1 Introduction
The whole Sahelian region and, in particular, Senegal and the Ferlo area are affected by several epidemics with a destructive repercussion on populations, livestock and country's economy. Indeed, these diseases, mostly vector-borne ones (malaria, RVF, dengue, etc.), are influenced by seasonal climate variability, particularly that related to rainfall and temperature anomalies with the increased warming since decades ago [1–3]. Even if climate alone is not responsible for these outbreaks [4], a relationship is probably detected through previous studies [5–7]. This justifies the possibility of predicting diseases’ transmission risks taking malaria as a case of study. Our research is based on climate components from observations and simulations. It is in this context that the Liverpool Malaria Model (LMM) is used in our study to investigate the relationship between climate and health.
Paradoxically, the Sahelian drought since the 1970s has greatly reduced the distribution, abundance and infection rates of malaria anopheline vectors, without being associated with a drastic reduction in the impact on malaria for the most affected populations [8]. The weak efficiency of anti-malaria treatments (ACT pills), including urban areas, partly explains this finding [9]. However, this feature should be also explained by the lack or loss of population's immunity such as children and pregnant women, who are particularly vulnerable. A weak immunity to malaria makes these populations more vulnerable to infection, and epidemic phenomena can arise due to exceptional climate events such as drought or flood.
The QWeCI observatory study, the Ferlo region in Senegal, belongs to the Sahelian climate domain, whose features have often been considered over the normal 1961–1990 period. The main area covered by this study is the Ferlo through its synoptic station of Linguere (and Dahra, which is less documented) and its health district consists of health posts, Barkedji being the most targeted one. In addition to the rain gauge stations of the QWeCI in Barkedji, Linguere station belongs to the Agence nationale de la météorologie et de l’aviation civile (ANACIM) monitoring network and therefore has a long and reliable time series for analysis. Rainfall is measured before the 1950s, as other parameters we use in this study; the main monitoring is done during the 1970s due to the drastic decline in rainfall in the Sahel, attracting the attention of many researchers and inducing reflex documentation to understand this particular meteorological climate accident. To sum up, Linguere also remains the reference climate station of the Ferlo.
2 Data and methodology
2.1 Data
To study the relationship between climate and malaria, we use the clinical malaria index and climate data observations from the districts and stations of Linguere and Dahra in the Ferlo.
Part of this study is devoted to climate and epidemiology characteristics at continental (Africa), regional (Sahel and West Africa), national (Senegal), and local (Ferlo) scales, studied from 1980 to 2009 and from 1950 to 1979 (normal periods).
Some analyses will be done mainly for studying seasonal changes in climate parameters on regional to local scales, particularly for rainfall and temperature in order to capture their variability and their impact on the seasonal transmission of malaria and other vector-borne diseases.
The last part will show the future climate change along time and space on the Ferlo observatory in particular, and on Senegal or the region delimited by the Sahel, to provide also the risk of malaria transmission.
The study is based on data observations and model simulations for daily and monthly rainfall, temperature (maximum and minimum), relative humidity (maximum and minimum), wind speed and direction, temperature and rainfall satellite data (TRMM) and re-analysis from ERA-Interim.
The clinical data corresponds to the number of malaria cases, malaria incidence, morbidity specific, etc.
The simulations from LMM [10] provide data of incidence and prevalence. The model input data are observations such as daily rainfall and temperature, these simulations being performed also for climate change scenarios.
2.2 Methodology
The data has been processed with various scientific computing tools as FORTRAN and MATLAB and several visualization programs as xmgrace, GrADS and FERRET.
The methodology is based on a combined use of data analysis to describe, classify and order the information, but also to reduce large paintings. Spectral analysis and filtering have been also used to highlight periodicities, to extract signals from the studied parameters.
3 Results
3.1 Observation
The seasonal variability of climate parameters, due to their influence on the malaria transmission risk, allows us to better analyze the relationship between climate and malaria. The study of this relationship is conducted at a local scale (Ferlo), but can be extended also to the national and regional levels (Senegal and Sahel). Fig. 1, which represents the annual cycle of mean temperature in the Linguere (Ferlo) station from 1951 to 1998, shows two peaks of temperatures in the months of May and October, corresponding to the start and the end of the monsoon. Although temperature appears to be an important indicator for malaria transmission [11], simultaneous observations of temperature and malaria index covering the same spatial and temporal scale in our study area are insufficient.
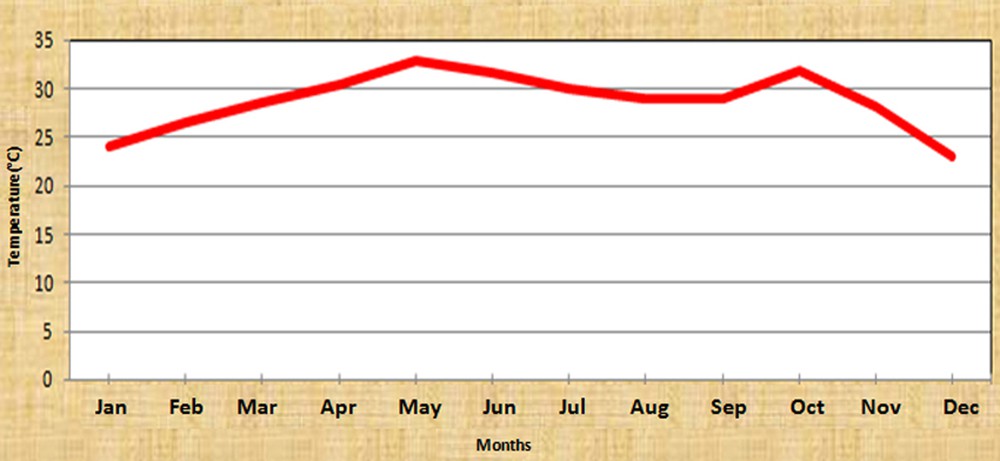
Seasonal variability of mean temperatures in Linguere (1951–1998).
Fig. 2 represents the mean annual cycle of rainfall from 2001 to 2009. It shows a peak in August as it is seen in the rest of the country, which is in agreement with the results of Ndione [12]. The rainy season takes place between June and July before withdrawing progressively from September onwards.
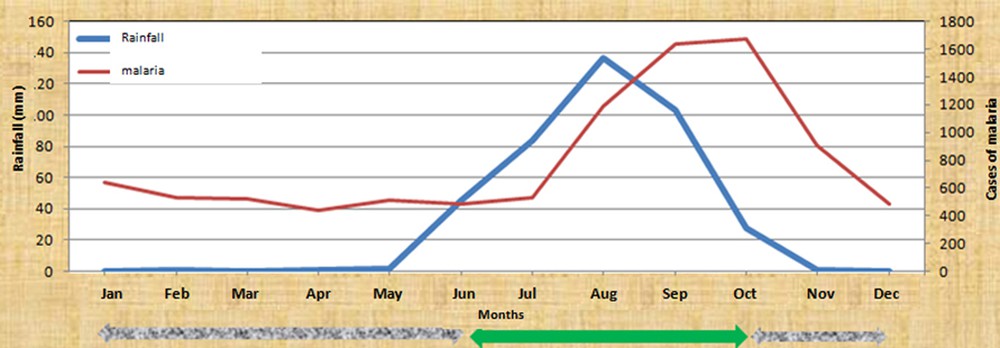
Seasonal variability of rainfall and simultaneous malaria transmission in Linguere (2001–2009).
The evolution of the emergence of malaria follows the same trend as precipitation. Nevertheless, it is important to note the shift in the malaria peak one to two months compared to rain. Unlike the rainfall reaches its maximum in August, the malaria peak is around the month of October.
Concerning Ferlo observations, Fig. 3 shows the existence of two distinctly different climatic periods in humidity. Moisture over the normal 1980–2009 period is lower than over the 1950–1979 one. In summary, since the 1970s, a drastic reduction in rainfall is observed compared to the 1950s. However, we can notice how the rainfall situation has improved in recent years, especially in 2012.
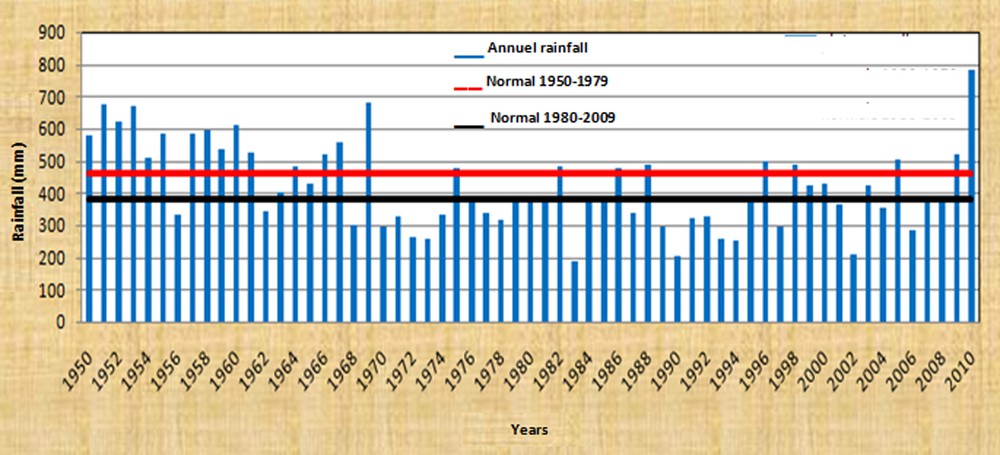
Interannual variability of rainfall in Linguere (1950–2008).
Unlike the interannual rainfall cycle, temperatures are evolving in the opposite direction, as it can be seen in Fig. 4, where the temperatures carry on increasing with some exceptional years.

Interannual variability of temperature in Linguere (1950–2008).
3.2 Simulations
For this part, we mainly do plots with DMC (Cradle Diseases Malaria) and LMM. DMC is the application interface of the LMM. Its purpose is to allow end users to run the model in their local institutions with their own sets of meteorological data, and to study and validate the results against observations on the epidemiology of malaria (e.g., malaria incidence, number of infected mosquitoes, etc.).
These simulated indices are malaria-specific morbidity, prevalence, length of gonotrophic and sporogonic cycle, the number of adult mosquitoes, etc. These simulations are based on daily data climate parameters (rainfall and temperature). These inputs must be identified by geographical coordinates at any point on the spatial scale. For this, the satellite data are quite comprehensive and accessible to give a clearer picture than a map. We also use re-analysis of data to complete our observations database.
Indeed, in order to improve the control of climate variability and to better anticipate and predict its behavior in the near and far future, the model simulation of precipitation is a tool that can be used for two main reasons:
- • the climate data are unevenly distributed both within and between countries;
- • the available data are not sufficient to give a complete view of the rainfall in the country, particularly in the African continent.
The simulations allow us to complete the collection of observational data in less documented areas. They also help to better understand the climatic cycle in spatial and temporal scales, and to study its relationship with malaria in different areas to be considered to include risks. It is in this context that we use different databases as data re-analysis for mapping rainfall in Africa, the Sahel, Senegal, and the Ferlo (observatory area).
Fig. 9 illustrates rainfall mapping in Africa, showing that precipitation data are not uniform through the continent. The Sub-Saharan area and Central Africa are wetter than the extreme north and south zones that are interesting to analyze well due to the high malaria transmission, particularly in this part of the continent (Figs. 13 and 15). There is a strong latitudinal variability of monthly totals, which are higher between 20° S and 10° N, where monthly cumulated rainfall can reach 200 mm. For the southern part and the Maghreb, the monthly totals vary between 0 and 40 mm. Precipitation values higher than 240 mm/month have been found in Guinea, Sierra Leone, Liberia, Cameroon and Chad, but also in Madagascar. In the case of Senegal, the large disparity in rainfall already mentioned with our observations is found with the data re-analyses (Figs. 5, 7 and 8). Fig. 7 shows the zonal and seasonal variability of rainfall in Senegal. These results are in agreement with those obtained from our observation data at Linguere (Ferlo). We found the southern region wetter than the rest of the country, the north being marked by relatively less precipitation. Most of the rainfall is recorded frequently between July and September in the north and between June and October in the south.
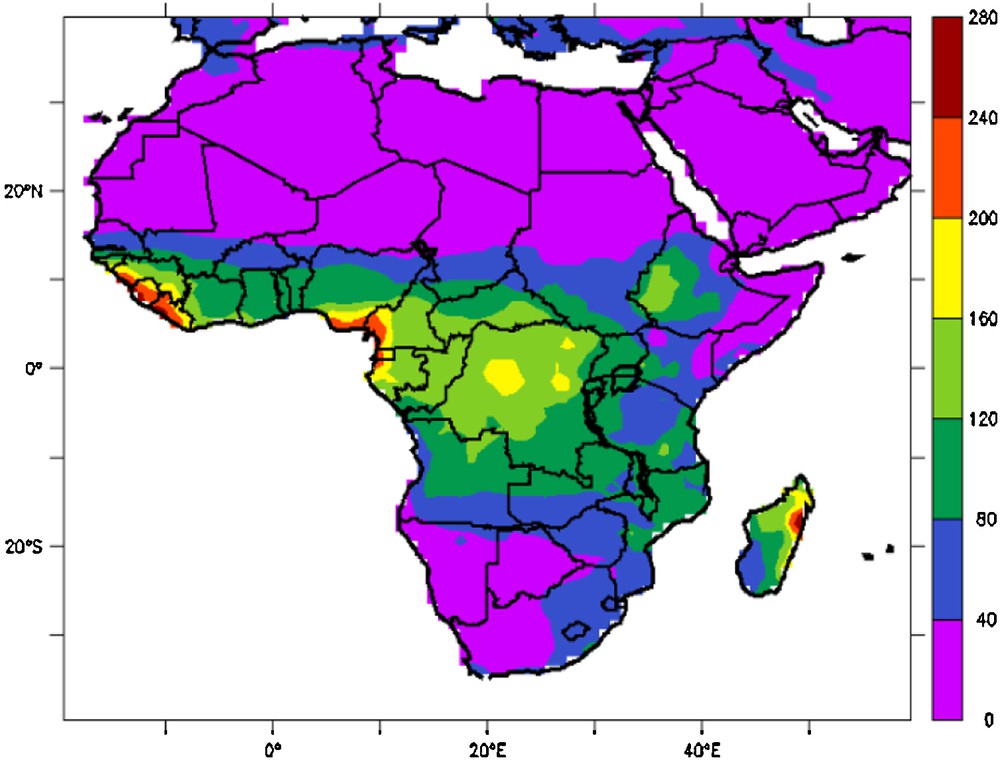
Spatial variability of monthly rainfall over Africa (GPCC data from 1901 to 2012).
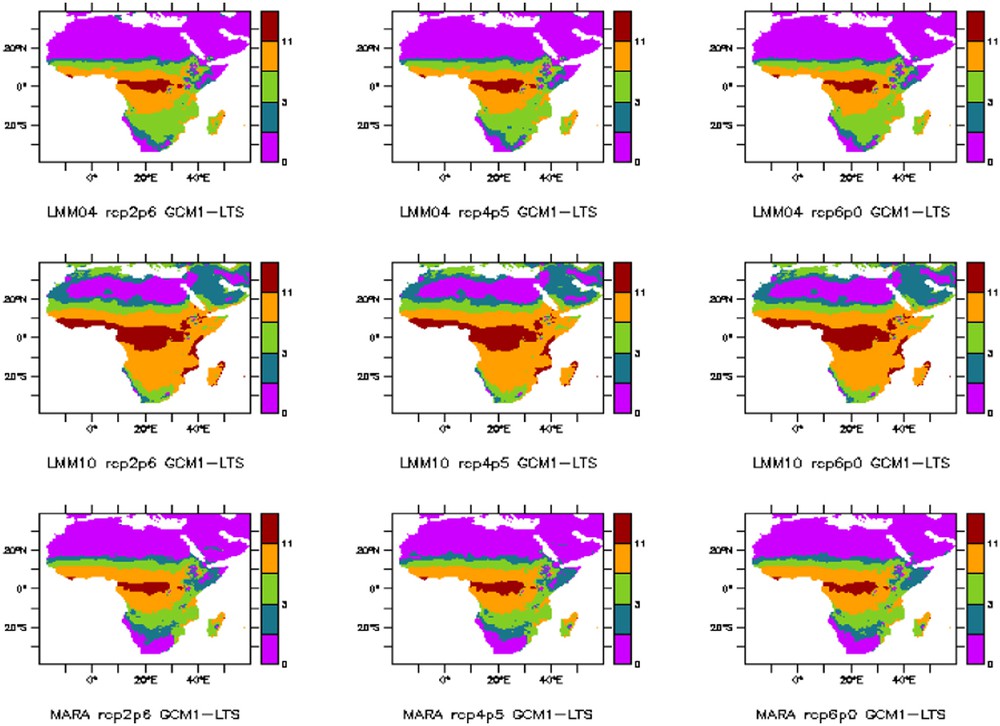
Length of the seasonal cycle of malaria over Africa simulated for the period 2006–2035 by biological models LMM and MARA coupled with climate models HADGEM (GCM1) and IPSL (GCM2).

CS (climate suitability) of malaria over Africa simulated for the period 2006–2035 by biological models LMM and MARA coupled with climate models HADGEM (GCM1) and IPSL (GCM2).
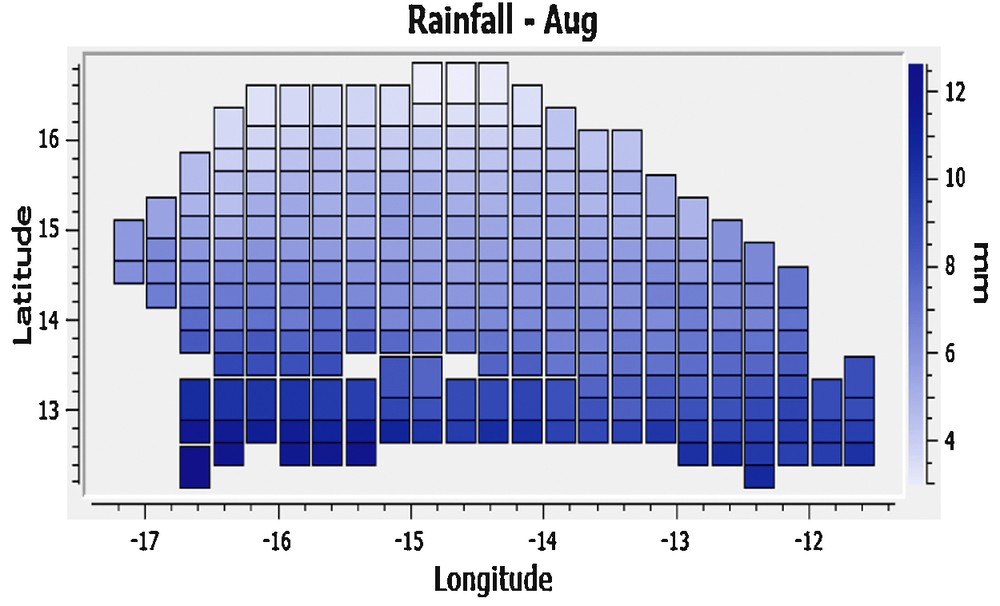
Distribution of mean precipitations over Senegal during the rainier month (August), 1998–2010.

Spatial variability of daily rainfall (mm/day) on JJAS rainfall in Senegal (NCEP re-analysis-DOE AMIP II 1983–2012).

Rainfall cycle from May to October with daily accumulations (mm/day) of rainfall in Senegal.
If we consider Fig. 10, showing the annual cycle variability averaged over the Sahel, Senegal and then the band of the Ferlo, there is a same tendency for the three simultaneous spatial domains considered. Precipitations significantly start from June (50 mm at Barkedji-Ferlo and even with a higher amount for the Sahel and Senegal). However, it should be noted that the averaged monthly cumulated rainfall over the Sahel is not negligible from May onwards (about 25 mm). The peak in August for the average over Senegal is more pronounced (near 250 mm) than averages over the Sahel and the Ferlo (less than 190 mm).
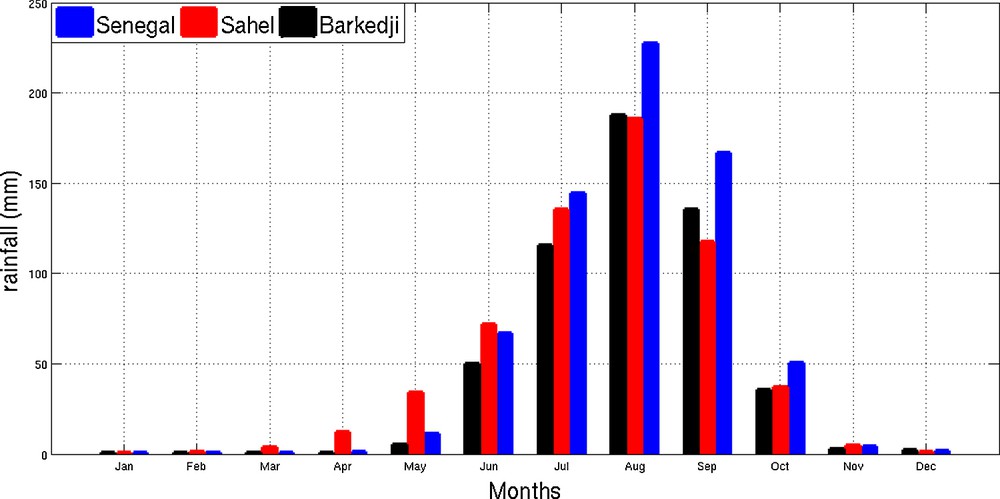
Annual cycle of precipitation averaged over the Sahel, Senegal and Barkedji (GPCC 1901–2012).
Regarding the end of the rainy season, it takes place during the month of October, with more cumulated precipitations over Senegal than over the Sahel area. Those high cumulated values reach 50 mm for Senegal. The end of the rainy season takes place from October in the northern and central parts of Senegal. Therefore, the rainy season can be extended until November for the southern and southeastern parts of the country.
The time evolution of rainfall in Fig. 11 shows positive anomalies until the 1970s becoming mostly negative, a feature that is more noticeable for the anomalies of Senegal, where they are more pronounced.

Average rainfall anomalies over the Sahel, Senegal and Barkedji (GPCC 1901–2012).
However, like it happens with the rainfall data, it would be interesting to have simultaneously malaria observation data for comparisons between the whole Sahel, Senegal and the Ferlo area.
With the DMC (LMM interface) also, Fig. 5 represents the distribution of August (the wettest month in Senegal) rainfall from 1998 to 2010. One can see a latitudinal gradient with rainfall intensity decreasing from the southern to the northern areas. Fig. 6 shows the two peaks of temperature respectively in May and October, in agreement with the observations.
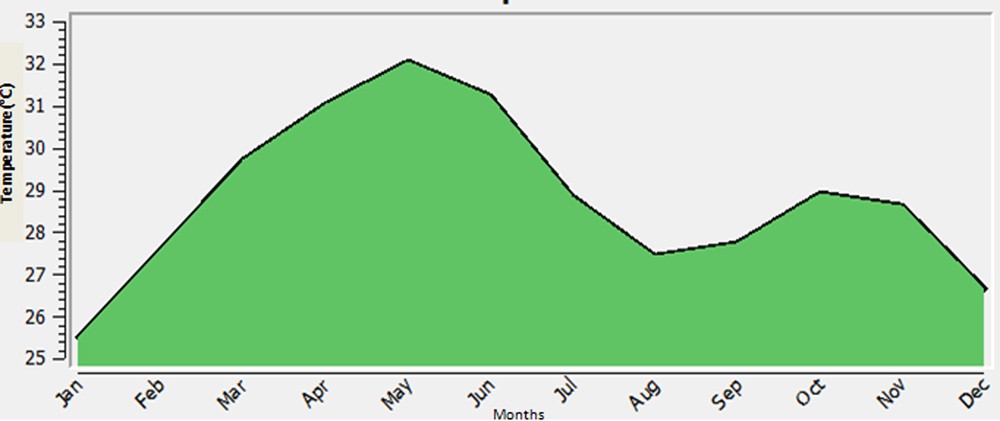
Seasonal variability of mean temperatures in Senegal (1998–2010).
The higher malaria transmission is recorded in Senegal during October. The evolution of the transmission cycle is remarkable, but summarized at the end of the rainy season, as it can be also observed in Fig. 12.
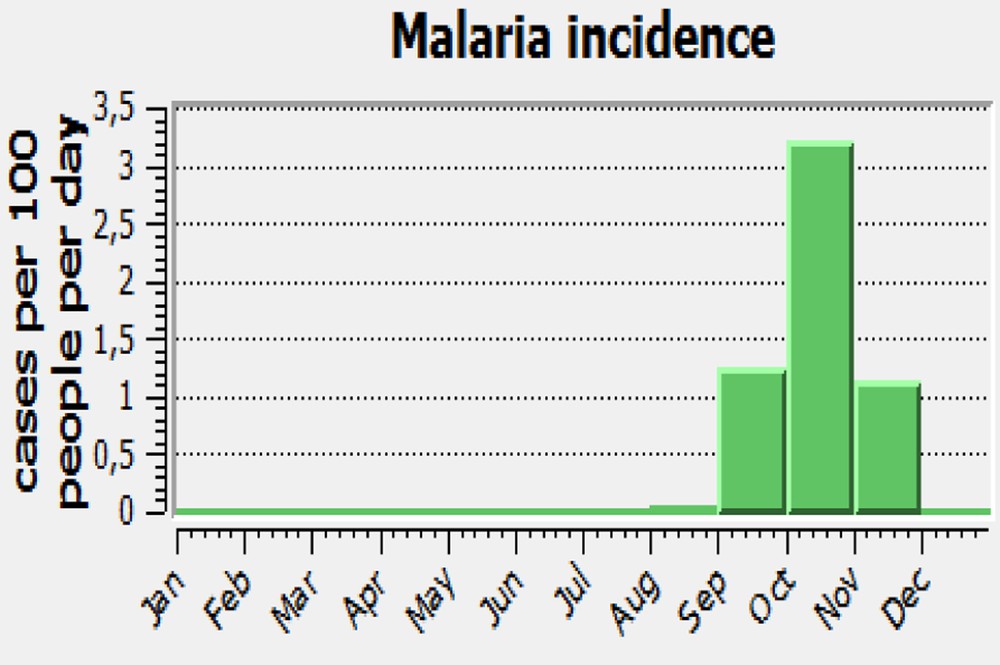
Annual cycle of malaria incidence simulated using climate parameters over Senegal (1998–2010).
October, the month during which a high malaria transmission in Senegal is recorded is also highlighted. The evolution of the transmission cycle, remarkable but taking place mainly at the end of the rainy season, is also displayed in Fig. 12.
Other indexes of malaria such as the length of the transmission cycle (e.g., LTS, length of malaria transmission season) and CS (climate suitability) can also be simulated with LMM and MARA models coupled with regional climate models such as HADGEM (GCM2) and IPSL (GCM2), over Africa and Senegal.
For all climate change scenarios provided here, validation data are those from CRU for the period from 1960 to 1999. Figs. 13 and 14 show the distribution of LTS over Africa and Senegal. Fig. 13 illustrates that Sub-Saharan Africa is the region most affected by endemic malaria. The LTS does not exceed more than two months in the extreme North and South of the continent. For Senegal (Fig. 14), the transmission takes place during the rainy season, mainly toward the end. We also notice that the LMM2010 version overestimates the LTS with new parameters considered in the current version. For the CS (Figs. 15 and 16), also, the observation is the same, e.g. the Sub-Saharan is more affected with high values of CS and for the case of Senegal, the maximum of CS is found over the southern area. It is important also to represent all models and their different simulations, and make an emphasis on Senegal, in particular on the Ferlo. For future simulations, the LMM model needs to be coupled with a regional climate model. A set of models was tested by comparing simulations with observations (blue curve) over the period 1979–2000. Fig. 18 shows that models GFDL2.0, GFDL2.1, GISS and MUIB overestimate the peak of precipitation compared to observations, while IPSL, CNRM and MRI underestimate it. Data from ECHAM5, CSIRO and CCCMA approach better the observations. The best model of this set could be identified for coupling with the LMM.
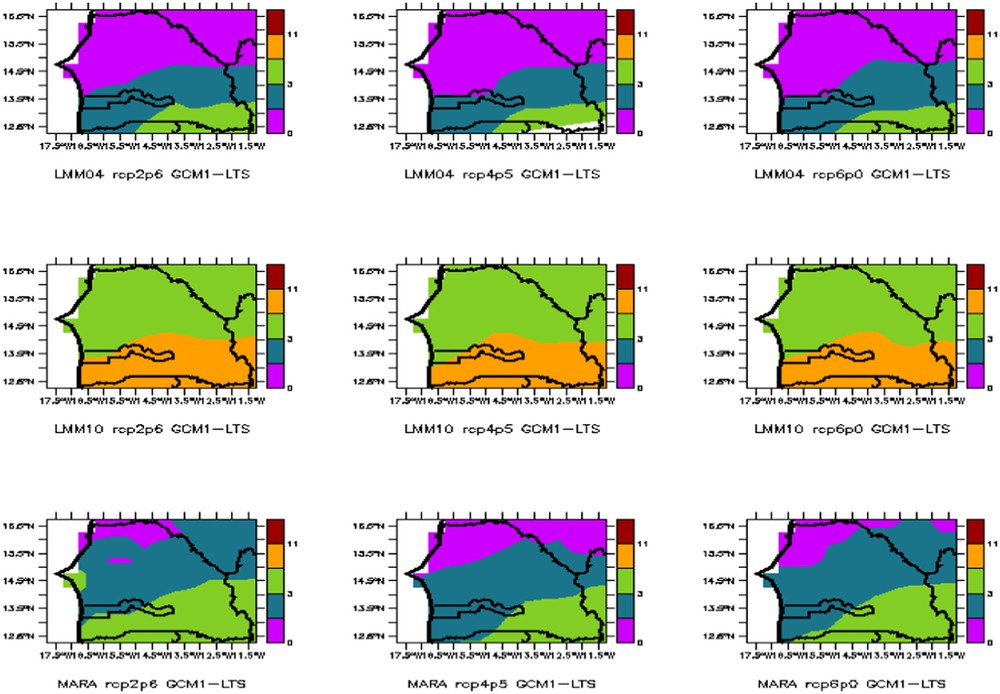
Length of the seasonal cycle of malaria over Senegal simulated for the period 2006–2035 by biological models LMM and MARA coupled with climate models HADGEM (GCM1) and IPSL (GCM2).
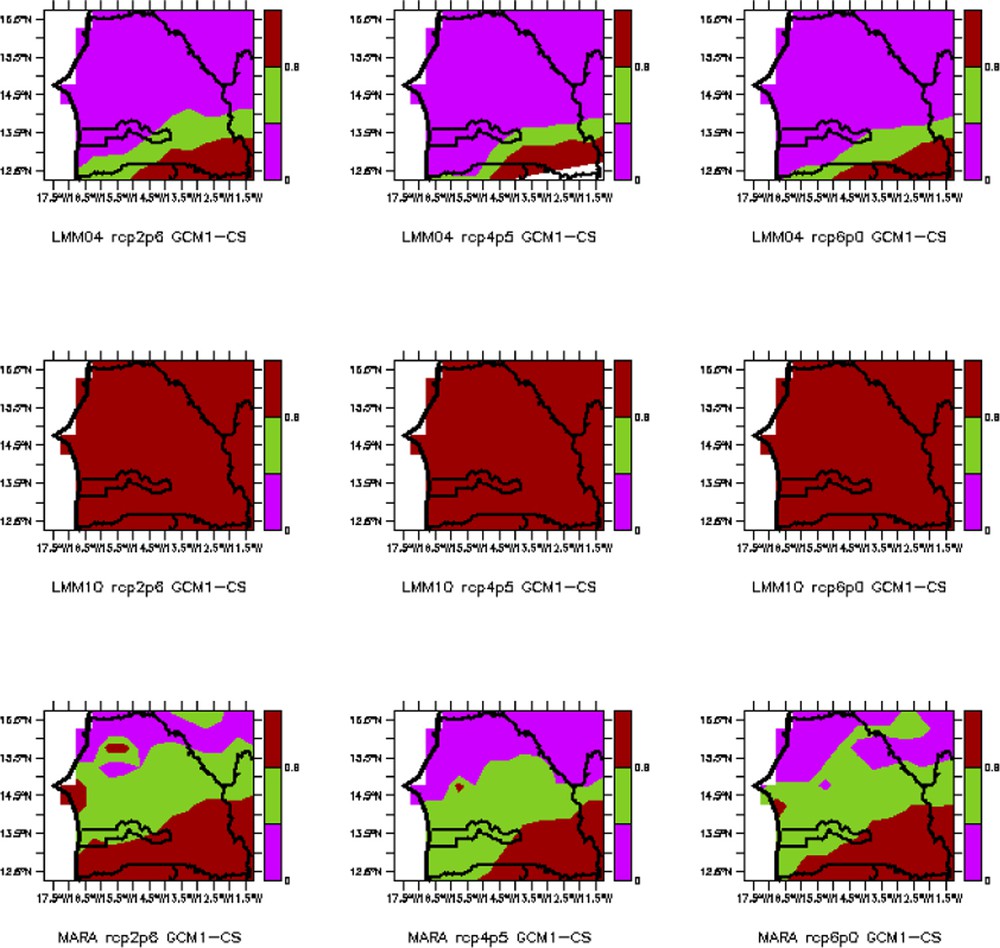
CS (climate suitability) of malaria over Senegal simulated for the period 2006–2035 by biological models LMM and MARA coupled with climate models HADGEM (GCM1) and IPSL (GCM2).
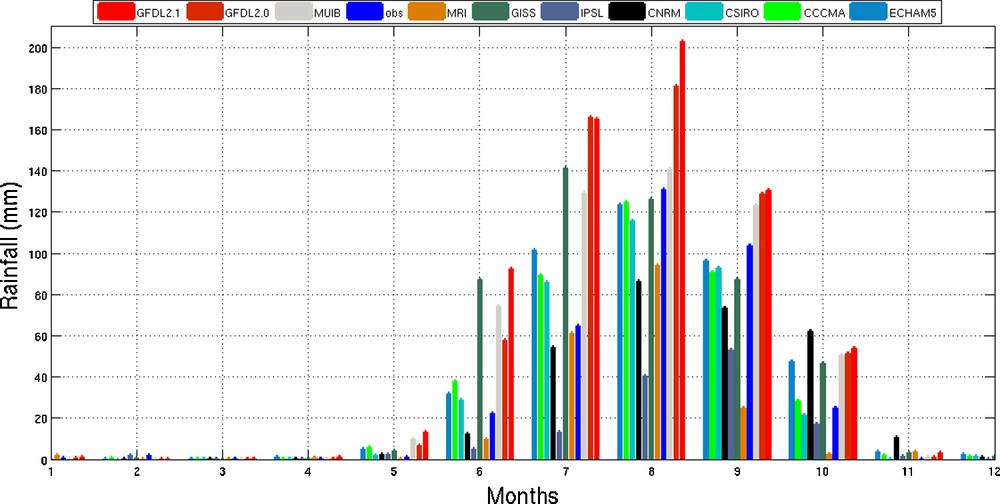
Evolution of the average annual rainfall cycle in Ferlo, comparison of observed data with simulations of various models for the period 1979–2000.
In Figs. 17 and 18, the annual cycles of precipitation and temperature in the Ferlo are studied with ten models to compare simulated and observation data over the same period 1979–2000.
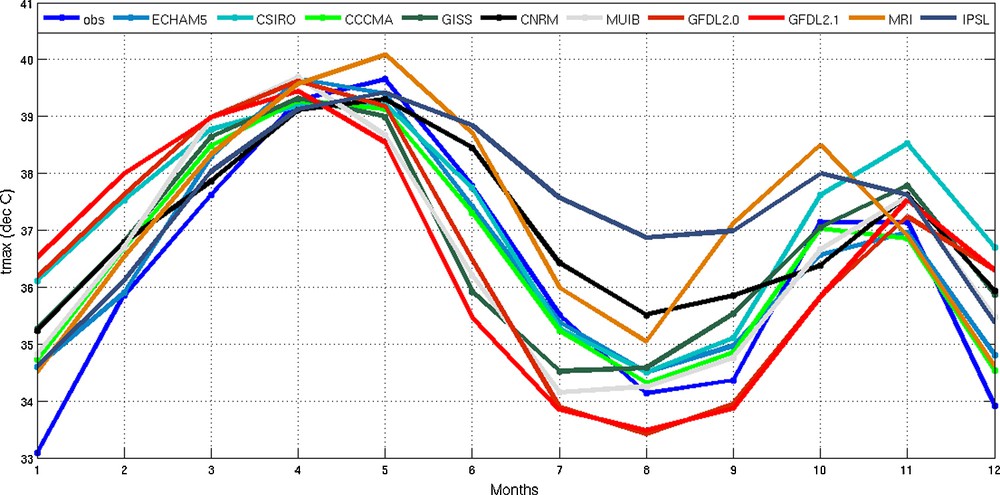
Evolution of the average annual temperature cycle in Ferlo, comparison of observed data with simulations of various models for the period 1979–2000.
The annual cycle is compared in Figs. 19 and 20 between models and observations, showing how some models overestimate the maximum, while others underestimate it. ECHAM5, CSIRO and CCCMA simulations are close to the observations (blue curve). These figures show that GFDL2.0, GFDL2.1, GISS and MUIB overestimate the observed peaks in the rain, whereas IPSL, CNRM and MRI underestimate it.
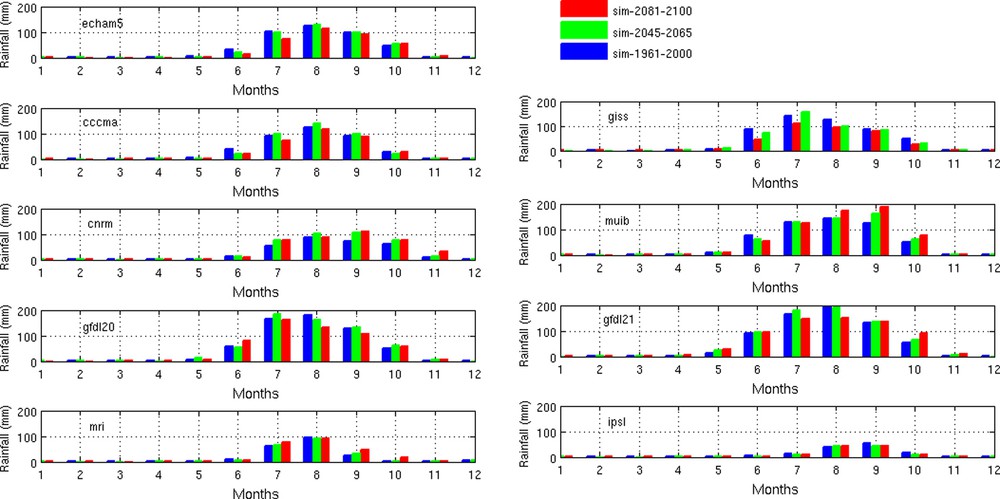
Evolution of mean monthly rainfall in Ferlo simulated for the recent past period (1961–2000), the near future (2046–2065) and the far future (2081–2100) by various models, ECHAM5, CCCMA, CNRM, GISS, MIUB, GFDL2.0, GFDL2.1, MRI and IPSL, models.
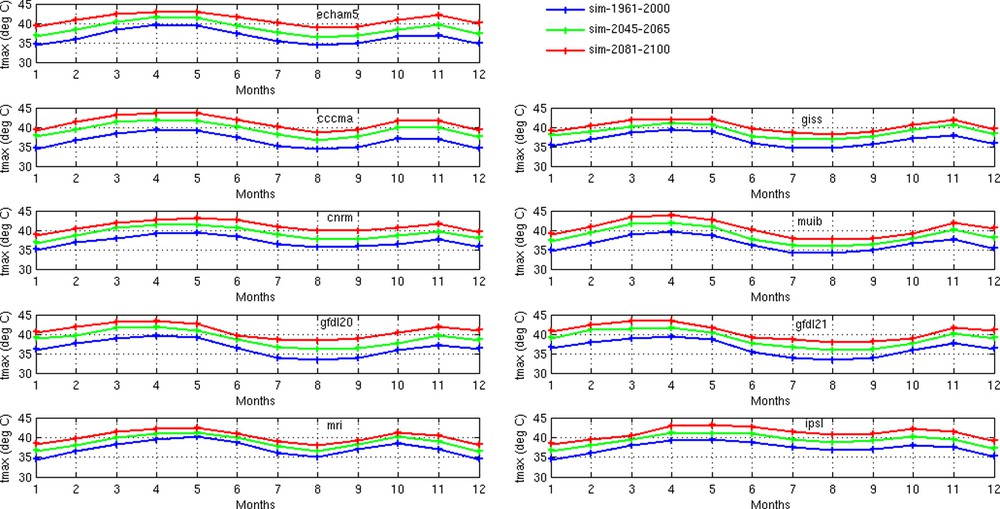
Evolution of mean monthly maximum temperature in Ferlo simulated for the recent past period (1961–2000), the near future (2046–2065) and the far future (2081–2100) by various models, ECHAM5, CCCMA, CNRM, GISS, MIUB, GFDL2.0, GFDL2.1, MRI and IPSL, models.
The two maximum temperatures in May and October are also found with the simulation data of these models. This comparison aims to identify the best climate model that has to be chosen for coupling with the LMM.
Simulations of the same models are used to predict rainfall and temperature settings based on climate change scenarios for the near (2046–2065) and far future (2081–2100).
The A2 scenario is based on the assumption that countries are more autonomous in their lifestyle with a more divided world, a growing population and rapid economic development, and regionalized. A2 is considered as a “pessimistic” scenario.
The B1 scenario is characterized by an integrated economic development, but also a growing population that will reach nine billion by 2050, before experiencing a decline. It is also based on advanced technology and a particular emphasis on global solutions to social, economic and environmental problems. B1 is considered as an “optimistic” scenario.
For the temperatures provided, there is an increase compared to the period (1961–2000). These growth temperatures persist in the distant future (2081–2100) with peaks that may exceed 43 °C, estimated with different models.
For precipitation, some models predict a decline, while others show an increase. In both cases, the difference in rainfall variability is less important than the temperature increase.
4 Conclusion
Our research on spatial and temporal variability of climate parameters such as rainfall and temperature, and their impact on the seasonal malaria incidence risk, is an important component in QWeCI project. The main issue is to understand and show the links between seasonal vector-borne diseases and climate variability. In our case of study, we have analyzed the links between malaria and climate variability in the Ferlo for the present and future projections. The study was mainly carried out in the Ferlo (Senegal) observatory, and extended to the Sahelian and West African areas in order to consider the large scale also. The study is based on observations and simulations based on a monitoring database. For this reason, we use the Liverpool Malaria Model (LMM) but also its interface Disease Model Cradle (DMC) for our local application framework for running the LMM.
As it is shown with our observations in conjunction with the Programme national de lutte contre le paludisme au Sénégal (PNLP), but also with the LMM and DMC simulations, the high malaria transmission is concentrated towards the end of the rainy season in Senegal. The rains in the Ferlo and Senegal are generally characterized by a strong seasonality with a maximum frequency in August, and a strong interannual variability for all the West African and Sahelian regions. Moreover, a relationship between the observed rainfall variability and the malaria incidence transmission risk during the following months can be noticed. This shift from one to two months is consistent with the knowledge of the parasite sporogonic cycle, the vectors’ livelihood and the parasite–vector–human interactions.
Preliminary results obtained are quite interesting; but we need a complete climate and clinic database in a spatial and temporal scale. Our observations on the climate over the Ferlo and its relationship with the risk of malaria emergence encourage the idea of modeling applied to climate–health interaction research.
Preliminary results have been presented as talks and/or posters during national and international official scientist events, such as the QWeCI international workshop in Dakar (Senegal) in 2011, the 4th AMMA conference in Toulouse (France) in 2012, and the Panafrican ANSTS–COPED colloquium in Dakar, in 2012.
The main issue of the following work consists in considering CMIP5 and CORDEX or statistical model simulations with different scenarios and then in using them as inputs to force LMM for malaria projections evolution.
Also, the analysis of the LMM outputs and its predictability from oceanic variables, as changes in SST, are future works to be considered.
Disclosure of interest
The authors declare that they have no conflicts of interest concerning this article.
Acknowledgments
This study was funded by the EU project QWeCI (Quantifying Weather and Climate Impacts on health in developing countries; funded by the European Commission's Seventh Framework Research Programme under the grant agreement 243964). The authors of this paper present their warm thanks to the Project.
The authors also thank the Erasmus Mundus Action 2 Strand 1 Lot-15 (ACP) program whose scholarship awarded to Ibrahima Diouf permits the continuation of this research.