1 Introduction
Suitability of stratospheric predictors in generating extended range forecast of tropospheric variables is well documented in the literature (Baldwin et al., 2003; Jung and Barkmeijer, 2006). Most of the energy that drives the climate system comes from the sun and variations in solar radiation on several timescales are linked with substantial variations of Earth's climate. Because of the absorption of ultraviolet (UV) radiation by ozone in the stratosphere, its concentration varies with the intensity of UV radiation (Alexandris et al., 1999, Katsambas et al., 1997). Ozone variations have a direct radiative impact on the stratosphere and troposphere, and it has been observed that the atmospheric temperatures are broadly consistent with the radiative processes (Baldwin and Dunkerton, 2005). Based on the concept of general circulation model (GCM), it is shown that the changes in tropospheric circulation and temperature are produced in response to stratospheric thermal perturbations (Simpson et al., 2009). Several authors investigated the variability of stratospheric ozone and temperature on monthly to inter-annual timescales (Fusco and Salby, 1999; Kiladis et al., 2006; Randel and Cobb, 1994).
Ozone plays a fundamental role in controlling the chemical composition and climate of the troposphere (Cracknell and Varotsos, 1994; Kondratyev and Varotsos, 1996; Varotsos, 1987, 2002). Tropospheric photochemistry is initiated by photolysis of ozone, which leads to the formation of hydroxyl radical. Absorption of thermal radiation by ozone influences the energy budget of the troposphere (Kondratyev and Varotsos, 1995; Logan and Kirchhoff, 1986). In an analysis of mean-monthly temperature and total ozone data, Angell and Korshover (1964) suggested that quasi-biennial oscillations extend to the polar latitudes of both hemispheres. Much of the observed variability of stratospheric ozone and temperature on monthly to inter-annual timescales is attributable to trend like changes or to forced variations with specified timescales (Cracknell and Varotsos, 1994; Randel and Cobb, 1994; Varotsos, 2004). Correlations between ozone and temperature are commonly used to investigate the photochemical and dynamical aspects of satellite-derived ozone data. The dynamical contributions to the ozone temperature correlations are examined by Rood and Douglass (1985). Sensitivity of ozone to temperature in the upper stratosphere and lower mesosphere is investigated by Froidevaux et al. (1989). Shibata and Deushi (2005) investigated the radiative effect of ozone on the quasi-biennial oscillation with a chemistry-climate model. The changes in temperature have an impact on ozone chemistry in the stratosphere, which will give feedback on temperature, since ozone itself is an absorbing gas. Changes in stratospheric ozone will also alter the radiation field at the lower height levels, thus affecting the dynamics and chemistry down into the troposphere (Shindell et al., 1998). From the results of a global climate model including an interactive parameterization of stratospheric chemistry, Shindell et al. (1998) showed how upper stratospheric ozone changes may amplify the 11-year solar cycle irradiance changes to affect the climate.
It is known that the effect of total ozone (TO), which is comprised of the tropospheric and stratospheric ozone contents (Cracknell and Varotsos, 1995; Varotsos et al., 1995), has significant effects on the climatic condition over the Indian subcontinent. The TO variability is mainly dominated by annual cycles, quasi-biennial oscillations (QBO), El Niño Southern Oscillation (ENSO) and solar cycle (Cracknell and Varotsos, 1995; Varotsos et al., 1994). A significant negative correlation between TO and QBO has been observed for three Indian stations: New Delhi, Varanasi, and Pune, and positive correlation for Kodaikanal station (Londhe et al., 2003). All the stations showed significant positive correlations between TO and solar flux. It was observed by Hingane (1990) that the appearance of minima in total ozone over the subtropical belt of the Indian subcontinent may be the result of some unique signatures of thermal and dynamic processes appearing in that region. The association between the monsoon circulation and TO over India was studied by Londhe et al. (2005). Due to the non-linearity of ozone concentrations and the complex interactions between meteorological variables and ozone, the development of non-linear models like Artificial Neural Network (ANN) (Gardner and Dorling, 1998, 2000), a potent mathematical tool for modelling non-linear geophysical processes, has gained the attention of several researchers working in the area of forecasting tropospheric ozone (e.g. Abdul-Wahab and Al-Alawi, 2002; Chaloulakou et al., 2003; Clark and Karl, 1982; Corani, 2005; Gardner and Dorling, 1998, 2000; Gómez-Sanchis et al., 2006; Hsieh and Tang, 1998; Salazar-Ruiz et al., 2008; Wang et al., 2003). Comrie (1997) compared the performance of ANN with conventional regression method in forecasting the surface ozone concentration and established the supremacy of the ANN over conventional regression. In a comparative study based on a wide set of forecast quality measures, Chaloulakou et al. (2003) indicated that the ANNs provide better estimates of ozone concentrations, whilst the more often used linear models are less efficient at accurately forecasting high ozone concentrations. Sousa et al. (2007) studied a comparison between ANN and multiple linear regression by removing the effect of multicollinearity by means of principal components and found that ANN equipped with principal component analysis performed significantly better than the multiple linear regression model. Salazar-Ruiz et al. (2008) compared the performance of different types of ANN with Ridge regression and obtained results with similar margins of errors. Although the application of ANN in forecasting tropospheric ozone is available in handful, the application of ANN is not so frequent in modelling and forecasting the TO, which is characterized by immense non-linearity and complexity (Chattopadhyay and Chattopadhyay, 2008a, 2010a; Koçak et al., 2000). Chattopadhyay and Chattopadhyay (2009a, 2009b, 2010b) implemented ANN in modelling TO. Although the study of Chattopadhyay and Chattopadhyay (2009a) was made in a multivariate environment, the studies of Chattopadhyay and Chattopadhyay (2009b, 2010b) were based on univariate approaches where autoregressive ANN models were generated and compared with autoregressive moving average and autoregressive integrated moving average models.
The ANN applications discussed in the last paragraph were involved with prediction of ozone or TO based on other meteorological parameters or the past values of TO time series. However, the present study deviates from the aforesaid studies in the sense that instead of predicting TO using meteorological parameters, it has adopted TO as the predictor to generate a predictive model for surface temperature, which is a vital parameter for various meteorological processes. The average air temperature at the surface of the Earth is a frequently used parameter for sensing the state of a climatic system (Ceschia et al., 1994; Chattopadhyay et al., 2010a, 2010b). Necessity for predicting the surface temperature has been emphasized in various papers (e.g. Hussain, 1984; Rehman et al., 1990; Said, 1992; Tasadduq et al., 2002). The study area, Kolkata, of the present paper belongs to Gangetic West Bengal, which is characterized by severe pre-monsoon (March–May) thunderstorms and heavy summer-monsoon (June–September) rainfall. Role of temperature in the genesis of thunderstorm is studied in Haklander and Delden (2003), Huntrieser et al. (1997), Schmeits et al. (2005) and the role of temperature in rainfall is discussed in Elliott and Angell (1987), Kumar et al. (1997, 1999), Liu and Yanai (2001). The primary objective of the present article is to discern how the monthly maximum temperature (Tmax) over Kolkata is associated with the monthly TO. The newness of the present study can be summarized as follows:
- • to some extent, TO is a tracer for large-scale meteorological processes in the upper troposphere and lowermost stratosphere (Hoinka, 1998). In particular, TO tends to be highest on the cyclonic side of upper-level jet streams and in the region of isolated cyclonic vortices (cut-off lows). The observed connection between TO and tropopause height (TH) is valid not only on short time scales but also on long time scales. The increasing TH and the decreasing tropopause temperature are qualitatively associated with the decreasing trend of ozone in the stratosphere. It should be noted that the midtropospheric temperature is used as a proxy for the temperature at the earth's surface (Varotsos and Kirk-Davidoff, 2006). Also, greenhouse gases (GHGs) warm the earth's surface but cool the stratosphere radiatively and therefore affect ozone depletion. In addition, due to the ozone depletion, less heat would be absorbed in the stratosphere while, due to the increase in GHGs content, more heat would be trapped in the atmosphere;
- • in the existing literature on the climate over India, surface temperature has been used as a predictor for summer-monsoon rainfall (Liu and Yanai, 2001; Parthasarathy et al., 1990; Sikka, 1980) and TO has been studied for its trend (Sahoo et al., 2005) and inter-annual variability (Singh et al., 2002). However, despite the physical association between TO and temperature, no study till date has been attempted to forecast surface temperature using TO as predictor. The present study examined the association between TO and maximum surface temperature (Tmax) and predicted Tmax based on TO as predictor;
- • in the existing ANN applications, TO has always been the predictand with other meteorological parameters as predictors (Chaloulakou et al., 2003; Sousa et al., 2007). The present study developed ANN model with TO as predictor and meteorological parameter Tmax as predictand;
- • existing works compared the ANN with multiple linear regression models while forecasting TO (Comrie, 1997; Sousa et al., 2007). The present paper compared the performance of ANN with linear regression as well as non-linear regressions like quadratic regression, exponential regression and logistic regression;
- • in a work by Chattopadhyay et al. (2010a), univariate modelling of monthly Tmax over Kolkata was attempted and it was established that previous four months Tmax data can produce high Willmott's index when applied to a modular neural network. However, in the present paper no Tmax data for the previous month is required and only TO data of the previous month is considered as the predictor. Hence, a newness of the work is the less number of previous data.
The organization of the rest of the article is as follows: in Section 2, the data under consideration are analyzed through autocorrelation function, cross-correlation function, and through periodogram method of analysis. In Section 3, ANN models are generated to forecast Tmax based on TO as predictor. In Section 4, various regression models are generated and finally, it has been examined whether an extended range forecast of monthly maximum temperature can be estimated using ANN with its non-linear methodology.
2 Different statistical analyses of the data
2.1 Data
In the present paper, the data are derived from the measurements made by the Earth Probe Total Ozone Mapping Spectrometer (EP/TOMS). The EP/TOMS experiment provides measurements of the Earth's total column ozone by measuring the backscattered Earth radiance in the six 1 nm bands (NASA, 1998). The total ozone (TO) data are available at ftp://jwocky.gsfc.nasa.gov/pub/eptoms/data/overpass/OVP075_epc.txt. In this paper, January 1997 to December 2002 monthly TO data over Kolkata has been utilized. The corresponding monthly maximum temperature (Tmax) data for Kolkata have been collected from the website of India Waterportal (http://www.indiawaterportal.org/taxonomy/term/1216). The time series of Tmax and TO are plotted on Fig. 1.
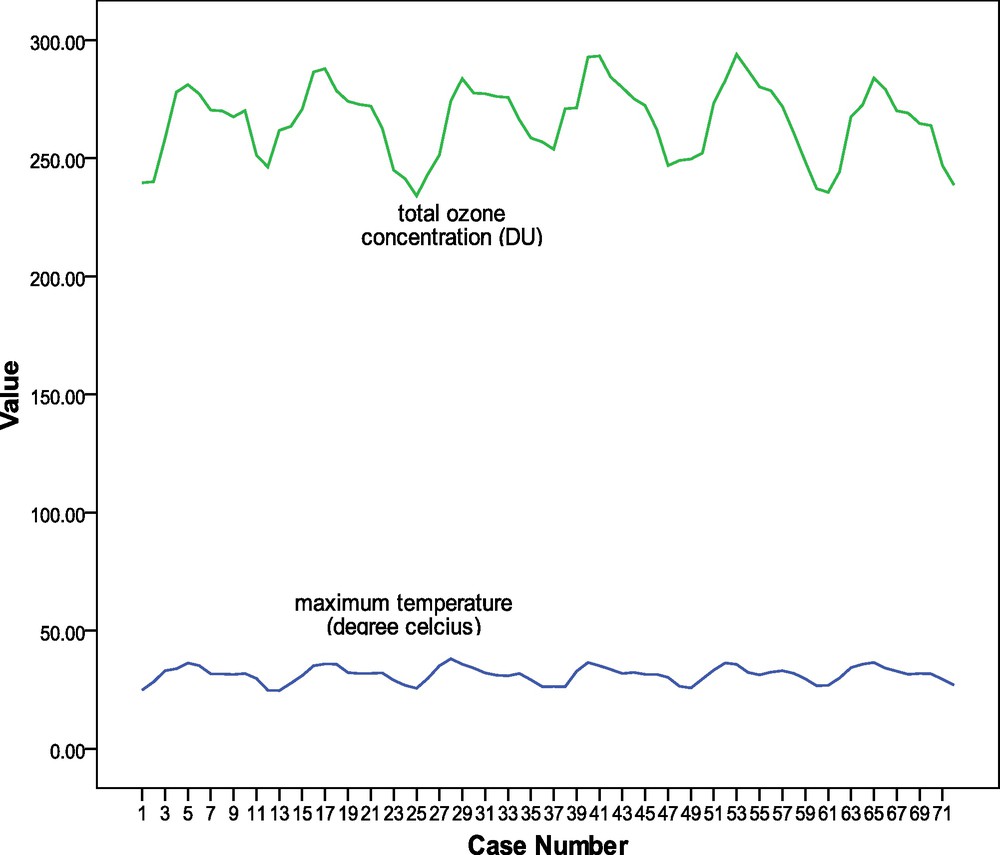
Time series of monthly maximum temperature (°C) and monthly total ozone concentration (DU) over Kolkata during the period 1997–2002.
Séries temporelles de température (°C) mensuelle maximum et de concentration (DU) mensuelle d’ozone total sur Kolkata, pendant la période 1997–2002.
2.2 Autocorrelation structure analysis
Atmospheric variables often exhibit statistical dependence with their own past or future values. In the terminology of the atmospheric sciences, this dependence through time is usually known as persistence. Persistence can be defined as the existence of (positive) statistical dependence among successive values of the same variable, or among successive occurrences of a given event (Wilks, 2006). Positive dependence means that large values of the variable tend to be followed by relatively large values, and small values of the variable tend to be followed by relatively small values. The persistence is measured by means of autocorrelation coefficients computed as (Wilks, 2006).
(1) |
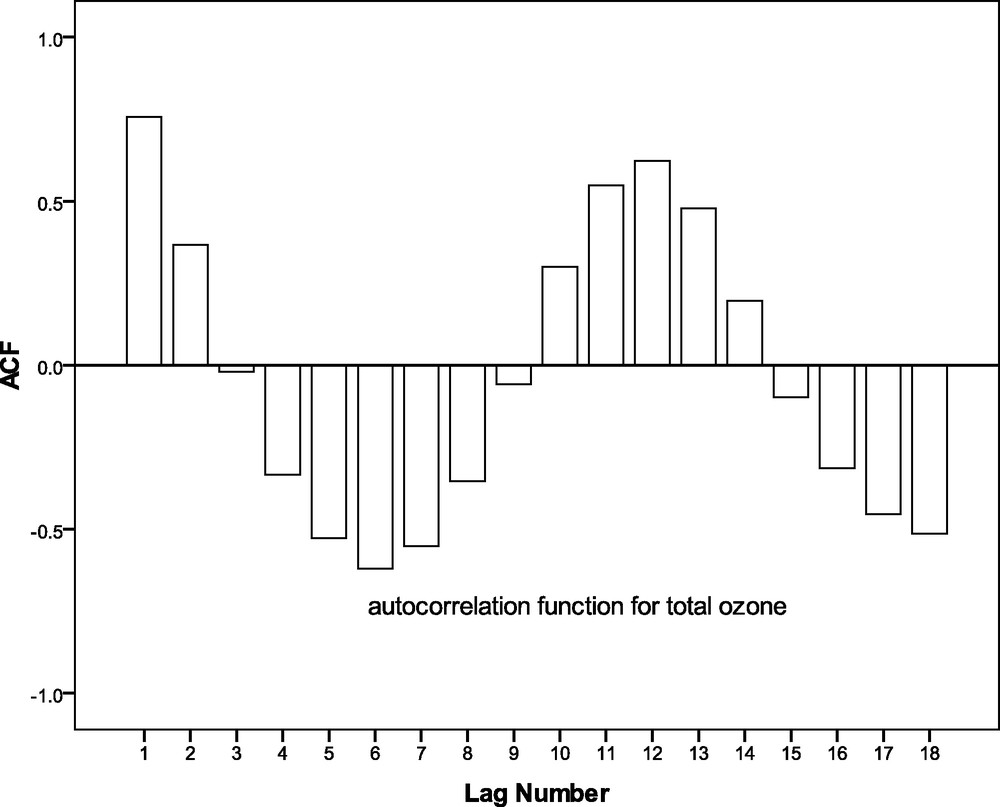
Autocorrelation function for the monthly total ozone concentration over Kolkata during the period 1997–2002.
Fonction d’autocorrélation pour la concentration mensuelle d’ozone total sur Kolkata, pendant la période 1997–2002.
In the case of Tmax over the study zone, it is found from Fig. 3, that the lag1 autocorrelation is 0.684, which indicates existence of significant persistence within the time series. At lag12, it is 0.772. However, in the negative side, the autocorrelation coefficients are not as large as they are in the case of TO. From Figs. 2 and 3, a similarity is observed between the TO and Tmax over Kolkata. Both the time series are completing a cycle in 12 months. Moreover, in both the cases, lag1 and lag12 autocorrelation coefficients are significantly high.
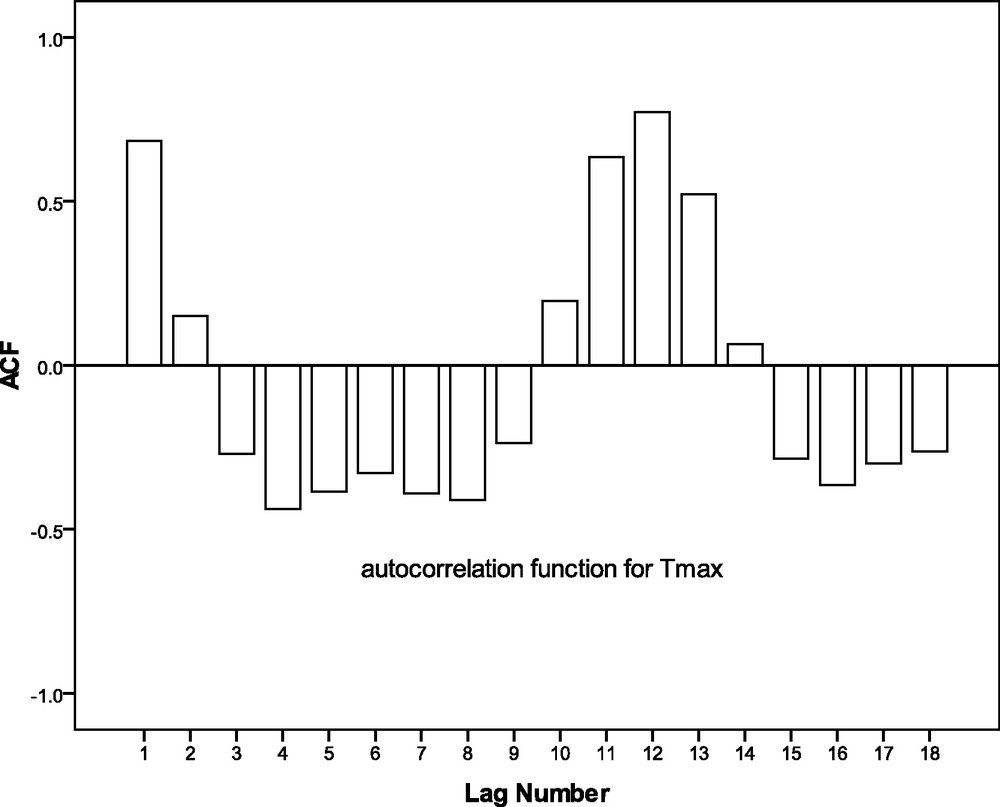
Autocorrelation function for the monthly maximum temperature over Kolkata during the period 1997–2002.
Fonction d’autocorrélation pour la température mensuelle maximum sur Kolkata, pendant la période 1997–2002.
The search for the relationships usually involves the calculation of a sample cross-correlation function (CCF) for the pairs of time series (Chang et al., 1997; Kripalani and Kumar, 2004). Considering the CCF between monthly TO and Tmax time series on Fig. 4, it is observed that the cross-correlation coefficients lie between –0.6 and 0.8. It should be noted that dominant negative and positive cross-correlation coefficients (CC) are existing. The pattern of CCF for the lags –7 to –3 and 3 to 7 are having similar patterns with high negative CC at the lags –5 and 7. A high positive CC is occurring at lag 0. It may be further noted that like the ACF, the CCF is also exhibiting a sinusoidal pattern. The CCs are prominent at 99% level of significance. From these observations, it can be interpreted that the Tmax and TO show significant degree of similarity in their temporal variation. Finally, it is observed that the CCF is symmetric about the horizontal axis representing the lags as negative and positive levels. The stability or symmetry of the CCF about the horizontal axis indicates a stable relationship between TO and Tmax. Hence, it is possible to generate a predictive model for Tmax using TO as predictor.
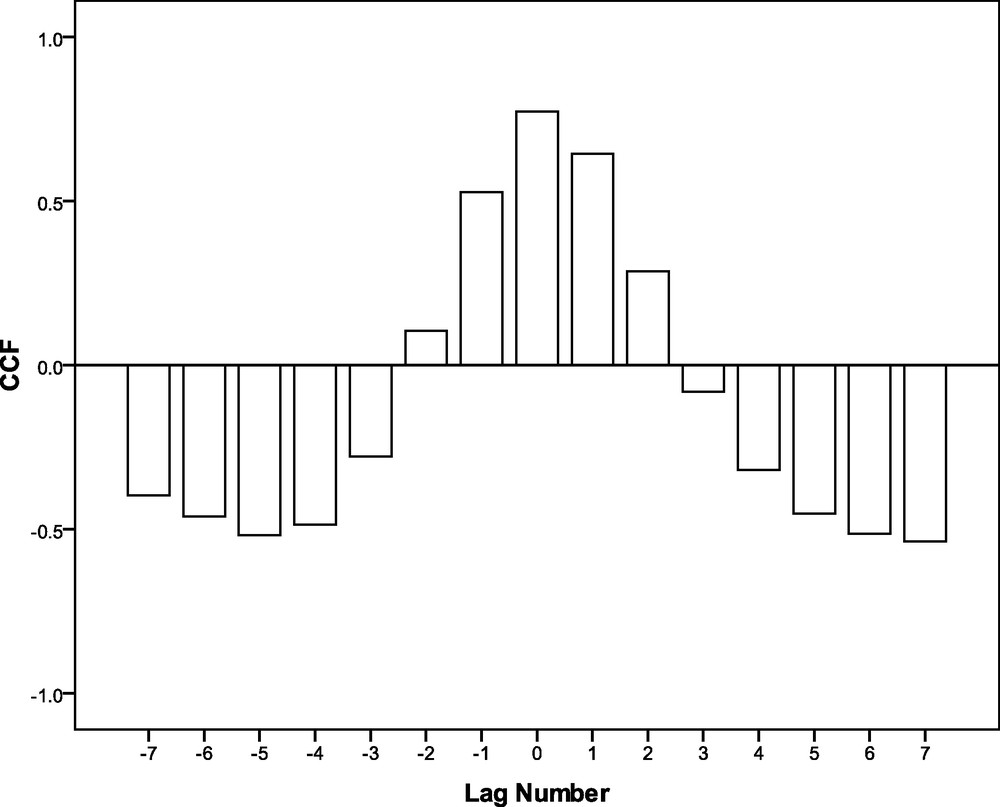
Cross-correlation coefficients for the monthly total ozone concentration and monthly maximum Temperature over Kolkata during the period 1997–2002.
Coefficients de corrélations croisées pour la concentration mensuelle d’ozone total et la température mensuelle maximum sur Kolkata, pendant la période 1997–2002.
2.3 Periodogram analysis
There have been literally thousands of attempts to find cycles in meteorological phenomena. Application of periodogram method in the analysis of time series of climatological data is well discussed in various papers (Gil-Alana, 2005; Marr and Harley, 2002; Prouse and Ervin, 1935; Seleshi et al., 1994; Vyushin et al., 2007). Any data series consisting of n points can be represented by adding together a series of n/2 harmonic functions as (Wilks, 2006).
(2) |
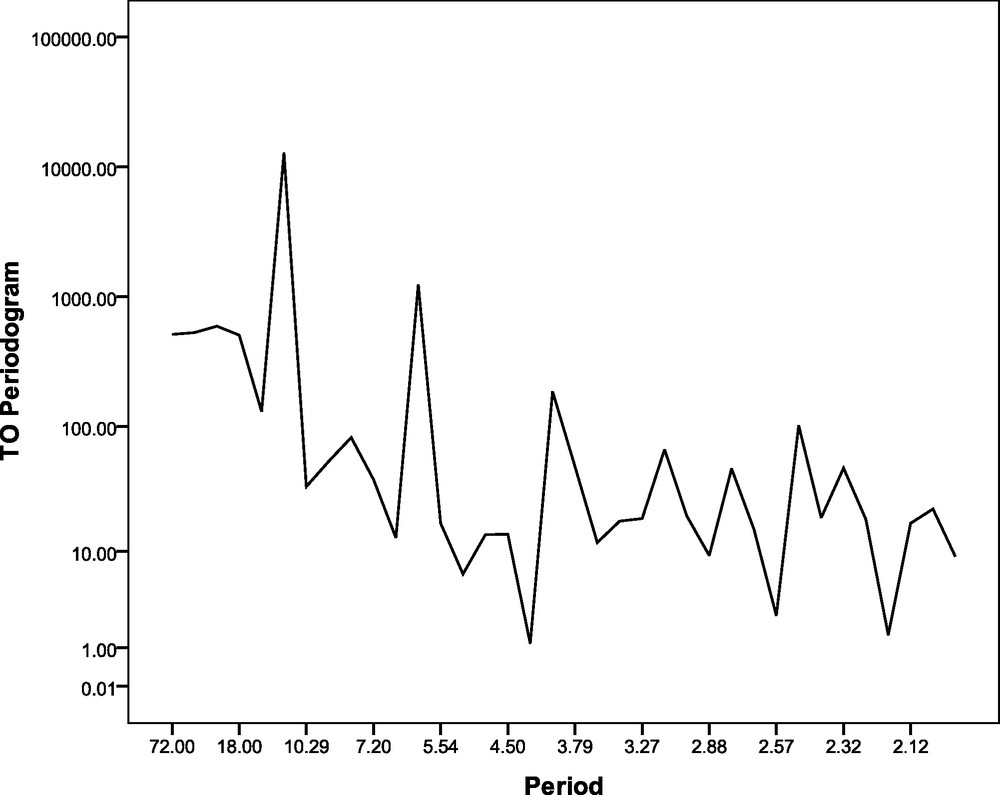
Periodogram analysis for the monthly total ozone time series over Kolkata during the period 1997–2002.
Périodogramme pour la série temporelle d’ozone total mensuel sur Kolkata, pendant la période 1997–2002.
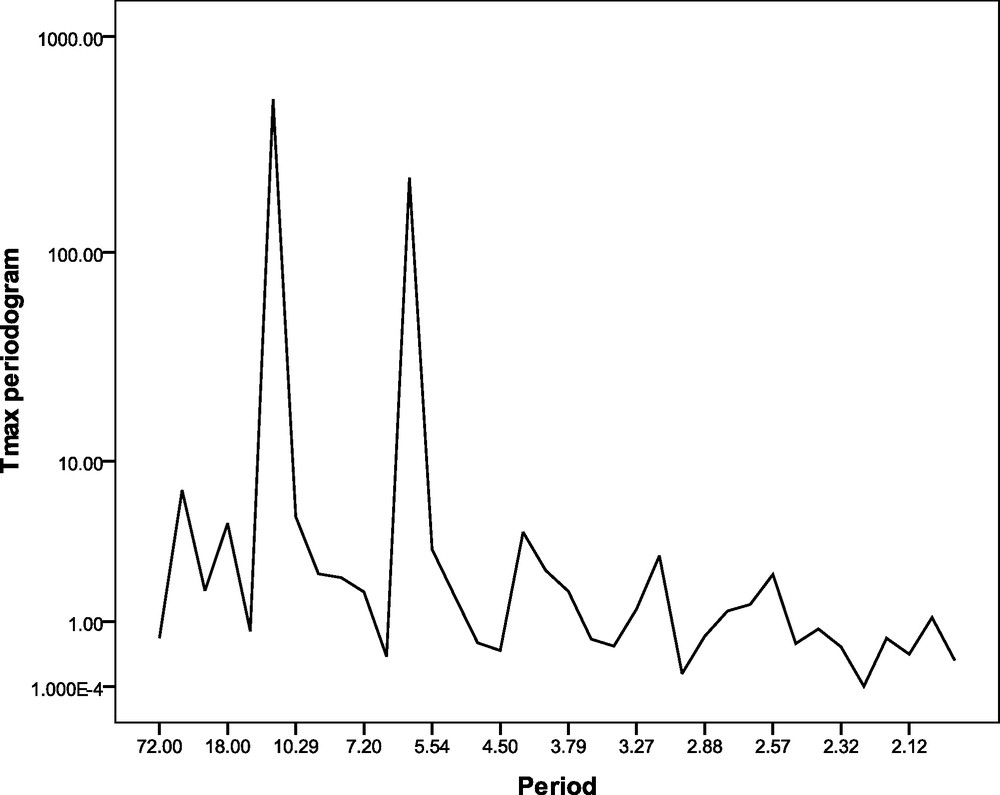
Periodogram analysis for the monthly maximum temperature time series over Kolkata during the period 1997–2002.
Périodogramme pour la série temporelle de température mensuelle maximum sur Kolkata, pendant la période 1997–2002.
In the subsequent sections, it will be examined whether a predictor-predictand relationship exists between TO and Tmax. To do the same, one has to examine whether Tmax of the given month can be estimated on the basis of TO of the previous months. The ANN would be implemented for this purpose. A brief overview and implementation procedure of ANN would be elaborated in the subsequent sections.
3 Artificial Neural Networks: a brief review
Fundamental development in the area of feed forward ANN during the period 1960–1990 was reviewed extensively by Widrow and Lehr (1990). Introduction of back propagation algorithm (Rumelhart and McClelland, 1986) opened up new avenues to the application of ANN in modelling various time series problems (Hsieh and Tang, 1998). In the simplest form, backpropagation training begins by presenting an input pattern vector to the network, sweeping forward through the system to generate an output response vector, and computing the errors at each output (Widrow and Lehr, 1990). The next step involves sweeping the effect of the errors backward. Finally, the weights are updated based on the corresponding error gradient. A wide review of application of backpropagation learning to the atmospheric problems is available in Gardner and Dorling (1998). Maier and Dandy (2000) presented an extensive review of the application of ANN in predicting hydrological time series. Several applications of ANN are available in meteorological forecasting problems like thunderstorm (Manzato, 2005; Marzban and Stumpf, 1996), rainfall (Chattopadhyay and Chattopadhyay, 2010a; Kuligowski and Barros, 1998), tide (Leea and Jeng, 2002), groundwater level (Coulibaly et al., 2001), evapotranspiration (Zanetti et al., 2007) etc. In Section 1, various examples of applications of ANN in modeling and forecasting of tropospheric ozone are discussed.
The present paper applies ANN in the form of multilayer perceptron (MLP) (Gardner and Dorling, 1998; Haykin, 2008; Rojas, 1996). In MLP, each network consists of several simple processors called neurons, or cells, which are highly interconnected and arranged in several layers. There are three basic types of layers: input layer, hidden layer(s), and output layer. Input and output layers are connected through hidden layer(s). There may be one to several hidden layers in between input and output layers. In mathematical form, the adaptive procedure of a feed forward MLP can be presented as (Kamarthi and Pittner, 1999).
(3) |
(4) |
The backpropagation algorithm in which the weights of the network are updated immediately after the presentation of each pair of input and target output is called the sequential learning. The other learning procedure in which the whole training set is considered as a batch is called the batch learning. Silverman and Dracup (2000) identified the advantages of ANN over conventional statistical methods as:
- • a priori knowledge of the underlying process is not required;
- • existing complex relationships among the various aspects of the process under investigation need not be recognized;
- • constraints and a priori solution structures are neither assumed nor enforced.
4 Implementation of Artificial Neural Network
Several conjugate gradient algorithms are there in the literature of ANN learning. Johansson et al. (1990) described in detail the theory of general conjugate gradient methods and how to apply the methods in feed-forward ANNs. Møller (1993) introduced a variation of a conjugate gradient method (Scaled Conjugate Gradient, SCG), which avoids the line-search per learning iteration by using a Levenberg-Marquardt approach in order to scale the step size. The Conjugate Gradient methods choose the search direction and the step size more carefully by using information from the second order approximation (Møller, 1993).
(5) |
(6) |
Since its introduction, the SCG has been used in several ANN applications for geophysical problems (e.g. Abraham and Nath, 2001; Khan and Coulibaly, 2006). In the present paper, SCG has been applied with sigmoid activation function . Advantage of the sigmoid function is the form of its derivative. Gardner and Dorling (1998) discussed the advantage of this activation. In Any model development process data pre-processing can have a significant effect on model performance. As the outputs of the logistic transfer function are between 0 and 1, the data are generally scaled in the range 0.1–0.9 or 0.2–0.8. If the values are scaled to the extreme limits of the transfer function, the size of the weight updates is extremely small and flatspots in training are likely to occur. Before developing the proposed ANN model for prediction of Tmax based on TO concentration, the data are scaled to [0.1,0.9] to avoid the asymptotic effect caused by the sigmoidal activation function. The scaling is done by using the transformation
(7) |
Minimization of mean squared error is taken as the stopping criterion for the ANN model. It is common practice to split the available data into two sub-sets; a training set and an independent validation set (Maier and Dandy, 2000). Typically, ANNs are unable to extrapolate beyond the range of the data used for training. One method, which maximizes utilization of the available data, is the holdout method (Maier and Dandy, 2000) that we have used in this article. The basic idea is to withhold a small subset of the data for validation and to train the network on the remaining data. Once the generalization ability of the trained network has been obtained with the aid of the validation set, a different subset of the data is withheld and the above process is repeated. Different subsets are withheld in turn, until the generalization ability has been determined for all of the available data (Maier and Dandy, 2000). In the present paper, we have divided the dataset into the ratio of 67:33 in order to use two third of the entire dataset as the training set and the remaining one third as the test set. As the generalization ability is determined for all of the available data, the prediction performance is measured by Willmott's index (WI) (Comrie, 1997; Chattopadhyay and Chattopadhyay, 2010b; Willmott, 1982) given by
(8) |
The usefulness of WI in meteorological modeling is discussed in Chattopadhyay and Chattopadhyay (2008b).
In the present ANN modeling problem with SCG learning, the data set contains the TO and Tmax data pertaining to January 1997 to December 2002. Thus, in monthly scales, there are 72 data points. In this data set, ANN model in the form of MLP will be generated. The first target is to examine whether it is possible to estimate Tmax of a given month using TO concentration of the immediately previous month. In this case, there would be 71 input patterns in the MLP model. The order of the input matrix would be (71 × 2). The first column of the input matrix corresponds to TO and the second column corresponds to the observed monthly Tmax, which is the target output of this supervised learning methodology. The MLP is learned through SCG with single hidden layer and the optimum size of the hidden layer, i.e., the optimum number of nodes in the hidden layer is recorded. After training and testing, the model is validated over the entire set of target outputs. The WI is found to be 0.863 and the value of R2 is 0.560. The actual Tmax and those predicted by this ANN model are plotted as line diagram in Fig. 7a. In this line diagram, it is observed that in some of the test cases, the actual and predicted values are almost coincident. In Fig. 7b, a scatterplot is presented to view the degree of linearity between the actual and predicted Tmax. A prominent positive slope is discernible in the scatterplot that indicates a good positive association between the actual and predicted Tmax. Calculating the percentage error of prediction in the test cases, it is found that in 83.1% and 70.4% test cases, the errors of predictions are below 5% and 2.5%, respectively. Thus, the predictions yields for 5% and 2.5% acceptable errors are 0.831 and 0.704 respectively. This further proves the goodness of fit of the ANN model in the form of MLP. The Pearson correlation coefficient between actual and predicted values is 0.748. From the above observation it is felt that it is possible to predict the Tmax over Kolkata for a given month predicted by the TO concentration of the immediately previous month. The WI and R2 values from this MLP model are presented pictorially in Fig. 9 along with those obtained from regression models, to be discussed in the next section.
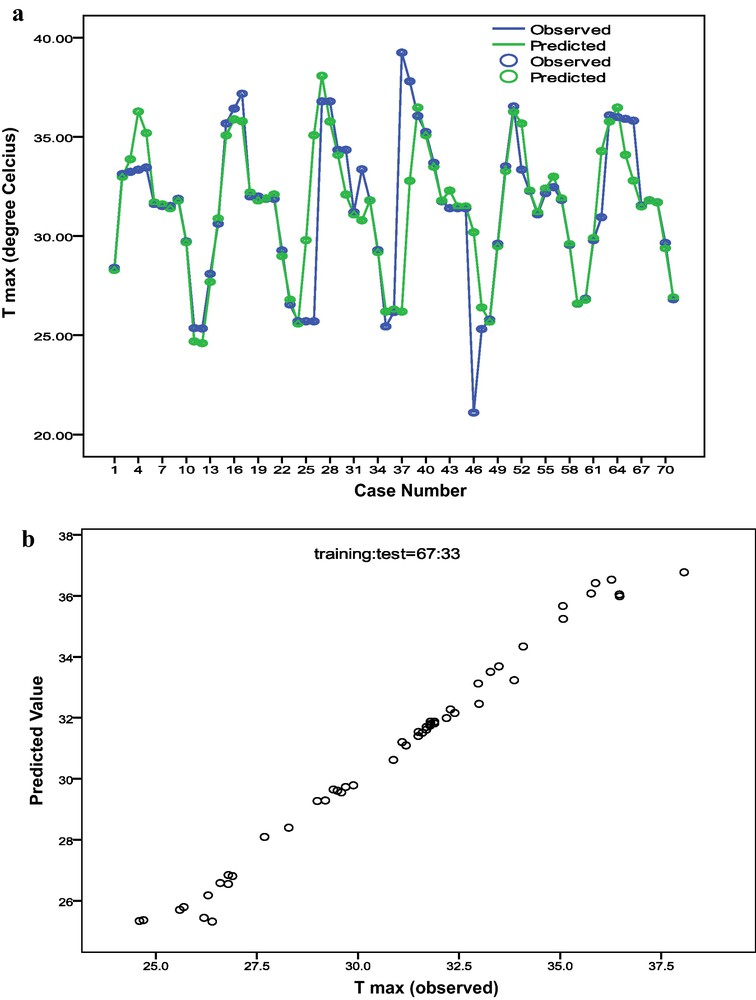
Diagrams showing the association between the actual monthly maximum temperature (̊C) (Tmax) and those predicted by multilayer perceptron model. The line diagram is plotted in 7a and scatterplot is presented in 7b.
Diagrammes montrant l’association entre température (̊C) mensuelle maximum actuelle (Tmax) et celle prédite par le modèle perception multi-couche. Diagramme linéaire en 7a et en nuage de points en 7b.
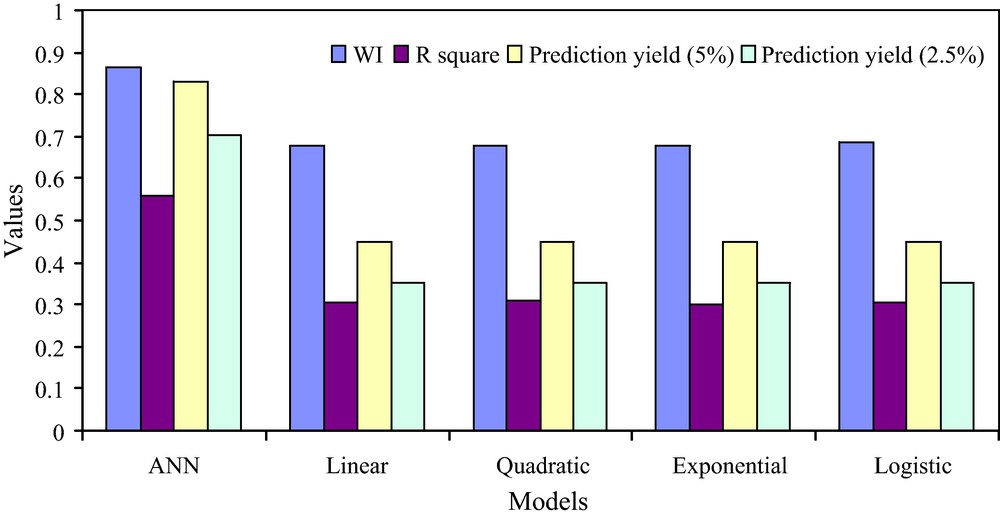
Values of different statistics measuring the suitability of different models in predicting the monthly Tmax over Kolkata based on total ozone as predictor.
Valeurs de différentes statistiques mesurant l’harmonisation des différentes méthodes dans la prédiction de la température mensuelle maximum sur Kolkata, sur la base de l’ozone total comme prédicteur.
A second model is generated by incorporating one more predictor in input matrix for the MLP. In this model, TO of months n and (n + 1) would be used to predict the Tmax of month (n + 2). Dividing the entire input matrix into training and test cases as above and implementing the identical training and test methodology, we generate the prediction model. In this case, WI is 0.866 and R2 is 0.564. The WI and R2 for the second model are almost equal to those of the first model. This indicates that the two models are of almost equal prediction capacity. However, the first model requires less number of predictors and hence it is more acceptable than any other model of similar prediction capacity and dependent on more number of predictors.
5 Comparison with regressions
In the present section, the performance of the first MLP model is compared with linear as well as non-linear regression models. Superiority of MLP in ozone forecasting over multiple linear regression is established in Agirre-Basurkoa et al. (2006). Non-linear regression models are chosen as quadratic regression, exponential regression and logistic regression. The regression equations are found to be as follows (Abdul-Wahab and Al-Alawi, 2002; Chattopadhyay et al., 2010a, 2010b;Comrie, 1997; Manel et al., 1999):
(9) |
(10) |
(11) |
(12) |
The coefficients of determination R2 for the above four regression models are computed as 0.306, 0.309, 0.311 and 0.322, respectively. For the above models, WI is calculated and the values are found to be 0.676, 0.678, 0.680 and 0.687, respectively. The line diagram presented on Fig. 8 shows the actual and predicted Tmax values. The line diagram shows that the linear as well non-linear regression equations captured the pattern of the observed Tmax time series. However, they are unable to yield predictions very close to the actual Tmax values especially when the Tmax value is very low or very high. Therefore, it may be concluded that the linear or non-linear regressions are unable to predict the extreme Tmax cases. The WI and R2 values corresponding to the regression models are presented on Fig. 9. It is observed from the above WI and R2 values that logistic regression performs better than the other three regression models. The prediction yields for the regression models are also presented in Fig. 9. It is observed that in all the regression models the prediction yields are below the ANN model when 5% and 2.5% error of prediction are allowed.
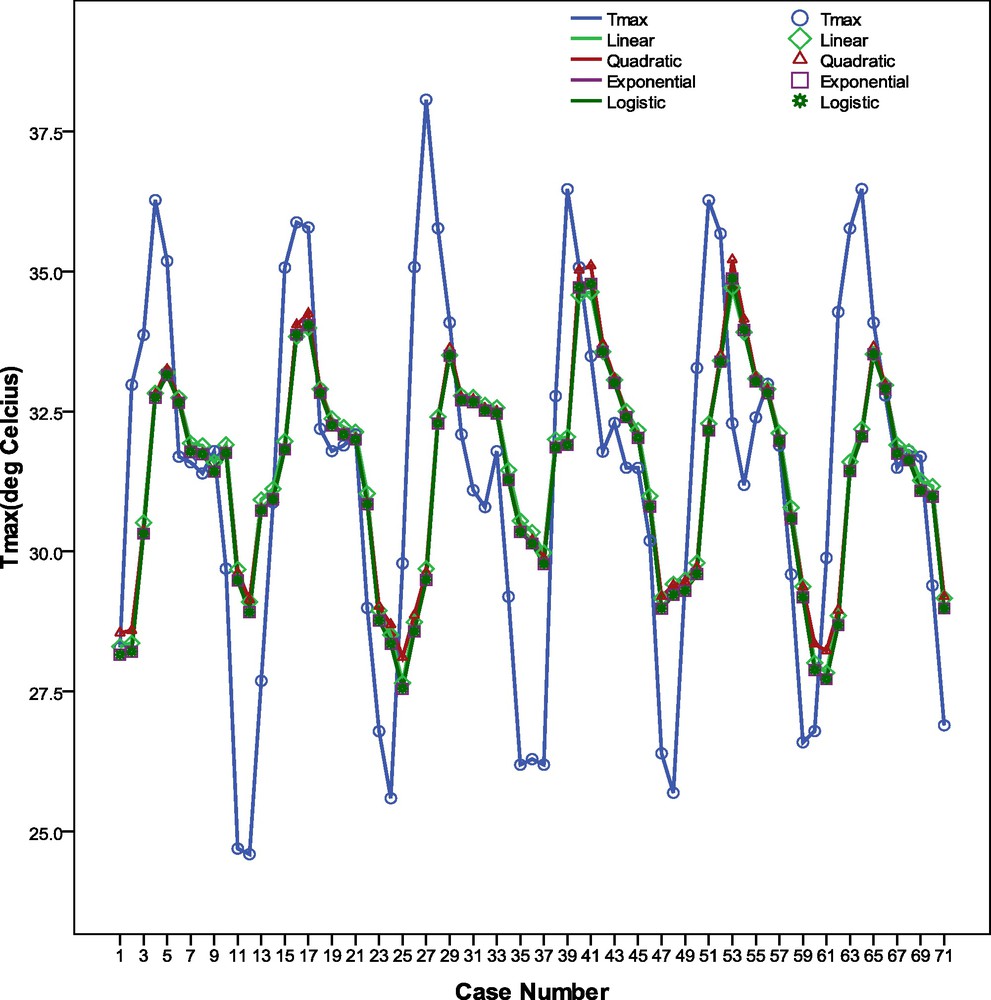
Diagram showing the association between the actual monthly Tmax (°C) and that predicted by conventional Regression model.
Diagramme montrant l’association entre la température mensuelle maximum actuelle Tmax (°C) et celle prédite par le modèle de régression conventionnel.
From the above discussion, supremacy of ANN over linear and non-linear regression models is established. To compare their performance in extreme cases, the percentage of error (PE) of prediction for the months having Tmax ≥ 35 °C and ≤ 25 °C are calculated for the first ANN model and the four regression models explained above. The PE are presented on Fig. 10, which shows that in 12, out of 16 cases, the PE produced by ANN lies below those produced by regression models. This established that ANN has higher potential than the regression models to make prediction of extremely high or low Tmax situations over the study zone.
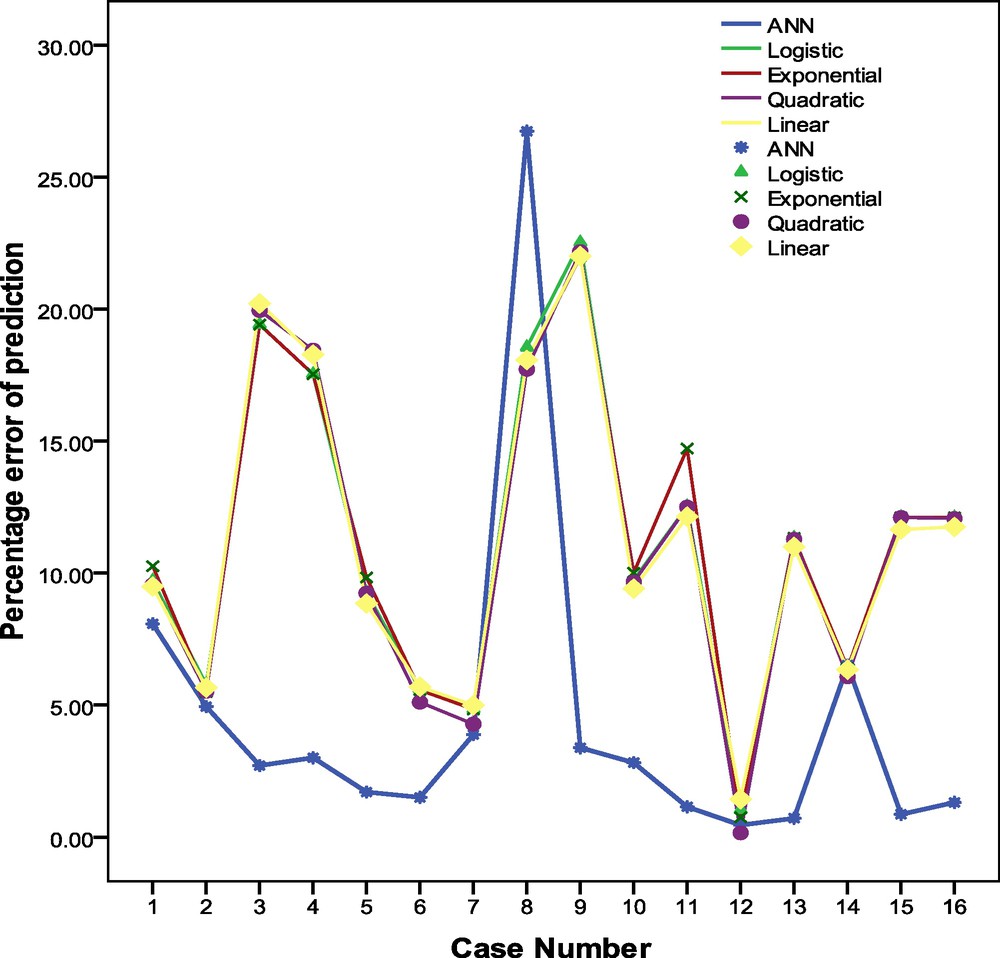
Schematic showing the percentage errors of prediction for the months in which Tmax ≥ 35 °C or Tmax ≤ 25 °C over Kolkata.
Diagramme montrant les erreurs en pour cent de prédiction pour les mois pendant lesquels Tmax ≥ 35 °C ou Tmax ≤ 25 °C sur Kolkata.
6 Conclusion
In the present article, an attempt has been made to analyze the monthly maximum temperature over Kolkata using monthly total ozone concentration as predictor. The interrelation between the predictors and the predictand has been investigated through autocorrelation, cross-correlation, and periodogram. Two ANN models have been generated in the form of MLP using scaled conjugate gradient learning with sigmoid activation function. The Willmott's indices of two MLP models are 0.863 and 0.866, respectively. The Willmott's index for the second model is almost equal to that of the first model. This indicates that the two models are of almost equal prediction capacity. However, the first model requires less number of predictors and hence it is more acceptable than any other model of similar prediction capacity and dependent on more number of predictors. To examine the skillfulness of the first MLP model, its performance has been finally compared with linear regression model and non-linear regression models in the forms of quadratic, exponential and logistic regression and the efficiency of the first model has been established. The conclusion is that, the first MLP model has considerable potential for estimating monthly Tmax using monthly total ozone concentration as predictor.
Acknowledgements
The authors acknowledge with thanks the financial support from Indian Space Research Organization (ISRO) through S.K. Mitra Centre for Research in Space Environment, University of Calcutta, Kolkata, India for carrying out the study.