1 Introduction
Atmospheric aerosols, small particles suspended in the air, interact with solar and thermal radiation. By scattering sunlight and reflecting a fraction of it back to space, aerosols first cool the atmosphere–surface system. By absorbing sunlight in the atmosphere, they further cool the surface but warm the atmosphere. As a result, they change the temperature and humidity profiles and the conditions for cloud development. They also impact the cloud properties by acting as cloud condensation nuclei and ice nuclei. They modify the cloud droplet concentration and tend to decrease the droplet size, which prevents or delays development of precipitation and they modify the cloud albedo (see, for example, (Hansen et al., 1997; Ramanathan et al., 2001)).
For estimating the aerosol radiative forcing on the climate, there is a need for discriminating between man-made aerosols and those resulting from natural processes. This can be achieved by determining their physical properties since the aerosol size distribution includes several modes that are associated to different processes. The fine (or accumulation) mode, around roughly 0.1–0.2 μm, is formed from condensation or chemical conversion of gases to the liquid phase. So, smoke and urban/industrial pollution resulting from human activity are mainly in fine mode particles and can present a large absorption. Natural aerosol particles, wind driven sea spray, wind blown dust and soil, fly ash and biogenic particles, are mainly larger than 1.0 μm; they are within the coarse mode and are also characterized by low absorption in the visible part of the solar spectrum.
A review of the multiple approaches used, or to be used, for aerosol remote sensing from space has been done 10 years ago (King et al., 1999). Hereinafter, we focus on the present satellites that are expected to advance our understanding of the aerosol effect on the hydrological cycle and climate (Kaufman et al., 2002). We show that several relevant aerosol properties for climate studies can be derived by measuring the spectral, angular and/or polarization1 radiances scattered through the aerosol layers to the top of the atmosphere. Improvements by introducing active measurements with lidars are also described later.
2 The large-scale picture of aerosol distribution
The parameters that are primarily relevant and accessible from remote sensing are: (i) the aerosol optical depth (AOD), that is a measure of the integrated aerosol load through the atmosphere valuable for evaluating aerosol amount and its variability; and (ii) the Angström Exponent (AE) related to the AOD spectral dependence, that gives an indication of the column integrated aerosol size distribution.
The aerosol remote sensing from space has started in the 1980s, but the sensors were not specifically designed for observing aerosols, so the number of retrieved parameters was very limited and retrievals restricted over the oceans. These pioneer studies used observations provided by geostationary satellites, such as GOES or METEOSAT, or by polar orbiting platforms, such as the NOAA/AVHRR series (see, for example, (Fraser et al., 1984; Husar et al., 1997)). Since the radiance was available in a single wavelength and at a single angle of observation, an assumption on the aerosol model was required and only the aerosol content was inverted. More recent analysis has used the second channel of the AVHRR instrument (not used in the earlier studies because of contamination by water vapor absorption), then information on the aerosol type was derived using the spectral information (Mishchenko et al., 2003; Nakajima et al., 2001; Sekiguchi et al., 2003). Over land, the surface contribution to the satellite radiance is much larger than over the oceans and difficult to correct for, so the aerosol detection is more challenging. In the UV, most of the Earth's surfaces are dark enough and the spectral ratio of radiances in two channels of the TOMS series instruments was found to be sensitive to the presence of elevated smoke or dust layers above scattering atmosphere (Herman et al., 1997a), but the analysis in terms of aerosol content requires an assumption on the height of the aerosol layer (Torres et al., 2002). Although providing limited information, TOMS and AVHRR are very attractive sensors since they have been operating for almost 30 years.
Geostationary satellites, METEOSAT and GOES, have one major advantage compared to the polar orbiters, that is their ability to monitor aerosol transport over a given area with high temporal sampling. The visible channel of the METEOSAT series has been extensively used over the oceans to follow dust events originating from the Saharan and Sahel dry areas (Dulac et al., 1992; Jankowiak and Tanré, 1992; Moulin et al., 1997). The first generation of METEOSAT satellites, with only one wide spectral band in the visible band (0.45 to 1.0 μm), was unable to distinguish between aerosol types, like dust or biomass burning aerosols, but this limitation does not apply to the METEOSAT Second Generation (MSG) that carries three channels in the solar spectral range, from 0.6 to 1.6 μm (Thieuleux et al., 2005). For instance, AOD and AE operationally derived from MSG data are presently available at the ICARE data center (http://www.icare.univ-lille1.fr/msg/browse/). Over land, the thermal infrared channel of METEOSAT can be used to locate the main source areas of dust in northern Africa, through their impact on the apparent temperature at midday during dust storms (Legrand and N’doumé, 2001). Nevertheless, quantitative estimates are difficult to achieve since several other atmospheric parameters are involved. It is expected that the recent generation of geostationary satellites will permit an excellent monitoring of the aerosol sources and transport over the African continent.
3 Remote sensing of aerosols by dedicated satellite instruments
For estimating the aerosol radiative forcing in climate models (see, for example, (Menon et al., 2002)) or for evaluating transport models (see, for example, (Chin et al., 2002)), more quantitative information on aerosol type and concentration is required at a large scale. The A-Train satellite formation, which consists presently of five satellites flying in constellation, was specifically designed to measure aerosol, clouds and precipitation (http://aqua.nasa.gov/doc/pubs/A-Train_Fact_sheet.pdf). The combination of the different sets of observations is expected to better retrieve the aerosol parameters and to better understand the processes related to climate change.
Instruments dedicated to aerosol monitoring and using the solar radiances reflected by the Earth, are PARASOL/POLDER and AQUA/MODIS. By measuring the wavelength, angular and/or polarization properties of the radiance at the top of the atmosphere, one can better quantify AODs, improve the derivation of fine and coarse aerosols, and estimate their refractive index. The current algorithms are based on a comparison between measurements and look-up tables (LUTs) built for a set of aerosol models (size distribution, refractive index, shape), content (optical thickness) and other atmospheric conditions, as close as possible to the actual situation. At this stage, ground-based measurements bring key information concerning the aerosol models that have to be used to build these LUTs (Dubovik et al., 2002a; Dubovik et al., 2002b; Holben et al., 2001). Although the TERRA/MISR instrument is not part of the train, it is also described because of its specific angular capabilities. The approach used by ATSR-2 and AATSR (Advanced Along-Track Scanning Radiometer) on board the European Space Agency (ESA) satellite ENVISAT combines the capabilities of the above sensors by using two view directions and a wide spectral range (0.55–1.65 μm). It is not described, but details about the algorithm can be found in (Veefkind et al., 1998) or (North et al., 1999). With active (lidar and radar) remote sensing technics, global measurements of the aerosol and cloud profiles can be collected, profiles that are needed to accurately assess the aerosol radiative forcing. The CALIOP space borne lidar launched in 2006, is thus described later.
3.1 PARASOL/POLDER
The PARASOL (Polarization and Anisotropy of Reflectances for Atmospheric Science coupled with Observations from a Lidar) payload is largely based on the POLDER instrument (Deschamps et al., 1994) and is the second in CNES (Centre national d’études spatiales) Myriade line of microsatellites. PARASOL was launched on December 18, 2004 and is routinely acquiring data since March 2005. Its payload consists of a digital camera with a 274 × 242-pixel CCD detector array, wide-field telecentric optics and a rotating filter wheel enabling measurements in 9 spectral channels from blue (0.443 μm) through to near-infrared (1.020 μm) and in three polarization directions. Polarization measurements are performed at 0.490 μm, 0.670 μm and 0.865 μm. Because it acquires a sequence of images every 20 sec, the instrument can view ground targets from different angles, ±51° along track and ±43° across track. A limitation of POLDER is the rather coarse spatial resolution of about 6 km, which affects the ability to account for scene heterogeneity. The POLDER instrument flew also onboard the ADEOS 1 and 2 platforms in 1996–1997 and 2003, respectively. Unfortunately, due to the failure of the satellite solar panels, the measurement time series are limited to respectively 8 and 7 months.
Algorithms have been developed to process the measurements in terms of aerosols provided at 18.5 × 18.5 km2 resolution (Deuzé et al., 2001; Herman et al., 1997b). Over the oceans, the satellite algorithm (Herman et al., 2005) assumes spherical or non-spherical particles, non-absorbing particles and that the size distribution follows a combination of two lognormal aerosol size distributions, one in the fine or accumulation mode (sub-micron size, effective radius reff typically smaller than 0.5 μm) and one in the coarse mode (reff typically larger than 1.0 μm). When the geometrical conditions are optimal, the shape (spherical or not) of the particles is derived. The refractive index retrieval is next attempted from the polarization measurements. If the real part of the refractive index of the coarse mode is retrieved when spherical particles are present (generally close to 1.35, indicating hydrated particles), the derivation of the refractive index of the accumulation mode is still tentative. The optical depths of the different aerosol modes derived from PARASOL over the ocean are reported in Fig. 1 for September 2005. Comparisons of AODs derived from POLDER with ground based AERONET measurements (Holben et al., 1998) show good agreement, with typical RMS errors on the order of 0.05 with no significant bias (Goloub et al., 1999). The fine-mode optical depth can also be compared to AERONET measurements, albeit with some uncertainty in the aerosol radius cutoff. Statistical results indicate a bias of 0.02 with a standard deviation of 0.02. Let us add that PARASOL has at least one viewing direction out of the glint, making aerosol retrievals possible everywhere over water. Over land surfaces the retrieval of aerosol properties is based on polarized reflectance measurements. Polarized reflectance of land surfaces is small and fairly constant, although it does have a very strong directional signature (Nadal and Bréon, 1999). Scattering by submicron (accumulation mode) aerosol particles generates highly polarized light (Deuzé et al., 1999), which makes the polarized satellite radiances more sensitive to the presence of aerosols than the total radiances. On the other hand, larger aerosol particles, such as desert dust, almost do not polarize sunlight and are therefore hardly detected from polarization measurements. In regions where dust-loaded atmospheres are excluded, i.e. in regions affected by biomass burning or pollution aerosols, comparison with AERONET measurements shows no significant bias and an RMS error on the order of 0.04. A specific study (Fan et al., 2008) comparing AERONET data over Beijing and Xianghe in China demonstrated the capability of PARASOL for determining the anthropogenic contribution (particle radii less than or equal to 0.3 μm) of regional aerosols. Correlation between the two data sets gives a slope close to one and a 0.03 RMS on AOT when the contribution of the accumulation mode to the AOT at 865 nm is larger than 30%. The retrieval could be improved over a given area when the surface reflectance model is constrained and validated by use of independent data sets of coincident sunphotometer and POLDER measurements (Léon et al., 1999).
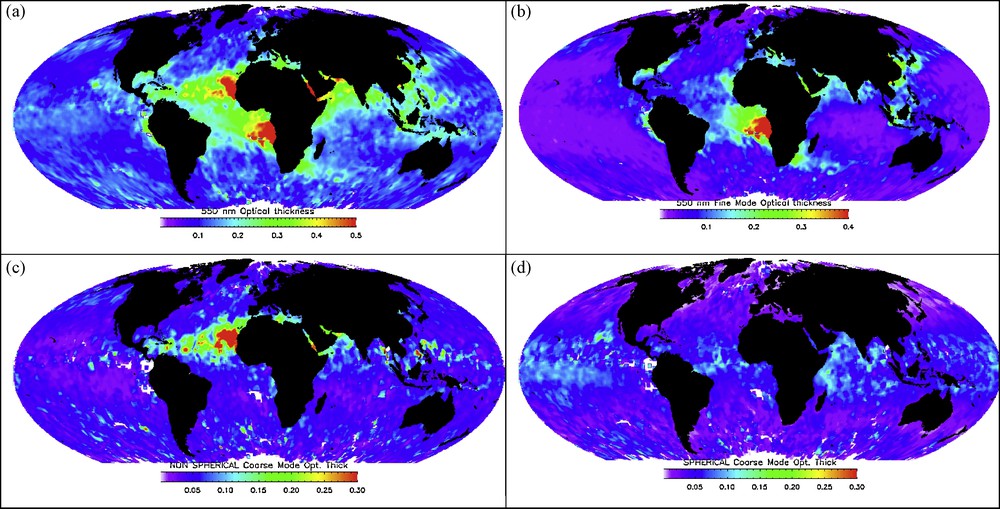
POLDER aerosol monthly optical depths at 550 nm for September 2005. (a): total AOD, (b): AOD coming from aerosols within the accumulation mode, (c): AOD resulting from non-spherical particles within the coarse mode, (d): AOD resulting from spherical particles within the coarse mode. Note the long-range transport of dust over the Atlantic ocean (c).
Épaisseurs optiques à 550 nm des aérosols, moyennées sur le mois de septembre 2005. (a) : épaisseur optique totale, (b) : épaisseur optique correspondant au mode d’accumulation, (c) : épaisseur optique correspondant aux particules non sphériques du mode grossier, (d) : épaisseur optique correspondant aux particules sphériques du mode grossier. On notera sur l’image (c) le transport à grande distance des poussières désertiques sur l’océan Atlantique.
3.2 MODIS/Aqua and Terra
MODIS (MODerate resolution Imaging Spectroradiometer) sensor is on both the Terra and Aqua satellites. It started collecting data in February 2000 from the Terra spacecraft (10:30 am equatorial crossing time) and in June 2002 from the Aqua spacecraft (1:30 pm equatorial crossing time). MODIS provides radiance measurements from 0.41 to 14 μm in 36 spectral bands very suitable for aerosol and cloud monitoring (King et al., 1992). The aerosol characteristics are derived at the 10 × 10 km2 resolution over the oceans and land using independent algorithms (Remer et al., 2005).
Over oceans, the MODIS aerosol algorithm uses the radiances from six MODIS bands (0.55–2.1 μm) to distinguish particles within the fine mode from particles within the coarse mode. Specifically, in cloud-free and glint-free ocean scenes (Martins et al., 2002), MODIS retrieves the spectral aerosol optical depth, the fraction f550 of τ at 0.550 μm that is contributed by the fine mode aerosol and the effective radius of the aerosol size distribution (Tanré et al., 1997). Over land, MODIS uses the 2.1 μm channel to estimate surface reflectance and to derive the aerosol optical depth in the 0.47 and 0.66 μm channels (Kaufman et al., 1997; Levy et al., 2007); the spectral dependence between the 0.47 and 0.66 μm channels is used to derive f550. The MODIS-derived aerosol properties have been validated using AERONET measurements (Remer et al., 2005). In agreement with theoretical error analysis (Kaufman et al., 1997; Tanré et al., 1997), the inversion scheme can retrieve the aerosol optical depth with an error of Δτ550 = ± 0.03 ± 0.05 τ over the oceans and Δτ550 = ± 0.05 ± 0.15 τ over land. Since the inversion scheme is only a part of the algorithm, which includes, for example, calibration, cloud-screening, or spatial variability of the aerosol field, the accuracy of the operational products can be deteriorated over specific regions and/or in some aerosol conditions.
From MODIS, it has been shown that the fraction of aerosol optical depth due to anthropogenic sources over the oceans is around 21 ± 7% (Kaufman et al., 2005). The global distribution of aerosol optical depth (AOD) has been also derived from MODIS/AQUA that illustrates the large seasonal variability of the source regions (Remer et al., 2008) and their yearly evolution. An aerosol optical thickness anomaly for 2008 as compared with the 2000–2008 long-term mean calculated from MODIS-Terra annual mean values is reported in Fig. 2. It shows the importance of the regional aspect of the aerosol component. The figure shows also the difficulty to retrieve aerosol over deserts where alternative methods have been developed (Hsu et al., 2006).

Aerosol optical thickness anomaly for 2008 as compared with the 2000–2008 long-term mean calculated from MODIS-Terra annual mean values. Blues indicate that 2008 was lower than the long-term mean and reds indicate higher values (credit: L.A. Remer from GSFC/NASA).
Anomalie de l’épaisseur optique pour l’année 2008, comparée à la moyenne des observations de MODIS/TERRA pour la période 2000–2008. Les zones bleues correspondent à des valeurs 2008 inférieures à la moyenne, les zones rouges à des valeurs supérieures (avec l’aimable autorisation de L. Remer du GSFC/NASA).
3.3 MISR/TERRA
The MISR (Multi-angle Imaging Spectro Radiometer) instrument (Diner et al., 1998) is on-board the TERRA platform. Its main advantage compared to other instruments is its capability to measure the radiance at nine viewing angles from nadir up to 70° forward and backward, along the satellite's track and in four spectral bands within the 0.44–0.87 μm range. The 70° forward and backward viewing directions minimize the surface contribution and carry more information on the aerosols than the nadir observation. The AOD and aerosol type are derived over 17.6 km retrieval regions.
Over water, by using the MISR's scattering angle coverage (∼60°–160° in mid-latitudes), particle size and shape can be derived (Martonchik et al., 1998; Kahn et al., 2001). Recent work indicates that MISR is also able to separate different mineral dust shape classes (Kalashnikova et al., 2005; Kalashnikova and Kahn, 2008). Over land, the algorithm makes use of the systematically changing ratio of surface to atmospheric radiance with view angle to separate the surface from atmospheric signals (Martonchik et al., 1998). As a result, MISR can detect and quantify aerosols also over bright surfaces like desert regions. The resulting optical depths show good agreement with values measured by AERONET (Abdou et al., 2005; Diner et al., 2001; Kahn et al., 2005; Martonchik et al., 2004).
One more characteristic of MISR is its capability to obtain the heights of cloud and aerosol plume tops stereoscopically as long as there is some spatial contrast. The method can be applied to aerosols near volcanic, forest fire, and dust source regions, where features are present. An example for a fire that occurred in 2002 near Denver, Colorado is shown in Fig. 3 extracted from (Kahn et al., 2007a).
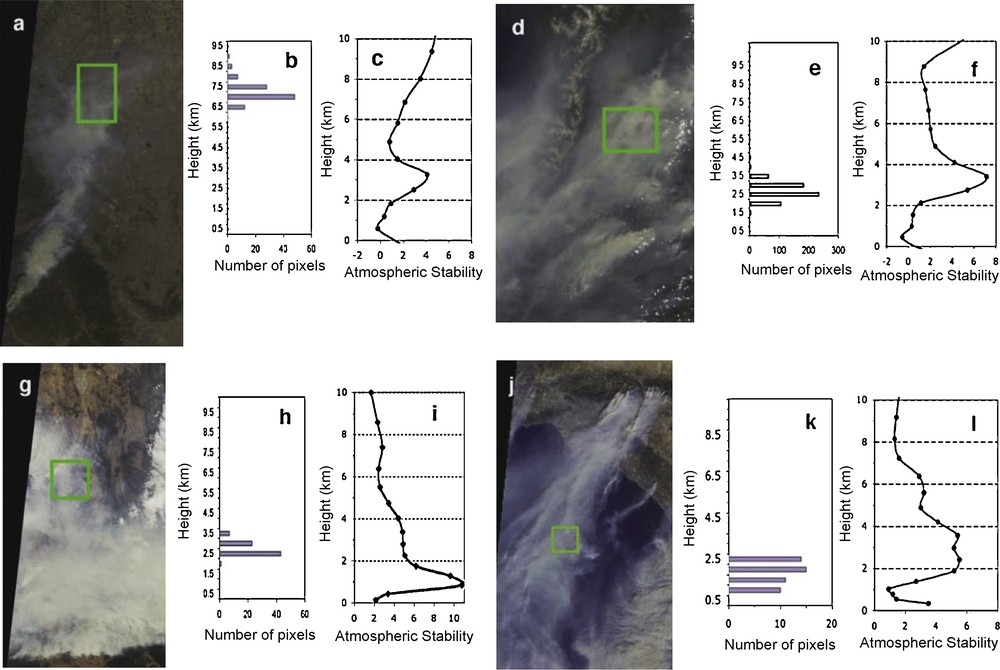
Fire plume height and atmospheric stability. (a–c) 9 June 2002 fire originating 65 km SW of Denver, CO (about 37–39°N lat, 105°W lon), orbit 13170, path 032, blocks 59–61. (a) MISR true-color nadir image with study patch marked in green. (b) Plume height histogram for the study patch, from MISR Standard Stereo Height product. (c) Atmospheric stability profile, calculated from the NCEP GDAS (Saha et al., 2006; Kahn et al., 2007a). (d–f) Same as Figure 3a–3c, but for the 11 June 2003 fire in the Siberian Taiga, south of Lake Baikal (about 51–54°N lat, 110–112°E lon), orbit 18506, path 130, blocks 47–49. (g–i) Same as Figure 3a–3c, but for the 19 January 2003 brush fire near Canberra, Australia (about 35–37°S lat, 148–151°E lon), orbit 16421, path 089, blocks 118–120. (j–l) Same as Figure 3a–3c, but for the 26 October 2003 wildfires near Los Angeles (about 32–35°N lat, 117–120°W lon), orbit 20510, path 040, blocks 63–64. From Kahn et al., 2007a, with the permission of the AGU
Hauteur d’un panache de fumée et stabilité verticale de l’atmosphère. (a–c) Feu observé le 9 juin 2002 et situé à 65 km au sud-ouest de Denver, Colorado (vers 37–39°N lat, 105°W lon), orbit 13170, path 032, blocks 59–61. (a) image en vraie couleur obtenue à partir de la direction “nadir” de MISR, le rectangle vert correspondant à la zone d’études. (b) Histogramme de l’altitude du panache dans la zone considérée. (c) stabilité verticale de l’atmosphère obtenue à partir des analyses du NCEP/GDAS (Saha et al., 2006; Kahn et al., 2007a). (d–f) Figures identiques à 3a–3b, mais pour un feu observé le 11 juin 2003 dans la Taiga sibérienne (vers 51–54°N lat, 110–112°E lon), orbit 18506, path 130, blocks 47–49. (g–i) Figures identiques à 3a-3b, mais pour un feu observé le 19 janvier 2003 proche de Canberra, Australie (vers 35–37°S lat, 148–151°E lon), orbit 16421, path 089, blocks 118–120. (j–l)) Figures identiques à 3a-3b, mais pour un feu observé le 26 octobre 2003 proche de Los Angeles, USA (vers 32–35°N lat, 117–120°W lon), orbit 20510, path 040, blocks 63–64. Figure provenant de (Kahn et al., 2007a). Avec la permission de l’AGU.
3.4 CALIOP/CALIPSO
As already pointed out, absorbing aerosols warm the atmosphere and cool the surface. So they reduce the atmosphere vertical temperature gradient, which is expected to slow the hydrologic cycle, reduce evaporation from the surface and reduce cloud formation. In cloudy conditions, the aerosol radiative effect depends on the fraction of absorbing aerosol located above clouds where they may even have a positive (warming) rather than negative (cooling) radiative impact. Regarding the first indirect impact, the effect of aerosols on cloud droplet size is also depending on the respective locations of the CCN and cloud droplets. Knowledge of the vertical distribution of aerosols and clouds is therefore needed to understand and model the processes.
Lidar capabilities from satellites were first demonstrated onboard the Shuttle and MIR platforms respectively in 1994 and 1996 (Chanin et al., 1999; Karyampudi et al., 1999). Then the Ice, Cloud, and land Elevation Satellite (ICESat), launched in 2003, was the first long-duration platform with a lidar (GLAS, Geoscience Laser Altimeter System) on board. The Cloud-Aerosol Lidar and Infrared Pathfinder Satellite Observation (CALIPSO) satellite launched in April 2006 is the first mission including an active sensor specifically devoted to cloud and aerosol monitoring. The CALIPSO payload includes the CALIOP lidar (Cloud-Aerosol Lidar with Orthogonal Polarization), a passive Infrared Imaging Radiometer (IIR), and visible Wide Field Camera (Winker et al., 2003; Winker et al., 2009). Mie scattering lidars, which are the simplest lidar technique, have a limitation in quantitative measurement, since the lidar equation can be solved only with an assumption on the backscatter-to-extinction ratio (BER) or lidar ratio. Additional constraints such as independent optical depth measurement, for example with a sunphotometer, can improve the inversion scheme. If the aerosol type has to be assumed constant over the whole column when using a single wavelength, this shortcoming can be overcame by using multiple wavelengths since they provide useful information for characterizing the aerosol type along the vertical. An additional feature of Mie scattering lidar is the depolarization ratio measurement, which is a good index of non-sphericity of the scatterers. It is used for detecting mineral dust particles. The lidar ratio's that are considered in the present CALIOP algorithm, have been derived for different aerosol types from a comprehensive cluster analysis applied to the AERONET data gathered from numerous sites around the globe (Omar et al., 2005; Winker et al., 2004). Combing lidar and spectral measurements like MODIS is also promising for deriving information on the size distribution along the vertical (Kaufman et al., 2003; Léon et al., 2003), but the approach has not yet been applied to actual satellite data. The ability to derive vertical structures from CALIPSO has been demonstrated (see, for example, (Kim et al., 2008; Vaughan et al., 2009; Winker et al., 2007)) and illustrated with a view of the 3D distribution of dust (Liu et al., 2008) that shows the major dust sources, the associated long-range transport and confirms the large horizontal and vertical coverage of dust aerosols. A new approach to retrieve the AOD over the ocean combining CALIOP and the radar onboard the CloudSat platform (Stephens et al., 2002) has been also developed (Josset et al., 2008).
Let us mention that there are techniques where the limitation of the a priori knowledge of the lidar ratio can be overcame using independent measurements of the extinction and backscattering coefficients. Two methods are presently used, the Raman lidars that utilize Raman scattering of atmospheric molecules (Whiteman, 2003) and the HSRLs (High Spectral Resolution lidar) (Liu et al., 1999).
4 Discussion
As recently reported in (Liu and Mishchenko, 2008) and (Li et al., 2009), there are discrepancies between aerosol datasets resulting from different satellite-based observations. While it is not unexpected when comparing past sensors like AVHRR or TOMS, it is more surprising using recent sensors like MODIS, MISR or POLDER. Discrepancies differ depending on the retrieved parameters (total AODs or size distribution from ratios between the fine and the coarse modes or from the Angström Exponent), on the levels of the products (daily or monthly, gridded or not at 1 × 1°) and on the underlying surface, ocean or land. In addition to the assumptions used in the inversion algorithm itself (aerosol models of the LUT's and surface effects), there are external elements that affect the quality of the retrieval: (i) calibrations of the sensors (Lallart et al., 2008); (ii) cloud screening may be more or less stringent; (iii) spatial uniformity of the aerosol field; and (iv) its temporal evolution.
The agreement between sensors is found to be generally fair for the AOD over the ocean. Between MODIS and MISR, although the retrieval algorithms produce AOD biases in some conditions, the agreement is acceptable in many cases as reported in (Liu and Mishchenko, 2008) Liu and Mishchenko (2008) and the results fall within the expected uncertainties (Kahn et al., 2007b; Kahn et al., 2009). In the case of MODIS and POLDER, when spherical particles dominate, results (AOD, Angström exponent and relative concentrations) of both inversions are comparable, especially for aerosols in the fine mode. On the other hand, when POLDER inversion detects the presence of non-spherical particles, the agreement between AODs is not as good (Gérard et al., 2005). Concerning the aerosol size, it is more challenging and the choice and the number of the aerosol models that are used to build the Look-Up-Tables are crucial. For instance, in presence of non-spherical particles, the spectral dependence of the radiance is more similar to that of particles smaller than those really present. In addition, the accuracy of the size retrieval, reff, f550 or AE, depends on the aerosol content; for low optical thickness, there is greater susceptibility to algorithmic uncertainties. A deep analysis of the AE at global scale is still needed to understand the origin of the current discrepancies.
Over land, when the surface is bright, aerosol remote sensing requires more sophisticated approaches than over oceans, since the sensitivity to the presence of aerosol decreases. The previously described methods are based on very different assumptions and the surface contribution is the most important perturbing factor. At a first glance, comparison between MODIS and MISR for the main aerosol parameter, the spectral AODs, is unsatisfactory (Liu and Mishchenko, 2008; Li et al., 2009). There are several identified shortcomings that can partly explain the differences. In the case of MODIS, the geographical distribution (Remer et al., 2005) of the aerosol type is an issue, since different aerosol types may be mixed. A similar problem exists for MISR; even if the model is derived along the algorithm, it is difficult to consider all possible cases in the LUT. The properties of the initial aerosol models are more crucial over reflecting surfaces than over the ocean; for instance the impact of the aerosol single scattering albedo is larger over bright surfaces. The impact of some previously cited external factors is also larger over land. Let us add that because of the errors in the spectral AODs, information on the aerosol type, such as the AE, is limited. For MODIS, AE is simply considered as an algorithm diagnostic (Remer et al., 2005). In the case of PARASOL/POLDER, the AOD is derived for the fine mode only and comparison to other retrievals is even more challenging since information on the aerosol size distribution is therefore needed.
Why do the early validation exercises performed from AERONET for the different instruments not reflect such discrepancies? First of all, the primary objective was to validate the core of the retrieval, which is the inversion scheme. The data set are so limited to the “best observation conditions”: (i) spatial and temporal variations of the aerosol field are usually discarded (Goloub et al., 1999; Ichoku et al., 2003); (ii) consistency of the aerosol type over the time is sometimes required; (iii) because the automatically cloud screening of AERONET is very severe, cloud contamination is not an issue; (iv) cconcerning the calibration of the satellite sensor, it results in a systematic bias and retrieved AOD's can fall within the accuracy for each individual sensor, but out of the bounds when comparing sensors. Let us point out that, earlier in the missions, adjustments to maintain calibration sensors were less severe.
Overall, it is worthwhile to note that the accuracy of the present satellite retrieval over the ocean is acceptable for the AOD, and is sufficient for discriminating aerosols that are in the accumulation or in the coarse modes, which was not possible ten years ago. For total AODs over land, agreement is within the expected errors and the location, the strengths, the seasonal variability of the aerosol sources are now available at a global scale, which, again, was not possible with previous sensors.
Most of the discrepancies occur over areas that are expected to be difficult because of surface or aerosol conditions. The present definitions of the QA (Quality Assurance) parameters do not exactly reflect the limits and the shortcomings of the retrievals. On the one hand, they need to be revised; on the other hand, they are not used, as they should by the users. For level 3 products, consideration should be brought during the averaging and/or sampling of the level 2 data; sampling itself can introduce large differences, even from the same sensor.
5 Conclusion
In the last decade, space borne instruments and the international ground network of radiometers represent a major step in our knowledge of aerosol properties at a global scale. The separation of fine, mostly anthropogenic aerosols, from coarse, mainly natural, aerosols is the first step to describe the aerosol direct forcing at the top and bottom of the atmosphere. Active measurements, lidar for aerosols and radar for clouds, are also very promising for a deeper analysis of the complex aerosol indirect effect.
When single radiance-only measurements provide estimates of column optical thickness and of aerosol size (through the Angström Exponent, fine mode fraction, or effective particle size of aerosols), directional and polarized radiances better constrain the inversion to provide improved estimates of the above parameter and also additional information on aerosol composition (through the refractive index), single scattering albedo and shape. Extension to the short infrared spectral (such as MODIS) of the present directional and polarization capabilities of POLDER will provide a more detailed estimate of aerosol properties. There are several projects for building such an instrument. The more advanced is the multi-spectral photopolarimeter APS (Aerosol Polarimetry Sensor) on board the Glory mission (Mishchenko et al., 2007) that National Aeronautics and Space Administration (NASA) will launch soon. On the one hand, it will combine the benefits of the above individual sensors; on the other hand the measurements will only be available along the spacecraft ground track with a spatial resolution of 6 × 6 km2 and a repeat cycle of 16 days.
It is finally worthwhile to remember that aerosol algorithms avoid clouds and contamination by subpixel clouds. Although there are some attempts for deriving AODs above clouds (Waquet et al., 2009), the current values of AOD's are biased to cloud free conditions.
Acknowledgments
This article is dedicated to the memory of Yoram Kaufman. The author would like to thank J.L. Deuzé and M. Herman from LOA for valuable discussions. Lorraine Remer and Ralph Kahn from GSFC/NASA are acknowledged for providing Figs. 2 and 3. The author is also thankful for the support from CNES and CNRS and to the reviewers for their helpful comments.
1 Polarization is the degree of organization of the electric field of the scattered solar radiation into a given direction.