1 Introduction
The dynamics of mathematical models of complex systems generally involves a very large number of variables evolving according to a set of nonlinear differential equations that cannot be treated analytically. Therefore, it is necessary to look for approximation methods that enable to simplify the model. A hierarchically organized system is an interesting case because the structure of such a system shows a partial decoupling between degrees of freedom belonging to different levels in the hierarchy or to different groups at the same level. Consequently, models of complex hierarchical systems can be simplified, however the simplification is generally based on approximation methods and thus the question of its validity has to be examined.
Roughly speaking, in biology, one considers the molecular level, the biochemical level, the cellular level, the organic level, and the organism level [1–3]. In ecology, one can think of the individual, the population, the community and the ecosystem levels. The hierarchical structure of systems in biology and ecology has been particularly studied by Allen et al. [4] and Auger [5]. Slow–fast models and perturbation methods permit some simplifications [6–8]. In this article, we focus on a method known as variables aggregation method. The main goal of this method is to reduce the dimension of a mathematical model of a system so that it becomes handled analytically. The aggregation of the model consists in defining a small number of global variables, which are functions of the state variables of the model, and then building a new system describing the dynamics of these global variables [9–16]. This paper gives a brief synthesis of the aggregation method for time continuous systems of ordinary differential equations (ODEs). For time discrete models, we refer to [17–21].
2 The complete dynamical system
We consider a dynamical system characterized by N time dependent state variables ni(t), i∈{1,2,…,N}, evolving according to a set of N first order ODEs:
(1) |
In a hierarchical system, the state variables can be considered as belonging to groups (Fig. 1). We denote groups by an index α; calling A the number of groups, we have α∈{1,2,…,A}. Of course, the number of groups is assumed to be smaller than the number of state variables, i.e., A<N. The variables are now designated by njα, α∈{1,2,…,A}, j∈{1,2,…,Nα}, with an upper index labelling the group and a lower index labelling the variable within the group, and we have ∑α=1ANα=N.
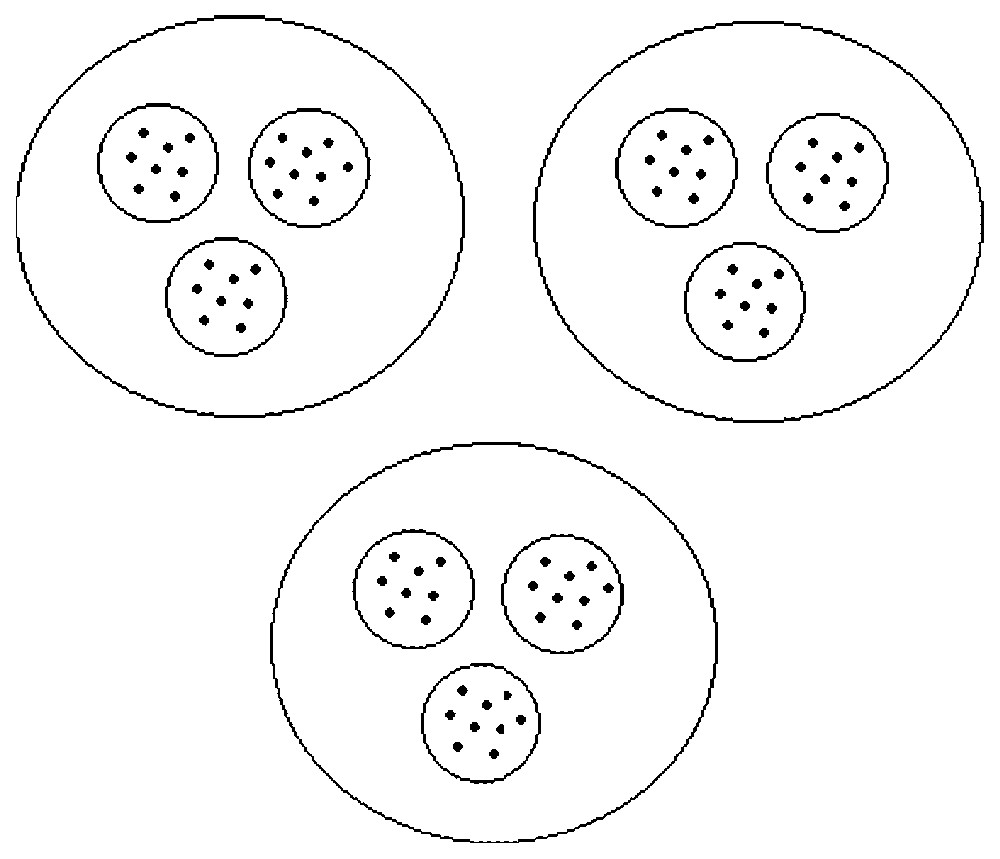
Schematic view of a hierarchical system. The system shown is composed of three groups that are themselves divided into three subgroups. The interactions within a group are strong and the interactions between the groups are weak.
In order to emphasize the hierarchical structure of the system, (1) is rewritten as follows:
(2) |
The (intra-group) part fjα of the differential equations, which contains only variables belonging to the group α of the particular variable njα, has been separated from the remaining (inter-group) part, which includes the coupling with the other groups. The basic assumption that defines a hierarchical system is that the intra-group part is much larger than the inter-group part, i.e., that the parameter ε is small. In other words, in a hierarchical system the interactions within a group are strong while the coupling between different groups is weak. As a consequence, the inter-group part in system (2) can be regarded as perturbations with respect to the intra-group one.
3 Choice of global variables
An important step in the aggregation method is to introduce for each group α a global variable Vα. Vα is a function of njα and the dynamics of Vα is expressed in terms of njα as:
(3) |
(4) |
(5) |
(6) |
The global variables Vα have to be chosen in such a way that their dynamics is slow with respect to that of the intra-group variables njα. An efficient way to define these variables is to look for conserved quantities for the intra-group dynamics, i.e., quantities that would remain constant if the inter-group interaction was turned off. The mathematical consequence for the choice of such variables is that the internal part vanishes, i.e., I=0. Consequently, the dynamics of the variables Vα is only governed by the slow part E of the complete system. It is therefore convenient to introduce a slow time unit t=ε·τ. Then, at the slow time scale, the system (4) becomes:
(7) |
4 The aggregated model
The last step of the aggregation method is to express E in terms of the global variable Vα only. A sufficient condition is that the fast part dynamics quickly reaches an asymptotically stable equilibrium, denoted . For every α, is the equilibrium of the complete system (2) when ε is set to 0. is a function of Vα (constant at the fast time scale), which can be substituted into equations (7) leading to the following system for the global variables:
(8) |
This method has been extended to systems where the fast part dynamics shows a stable limit cycle [22] and in some cases of infinite dimensional dynamical systems (systems of partial derivative equations) [23,24].
In system (8), the dynamics of each global variable Vα depends on global variables only: this system is called the aggregated model. The aggregated model is a set of A equations only while the complete system (2) is composed of N equations. For instance, in the case of a partition into three groups, each one containing three subgroups, like in Fig. 1, we get three global equations in (8) instead of nine equations in (2). This clearly shows how this method can lead to an important reduction in the number of equations.
An aggregated model is different from the initial complete model. However, it can be shown that the dynamics of the aggregated model is a good approximation of the dynamics of the complete one if (i) ε is small enough and (ii) the aggregated model is structurally stable. If the second proposal does not hold, one has to calculate further terms of the aggregated model that can be expressed as a Taylor expand in function of increasing powers of the small parameter ε [13,14,16].
5 Applications to population dynamics
In the context of population dynamics, the state variables njα are population densities. For instance, index j refers to different spatial patches and index α to different species. The intra-group dynamics is the migration between the patches for each species, which is conservative; the inter-group dynamics is the interaction between the species. The global variables Vα=∑j=1Nαnjα are the total populations per species, the partial derivatives of the global variables are simply for any α and j, and system (8) simplifies:
(9) |
In order to illustrate the general method, we are now going to present a new and original application to population dynamics. We consider a population that is distributed on two spatial patches. Let n1(t) and n2(t) be, respectively, the sub-populations densities on patch 1 and 2 at time t. The complete model is composed of the two following equations:
(10) |
The model is composed of two components, a fast part corresponding to migrations between the two patches and a slow part that relates to the sub-population growth on each patch. The fast part describes the migration between the two patches with constant migration rates kij from patch j to patch i. Imagine that the two patches would not be connected, i.e., the migration rates are equal to zero. Then sub-population dynamics on patch 1 would show an Allee effect [25]: for any positive initial condition below the threshold M, the population would decay and go to extinction; otherwise it would tend to the carrying capacity K. Patch 2 would be a source or a sink according to the sign of the growth rate r2. We now study the situation where the two patches are connected by fast migration. In this case, the aggregation method presented above can be applied. First, it is easy to show that the fast part has a positive and asymptotically stable equilibrium: (11)
(12) |
There are three different cases.
- (i) . This condition is equivalent to Δ<0. The origin is the unique equilibrium and it is asymptotically stable. The total population goes to extinction (Fig. 2A): the global dynamics is sink-like.
- (ii) . This condition implies that Δ>0. There exist three equilibria, the origin (stable) and two positive ones, the smallest being unstable and the largest stable (Fig. 2B). In this case, the global dynamics shows an Allee effect.
- (iii) . This condition implies that Δ>0. There exist three equilibria, the origin and two others, one positive and one negative. The origin is unstable and the positive equilibrium is stable. Thus, the global dynamics is logistic-like (Fig. 2C).

(A)–(C) Time evolution of the total population density in the aggregated model (12) with r1=0.2, M=0.5, K=2, , and (A) r2=−0.5, (B) r2=0.05, (C) r2=0.5. (D) The three types of dynamics shown by the aggregated model [12] with respect to and r2 (the curves shown correspond to the particular values of the parameters r1=0.2, M=0.5 and K=2, but their shapes are general).
The three previous cases are represented on Fig. 2D with respect to parameters and r2. In the sink-like and Allee effect cases, the total population has a global behaviour that is similar to the local behaviour of one of its sub-populations. However, in the logistic-like case, the total population behaviour is different from its sub-populations behaviours. This example shows that, in general, the global model may have a qualitatively different dynamics than the local ones.
Regarding other applications to population dynamics, aggregation methods have been used in the following cases: (i) modelling a trout fish population in an arborescent river network composed of patches connected by fast migration [26,27]; (ii) studying the effects of different individual decisions on the global dynamics of a prey-predator system in an heterogeneous environment composed of patches connected by fast migration [28–32]; (iii) modelling a sole larvae population with a continuous age with fast migration between different spatial patches [23]; (iv) modelling the influence of different individual strategies on the dynamics of a population of two competing populations using fast game dynamics [33–36]; (v) studying the effect of frequent migrations on the stability and persistence of host–parasitoid systems [37].
6 Conclusion
Starting from a complex dynamical system, the aggregation method presented in this paper enables to build a reduced system which dynamics is easier to analyse. This method relies on the existence of different time scales, i.e., the dynamics of the system involves fast and slow components. Then, the method allows to investigate the effects of the fast processes on the slow dynamics and reciprocally.
The method is general and can be applied in many contexts where hierarchical dynamical systems are used, i.e., in most fields of biology and ecology. Molecular biology made recent important improvements in understanding biological processes at the microscopic level. The next challenge is to develop an integrative approach to assess the influences of these processes at a macroscopic level. In this perspective, aggregation methods can be used as they are based on mutual dependence of the intra- and inter-level dynamics.