1 Introduction
The human body orchestrates gene expression by co-regulating genes whose products function together. Many challenges are posed due to the vast amount of genomic and expression data that has to be divided into functional biological groups using biological, statistical and computational tools. Many past studies centered on comparisons of diseased to healthy tissues, and were limited to a subset of human genes.
High-density oligonucleotide arrays enable highly parallel and comprehensive studies of gene expression. The transcription patterns produced, known as the expression profile, depict the subset of mRNA synthesized in a certain cell or tissue. At its most fundamental level, the expression profile describes, in a quantitative way, which genes are expressed in a particular tissue. More sophisticated issues such as novel gene functional characterization, gene identification in biological pathways, genetic variation analysis or identification of drug targets could be surveyed by using bioinformatics tools such as cluster analysis ([1,2] and self organizing-maps [3,4]).
The construction of gene expression databases is a high priority of today's research community. Such databases, closely integrated with other types of genomic information, promise not only to enhance our understanding of many fundamental biological processes, but also to accelerate drug discovery and lead to customized diagnosis and treatment of disease [5,6]. An example generated by our own group is presented the recent releases of GeneCards, which contain expression data both from array experiments and from ‘electronic Northern’ analyses [7].
As a significant extension of previous relevant efforts, we describe a whole-genome repertoire of expression profiles in twelve normal human tissues. Previously, only studies that compare a single diseased tissue with a healthy one were preformed [8–10]. Other surveys that were done on healthy human tissues were limited to one array type (i.e. HG-U95A [11], Hu6800 [12]). Here, for the first time, we present the expression analysis of the full complement of more than 60 000 gene and EST representations in 12 normal human tissues. It is shown that the additional, less characterized genes harbor important information, and that a whole-genome expression analysis is essential for generating comprehensive transcription analyses.
2 Materials and methods
PolyA+ RNA samples from twelve normal human tissues were purchased from Clontech (Palo Alto, CA). This collection of major human tissues includes: Bone marrow (catalog number: 6573-1), brain (6516-1), heart (6533-1), kidney (6538-1), liver (6510-1), lung (6524-1), pancreas (6539-1), prostate (6546-1), skeletal muscle (6541-1), spinal cord (6593-1), spleen (6542-1) and thymus (6536-1).
Preparation and hybridization of cRNA were done according to the manufacture's instructions [13]. Sufficient hybridization cocktail solution for five independent hybridizations reactions (i.e. the full set HG-U95A-E) was prepared. The hybridization reactions of all the arrays in the set were carried out simultaneously. The mRNA from each tissue was reacted in duplicates against the full human Affymetrix arrays set (HG-U95A-E) to yield two sets of results. The 3′/5′ signal ratios of GAPDH were always below the value of 3 as expected for a fine labeling reaction.
Arrays were analyzed and expression value was calculated for each gene by using Microarray Suit (MAS) version 5.0 software (Affymetrix, Santa Clara, CA). Expression values for each gene, called signal, were calculated using the MAS 5.0 software with its default parameter settings. Scaling was not done via a MAS 5.0 option. Instead, we normalized our data as follows: the intensities of each array were log10 transformed and scaled to a constant reference value (global normalization). This reference value was the mean of all log intensities in all of the tissues. Present calls percentages are presented in Fig. 2 for all samples according to the array type (see also Results and Discussion).

Percent of present calls in all samples according to the array type. ‘Present’ call percentages were taken from the MAS 5.0 report files and are presented for the various array types in all of the samples. The A–E letters represent the array type. The following are the tissue samples shortcuts: BMR for bone marrow, BRN for brain, HRT for heart, KDN for kidney, LNG for lung, LVR for liver, MSL for muscle, PNC for pancreas, PST for prostate, SPC for spinal cord, SPL for spleen, and TMS for thymus. The numbers attached to the sample name represent the replicate number.
3 Results and discussion
Whole-genome gene expression profiles were generated from the normalized signal values. The set of 12 tissue expression values for a given gene was defined as its tissue vector. Normalization was used to allow a meaningful comparison among different tissues. Fig. 1 shows a comparison of the intensities distribution of one heart sample for the five different arrays, before and after normalization. In this density plot, it is observed that the highest intensities are in arrays A and B, while arrays C, D and E produce considerably lower intensities. These results are similar to the earlier observations by Bakay et al. [8]. Aspects of the decreased array intensities are also presented in Fig. 2 which displays the percentage of ‘present’ calls along the samples for each array type. For example, it is well observed that the percentage of ‘present’ calls in array D is consistently below 10%. This could be the result of intrinsic hybridization differences. It could also be due to the average quality of the probe-sets, since most of the well-studied genes are found on array A while arrays B to E are constructed with increasing propensities of less well-characterized ESTs (Expressed Sequence Tags).
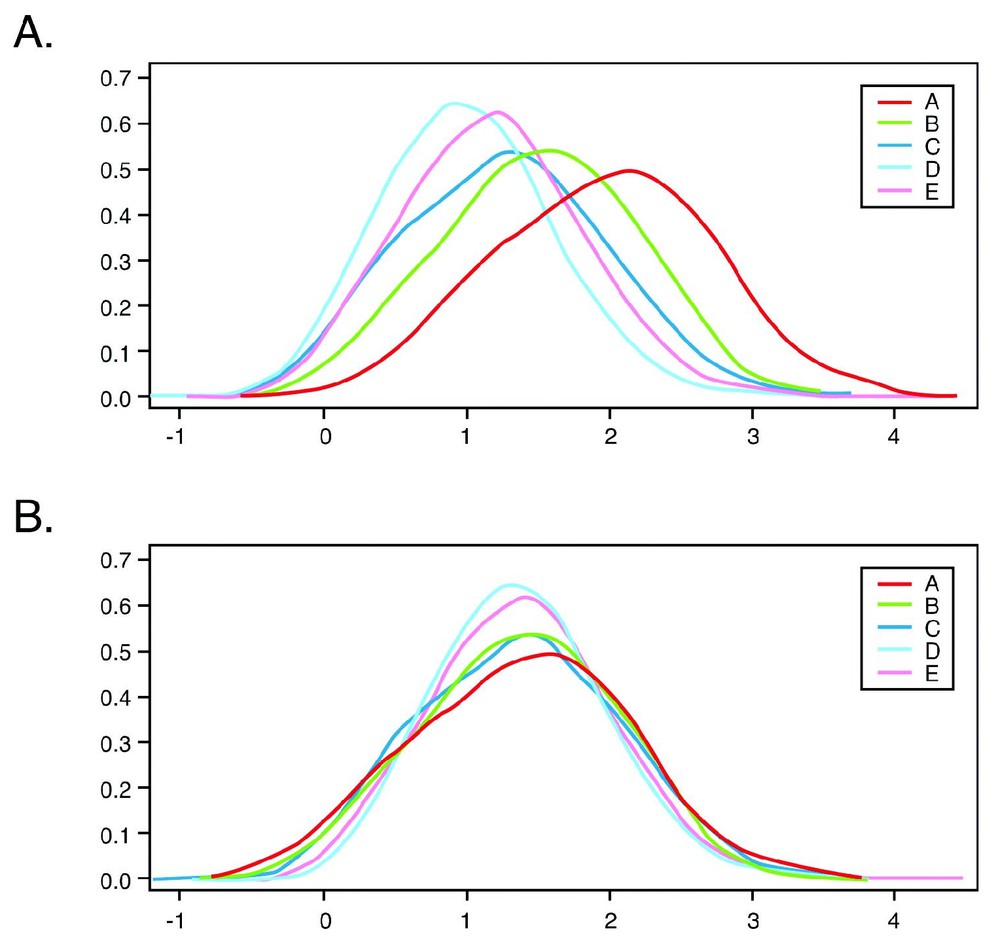
Intensity distribution in the different arrays set in heart sample. Density plots of one heart sample before and after normalization are presented. Panel A presents the density plot for log10 raw signals while panel B presents the normalized signals.
Tissue specific genes are supposed to have a significant role in tissue functionality. Hence, we examined the counts of such genes in the different tissue samples (Fig. 3). One representative sample for each tissue was chosen, and tissue specific genes were determined on the basis of the ‘absent–present’ calls of the Affymetrix MAS 5.0 software. A similar criterion was used in the past for housekeepings genes selection [14]. In this paper, we define tissue specificity as having a ‘present’ call in only one tissue. It is observed that brain and thymus had the highest number of tissue specific genes, almost twice the number observed for other tissues. The propensity of tissue specific genes is not much lower in arrays B–E when compared to array A, suggesting that important information also resides within the former. However, one should also take into consideration that the amount of ‘present’ calls is reduced on arrays C–E (Fig. 2). Consequently, the increased effect of tissue specificity may be an artifact of the array quality and design.
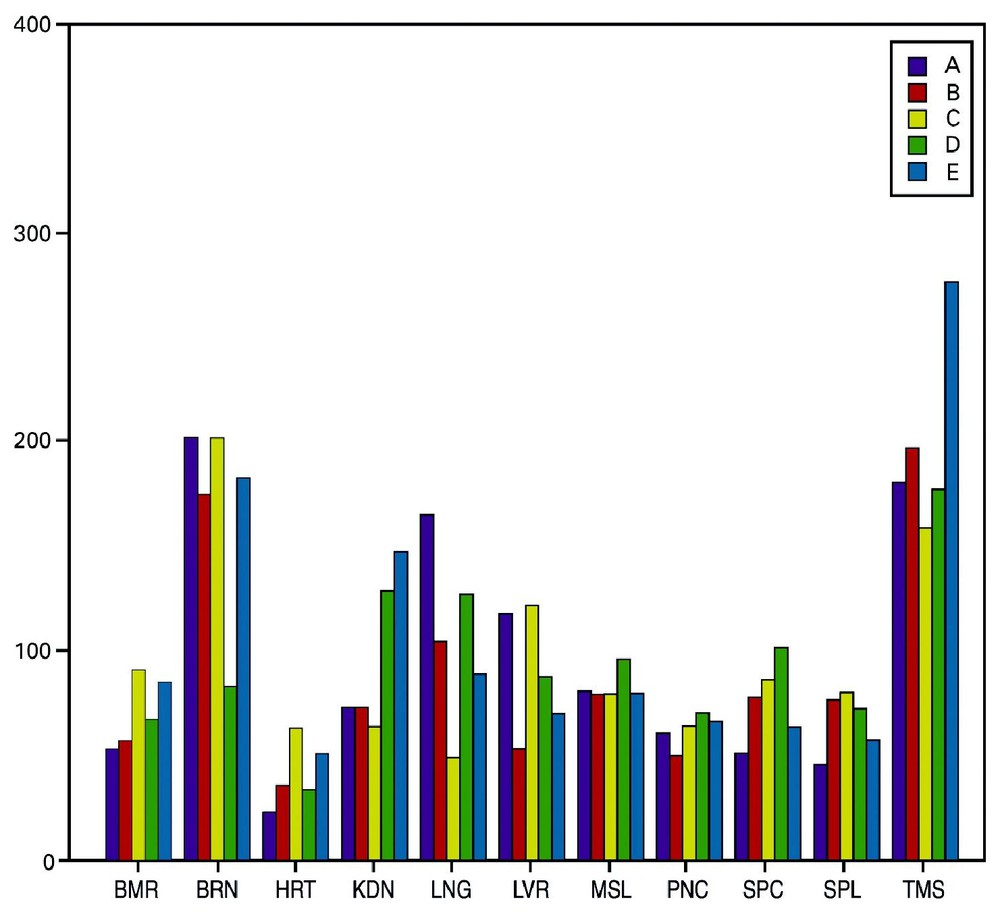
Tissue specific genes in all of the tissues sorted by the array set. Tissue specific genes were found based on the ‘absent–present’ call of the Affymetrix MAS 5.0 software. Tissue specificity was defined as having a ‘present’ call in only one tissue. One sample for each tissue was chosen for the search. The tissue specific genes distribution on the arrays set, HG-U95A-E is shown for all tissues.
The MAS 5.0 package is one of the most commonly used software tools for analyzing high-density micrroarray results. However its use of ‘present–absent’ calls is a controversial issue in the literature [15–17]. To address this, we have begun to develop alternative methods utilizing a normalized entropy-related Tissue Specificity Index (TSI), computed directly from the tissue vectors. This algorithm is currently being evaluated as compared to other methods (O. Shmueli et al., manuscript in preparation).
Criticism has also been directed towards the subtraction of the mismatch probes (MM) from the perfect match probes (PM) creating PM-MM values as an underlying computation for assessing background levels and signal computations. Several methods have been developed to evaluate the background level of intensity above which the value truly reflects the gene expression value [15,16,18–20]. Some of these models fit log(PM-Background), instead of PM-MM, though in low-intensity regions the current models are still very questionable [16]. Consequently, the validity of the zone of low-intensity probes, which is close to the background level, remains undetermined.
The tissue vectors for each gene were drawn on a root scale representation [7] as exemplified in Fig. 4 for the gene APCS (Amyloid P-component, serum) [21]. APCS is a precursor for amyloid component P which is found in basement membrane and associated with amyloid deposits. A root scale enables one to visualize several orders of magnitude, similar to a logarithmic scale, but preserves some advantage of the linear scale, i.e. a differential increase with the different orders of magnitude. This affords an effective view-at-a-glance of the tissue vectors.
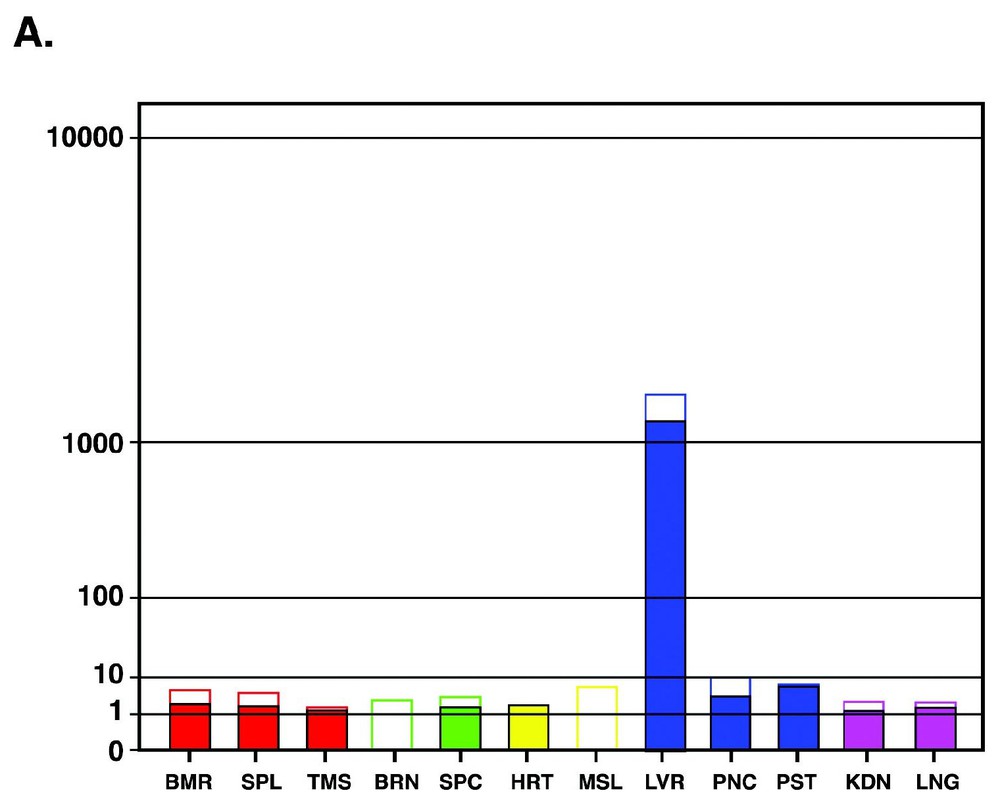

Tissue vector for the gene APCS [21]. Tissue vector for the gene APCS was calculated from the normalized signals and is presented in a graphical way (panel A). Tissues were grouped according to their origin and the groups colored accordingly (e.g., nerve tissues in green). The range between the lower and higher measurements was represented by a white box above the colored minimal measurement bar. The graph is presented on the y-axis with a special root scale [7] Y=X(1/B) where B=log210. Panel B presents the tissue vector colored map.
A major aim of this work is to enable prediction of the function of novel genes based on their expression profiles. It is expected that genes that display similar expression patterns are functionally related, since they are co-regulated under all of the developmental conditions [22,23]. On the basis of the expression profile similarities, ten tissues were ordered in a tissue correlation matrix (Fig. 5). It is clearly seen that array A (Fig. 5A) and arrays B–E (Fig. 5B) show the same pattern of correlations. The high correlations found between tissue replicates is an indication of the validity of the results [24,25]. In addition, the correlation matrix revealed three groups of closely related tissues. The first group with high inter-tissue correlation includes brain and spinal-cord, the second is heart and muscle and the last includes thymus, spleen, bone-marrow and lung. This is in agreement with the expected biological origin of the tissues, since they represent the nervous system, muscle, and immune system respectively.
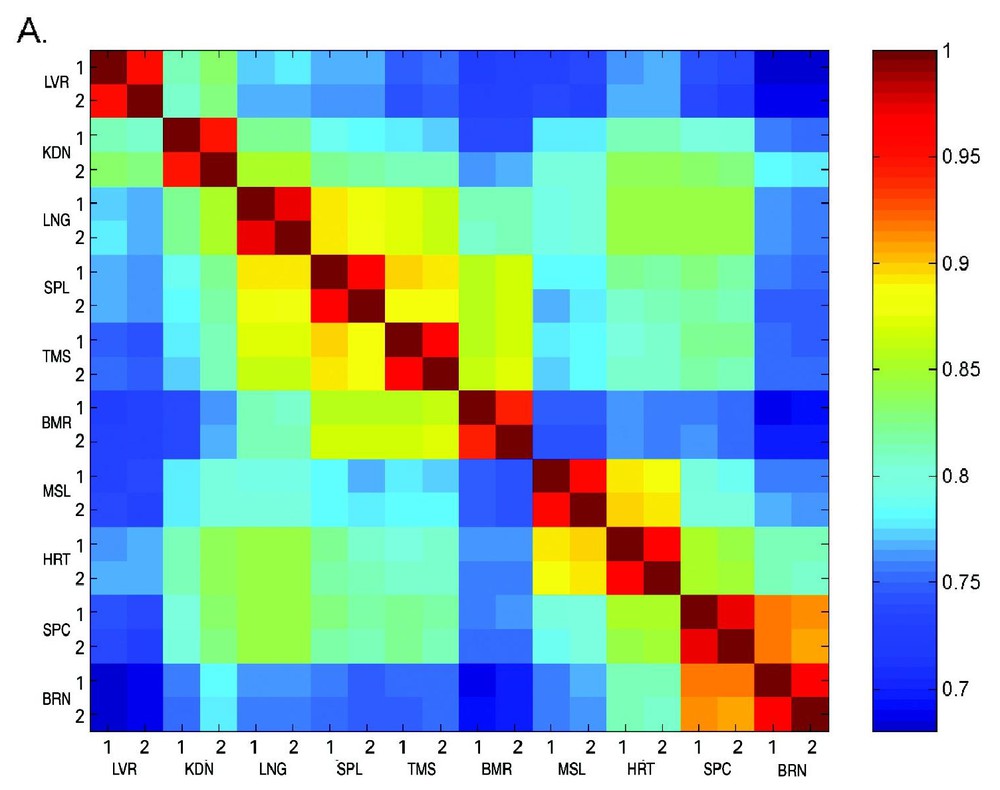
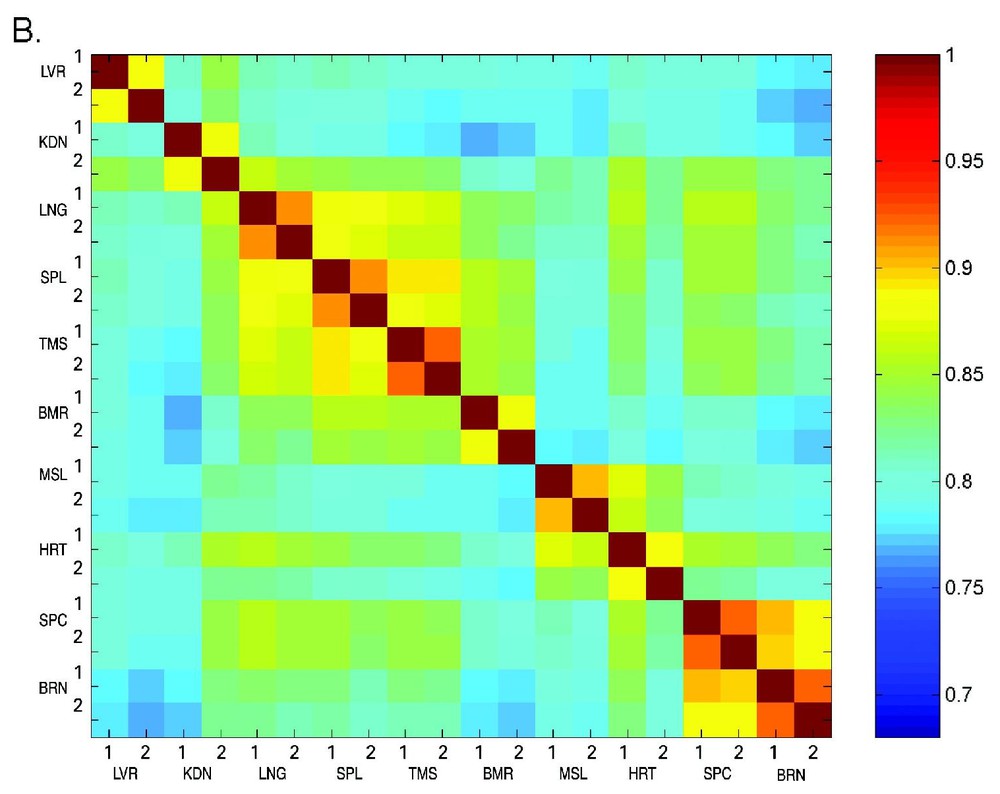
Tissue correlation matrix. Tissue vectors of the normalized signals (log transformed and scaled) were used to calculate correlations between twenty tissue samples. The correlations were calculated using the Pearson correlation coefficient and presented in a tissue vector correlation matrix. Different correlation levels are presented according to the color scale on the right. Panel A presents the tissue vector correlation matrix for array A, while panel B presents arrays B–E.
All expression data, raw as well as normalized, have been stored in the GeneNote database (http://genecards.weizmann.ac.il/genenote/). GeneNote tissue vectors are provided in GeneCards (http://bioinfo.weizmann.ac.il/genecards) for all genes that could be explicitly assciated via the Affymetrix annotation (currently ∼20 000 genes).
The preliminary results presented above provide a clear indication of the importance of surveying gene expression in an as broad as possible a gene set. The combination of comprehensive experimental data gathering, computer analysis, and database display provide relevant and useful tools for the transcriptome community.
Acknowledgements
The work described in this paper was supported by the Abraham and Judith Goldwasser foundation. Doron Lancet is the incumbent of the Ralph and Lois Silver Chair in Human Genomics. Eytan Domany is the incumbent of the Henry J. Leir Professorial Chair.