exposure at the population level with the dose-response function corresponding to the exposure, in order to obtain an estimate of the number of disease cases attributable to the exposure. This number of cases is more meaningful in a public health perspective than a measure of association such as the odds-ratio. The approach can be repeated for all health outcomes possibly induced by the exposure, and can also be used to estimate any change in life expectancy or number of disability-adjusted life years (or DALYs) lost because of exposure. The resulting number of disease cases or of years of life expectancy lost because of the exposure can be “converted” into monetary units, as a challenging but convenient way to sum disease cases of various pathologies. This approach has been used to estimate the economic cost corresponding to the health effects of exposure to suspected EDs in the European Union (EU) [14,36]. The study gathered all exposure data available for the populations of EU countries (thus excluding many potential EDs on which no exposure data are accessible), and identified the corresponding dose-response functions in the epidemiological and toxicological literature; the corresponding costs were weighted according to the strength of the scientific evidence on each dose-response function, as an appealing way to take into account uncertainties. According to this study, the economic cost of exposure to EDs in the EU is in the 100–200 billion euros range, which corresponds to about 1% of the EU gross product; this is about one fourth of the cost of tobacco smoke exposure, which has been estimated to correspond to 544 billion euros for the year 2009 [37].
4 Issues related to exposure assessment
4.1 Current chemicals are moving targets in the body
Exposure assessment is a central issue in epidemiological studies exploring the health effects of EDs. In the context of EDs with multiple sources and routes of exposure, such as pesticides, bisphenols or phthalates, the concentration of the chemical or its metabolites (also called biomarker of exposure) is commonly used as a proxy of the internal dose. For rapidly metabolized chemicals, the concentration measured in urine, an easy to collect and non-invasive matrix, is often used.
In most epidemiological studies on the health effects of EDs, biomarkers are measured in a small number of biological samples (often only one) in each person. Chemical compounds currently produced (Fig. 4) have a short toxicological half-life. For such chemicals with short half-life and with sources of exposure related to episodic personal behaviours such as dietary intake or cosmetic use, individual urinary concentrations are likely to be highly variable within a day and between days [38,39] (Fig. 5). Consequently, the concentration measured in a spot urine sample is a (sometimes very) imperfect proxy of the averaged exposure during a time window longer than a few hours, such as a week, a month or a pregnancy.

Qualitative depiction of the production levels of typical chemicals in Western countries during the Anthropocene era [49]. The vertical axis corresponds to an arbitrary scale.
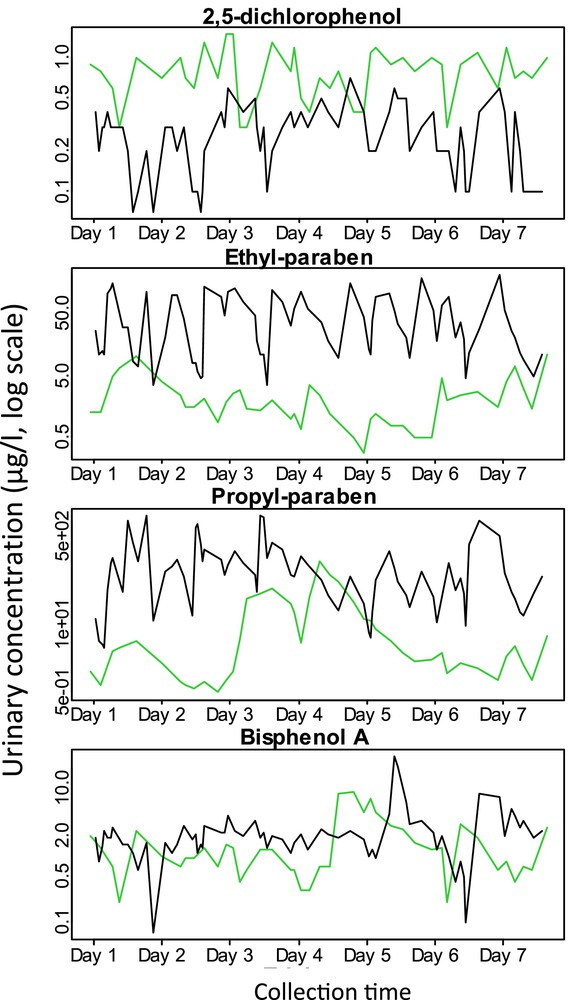
Within-person variations in urinary concentrations (μg/l, log scale) of select phenolic compounds in two pregnant women during a week. Based on a total of 114 urine samples collected during a 7-day period in two pregnant women from SEPAGES-feasibility study (Vernet et al., in preparation). Assays were performed in A. Calafat's laboratory (USA). Each colour corresponds to a different woman.
4.2 Exposure misclassification: nature and consequences
In this context, using a spot urine sample to assess exposure to short half-lived EDs is likely to lead to measurement error; the structure of error is expected to be of classical type. This type of error indeed arises when the estimator of the true exposure (i.e., the biomarker concentration measured in a spot urine sample) varies around the true unmeasured value (i.e., the averaged exposure over the time window of interest) in such way that the average of many replicate measurements approximates the true unmeasured value [40]. Measurement error of classical type in expectancy biases dose-response relationship towards the null (i.e., attenuation bias) [40]. It has been shown that, for chemicals with extreme temporal variability such as bisphenol A (with an Intraclass Correlation Coefficient of 0.2 [41]; this coefficient approximates the correlation between two random measurements done in the same subject), the observed effect estimate can be attenuated by as much as 80% compared to the real effect. Bias in the effect estimate depends on the biomarker concentrations variability and is lower for chemicals with lower variability; for example, the attenuation bias is about 40% for chemicals with an Intraclass Correlation Coefficient of 0.6 [42]. The statistical power is also consequently decreased, i.e. the false negative rate increases, while no inflation in the false positive rate (first type error) is expected. In other words, disregarding any other source of bias, classical type error in exposure is expected to increase the proportion of null studies, but not of studies highlighting a positive association between short half-lived EDs and a health outcome [42]. Thus, classical type error will make the literature on a short half-lived compound with a causal effect on a health outcome look more heterogeneous (i.e. the proportion of “null” studies, not reporting an association, will be increased, compared to a situation without classical-type exposure misclassification). Note that this heterogeneous literature should generally not be qualified of being inconsistent, since a null study is no proof of a lack of association, unless its power is close to 100%.
4.3 Reducing exposure misclassification through within-subject pooling of biospecimens
An efficient way of reducing measurement errors of classical type and accordingly the bias in the dose-response function is to increase the number of samples collected per participant during the toxicologically-relevant time window [43]. For chemicals showing high temporal variability such as bisphenol A, the number of samples required to reduce bias to less than 10% could be as high as 25 per participants [42]. This involves a cumbersome (yet feasible) organization for the study participants and the research team, and leads to increased analytical costs if biomarkers are assessed in each spot sample separately. This may explain (but not justify) why the reliance on a small number of biospecimens per person (often one, rarely two or three) remains the rule today in studies on the health effects of short half-lived chemicals.
An option to benefit from the collection of repeated biospecimens per person without increasing analytical costs, compared to the situation where one sample is available, is to pool the biospecimens of each person collected in the same toxicologically-relevant time window, before assaying the chemical of interest. Despite its simplicity, within-subject pooling has been very little used in biomarker-based environmental epidemiology. By efficiently reducing bias without increasing analytical costs [42], within-subject person biospecimens pooling could induce the shift in practice that the amount of attenuation bias likely to exist in current studies requires.
4.4 Measurement error models
If analytical cost is not an issue, assessing biomarkers in repeated spot samples per participant, without pooling, is another option. It provides information on their intra-individual and temporal variability. Such information can then be used in the framework of measurement error models such as regression calibration and SIMEX [40,44]. These models make use of the assay of several biospecimens per person to limit the impact of measurement error on the dose-response function. For a given number of biospecimens per person, bias is further reduced when the repeated biospecimens are considered within the framework of measurement error models, compared to within-subject pooling [42]. However, compared to the within-subject biospecimen pooling approach, measurement error models do not increase power and entail higher analytical costs.
All in all, there are now appealing approaches to limit exposure misclassification in studies of short half-lived EDs. These approaches will however not correct for the fact that urinary concentrations are not equivalent to the dose of interest, which can be the dose that reaches a specific organ or the foetus (in the context of prenatal exposure). Toxicokinetic modelling and so-called reverse dosimetry approaches [45] are tools worth considering to try obtaining an estimate of dose, or possibly organ-specific doses, which would be relevant to facilitate comparisons with toxicological studies, in which exposure is typically known, but not the urinary levels of the compounds of interest.
5 Conclusion towards a new generation of birth cohorts
5.1 Three generations of birth cohorts
We have presented the design of the birth and pregnancy cohorts conducted so far to characterize the effect of EDs and other environmental factors on health. We have termed these the first (characterized by a postnatal recruitment and a lack of prenatal biospecimens) and second (with recruitment during and sometimes before pregnancy and some biospecimens collected before delivery) generations of birth cohorts. We have also presented issues associated with the assessment of exposure to EDs with a very short biological half-life when there is a single biospecimen per person, as was the case for the majority of previous epidemiological studies, and the promises held in studies resorting to repeated within-subject collection of biospecimens. These issues do not invalidate previously reported associations, since the bias corresponds to attenuation in dose-response function, without increased false positive rate [42]. With the advent of molecular biology, the decrease in the cost of exposure biomarker assays, the better understanding of the exposome concept (defined as encompassing all environmental exposures, including lifestyle factors, from conception and throughout life [46]) and of the effect of the epigenome on health and of its sensitivity to environmental factors including EDs [47], with the possibility to rely more strongly on information and communication technologies and personal sensors [48], we believe that the road is now open for the development of a new generation of pregnancy cohorts.
These cohorts of a new generation (Fig. 6) would be characterized by (i) very early recruitment during or if possible before the pregnancy of women and their partner, and long-term follow-up of the offspring; (ii) collection of a variety of biospecimens (urine, hair, milk, placenta, stool samples, parental blood, umbilical cord and child blood samples) in each couple–child trio, with repeated collection of biospecimens for urine and possibly other matrices such as faeces (allowing characterization of the microbiota) or milk; (iii) collection of tissues (e.g., placenta) allowing the extraction of DNA, RNA, possibly proteins, if possible also repeated within person; (iv) collection and storage of living cells (e.g., from umbilical cord blood); (v) regular contacts and assessments of behavioural and health parameters, through specific devices (e.g., GPS for time-space activity, accelerometers for physical activity) and Internet or paper-based questionnaires, as a way to collect accurate information on potential confounders and effect modifiers.
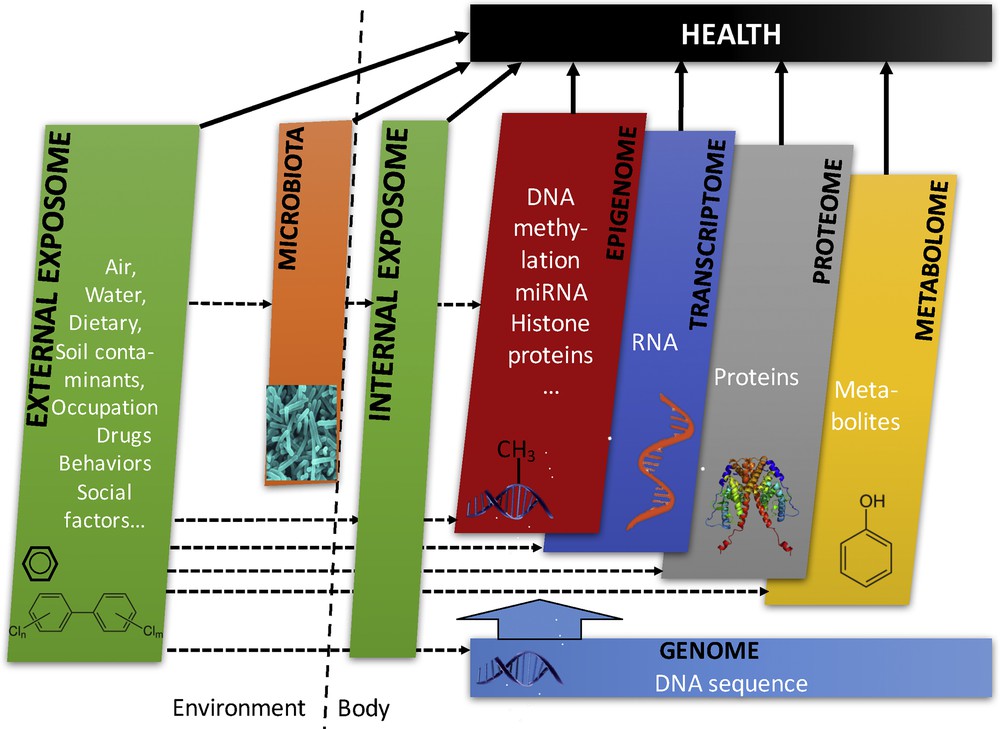
Overview of the cross-omics and other layers of data that can be collected in the third-generation of birth cohorts. From Siroux et al. [46].
Examples of this approach include, e.g., SEPAGES couple–child cohort coordinated by INSERM (recruitment, 2014–2017, 484 couple–child trios), in which over 40 urine samples per woman have been collected during pregnancy as well as several urine samples in the offspring to assess exposure to EDs with a short biological half-life such as phenols and phthalates. The main aim of the SEPAGES couple–child cohort is to characterize the impact of these and other EDs, as well as atmospheric pollutants, on growth, respiratory health and neurodevelopment; DNA, RNA in placenta and parental blood, live cells in maternal and cord blood as well as repeated stool samples are also collected.
There is of course a continuum in the design of cohort studies, but we believe that this distinction between various generations of pregnancy cohorts can be helpful to show a direction that is expected to allow decreases in exposure misclassification for short half-lived chemicals, improved control for potential confounders and exploration of intermediate biological pathways, such as alterations in epigenetic marks, gene expression or microbiota composition and function.
5.2 Let us not forget our past: epidemiology and toxicology working hand in hand on EDs
The field of ED research has since the beginning been built upon the work made by various disciplines of life and environmental sciences operating at various scales. The development of pregnancy cohorts including large biobanks such as those highlighted above now makes it possible to develop tighter collaborations between these disciplines and move from an interdisciplinary to a more transdisciplinary perspective; for example, the comparability of toxicological and epidemiological studies can be greatly enhanced by focusing on similar biological and health endpoints assessed in similar ways, or by more systematically relying on one discipline to generate hypotheses that could be confirmed by the other discipline. As an illustration, high-throughput toxicological designs could be used to identify chemicals with an increased likelihood of ED mode of action, while in vivo toxicological studies and epidemiological studies could be used to confirm the relevance of the mechanisms in organisms and humans and identify possible short and long-term health effects. Such integrated approaches could allow an enhanced comparability of studies at different scales, deeper insight about effects in humans, and faster conclusions regarding the effects and the mechanisms of action of suspected EDs, for the benefit of public health.
Acknowledgements
R.S. and SEPAGES cohort are supported by the European Research Council (ERC consolidator grant No. 311765-E-DOHaD, PI, R. Slama) and the French Agency for Research (ANR). The SEPAGES feasibility study was supported by ANSES (PI, V. Siroux) and the “Fonds pour la recherche en santé respiratoire” (FRSR).