1 Introduction
An impressive amount of detailed information has been gathered over the past decades on how external stimuli activate plasma membrane receptors, how they translate to the activation of linear downstream signalling cascades and eventually affect cell fate. Recently, the advent of highly sensitive proteomic methods has produced maps of protein interactions and led to the reconstruction of biochemical networks [1]. As a consequence, it is now widely accepted that signalling pathways are organized as coordinated communication networks in which multi-protein complexes process and integrate the signal fluxes. Now, the challenge in cell signalling is to understand the behaviour of these intertwined communication networks in order to decipher the cellular language [2]. G protein-coupled receptors (GPCR) represent the largest class of membrane receptors. They are capable of binding a wide diversity of molecules that regulate most physiological processes and are involved in a plethora of diseases. Noteworthy, GPCRs have long been preferential targets of therapeutic research and development and they currently account for up to 50% of marketed drugs [3].
2 The growing complexity of GPCR-induced signalling
Classically, upon ligand binding, GPCRs undergo a conformational change that leads to heterotrimeric G protein recruitment and activation, followed by the generation of diffusible second messengers such as cAMP (cyclic Adenosine Mono-Phosphate), calcium or phosphoinositides. However, it is increasingly recognized that GPCRs trigger multiple signalling pathways which lead to the formation of signalling networks [4] (Fig. 1). For instance, some GPCRs have the ability to couple to multiple G protein subtypes [5] and many GPCRs directly interact with non-G protein signalling effectors through specific protein–protein interaction domains [6]. But quite remarkably, outside of heterotrimeric G proteins, only two protein families are able to specifically interact with the majority of GPCRs in their activated conformation: G protein-coupled receptor kinases (GRKs) and β-arrestins [7]. Historically, GRKs and β-arrestins have been associated with the desensitization and internalization/recycling of most GPCRs [8]. However, recently, GPCRs have also been demonstrated to elicit signals, independently of heterotrimeric G protein coupling, through interaction with β-arrestins 1 and 2. Indeed, β-arrestins have been shown to act as multifunctional scaffolds and activators for a growing number of signalling proteins including ERK, p38, JNK, , Akt and RhoA [7,9–13]. Moreover, a recent proteomic study has reported as many as 337 protein interactions involving β-arrestins [14], strongly suggesting that they play a central role in the ability of GPCRs to activate very complex signalling networks. In addition, GRKs have also been reported to elicit signalling responses on their own right through protein/protein interactions [13]. Indeed, GRKs interact with a variety of proteins involved in signalling and trafficking such as , , , clathrin, GIT (G protein-coupled receptor kinase-interacting protein) and caveolin [15]. Phosphorylation of Raf kinase inhibitor protein (RKIP) by PKC displaces it from Raf and increases its association with GRK2 [16]. In addition, the physical interaction between GRK2 and Akt leads to the inhibition of Akt activity [17]. Finally, GRK2 and MEK1 have been found in the same multimolecular complex and this interaction is correlated with an inhibition of MEK activity [18].

GPCR-induced signalling networks. Schematic representation of the different transduction mechanisms leading to the activation of signalling pathways upon activation of a GPCR and how they define a complex signalling network. Cross-activations and cross-inhibitions between the G protein-dependent and -independent sub-networks are depicted as green and red arrows respectively. Gs, Gq, Gi and G12: different alpha subunits of heterotrimeric G protein; Gβγ: G beta and gamma subunits of heterotrimeric G proteins; other GIPs: other GPCR interacting proteins; β-arrs: β-arrestin 1 and 2. (For interpretation of the references to colour in this figure legend, the reader is referred to the web version of this article.)
Adding to this complexity is the fact that GPCR-induced signals can be spatially and temporally encoded. Signalling networks actively modulate the transmitted signals: negative feedback allows pathways to adapt or desensitize to persistent stimuli whereas cross inhibition is used to avoid crosstalk between pathways [19,20]. In addition to transmit qualitative information (e.g. the presence or absence of a stimulus), signalling pathways must also convey quantitative information about the strength of the stimulus (i.e. ligand concentration). It has been recently shown that signalling pathways can take advantage of their non-linear nature to convert stimulus intensity into signal duration [21]. Modulation of signal duration increases the range of stimulus concentrations for which dose-dependent responses are possible as dose-dependent responses are still possible after apparent saturation of the receptors. Another well documented example of spatial and temporal encoding in GPCR-induced signalling pathways is the dual activation mechanism of ERK by G protein and β-arrestins [22–26]. G protein-mediated ERK activation is rapid, transient and translocates to the nucleus. In contrast, the ERK activated via β-arrestins are slower in onset (∼5–10 min to reach maximum), very persistent () and are sequestered in the cytosol. Such spatial and temporal differences in GPCR-induced signals substantially increase the complexity of signalling systems, hence their processing power. Collectively, these features highlight the importance of considering the dynamic properties of signalling pathways when characterizing their behaviour.
3 Pathway-selective ligands for GPCR: A new era in drug discovery?
Interestingly, this emerging conceptual framework opens new research avenues for the development of therapeutics [27]. There is increasing evidence that some GPCR targeting drugs can selectively modulate a subset of the signalling events triggered by the full agonist. These effects have been given various names including “stimulus-trafficking”, “biased agonism”, “collateral efficacy” or “functional selectivity” [28]. Moreover, several GPCR ligands have already been reported to selectively activate or inhibit β-arrestin signalling [29–31]. Consideration of these new concepts might lead to the development of therapeutics with more selective actions, hence less side-effects. For instance, one drug, carvedilol, a β adrenergic receptor antagonist, has proven particularly effective in the treatment of heart failure. Interestingly, of 16 clinically relevant β adrenergic receptor antagonists, carvedilol displays a unique ability to stimulate β-arrestin-mediated signalling while preventing receptor coupling to Gs [31].
4 What added value can systems biology provide?
GPCRs' complex signalling mechanisms probably lead to context-adapted cellular responses relying on emerging system-level properties that cannot be predicted from the individual components of the induced networks. Therefore, it would be of paramount interest to provide a conceptual framework for deciphering and possibly predicting how an extra-cellular signal that activates a GPCR translates into a given biological or pathological response. This “global” level of analysis of GPCRs' biology is in its infancy. Pioneer work carried out in yeast has recently shown the value of systems biology for elucidating complex signalling mechanisms triggered by GPCRs [32,33]. Aspects of cell signalling and the mechanisms (i.e. feedback and feed-forward regulations) that regulate pathway activity triggered by GPCRs have been studied in yeast, and nicely illustrate how mathematical modelling can be used to understand the logic of various pathway architectures. In mammals, a system-level grasp of GPCR-mediated signalling networks would be a significant asset to rationalize and speed-up the discovery of new “pathway-selective” drugs. Indeed, the rate of new drug discovery using standard approaches, based essentially on heterotrimeric G protein-dependent activities, such as second messenger accumulation, has been slowing down despite increased investments by the pharmaceutical industry.
It has been proposed that computational modelling offers a powerful tool for examining GPCR pathways [34]. Such models can be used to better understand hypothesized mechanisms, run virtual (in silico) experiments, interpret data, suggest new drug targets, motivate experiments, and offer new explanations for observed phenomena. In the remaining part of the present review, we will identify and discuss the different challenges that the scientists in the field will be confronted with in their efforts to establish a systems biology approach to GPCR signalling.
Understanding the whole cell as an integrated system has been a goal for almost a century [35]. As in other fields of cell biology, deciphering GPCR signalling by at least descriptive, at most analytical methods has occupied the last 4 decades. Consequently, an enormous amount of data is published every year on some GPCR signalling aspects. However, these studies are highly heterogeneous in terms of the nature of the GPCR studied, the signalling pathway studied, the cellular system used, etc. As a consequence, it has been tremendously difficult to transform this huge amount of information into general concepts. An important effort of standardization and data sharing between laboratories in the field is needed. In addition, publicly accessible databases gathering and distributing standardized raw data related to GPCR signalling, especially dynamic data, would undoubtedly stimulate the modelling of GPCR-induced signalling networks.
5 Challenges in high throughput generation of signalling data
More recently, feeding system-level analyses with relevant high quality biological data have become possible thanks to new experimental techniques that allow large scale accumulation of unbiased signalling data (Fig. 2). Speeding up the production of new data sets and enhancing their quality is not only essential to feed model-building but also to allow testing of key model findings. Signalling events are often propagated within the cell by post-translational modifications involving protein–protein interactions and enzymatic activities. Noteworthy, reversible protein phosphorylation is centrally involved in signal transmission within cells. The comprehensive and quantitative analysis of the protein phosphorylation patterns in different cellular backgrounds is therefore critical to reach a system level analysis of cell signalling. Lately, high-throughput has spread to molecular biology and biochemistry, giving access to most items of information necessary for the comprehension of organisms' behaviour [36]. In particular, breakthroughs have been achieved in the isolation of phosphorylated peptides from complex samples, as well as in their analysis by mass spectrometry coupled to computational methods. Using such approaches, thousands of phosphopeptides and phosphorylation sites can now be identified in a single sample [37–39]. However, despite their unparalleled analytical power, mass spectrometry-based approaches present a static snapshot of cellular events; they do not allow the acquisition of dynamical phosphorylation data at large-scale and with high throughput. This limitation remains a major hurdle towards the development of powerful systems biology approaches in the field of cellular signalling. Over the last decade, the possibility to analyze the proteome using protein microarray-based methods has emerged into proteomics research, diagnostics, and drug discovery. In particular, automated spotting of concentrated and complex protein extracts permits their analysis with phosphospecific antibodies. This method, referred to as Reverse-Phase Protein Array (RPPA), uses very small quantities of biological material, which allows a wide sample collection with a high number of different antibodies to be screened [40–43]. Since it has a very high throughput, detailed kinetic experiments can be systematically carried out and analyzed. Moreover, the simultaneous quantification of thousands of samples achieved with RPPA drastically reduces data heterogeneity and variability which traditionally hamper modelling. Therefore, RPPA potentially represents a very attractive approach to capture the subtle and highly dynamic nature of phosphorylation cascades out of very large sample collections.

Schematic representation of three high-throughput methods particularly adapted to the model-building process in the context of GPCR-induced signalling. A. Reverse phase protein array (RPPA) relies on the ordered micro-arraying of cellular lysates. Specific signals are detected using a primary antibody-fluorescently-labelled secondary antibody revelation system. This technology is easy to automate and when coupled with the use of a repertoire of phospho-specific antibodies raised against signalling intermediates, it allows the analysis of signalling networks at an unprecedented throughput. B. Fluorescence resonance energy transfer (FRET) biosensors are genetically-encoded fusion proteins linking two fluorescent proteins with a peptide sensitive to either phosphorylation by a specific kinase or second messenger binding. Once altered by a signalling event, the linker undergoes a conformational change that modifies the relative positions of the two fluorescent proteins hence their resonance. Cells transfected with the sensor plasmid are analyzed using a microscope. The emission wavelength of the first fluorescent protein (generally CFP) corresponds to the excitation wavelength of the second fluorescent protein (generally YFP). Therefore, FRET can be quantified in living cells with exquisite time-resolution by using an excitation wavelength specific of the first fluorescent protein while recording the emission at the wavelength specific of the second fluorescent protein. C. Transfected cell array (TCA) consists of parallelized micro-spots containing a transfection reagent, a plasmid and/or a siRNA. Each spot is different and a very large number of them can be arrayed on one slide allowing large-scale screening. Cells are seeded directly on the slide so that they attach and grow on it while being specifically transfected by the genetic material underneath. The effect of each transfection can be analyzed for each spot individually based on morphology criteria, immunocytochemistry or reporter-gene activity.
Recently developed imaging approaches that use fluorescent sensors of signalling activities combine unmatched time and spatial resolution. Genetically encoded fluorescence resonance energy transfer (FRET)-based reporters have been used in living cells to monitor the spatiotemporal patterns of diffusible second messengers, kinase activities and GPCR activation [44–46]. Protein–protein interactions can also be analyzed in real time in living cells using either FRET or BRET (bioluminescence resonance energy transfer) [46–48]. When used in multi-well plate format, both FRET and BRET-based methods ensure the production of huge amounts of high content dynamic data which are very well suited to feed systems biology approaches.
If the ability to measure signalling outputs in a dynamic way is a key-point, so is the aptitude to control the inputs (i.e. agonist stimulation) both in time and concentrations. Chemical signalling in/between cells is crucial as it determines the cellular response, and is characterized by various time-scales ranging from a few milliseconds to several minutes [44]. However, with conventional experiments, mimicking in vivo conditions remains a challenge. The implementation of microfluidic devices might help suppress the limitations found with conventional approaches and allows multiplexed analysis with various stimulation patterns to be performed. Indeed, microfluidics offers the possibility of not only tightly controlling and modulating cell culture conditions but also of applying well-defined (in intensity, space and time) chemical stimulation (receptor ligands, inhibitors, etc.) [49,50]. This should notably facilitate an iterative dialog between computational modelling and “wet lab” experiments (i.e. prediction versus validation).
An important aspect when trying to decipher and model signalling networks is the ability to specifically apply perturbations and to measure the interactions of signalling pathways considered in the recent past as isolated entities (Fig. 3). In addition to classical approaches (e.g. kinase inhibitors, dominant negative constructs, etc.), interfering RNAs offer the unique opportunity to easily and specifically achieve gene knock-downs. Moreover, genome-wide siRNA screening are now available either in multi-well liquid phase or in transfected cell array format [51–53]. Large-scale siRNA screening may very well be an experimental breakthrough facilitating the edification of highly complex signalling networks.

GPCR-induced signalling systems can be specifically perturbed using different strategies: A. chemical inhibitors and toxins can be used to specifically block some of the proximal transduction mechanisms as well as some of the nodes of the downstream network; B. interfering RNA provide the unique possibility to knock-down any single gene putatively involved in the signalling network; C. when available, the use of signalling pathway-selective “biased ligands” provides a very interesting way to imbalance the proximal transduction mechanism allowing to measure the consequences on downstream network; D. alternatively, signalling pathway-selective mutant GPCR can be engineered; E. an interesting and potentially powerful way to favour the much needed iterative interactions between model-based predictions and experimental validations consists in applying tightly controlled agonist stimulation patterns to cells. Of course, this approach implies the use of adapted microfluidic devices; F. finally, genetically-encoded modulators can also be serviceable for instance to modify reaction stoichiometry by overexpressing a protein. Dominant negative constructs can also block a specific reaction or interaction.
6 Challenges in bioinformatics
Knowledge and data management is instrumental to systems biology. Indeed, high-throughput approaches generate huge quantities of heterogeneous data that cannot be handled with classical labbooks, or even flat files. Therefore, computational methods, standards and tools must be developed and used to tackle this problem. Beyond the handling problem, the design, validation and refinement of high fidelity models require the use of all available experimental and non-experimental data. To reach this objective, data produced by experimental biology and bioinformatics have to be accessible, in compatible formats, and easy to correlate. Experimental data management systems, called Laboratory Information Management Systems (LIMS) should allow biologists to capture all the data relative to an experiment (conditions, protocol, results). So far, the availability of a LIMS to manage all the pieces of information required has been the major stumbling-block of the design of models in systems biology.
Once the data and knowledge are available and organized, the first modelling step consists in the construction of a detailed representation of the studied system. For intracellular signalling networks, the reaction/interaction graph is commonly used. Its construction is achieved manually, in a hypothesis-driven manner by the expert, using prior knowledge of the system and chosen experimental data. Sophisticated data analysis and visualization tools, such as clustering, help in this task. However, when the system is very large, choices have to be made by the expert, and the resulting model is biased, incomplete and often fails to reveal emerging features from the studied network. The development of methods to automatically infer influence graphs directly from the ensemble of data would allow the production of unbiased, thus potentially very innovative models from large data sets. It is certainly a promising research area but to date, only a few and limited attempts have been reported [54].
7 Challenges in mathematical modelling
Currently, relatively detailed mechanistic models of GPCR-induced signalling network can be formalized using the systems biology Markup Language (SBML) for representing the elementary interactions [55]. In this initial formalization step, signalling networks can be either directly written in SBML or drawn using CellDesigner which provides an intuitive SBML-based graphical modelling environment [56,57]. Of note is the Biochemical Abstract Machine BIOCHAM [58] which can be used to compute the influence graph between molecular species from the reaction/interaction graph [59], formalize the (observed) biological properties of the system by temporal logic formulae, and automatically verify their satisfiability by model-checking algorithms. From the influence graph, temporal logics and model-checking algorithms have proven useful to express biological properties of complex biochemical systems and automatically verify if they are appropriate. This approach has allowed the analysis of reachability and temporal logic properties of signalling systems under various conditions [60].
Considering the highly dynamic nature both in time and space of GPCR-induced signalling pathways, a dynamic modelling approach is interesting and should yield more predictive power than static approaches. The aim of a dynamic model is to reproduce time-course experiments and provide intrinsic concentrations for molecular species that are experimentally unreachable. There are several dynamical modelling approaches such as ordinary differential equations (ODEs, population view), Petri nets (discrete and independent mechanisms), and pi-calculus (stochastic approach) [61,62]. For all those methods, kinetics parameters (activation rates, probability of transition) are needed to simulate the changes in molecule concentrations over time. Frequently, too many parameters are unknown and the initial large reaction/interaction graphs have to be reduced. Stability analysis of the model (study of steady states, bifurcation diagrams, etc.) can help to restrict the parameter space to biologically relevant areas and reveal important differences in reaction speeds therefore providing an accurate way to reduce the model. Despite its usefulness, stability analysis remains too limited to ensure sufficient model reduction on its own. In addition, the qualitative properties of the influence graph can help develop a reduced dynamic model amenable to numerical simulations and parameter optimization with respect to quantitative data [63]. This model reduction approach remains however mostly empirical and even if the main graphical properties of the network (i.e. positive and negative circuits in the influence graph, reachability properties in the reaction graph) are preserved, the simplification of the structure of the network may suppress some delays and may limit the repertoire of dynamic behaviours permitted by the original model.
Even though stability analysis can lead to the determination of some unknown kinetic parameters in function of the others, the number of remaining unknown parameters is often large. Thus, another central difficulty concerns the non-linear optimization techniques that are needed to infer the unknown kinetic parameter values from the experimental data obtained under various conditions. Several techniques of parameter learning by data/property fitting can be used, such as gradient-based methods, Monte Carlo methods, and the Covariance Matrix Adaptation Evolution Strategy CMAES [64]. The latter two approaches minimize the error by repeated random sampling. Once the unknown parameters have been optimized, simulations can be performed and provide all component quantities over time. Simulations can be performed with different stimulation patterns or with in silico perturbations (modifications of total protein amounts, suppression of pathways, modulation of kinetics parameters, etc.). Thanks to these perturbations, the robustness of the system can be appreciated. Indeed, robustness is classically defined as the error between the perturbed and the initial simulations. Interestingly, the formalization of the expected dynamic properties of the system in temporal logic with numerical constraints also makes it possible to quantify the robustness of the system with respect to some important properties [65]. This opens the way for integrating robustness criteria in the process of building the model.
8 Necessity of an iterative dialog between experimentation and modelling
The value of a computational model also needs to be assessed by its ability to fit all the available experimental observations made in either control or perturbed conditions. Next, it can be used to make in silico predictions. Simulations present the decisive advantage of providing the temporal evolution of all the constitutive molecular species involved, including those that are experimentally unreachable. In addition, it is easy to systematically perturb the signalling system in silico, for instance by suppressing one molecule or one reaction; the predicted effects can then be verified experimentally. Interesting predictions often result from this process. Another way to evaluate the predictive power of a signalling network model is to modify the agonist input to the system. Typically, different agonist concentrations or patterns (i.e. positive or negative gradients, pulsatile mode, etc.) can be applied in silico.
After initial experimental data gathering, a model is designed, parameterized and simulated. Predictions are made based on this initial model, and validation experiments are undertaken. In this initial phase, it often happens that predictions are not experimentally validated. In this case, the model has to be modified accordingly, and submitted to experimental validation again. This iterative process between modelling and experimentation is referred to as model refinement. According to this workflow, any prediction leads to either hypothesis validation or model refinement (Fig. 4). Thanks to this virtuous circle, the decoding of the intimate functioning of signalling networks' should move forward. Understanding how signalling pathways encode and transmit quantitative information about the external environment not only deepens our understanding of these systems, but will also lead to major achievements both in agriculture and medicine: models will point to molecular targets to be optimized in normal animal physiology and will help restore the proper function of pathways that have become deregulated in disease.
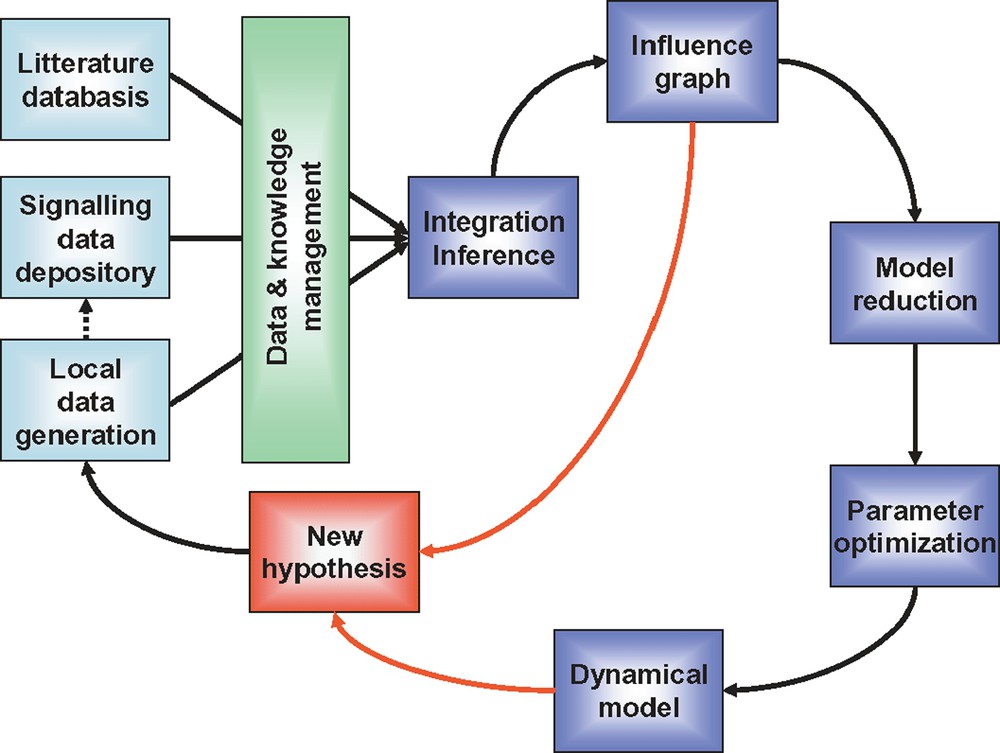
Proposed workflow to model signalling systems. Data are produced in the laboratory, using low and high throughput methods. Already existing relevant and carefully standardized data can be extracted from publicly accessible databases (note that, once exploited, locally produced data have the vocation to be deposited in such databases). Literature mining is also essential. Data and knowledge from these three sources are combined, organized, analyzed and made available for modelling using various bioinformatics tools integrated into a comprehensive LIMS (laboratory information management system). Influence graph is constructed and its qualitative analysis can lead to new hypotheses. In parallel, this initial model is reduced and parameters are optimized. The dynamical model can be analyzed and its predictive power evaluated. An iterative process then is engaged between experimentation and modelling which leads to novel findings and model refinement.
9 Conclusion
The acquisition of reliable data has long been the primary challenge in the field of GPCR signalling. Now, with the advent of high throughput technologies potentially able to generate an unprecedented amount of dynamic signalling data, and to take into account the growing complexity of GPCR signalling, the challenges are being re-centred towards more theoretical and conceptual aspects. State of the art bioinformatics and mathematics can already help better handle the complexity associated with the large signalling networks and with the tremendous development of systems biology, rapid improvements can be expected.
Acknowledgements
The authors thank members of the BIOS team for their advices and support. D.H. was funded by a fellowship from the INRA (ASC). This work was supported by the INRA AIP AgroBI and by the large scale project REGATE (REgulation of the GonAdoTropE axis).