1 Introduction: targeting protein–protein interactions
Pharmaceutical R&D undergoes a decline of productivity as the number of new drugs approved by the FDA regularly decreases [1, 2]. Besides market forces and difficulties such as demand and competition, pharmaceutical R&D has become increasingly challenging. Advances in the understanding of disease mechanisms highlight that complex and multifactorial systems could be dissected in order to identify reliable, safe and effective medicines [3, 4].
The origin of diseases often lies in a complex network of biological interactions that need to be understood not only at a clinical level, but also at phenotypic and molecular levels. In that perspective, a wide range of ‘omics’ approaches (gene, RNA, protein, metabolism, etc.) have been developed and data are accumulating with the hope that they will become useful for personalized treatments [5]. Among them, proteomic approaches (including yeast two-hybrid, affinity purification coupled to mass spectrometry, and protein complementation assays) led to the establishment of protein–protein interaction (PPI) networks in different model species and several human cell lines. These data are centralized in open-access databases (reviewed in [6]) and are regularly augmented by novel high- and low-throughput experiments [7].
PPI networks are highly interconnected, some proteins behaving as hubs, and involved in a large number of interactions (on average, each protein has 5 partners and hub proteins can associate with more than a hundred partners [8]). A typical example of a hub protein is the human protein p53 shown as a central node in the network in Fig. 1 and which is found mutated in multiple types of cancers. These interaction networks reorganize upon stress and in numerous diseases. Mutations leading to the inhibition of protein functions can also strongly impact PPI networks, but it is still very difficult to predict how far a PPI network can be perturbed by such mutations. Predicting the phenotypical consequences of a mutation in various cell types or tissues also remains out of reach although some studies are progressing toward that goal [9–11]. Interestingly, protein mutants associated with a disease were found to perturb PPI networks in a much larger proportion compared to common variants (whose mutations were not shown to be associated with any disease) [7] suggesting that pathologies could likely be related to the perturbation of PPI networks.
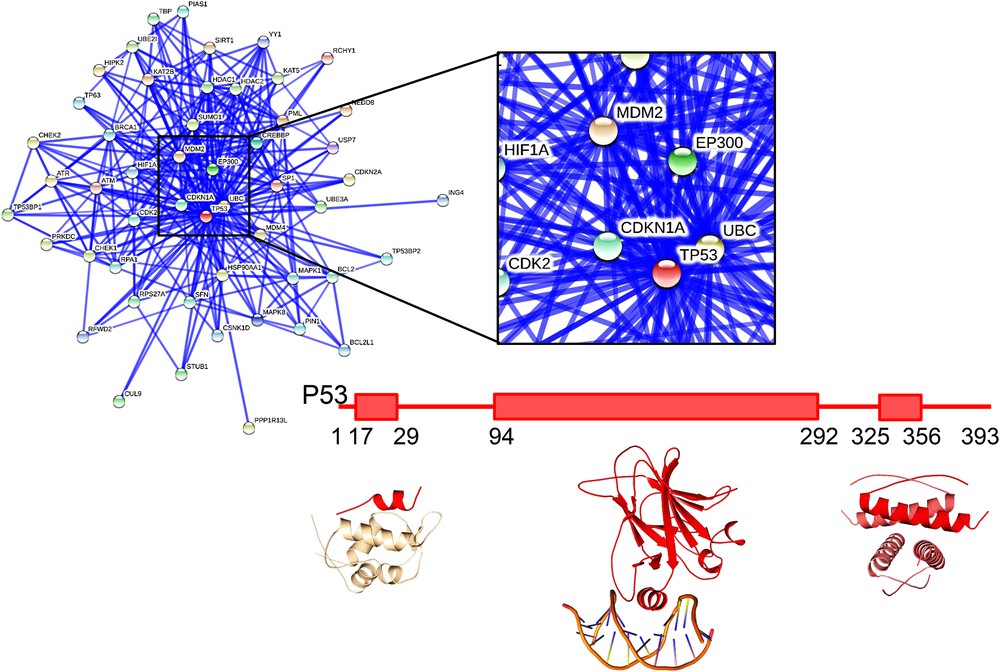
Interaction network and domain structure of the oncoprotein p53. Interaction network from the STRING database (http://string-db.org) for human p53. The lines indicate interactions between the proteins, with thickness of the lines reflecting confidence in the displayed interaction. The central region around p53 is zoomed in on. The domain structure of p53 and structures of individual domains in interaction with partners are also represented (from left to right: interaction with MDM2, pdb 1ycr [145], DNA, pdb 1tsr 8023157 [146], and self-association, pdb 1c26 [147]).
Deciphering the molecular logic associated with PPI networks remains a major challenge for the next decade, not only for fundamental research but also for pharmaceutical R&D. In this line, systematic knock-out or knock-down of genes has been performed at the genome scale in different model organisms of human cell types to analyze functional and genetic interactions between genes [12–15]. However, interpretation is hindered by the difficulty in disentangling the pleiotropic effects of protein depletion. Multiple pathways will likely be affected upon inactivation of a hub protein. In parallel, for decades, pharmaceutical R&D has generated molecules that are able to inhibit the catalytic activity of many protein targets associated with diseases or targeting G-protein coupled receptors (GPCRs) that are the starting point of major and complex cellular pathways. Such molecules can be used as tools to perturb PPI networks and evaluate the associated cellular response [4]. Historically, pharmaceutical companies concentrated their efforts on targeting GPCRs that are located on the surface of cells or intracellular enzymes because these targets present cavities surrounding their active sites and thus pre-existing small molecule binding sites. Yet, these targets often occupy a central position in PPI networks and their activity generally targets numerous substrates sharing common properties and distributed all over a PPI network. Consequently, enzyme-inhibiting molecules often block the pocket of a large set of substrates and affect many pathways downstream of the catalytic reaction (as is the case of kinases for example). In addition, inhibitory molecules can have limited specificity and inhibit not only the targeted enzyme but its family of paralogues leading to important side-effects [4].
An alternative to the pleiotropic effects of inhibitory molecules on highly interconnected nodes in PPI networks is the design of specific inhibitors of protein–protein interactions [16]. These tools not only help unraveling the complexity of interconnected networks, but also provide access to a much wider range of pharmaceutical targets (called PPI targets) [17]. Molecules inhibiting a single PPI are expected to act more specifically on biological pathways, improving the reliability of prognosis and reducing side-effects [18].
In this review, we will provide an overview of recent advances in the field of PPI inhibition. A number of issues are raised for identifying PPI inhibitors: the lack of starting molecules, the size and flatness of the surface to bind, the composition of current chemical libraries, the assessment of on-target effects. Over the past few years, these challenges have been tackled using a variety of strategies. We will first review the status of the field in the development of small molecules, and then discuss other tracks of research relying on inhibitory proteins. In the last section, peptide-based approaches will be presented, underscoring their specific advantages over the two previous approaches but also their current limitations.
2 The small molecule approach
It has been considered a difficult task to find small molecules competing with the binding of an intracellular protein partner. One difficulty in designing small molecules inhibiting a PPI is related to the size of the surface that should be covered by the molecule, while inhibiting an enzyme's active site is generally achieved through molecules that occupy most of the space available in the functional cavity of the enzyme. Compared to the active site of an enzyme or the binding site of a receptor, PPIs are stabilized through large interfaces formed by the burial of protein atoms, up to thousands of square angstroms [19]. In addition to the difficulty related to the size of the surface to compete with, PPI interfaces represent diverse and multifaceted binding sites that impose significant challenges in conceiving efficient inhibitors. Small molecule allosteric inhibitors, targeting another site of the protein and capable of inducing a conformational change leading to a loss of interaction with the targeted partner, were also described but they are not the majority [20].
A second difficulty associated with the search for small molecule PPI inhibitors, lies in the lack of catalytic activity to screen when compared to the enzyme inhibitor development, or the lack of simple functional assays for screening agonists or antagonists for targeting G protein related receptors (GPCR) [21]. In the case of PPI, high throughput screens (HTSs) had to be revisited. Thermal shift assays (measure of variation of the melting temperature of the protein alone and in the presence of a molecule) [22], SPR (surface plasmon resonance) [23], FRET/BRET (Fluorescence or Bioluminescence Resonance Excitation Transfer) [24, 25], Elisa (Enzyme-linked immunosorbent assay) [26], fluorescence polarization [27], and Far western [28] or other techniques (see [29] for a review), have been developed but suffer from a large set of false positives (due to protein aggregation, oligomer formation, and allosteric binding for example). More sophisticated (and lower throughput) biophysical methods that provide structural or thermodynamic details of the molecule binding mode (using isothermal calorimetry (ITC), Nuclear Magnetic Resonance (NMR), and X-ray crystallography) are then needed for further validation and characterization of potential hits. Alternatively, high throughput cellular screens measuring the loss of interaction using reverse two hybrid or BRET have been developed and offer the advantage of selecting only cell penetrable molecules [30, 31]. The latter approaches require further validation to assess the “on target” effect.
Favorable tracks for the development of small molecule PPI inhibitors emerged in the eighties from the energetic analysis of protein binding interfaces. Exploration of the contribution of different contacts established upon binding could be performed by alanine scanning of both partners. Based on this technique, it has been shown that some mutations of interfacial residues have little effect on the binding affinity of the two molecules while others have major destabilizing effects [32–34]. Residues which largely contribute to the binding affinity have been called “hot-spot” residues. A direct assumption from the “hot-spot” analyses was that a small molecule interacting at the position of hot-spots should compete with the binding of the cognate partner without necessarily covering the whole surface of interaction (Fig. 2).
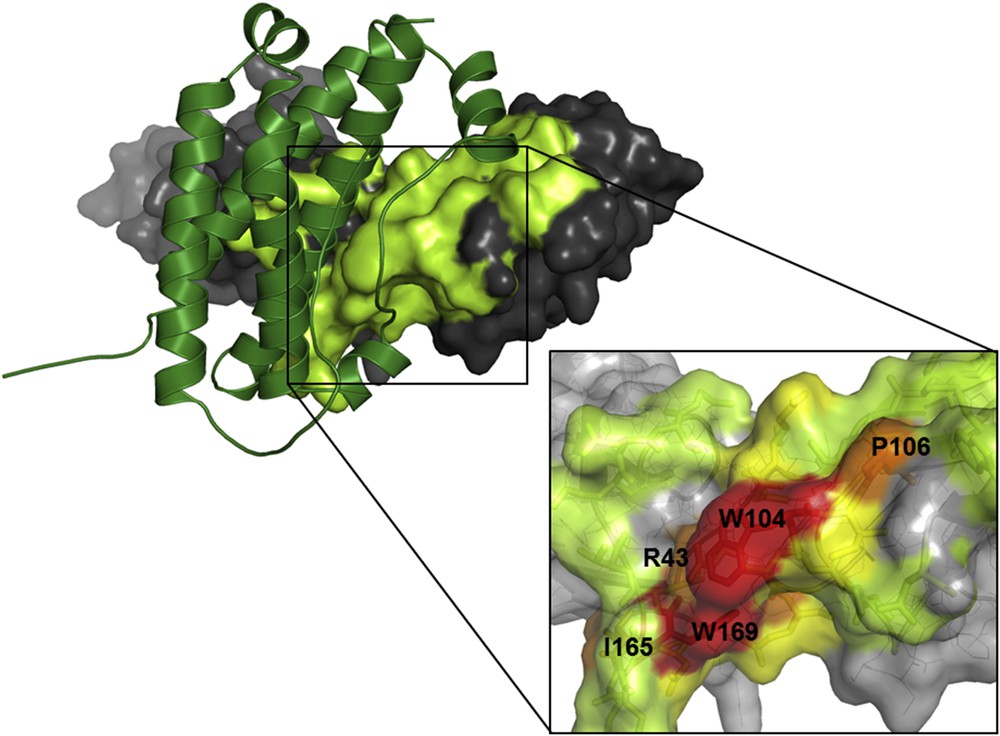
Hot-spots in protein–protein interfaces. Structure of the human growth hormone (in green ribbon) and extracellular domain of its receptor (on the surface with gray and light green color), pdb 3hhr [33, 148]. The binding interface of the receptor moiety is colored in light green. In the zoomed panel, hot-spots found experimentally are shown in red, orange and yellow depending on the loss of binding energy associated with the mutation of the residue (red: more than 4 kcal mol−1, orange: more than 2 kcal mol−1, yellow: more than 1 kcal mol−1).
The experimental determination of hot-spots remains however tedious and time consuming even if higher throughput approaches have been developed since the early alanine scans [35]. Based on the structure of two partners forming a complex, several groups developed computational methods for the prediction of hot-spots. These methods rely on different strategies that can be divided into three main categories: methods based on dedicated energy functions (such as FoldX, Rosetta, and PCRPi [36–38]), methods based on molecular simulations [39], and methods relying on machine learning algorithms (see for example HSpred [40], and HotPoint [41]). On an average, these methods present a success rate of about 80% in their predictions and combining them can even improve the accuracy of the hot-spot prediction (see [42] for a review).
As a positive step for the development of small molecule PPI inhibitors, the position of hot-spots correlates with the position of protein multiple binding sites. These sites are defined as residues at the surface of a family of protein homologs, bound either to small molecules or to another protein in the protein data bank (PDB) [43, 44]. They correspond to “ sticky zones ” of proteins playing a central role in stabilizing interactions with the natural protein partners and also prone to bind small molecules. These sticky zones have recently been shown to also concentrate the binding energy of inhibitors, opening promising developments for the rational selection of binding molecules [45, 46].
Hot-spots are generally clustered at the center of small to medium size binding interfaces (250–900 Å2). This property facilitates their targeting by small molecules. A typical example is the inhibition of bromo-domains [47]. These domains specifically bind linear protein motifs containing acetylated lysines. The modified residue (acetylated lysine) is inserted into a well-defined pocket at the surface of bromo-domains and is recognized by strictly conserved residues. Residues surrounding the pocket participate in the binding affinity and specificity (Fig. 3). Inhibitors of bromo-domains have recently been developed with remarkable success [48–51] (see [52] for a review).

Small molecule inhibitor of the interaction between bromo-domains and acetylated histones. A: Structure of the first bromodomain of human BRD4 in interaction with a diacetylated histone H4 peptide H4K5acK8ac), pdb 3uvw [149]. The bromodomain is shown in a surface representation (in gray and light pink for residues in contact with the histone peptide). The histone peptide is shown in purple sticks. B: Structure of the first bromodomain of human BRD4 in interaction with the small molecule inhibitor JQ1, pdb 3mxf [48]. The surface of the bromodomain in contact with JQ1 (in red sticks) is colored in dark pink. C: Chemical structure of the small molecule JQ1 [48].
In larger protein–protein interfaces, hot-spots can be more distributed over the interface with significant distance between them. They also cover a more complex combination of chemical functions, increasing the difficulty of finding a molecule interfering with all the hot-spots. As a first step in the development of computational tools for selecting PPI inhibitors, several groups analyzed the chemical properties of the growing number of small molecule PPI inhibitors already discovered. Until now, more than 40 PPIs have been successfully targeted, and at least 3 databases are dedicated to modulators of PPI: the 2P2I database [53, 54], TIMBAL [55, 56] and iPPI-DB [57]. PPI inhibitors exhibit a significantly larger size and molecular weight than traditional drugs (MW > 400 Da). PPI inhibitors are also more hydrophobic with a ALogP > 4; they contain a larger number of hydrogen bonds (HBA > 4) and at least four rings [58–60]. These chemical characteristics are clearly different from those usually used for selecting drug-like compounds, the so-called Lipinski rules [61]. Such deviation from the classical characteristics points out the necessity of assembling banks of molecules dedicated to PPI screenings, containing a higher chemical diversity and greater complexity than banks used for traditional drug design. Further exploration of natural products should typically help diversifying and complementing such banks of compounds [62]. Computational tools dedicated to the search for PPI inhibitors were also combined with experimental screens to accelerate and reduce the cost of screens. This strategy was particularly powerful in cases where no previously known inhibitor could be used as a starting point in a structure-based research program [63–65].
An alternative approach to the complexification of banks of compounds is the so-called “fragment approach”. Instead of selecting a starting molecule of relatively high molecular weight, it consists of searching a set of simple small molecules (fragment) with low affinity for one of the partners and builds the inhibitor step by step through a structure-based strategy [66–69]. For this strategy, identification of binding hot-spots is particularly useful to define crucial positions at which a molecule could bind to efficiently compete with the cognate partner. Because the affinity of the first selected compound is expected to be rather low, screens based on inhibiting a specific PPI as mentioned above are not the most adapted. Direct structural screens in primary screens using X-ray crystallography or NMR have been successfully employed. The starting molecule can then be directly selected based on its binding site. Even if the affinity of a starting compound typically falls in the milli-molar range, it was found to be enough for starting an optimization procedure by expanding the molecule size while simultaneously optimizing the binding affinity [68, 70]. Crystal contacts can also be used to find new binding sites [71], and increase the molecule size by tethering fragments. Another successful strategy to select a first fragment was to tether the molecule via an SS bond at a position close to the targeted PPI interaction [72, 73]. In this strategy, the presence of a covalent bond between the target and the initial fragment compound can help obtaining the structure of the complex [74].
3 The protein approach
When the targeted interaction includes a large PPI interface, alternatives to the small molecule approach were also envisaged. A first category of developments was the conception of monoclonal antibodies competing with the PPI formation. This approach, necessitating the ‘humanization’ of immunoglobulins to prevent an immune reaction, was remarkably successful, so that each year, tens of new antibodies started clinical trials [75] or reached FDA drug approvals [76–78] for therapeutic applications or diagnostic purposes. The first inhibitory antibody was designed using phage display (Fig. 4A) [79]. This technique rapidly allowed the design of thousands of tightly binding immunoglobulin domains with even higher affinity than those observed in nature [80]. Other approaches used immunization of humanized murine models [75]. The exploitation of simpler immunoglobulin systems such as the nanobodies found in camelids should give rise to more stable and simpler molecules (Fig. 4B) [81, 82]. Such nanobodies were found to be powerful for targeting G-protein coupled receptors (GPCRs) and used as research tools, diagnostics or therapeutics [81]. Scaffolds other than the immunoglobulin fold were also developed for targeting PPIs and protein binding in general. They include repetitive sequences also called artificial antibodies (Darpins, Fig. 4D, [83, 84], Alpharep, Fig. 4E, [85, 86] and also more diverse scaffolds, Fig. 4C [87, 88]).
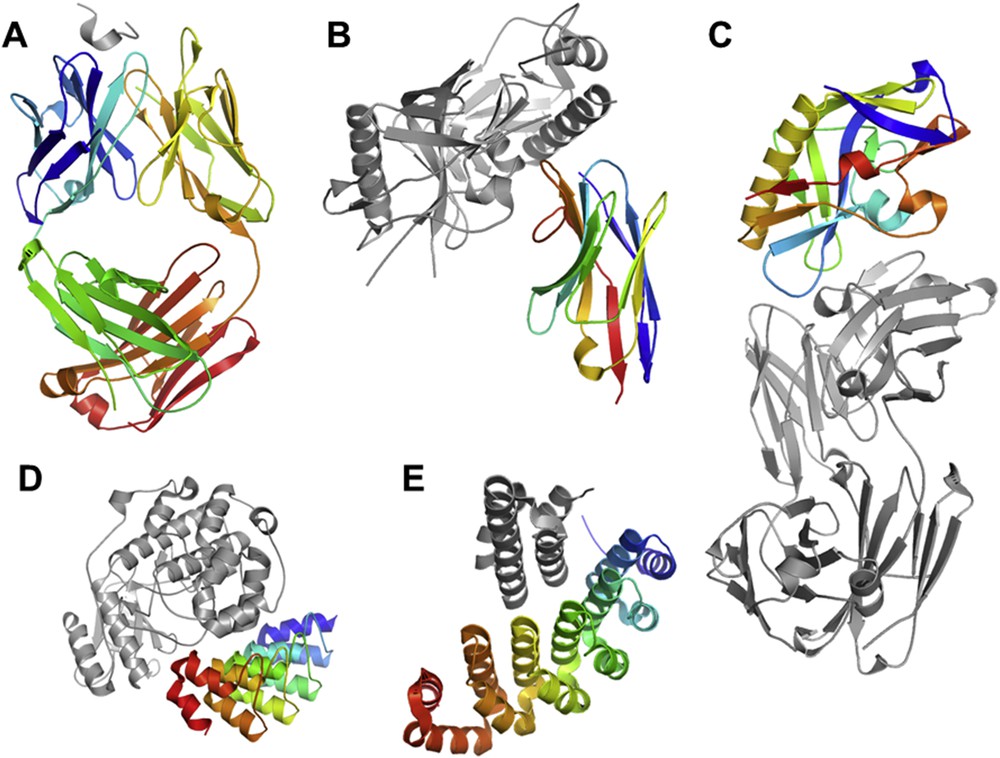
Structure of selected or designed proteins (in rainbow) bound to their target (in gray). A: Antibody against a 24-amino acid peptide from the third variable (V3) loop of human immunodeficiency virus-type 1 (HIV-1) gp 120, pdb 1acy [150]. B: Structure of the anti-HIV A12 VHH of llama antibody in complex with C1086 gp120, pdb 3rjq. C: Structure of the viral HIV-1 gp120 protein engineered for binding the neutralizing antibody, pdb 4jpk [90]. D: Selected ankyrin repeat binder of the kinase ERK, pdb 3zu7 [83]. E: Structure of an artificial helicoidal repeat protein (alphaRep) selected for binding an helical synthetic protein, pdb 4jw2 [85].
Computational methods also contributed significantly to this field. Modeling and design tools such as the Rosetta suite have reached sufficient accuracy to be able to guide the rational design of binders [89]. The combination of rational design strategies with state-of-the-art display technologies such as ribosome or yeast display coupled to deep sequencing technologies open great perspectives for the diversification of the classes of binders [88–91]. Computational methods can also be used to reduce the immunogenicity of designed proteins [92].
Natural, engineered or artificial antibodies designed for PPI inhibition were generally conceived to compete with the cognate partner (orthosteric mechanism); but in a few cases, they also act via an allosteric mechanism [93]. However, the latter mechanism of action is difficult to rationalize or predict because it implies subtle conformational changes in the targeted protein.
Finally, the major limitation of antibodies, nanobodies and other proteins selected for tight binding of a target remains to be the difficulty to bring these proteins into cells. Their applications remain mainly limited to extracellular targets.
4 The peptide/peptidomimetic approach
Peptides are natural compounds sufficiently large to potentially block an interaction and hit several hot-spots. However, when targeting intracellular PPIs, inhibitory peptides will have to cross the cell membrane. Major advances have been made in the elaboration of the so-called cell penetrating peptides (CPPs) which brought significant progress for the development of intracellular peptide-like drugs. Numerous academic laboratories, but also a growing number of companies, are working on different methods for developing cell-permeable or potential PPI inhibitor peptides [18]. The capacity of particular peptide sequences to penetrate cells was first discovered in small proteins acting as neurotransmitters (penetratin, [94]) and viral proteins (tat [95]). The penetration mechanism was then intensively studied, highlighting a variety of mechanisms depending on the type of CPP that are still under investigation [95–98]. Entry into cells involves endosomal uptake but also direct membrane crossing. CPP properties were transferred to active inhibitory peptides by adding a CPP sequence at the N or C-terminus [99] or by modifying the peptide's positively charged residues at positions that do not interfere with the binding of the target [100]. Penetrating sequences were varied and optimized [101–103] and also introduced from chemical modifications [104].
One point of great interest about the peptide strategy is that the affinity of the peptide for the target can be improved by methods already developed for proteins or antibodies, i.e. molecular biology, directed evolution, computational design tools, etc. The latter computational approaches need specific protocols, considering the increased flexibility of peptides compared to globular proteins [105–109]. Another advantage of the peptide strategy is the simple synthesis step which benefits from decades of solid-phase chemical synthesis developments. High-throughput approaches, such as peptide arrays, for the detection of first peptide hits or the investigation of peptide selectivity for homologous targets can thus be easily set up [110, 111].
The main limitation of peptides as drugs remains however their high susceptibility to proteases in vivo, even if a rapid internalization was shown to bring partial protection [112]. To overcome this obstacle commonly associated with peptide-based drugs, a large set of chemical modifications have been presented in the literature, containing increasing deviations from the natural peptide scaffold, with some remarkable successes for PPI inhibition. Introduction of non-natural side-chains was performed to increase the affinity by optimizing contacts for the target while increasing solubility or limiting proteolysis [113]. D-aminoacids can be introduced at some positions with limited perturbations for the peptide conformation [114, 115]. Peptide cyclization has also been performed through the design of simple cyclic peptides [113, 116] or the introduction of staples (Fig. 5A). Helix staples were shown not only to stabilize helical conformations but also to favor cell penetration [104,117–119] and protect peptides from proteolysis. More divergent from natural peptides, oligomers of non-alpha-amino-acid backbones have been designed to preserve their capacity to form well-defined compact structures. These so-called foldamers [120] can be designed to maintain the position of anchor residues for interacting with the protein target (Fig. 5) [119,121–127] while exhibiting more than 100-fold higher resistance to proteolysis [119,124–128]. Even less peptide-like, numerous strategies of non-peptidic foldamer alpha-helix mimetic scaffolds have been presented in the literature, such as terphenyl-based, terephthalamide-based, and Aryl-linked imidazolidin-2-ones[129] or various oligoamide foldamers [130, 131] (Fig. 5). These molecules successfully inhibited PPIs, such as calmodulin and small muscle myosin light chain kinase [132], Bax-BclXL [133, 134], the VEGF receptor [135] or p53-mdm2 [136], see [123, 137, 138] for reviews. Interestingly, synthesis of foldamers can be, in some cases, adapted to solid-phase synthesis, facilitating their production and purification [139, 140]. In addition, computational tools are currently developed for the rational design of foldamers [141, 142]. All these efforts should pave the way for inhibiting any PPI target with no limitation in the size and composition of the interface to compete with.
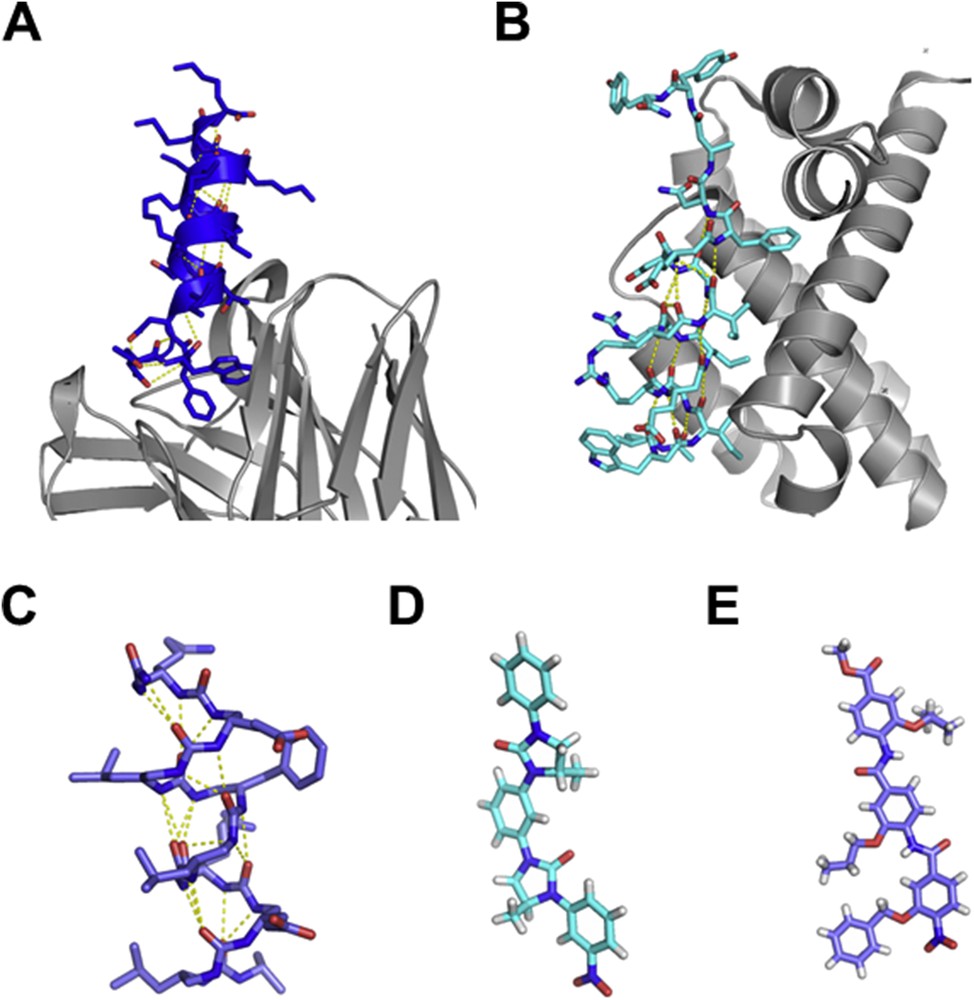
Structure of helical peptidomimetics. A: Crystal structure of the HIV-1 neutralizing antibody 4E10 Fab fragment (in gray ribbon) in complex with a hydrocarbon-stapled peptide (in blue sticks), pdb 4nhc [118]. B: Analogues of the Bim BH3 domain with an α/β-backbone pattern (in light blue sticks) in complex with Bcl2-like protein 1 (in gray ribbon), pdb 4a1u [151], C: urea based helical structure [121], D: Aryl-linked imidazolidin-2-ones as non-peptidic β-strand mimetics [129], E: functionalised aromatic oligamide as helical mimetics [130].
5 Conclusion and further challenges
Inhibiting protein–protein interactions has become a reality for a significant number of examples and could be envisioned as a more general strategy for deciphering the molecular logic associated with PPI networks, or for therapeutic applications. Depending on the size of the targeted interface, the affinity of the interaction and the position of hot-spots, different strategies can be envisioned. Small molecules are more suitable for small and compact interfaces whereas inhibition of large interfaces is more likely achievable by using peptidomimetics. Antibodies or artificial binders could be considered the approach of choice for targeting extracellular receptors. Remarkably, these strategies are not always independent, since efficient peptidomimetics or small molecules were successfully derived from peptides (as for example the mdm2-p53 interaction [113, 142, 143], or the Notch complex [104]), but also from antibodies [116, 118]. In some examples, peptides were used as first molecules to validate the target and facilitate the search for active peptidomimetics or small molecules [144].
Some important challenges still remain. Regarding the exploration of PPI networks, high throughput data including dynamic and spatial information of PPIs could help to unravel molecular mechanisms associated with PPI networks. Modeling tools are also to be developed, at least for simple networks, to be able to exploit all these high throughput data. An open question regarding the huge amount of data that can be generated by high throughput approaches is whether it is reasonable to collect HTS data in all tissues of each patient.
Considering the search for PPI inhibitors, enlarging the chemical space for efficient screening of small molecules is needed, and grouping together dedicated banks should be favored. Nucleic-acid aptamers could also be selected to bind protein surfaces and inhibit protein–protein interactions. Developments for the design of a broad range of peptidomimetics are still in progress and should contribute to increase the chemical space of molecules for targeting PPIs. A major obstacle for such molecules could be the immunologic response. New delivery systems avoiding broad distribution, delivering to the right target, and favoring transport across biological barriers will also have to be further developed. Finally, it has to be mentioned that, if disruption of PPIs is now considerably progressing, molecules able to restore a PPI lost due to a pathogenic mutation are still restricted to exceptional cases. Such molecules would be of great interest to develop new drugs in genetic diseases and this is probably a forthcoming important challenge in the field.
Acknowledgments
We thank R. Guerois and J. Andreani for discussions and reading of the manuscript. This work was supported by the CEA (Radiobiology program), by the Agence Nationale de la Recherche (CHAPINHIB 2013), and by the French Infrastructure for Integrated Structural Biology (FRISBI) ANR-10-INSB-05-01, and MB was funded by the canceropole, and La Ligue contre le cancer.