Abridged English version
1 Introduction
The well logs offer the opportunity to directly access some petrophysical parameters linked to the characteristics of a reservoir. Some preliminary studies have shown that the stochastic component of these measurements is a self-similar fractal signal [21]. It can be modelled as a fractional Brownian motion (fBm) and is governed by a scale law. The information about the power spectrum obtained by Fourier Transform (Fig. 1) is adequate to describe such statistical processes [21], but is not adapted to locate their spatial heterogeneities. To determine the distribution of local regularities of such signals, we use a Continuous Wavelet Transform (CWT) [1,3,6,7,11,16]. This numerical tool is applied to synthetic and actual datasets.

Densité spectrale de quatre diagraphies différentes mesurées dans un même puits. Toutes présentent une décroissance algébrique caractéristique des bruits en 1/f. L'intervalle fréquentiel exploré est contraint par les lois de Nyquist.
Periodogram of four well logs recorded in the same borehole. All show a power-law-type behaviour like the 1/f noise signals.
2 Signal processing
Because well-log data may be described by scale laws [4,9,10,14,15,19,21], their power spectra are approximately inversely proportional to the wave number in log–log plots:
(1) |
The spectral exponent is related to the number of Hurst according to:
(2) |
(3) |
3 Datasets and discussion
3.1 Synthetic dataset
Let us now illustrate, with an fBm process of a synthetic log, how the wavelet-based estimation of the spectral exponent can be used to detect the spatial variations of the signal. The quality of a given analysing method is assessed by the difference between the spectral exponent, more precisely the Hurst number H chosen for the model and the value estimated from the analysis. The synthetic stochastic fluctuations of well data are modelled here by filtering a normally distributed random number sequence by the square root of the power spectrum of a von Karman self-correlation function [15] in the wavenumber domain.
We have tested our algorithm on an academic model with eight layers (Table 1 and Fig. 2a). Its scalogram (Fig. 2b) reflects the energy distribution into the time/frequency space. It also shows local changes of the spectral exponent
Signal synthétique résultant de la concaténation de huit couches de type mBf dont les β varient entre 1 et 3 et les épaisseurs entre 32 et 512 échantillons. La profondeur cumulée est calculée en considérant le pas d'échantillonnage des diagraphies 0,1524 m
Parameters of the synthetic dataset resulting of the juxtaposition of eight mBf with 1<β<3. The cumulative depth is calculated using the sampling step of the well log: 0.1524 m
Couches | C1 | C2 | C3 | C4 | C5 | C6 | C7 | C8 |
N | 512 | 32 | 512 | 32 | 128 | 64 | 256 | 512 |
Profondeur cumulée (m) | 77,87 | 82,75 | 160,78 | 165,66 | 185,17 | 194,92 | 233,93 | 311,96 |
β | 1,0 | 1,4 | 1,6 | 2,0 | 2,3 | 2,6 | 2,9 | 3,0 |
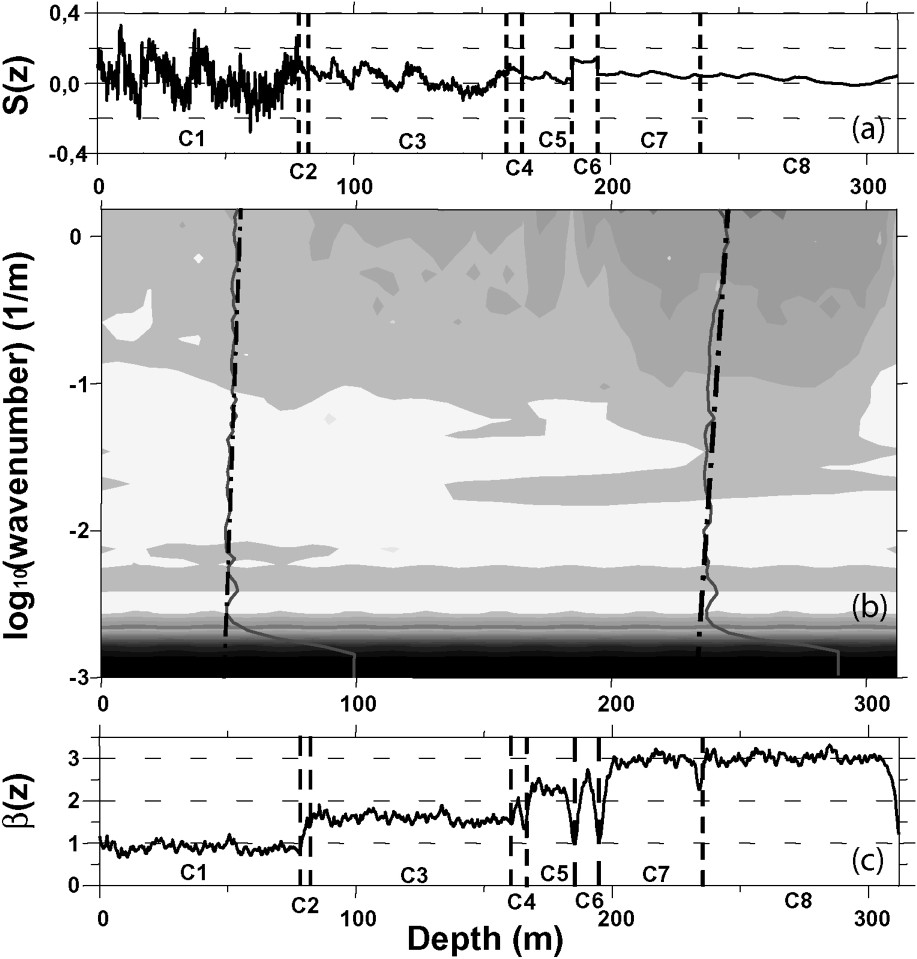
Analyse spectrale d'un signal synthétique de type mBf (a) ; son scalogramme obtenu par transformée continue en ondelettes (b) ; trace des exposants spectraux locaux (c).
CWT spectral analysis of a fBm synthetic signal (a); its scalogram, showing two local cross-sections (b); plot of the local spectral exponent (c).
3.2 Actual datasets
To better assess the potential of the CWT transform, we have extended its application to actual data coming from the MAR203 borehole located in the Rhone valley, near Marcoule (France). This well samples continuously the Cretaceous sedimentary deposits stacked on the western part of the Tethys passive margin. The Urgonian marine deposits are associated with a deformation of the carbonate platform. The Gargasian marl series, well known in the region, is absent below MAR203, where the margin does not sink before Albian time. Then, the platform gets immerse and is stacked with a tick silty layer (called CSM). Because the basin remains shallow, the upper part of the CSM is strongly bioturbated. During the Lower Cenomanian, a regression follows up at the top of the CSM, with an alternation of playa and lacustrine units [5,18].
Using the CWT method, we have determined the spectral exponent of the following well logs: SFLU, NPHI, v-DT and GR of this borehole. The plots of one of them, its stochastic component and its derived

Traitement des diagraphies du puits MAR203 : (a) log sédimentologique résultant de l'observation des carottes (document Andra) ; (b) trace de la diagraphie sonique ; (c) trace de sa composante stochastique ; (d) trace de son exposant spectral ; (e) détermination des propriétés réservoir à partir des quatre diagraphies SFLU, NPHI, DT et GR ; (f) détermination des propriétés réservoir à partir des quatre β(z) correspondants (voir le texte pour une discussion).
Interpretation of the MAR203 well logs: (a) reference log resulting from core observations; (b) plot of the sonic log; (c) plot of the stochastic component of the sonic log; (d) plot of the spectral exponent of the sonic log; (e) reconstitution of the reservoir properties from SFLU, NPHI, DT and GR; (f) reconstitution of the reservoir properties from their respective β(z) (see text for discussion).
1 Introduction
Comme tout signal, une diagraphie est composée d'une partie déterministe (par exemple, une formation sédimentaire est d'autant plus radioactive qu'elle est argileuse...) et d'une partie aléatoire, souvent implicitement assimilée au bruit instrumental.
De nombreuses lois phénoménologiques, telle la loi d'Archie, permettent de déterminer la lithologie traversée par le forage et de caractériser ses propriétés pétrophysiques à partir de la composante déterministe. Quoique peu nombreux, quelques travaux sont consacrés au traitement de la partie stochastique des données de puits [4,7,9,10,14,15,19,21]. Ils montrent l'importance de l'information spécifique portée par cette composante aléatoire, qui ne se réduit pas à un simple bruit blanc. En effet, dans l'espace des fréquences, leur densité spectrale suit une loi de décroissance caractéristique des « bruits en
Dans ce travail, au travers de l'étude d'un cas, nous montrons que nombre de propriétés pétrophysiques mesurées en forage présentent de telles caractéristiques d'autosimilarité, et comment il est possible d'extraire ces nouveaux attributs statistiques, caractéristiques du milieu géologique, à l'aide de la méthode de la transformée continue en ondelettes (TOC) dont nous rappelons qu'elle consiste en un produit de convolution du signal
(1) |
2 Méthodologie
Le périodogramme résultant de la transformée de Fourier (TF), même si sa mise en œuvre n'est pas toujours justifiée [6,17], permet d'explorer facilement la nature d'un bruit analytique. Si, dans une représentation bi-logarithmique, la densité spectrale est constante, le bruit est blanc ; si elle décroît en fonction de la fréquence, alors elle porte une partie de l'information qu'il serait dommage de négliger [1,3]. C'est le cas des diagraphies. Dans la Fig. 1, nous avons représenté les périodogrammes de la vitesse acoustique (vDT), d'une résistivité électrique, (SFLU), d'une radioactivité naturelle (GR) et d'une mesure nucléaire induite : la porosité « neutron » NPHI. Ces quatre signaux mesurés dans le même forage MAR203, mais choisis parce que mesurant des propriétés pétrophysiques très différentes, présentent tous le même type de comportement fréquentiel, caractéristique des bruits dits en
Le mouvement brownien fractionnaire (mBf), parce qu'il est gaussien, est le processus le plus couramment mis en œuvre pour modéliser de tels bruits. La TOC d'un mBf permet donc d'illustrer les potentialités de cette méthode appliquée aux bruits en
(2) |
L'analyse temps/fréquence – dans le cas des mesures en forage, on lira « profondeur » en lieu et place de « temps » – permise par la TOC est particulièrement bien adaptée à l'étude de tels processus. En effet, d'après [6], la stationnarité des accroissements du mBf, jointe au fait qu'une ondelette
(3) |
(4) |
Dans l'algorithme spécifique mis en œuvre pour le calcul de la TOC, nous avons adopté l'approche proposée par [15]. Fort du théorème de Parceval, elle consiste en un produit des TF du signal et de l'ondelette dans l'espace des fréquences, moyennant certaines adaptations, telles que
Parce que le mBf possède la propriété d'avoir des accroissements stationnaires, les processus qu'il modélise présentent des effets de corrélation à long terme. Autrement dit, leurs fluctuations sont d'autant plus régulières que H est proche de 1 et d'autant plus irrégulières que H est proche de 0. Ce comportement se traduit par l'existence d'une dimension fractale, dont on montre qu'elle vaut
(5) |
(6) |
(7) |
3 Applications
3.1 Modélisation de la composante stochastique d'une diagraphie
Afin de vérifier le pouvoir de résolution de la méthode mise en œuvre, nous l'appliquons à un signal synthétique présentant, par construction même, les propriétés d'autosimilarité requises. Le signal que nous avons généré (Fig. 2a) est construit en concaténant huit régimes mBf différents, dont les paramètres sont rassemblés dans le Tableau 1. Pour calculer chacune des parties, nous générons une séquence de
Le scalogramme (Fig. 2b) représente la distribution d'énergie dans le plan profondeur/nombre d'onde. Le signal dérivé obtenu par le calcul des exposants spectraux locaux en chaque point du signal est représenté dans la Fig. 2c. Deux exemples de spectres locaux sont superposés au scalogramme. L'écart de leurs traces à la verticalité permet d'illustrer le calcul des exposants spectraux locaux.
La comparaison du signal dérivé et du signal originel montre que les couches C1 et C3 sont identifiées sans aucune ambiguïté, à cause d'un échantillonnage suffisant. La couche C2 est noyée dans la transition entre C1 et C3, en raison de son faible nombre d'échantillons et d'un contraste insuffisant entre son exposant spectral et celui des couches adjacentes. La couche C4, quoique aussi mince que C2, est repérée par un pic identifiable, du fait d'un contraste suffisant de son
3.2 Application à un cas réel
Afin d'illustrer cette approche de traitement numérique des diagraphies, nous l'avons appliquée aux mesures enregistrées dans le forage MAR203 implanté dans le Gard rhodanien par l'Andra, lors de ses études préliminaires à la réalisation d'un laboratoire souterrain destiné à l'étude de faisabilité du stockage à long terme des déchets nucléaires en formation géologique profonde. Outre les diagraphies enregistrées, ce forage a fait l'objet d'un carottage en continu, ce qui a permis une description précise de la lithologie (Fig. 3a). Il a été arrêté à la cote −892 m dans la formation sommitale de la plate-forme carbonatée urgonienne. Les unités crétacées postérieures sont caractérisées par la présence d'une épaisse couche de silt, laminée à la base, bioturbée au sommet, la couche silteuse de Marcoule (CSM). Elle repose sur la dalle récifale urgonienne par un niveau de marnes calcaires, surmonté par un banc de grès glauconieux à gravier. Le cycle Vraconnien–Cénomanien, au cours duquel elle s'est déposée, se termine par l'alternance de faciès de plage et de faciès lagunaires [5].
Les densités spectrales des quatre diagraphies traitées sont représentées dans la Fig. 1. Elles satisfont toutes les quatre les critères de décroissance caractéristiques de signaux autosimilaires. Elles peuvent donc faire l'objet d'un traitement en ondelettes pour l'extraction des exposants spectraux des lois d'échelle sous-jacentes. À titre d'illustration, la trace de la diagraphie vDT, de sa composante stochastique et de son exposant spectral sont représentés dans les Fig. 3b–d. Si l'épaisse couche silteuse présente des propriétés pétrophysiques homogènes entre les cotes −482 et −774 m [18], tout juste marquées par un gradient vertical résultant de la compaction de la série, il n'en est pas de même pour les exposants spectraux, qui montrent des variations significatives, révélant la structure sous-jacente des milieux géologiques.
Afin de tirer parti de ces informations, nous avons déterminé la structure interne de la séquence crétacée, en traitant séparément, d'une part, les diagraphies elles-mêmes et, d'autre part, leurs signaux dérivés par TOC. Dans l'un et l'autre cas, nous avons mis en œuvre la même méthode de partitionnement des données que celle développée dans des travaux antérieurs [2,8] sur cette même série de données. Les résultats des deux traitements sont représentés dans les Fig. 3e et f. La transformation des données par le calcul des exposants spectraux apporte un gain substantiel d'information pour la partie sommitale gréseuse de la formation silico-clastique, faite d'une alternance de grès propres et de grès argileux, interlités avec des bancs minces de lignites ou de calcaires lacustres. Ces sous-unités sont bien différenciées, alors qu'elles ne le sont pas par le traitement direct des diagraphies. La structure de la CSM est également mieux résolue par le traitement des exposants spectraux. Sa partie supérieure, comprise entre les cotes −367 et −484 m, est une alternance de bancs décimétriques de grès glauconieux assez grossiers bioturbés et de marnes silteuses ou sableuses glauconieuses, plus ou moins bioturbées. Cette succession de bancs minces est mal rendue par le traitement direct des diagraphies, alors qu'elle l'est par le traitement des exposants spectraux. Entre les cotes −484 et −781 m, la CSM est également mieux résolue par le traitement des exposants spectraux que par celui des diagraphies. Ils permettent de mettre en évidence l'homogénéité de la couche silteuse jusqu'à la cote −700 m, puis le passage à l'unité basale, plus marneuse et surtout plus laminée. Dans l'un et l'autre cas, la semelle calcaire est bien identifiée et localisée en profondeur. La méthode des exposants spectraux semble être moins performante pour résoudre la partie basale de l'unité silteuse. Le découpage obtenu par le traitement direct des diagraphies semble être mieux en adéquation avec le log sédimentologique résultant de la description des carottes. Dans ce cas, la méthode des exposants spectraux surligne les discontinuités sédimentaires plutôt que les variations lithologiques.
4 Conclusion
Les composantes stochastiques des diagraphies sont des « bruits en
Remerciements
Nous tenons à remercier l'Andra pour avoir mis à notre disposition les données du forage MAR203, ainsi queles experts auxquels a été confié le manuscrit pour leurs remarques utiles à l'amélioration de sa structuration.