1 Introduction
The sediment transfer time in alluvial plains is a key parameter for the understanding and modelling of the evolution of the continental surface and its response to external forcing factors i.e. climate changes, tectonic events or human activities. Besides modelling, experimental and tracing approaches have been developed to estimate more or less directly these time-dependent sediment parameters, including the sediment basin response time to external perturbations (e.g., Castelltort and Van Den Driessche, 2003; Castelltort et al., 2004; Davy and Lague, 2009; Liébault et al., 2012; Métivier and Gaudemer, 1999; Paola et al., 1992; Sear et al., 2000). A more accurate estimate of these parameters can be obtained by using radiochronometers. The application of cosmonuclides would undoubtedly open new horizons in this field of research (Wittmann et al., 2011); however, so far, their sediment analysis has mainly been devoted to the estimation of denudation rates at the watershed scale (e.g., von Blanckenburg, 2005, 2006). On the other hand, an effort has been made in recent years to develop and use radioactive U-series disequilibria (i.e., 238U-234U-230Th). DePaolo et al. (2006, this issue and references therein), developed the concept of the comminution age based on the analysis of the (234U/238U) activity ratio which allows one to constrain the time “elapsed subsequent to bedrock being reduced by physical weathering to small grains”. Several other studies underline the potential of such an approach (e.g., Dosseto et al., 2010; Lee et al., 2010; Maher et al., 2006). At the same time, it has been proposed to use the variation of the U-series nuclides in sediments along rivers to recover the time constant of the sediment transfer within alluvial plains (Chabaux et al., 2006; Dosseto et al., 2006; Granet et al., 2007, 2010). The potential of these latter approaches has been emphasized in the study of the sediments from the Ganges river system (Granet et al., 2007, 2010), one of the world's largest purveyor of Quaternary alluvial sediments shed from the largest orogen, i.e., Himalaya. The analysis of sediments collected along the Ganges river and two of its Himalayan tributaries allowed one to define a transfer scenario with time constants for the sediment transfer which vary strongly in function of the sediments granulometry.
The aim of this article is to present the principles of this approach and to discuss some possible limits in the light of new data from samples collected at different locations within the Ganges watershed and its outlet.
2 Principle of the approach
The potential of U-series nuclides to provide information on the transfer time of sediments in alluvial plains is a consequence of the dual property of these nuclides: (1) to be fractionated during water-rock interactions and hence during chemical weathering processes; and (2) to have radioactive decay periods of the same order of magnitude as the time-scales of these processes (e.g. Chabaux et al., 2003a, 2008; Dosseto et al., 2008a). The approach, illustrated in the study of sediments from the Ganges river basin (Granet et al., 2007, 2010), mainly relies on the principle that in large alluvial plains sediments are only transferred along the streams and not affected by additional inputs of new weathering products from fresh bedrocks; in such a case, the intensity of 238U-234U-230Th disequilibria in river sediments will only depend on two parameters: (a) the duration of the transfer including the time spent in soils and in the river; and (b) the nature and the intensity of U-Th fractionations occurring in sediments during their transfer into alluvial plains. In the limiting case of a pure transport regime, without secondary U-Th fractionations, Th activity ratios of the sediments collected along the stream are only affected by radioactive decay. In this simple case, the determination of the sediments Th activity ratios along the river yields directly the “age”, which corresponds to the residence time of the sediment in the plain. If secondary U-Th fractionation occurred during the transit of the sediments within the alluvial plain, then the time information derived from the variation of U-Th disequilibria in sediments along the alluvial plain requires the identification of the origin of these secondary U-Th fractionations and their modelling by using realistic mathematical laws. For the sediments of the Ganges river system (Granet et al., 2007, 2010) it has been shown that the main U-Th fractionations are related to sediment weathering during their transit and storage in the plain. The interpretation of U-Th data relies in this case on a time evolution law of the U-series nuclides, which is the classical law used to account for the variations of the radioactive disequilibria during weathering, namely a loss and gain model (e.g., Chabaux et al., 2003b, 2011, 2012; Dequincey et al., 2002; Dosseto et al., 2008b; Ma et al., 2010, 2012). In the frame of such a scenario the temporal evolution of the number of the atoms of the four 238U-234U-230Th-232Th nuclides per gram of sample is described by the four following equations:
(1) |
(2) |
(3) |
(4) |
λi is the decay constant (in y−1) of nuclide i (here 238U, 234U, 230Th, and 232Th), ki is the first-order rate constant (in y−1) for leaching of nuclide i and fi is the input flux (in y−1) of nuclide i gained by the sample. For simplification, the input fluxes are expressed as the proportion of the number of atoms of nuclides added per year to the initial sample (expressed as λi N0).
Solving the above equation system allows one to calculate the theoretical activities of the various nuclides in the sample at time t and the three independent activity ratios (234U/238U), (230Th/238U), and (230Th/232Th). The nuclide activities and the activity ratios are a function of the mobility parameters (ki, fi) of the model, the initial activities of the different nuclides and the time t. The time t corresponds to the time span between the initial state of the sample at t = 0 and its present state. Thus, the time t corresponds to the time duration elapsed for a sample to move from the starting position to its current position. Usually the reference sample is collected at a locality upstream in the plain, which corresponds to the starting or reference position. It is important to note that such an approach implies that the transfer of sediments within the plain works at steady state.
The mobility parameters (ki, fi) are usually unknown. The approach retained to solve this problem is to collect a series of samples along the alluvial plain and to consider the mobility parameters as constant for the whole plain. The determined radioactive disequilibria of these samples are then used to determine: (1) the mobility parameters of the different radionuclides; and (2) the age of the different samples relative to the reference sample; this age corresponds to the mean residence time of the sample in the alluvial plain and defines the time span necessary to move from the reference position to its current position.
In the studies of the Ganges sediments and its tributaries, this mathematical approach allowed one to derive a long sediment transfer time of 100 ky or more for coarse-grained sediments; the fine-grained material, however, has a much shorter transfer time of a few ky (Granet et al., 2007, 2010).
3 Sampling locations and mineralogical and geochemical characteristics of sediments
In order to complement the first results obtained on the Ganges river system and to validate the previous conclusions concerning the transfer time of sediments within the alluvial Gangetic plains (Granet et al., 2007, 2010), additional sediments have been sampled in three other areas during several field campaigns (between 2004 and 2009) and analyzed. They come: (1) from Rishikesh and Kanpur situated at the upstream portion of the Ganges river; (2) from Harding Bridge at the outlet of the Ganges river just before the confluence with the Brahmaputra river; and (3) from the Kosi river, with one sample collected at the outlet of the Range and another one just before the confluence with the Ganges River (Fig. 1).

Map of the Ganges River basin and locations of river sampling, modified from Chabaux et al. (2001). MCT: Main Central Thrust; MFT: Main Frontal Thrust. Location numbers are those given in Table 1. Stars give the location of samples studied by Granet et al. (2010), whose results are given in insert of Fig. 2.
Carte du bassin du Ganges avec la localisation des échantillons (d’après Chabaux et al. (2001)). MCT : « Main Central Thrust » ; MFT : « Main Frontal Thrust ». Les numéros des positions sont ceux indiqués dans le Tableau 1. Les étoiles correspondent aux lieux de prélèvement des échantillons étudiés par Granet et al. (2010), dont les données sont représentées en encart de la Fig. 2.
For each sampling location, both suspended and bedload or bank river sediments (Table 1) were collected and analyzed. Bedload sediments were dredged in the center of the channel, whereas bank sediments, which correspond to the most recent deposits, were sampled in the active part of the channel. River bank sediments, which actually are rather bar sediments, have been collected at several places in the same sampling location, in order to integrate a total surface of about a few square meters. Suspended materials were collected at different depths of the water column by using a polypropylene bottle, ballasted and equipped with a depth probe. The sampling depth given in Table 1 corresponds to the average depth recorded during the filling of the bottle and may fluctuate by ± 0.5 m. Suspended sediments are collected by filtration of water samples through a 0.22 μm with a diameter of 99 mm PES filter in pressurized teflon coated filtration units within 12 to 48 h of collection (Galy et al., 2008; Granet et al., 2010; Lupker et al., 2011). To assess the temporal variability of U-Th series nuclides of the sediments during the monsoon season when 70–80% of the total annual rainfall occurs (Singh et al., 2007), the Ganges river at its outlet (Harding Bridge) was sampled four times (once in July 2004 and three times in 2008).
Données des séries de l’U pour les sédiments de la partie amont du Ganges, des affluents Kosi, Chambal et Gomti et du Ganges à son exutoire (Harding Bridge).
Type | Sampling date | Depth m | U ppm | Th ppm | (234U/238U) | (230Th/232Th) | (238U/232Th) | (230Th/238U) | Th/Nd | |
Ganges | ||||||||||
Rishikesh (#1, 30°7.60′ N, 78°19.82′ E) | ||||||||||
BR922 | SL | Aug. 2009 | 0.2 | 4.58 | 15.7 | 1.004 | 0.988 | 0.883 | 1.12 | 0.60 |
BR920 | SL | Aug. 2009 | 6 | 4.38 | 15.3 | 0.997 | 1.030 | 0.868 | 1.19 | 0.57 |
BR924 | Bank | Aug. 2009 | 2.91 | 12.5 | 1.003 | 0.754 | 0.704 | 1.07 | 0.56 | |
Kanpur (#2, 26°36.78′ N, 80°16.55′ E) | ||||||||||
BR907 | SL | Aug. 2009 | 0 | 3.85 | 17.8 | 1.001 | 0.998 | 0.655 | 1.53 | 0.59 |
BR945 | SL | Aug. 2009 | 3 | 2.77 | 12.7 | 1.001 | 0.901 | 0.659 | 1.37 | 0.57 |
BR905 | Bedload | Aug. 2009 | – | 1.87 | 10.7 | 1.007 | 0.628 | 0.530 | 1.19 | 0.55 |
Harding Bridge (#3, 24°3.17′ N, 89°1.48′ E) | ||||||||||
BR415 | SL | July 2004 | 0 | 3.78 | 19.2 | 1.018 | 0.773 | 0.596 | 1.30 | |
BR413 | SL | July 2004 | 4 | 3.43 | 18.5 | 1.010 | 0.703 | 0.564 | 1.25 | |
BR412 | SL | July 2004 | 6.5 | 3.15 | 17.2 | 1.014 | 0.716 | 0.555 | 1.29 | |
BR418 | Bedload | July 2004 | 10 | 6.03 | 44.1 | 0.998 | 0.446 | 0.415 | 1.07 | |
BR8218 | SL | 1st Sept. 2008 | 2 | 2.77 | 17.4 | 1.016 | 0.658 | 0.482 | 1.37 | |
BR8217 | SL | 1st Sept. 2008 | 4 | 2.72 | 17.4 | 1.010 | 0.633 | 0.476 | 1.33 | |
BR8216 | SL | 1st Sept. 2008 | 7 | 2.69 | 17.6 | 1.009 | 0.612 | 0.462 | 1.33 | |
BR8221 | Bedload | 1st Sept. 2008 | 12 | 5.11 | 41.7 | 1.004 | 0.397 | 0.372 | 1.07 | |
BR8253 | SL | 10th Sept. 2008 | 0 | 2.86 | 18.0 | 1.018 | 0.702 | 0.483 | 1.45 | |
BR8251 | SL | 10th Sept. 2008 | 5 | 2.63 | 17.2 | 1.012 | 0.623 | 0.464 | 1.34 | |
BR8281 | SL | 22th Sept. 2008 | 0 | 3.02 | 18.8 | 1.015 | 0.753 | 0.488 | 1.54 | |
BR8280 | SL | 22th Sept. 2008 | 6 | 2.97 | 18.9 | 1.015 | 0.657 | 0.476 | 1.38 | |
BR8283 | Bedload | 22th Sept. 2008 | 12.5 | 2.38 | 16.4 | 1.007 | 0.485 | 0.442 | 1.10 | |
Ganges tributaries | ||||||||||
Chambal (#4, 26°39.45′ N, 77°54.15′ E) | ||||||||||
BR938 | Bank | Aug. 2009 | – | 1.24 | 8.47 | 1.010 | 0.524 | 0.446 | 1.18 | |
Gomti (#5, 25°34.66′ N, 83°59.92′ E) | ||||||||||
BR8126 | SL | Aug. 2008 | 0 | 4.00 | 19.6 | 0.993 | 0.747 | 0.619 | 1.21 | |
Kosi Upstream (#6, 26°50.89′ N, 87°9.08′ E) | ||||||||||
PB68 | SL | July 2005 | 0 | 4.37 | 23.0 | 1.005 | 0.630 | 0.576 | 1.09 | 0.52 |
PB65 | SL | July 2005 | 3 | 4.26 | 21.8 | 1.003 | 0.635 | 0.594 | 1.07 | 0.54 |
PB70 | Bank | July 2005 | – | 2.68 | 14.9 | 0.994 | 0.560 | 0.547 | 1.02 | 0.51 |
Kosi Downstream (#7, 25°25.12′ N, 87°13.66′ E) | ||||||||||
BR103 | Bedload | Aug. 2001 | – | 3.44 | 25.0 | 1.008 | 0.475 | 0.418 | 1.14 | 0.55 |
The bedload or bank sediments (grain size of ∼200–500 μm) are mainly composed of quartz, feldspar, mica and heavy minerals (zircons, xenotimes, monazites and garnet) (Garzanti et al., 2010; Singh, 2009; Singh et al., 1993, 2007). Suspended sediments (grain size of ∼0–50 μm) are enriched in mica, contain some calcite, dolomite and fewer amounts of epidote, amphibole and garnet (details in Garzanti et al., 2011; see also Singh et al., 2007).
Sedimentological analysis of the river sediments collected in the Ganges basin from the Himalayan front downstream to the Ganges mainstream in Bangladesh suggests that mineral sorting strongly controls the chemical composition of the sediments (Lupker et al., 2011, 2012a). Grain size, shape and density of the sediment suspension are defined by the hydro-dynamical conditions of the water column. This results in a mineralogical and thus chemical differentiation of sediments at the scale of the water column; it is illustrated through the variations of major elements at the scale of the water column (for example, decrease of Al2O3 and increase of SiO2 with increasing depth). Major element data further indicate that sediments from Gangetic plain rivers (Ganges river, Himlayan rivers in the downstream part of the plain) suffered a higher degree of chemical weathering than the sediments from the Himalayan rivers at the outlet of the High Range (Lupker et al., 2011, 2012a).
4 Analytical techniques
U, Th concentrations and U, Th isotopic ratios were analyzed in Strasbourg (LHyGeS) by Thermal Ionisation Mass Spectrometry (TIMS) on a Thermo Scientific Triton. To improve the digestion of sediments, samples were crushed by using first an agate disk mill and then an agate ball mill. The grain size of the sample is < 100 μm with the first crushing step and decreases to < 50 μm after the second step. This is of prime importance in order to get a full digestion of the samples, in particular of the minerals such as zircons, xenotimes and monazites which are U and Th-rich.
For U and Th analyses, 100 mg of crushed powder were spiked with a mixed 233U-229Th tracer. The U-Th tracer was regularly calibrated by TIMS with the AThO rock standard. The sample-spike mix was then dissolved using a three-step procedure with HNO3-HF acids, HClO4 and HCl–H3BO3 acids (Granet et al., 2007, 2010; Pelt et al., 2008). Separation and purification of the U and Th fractions were achieved by using conventional anion exchange chromatography (Granet et al., 2007; Pelt et al., 2008). The accuracy and reproducibility of (234U/238U) activity ratio were controlled by analyzing the HU1 secular equilibrium standard solution. During the period of data acquisition (2008–2010), the mean (234U/238U) ratio of the HU1-standard was 0.999 ± 0.005 (n = 45; 2σ), which is in agreement with the value of 1 for a rock in secular equilibrium. During the course of this study, the accuracy of Th isotopic ratio measurements was assessed by analysing the BRGM Th105 standard solution (Innocent, 2008): 232Th/230Th =220316 ± 4480 (n = 27, 2σ). The reproducibility of U concentration and activity ratios was better than 2 and 0.5%, respectively. For Th, the reproducibility of concentration and activity ratios was better than 2%. The total procedure blanks were < 50 pg for U and < 500 pg for Th. This is negligible (< 2‰) compared to the amounts of elements analysed in the studied samples (at least 100 ng and 1000 ng of U and Th, respectively).
5 Results and discussion
5.1 Variations of U and Th nuclides in the upstream part of the Ganges and Kosi rivers
The U and Th isotope systematics in the sediments of the Kosi and of the upstream Ganges rivers are very consistent with those obtained previously, for the Ganges main stream, and two of its tributaries, the Gandak and Ghaghara rivers (Granet, 2007; Granet et al., 2007, 2010). Similar to previous studies, the data points of each sampling site define trends in the isochron diagram (Fig. 2) that can be interpreted in terms of mixing of coarse- and fine-grained sediments. In addition, the data imply a systematic upstream to downstream variation of the 238U-234U-230Th disequilibria in the sediments with different variation trends for the two sediment end-members: both the (230Th/232Th) and (238U/232Th) activity ratios decrease from upstream to downstream in the coarse-grained sediments, whereas only the (238U/232Th) ratio decreases significantly in the fine-grained suspended sediment (Fig. 2). This indicates that the upstream-downstream variation of 238U-234U-230Th disequilibria in the sediments along the rivers, with different variation trends for suspended and coarse-grained sediments, is probably a general feature of all Himalayan rivers flowing across the Indo-Gangetic plain. Therefore, using the new Ganges and Kosi river data, one might expect to arrive at interpretations and conclusions similar to those of Granet et al. (2007, 2010): sediment transfer time within the watershed varies as a function of the sediment grain size, with much shorter transfer time for the fine-grained than for the coarse-grained sediment.

Plot of the sediment data points in the (230Th/232Th) vs (238U/232Th) diagram for the sediment samples from Rishikesh (black squares), Kanpur (white triangles), Kosi downstream (grey triangles) and Kosi upstream (grey squares) rivers. Larger symbols are used to indicate bedload and bank river sediments, whereas the same but smaller symbols are used to indicate suspended river materials. Insert: data from Granet et al. (2010) for the Narayani-Gandak river sediments.
Représentation des données dans le diagramme (230Th/232Th) vs (238U/232Th) pour les sédiments de rivière collectés à Rishikesh (carrés noirs), Kanpur (triangles blancs), la Kosi aval (triangles gris), la Kosi amont (carrés gris). Les symboles plus gros indiquent les sédiments de fond/sédiments de berge, les mêmes symboles mais plus petits sont utilisés pour les sédiments en suspension. Encart : données de Granet et al., 2010 pour les sédiments de la Gandak et du Ganges.
5.1.1 Sediment transfer time calculation
A more quantitative estimate of the sediment transfer time can be made by using the loss and gain model, detailed in section 2, and already applied in the previous studies on Ganges plain sediments (Granet et al., 2007, 2010). This approach is basically similar to those developed for interpreting the variations of U series disequilibrium in weathering profiles (e.g. Chabaux et al., 2003b, 2011, 2012; Dequincey et al., 2002; Dosseto et al., 2008b, 2012; Ma et al., 2010, 2012). Such approaches allow for solving systems of significantly under-constrained equations, which is the general situation for all these studies. Thus, in the case of the Kosi and the Upper Ganges, the U-Th analysis of only two sediment samples per river, yields three independent activity ratios, namely (238U/232Th), (234U/238U) and (230Th/232Th) activity ratios, which is obviously insufficient to precisely determine the age of the downstream sample relative to the upstream one, and the eight mobility parameters of the model. The solving approach, developed for solving such under-constrained problem, aims to find a set of plausible parameters (here the mobility parameters ki, fi of the four nuclides 238U, 234U, 230Th, 232Th) and the age of the downstream sediment relative to the upstream one) from an a priori set of parameters, which fits best the activity ratios in a least square frame work. In the present study, the solving approach developed to perform the determination of these unknown parameters is a parallel stochastic Quantum-behaved Particle Swarm Optimization (Mikki and Kishk, 2005; Sun et al., 2004). To our knowledge, it is probably the first time that such an approach is used for this kind of problem in geochemistry. Such a method is well-adapted: (1) for situations where large numbers of parameters and low numbers of data are available (present case); and (2) for cases where an a priori relationship can be proposed for the different parameters, or at least some of them (more detail in Appendix A). For modelling the Ganges and the Kosi river data, some a priori information can be indeed provided on the values of the U and Th mobility parameters. The Th/Nd elemental ratios (Nd can be seen as a relatively immobile element) of the coarse-grained and fine-grained sediments are relatively invariant from upstream to downstream locations (Fig. 3). This implies that sediment weathering during their transfer and residence within the alluvial plain does not significantly mobilize Th, which is clearly not the case for U. This therefore justifies considering Th to be immobile (kTh and fTh ≈ 0). Furthermore, from the literature data it can be seen that the kU parameters range from 10−7 to 10−4 y−1 (e.g., Andersen et al., 2009; Dequincey et al., 2002; Dosseto et al., 2008b; Vigier et al., 2001). In the present study, we expanded the a priori interval of variations for kU to the interval 10−9–10−3 y−1. In addition, as in the Ganges system, the U activity ratios of waters are > 1 and < 1.5 (Chabaux et al., 2001; Granet et al., 2007), the k234/k238 ratios and f234/f238 ratios (with k and f the model mobility parameters) are also suggested to be in range between 1 and 1.5. Thus f234/f238 ratios can indeed be assimilated to the activity ratios of the incoming U input whereas k234/k238 ratios represent the differential removal of 234U relative to 238U for leaching processes. Therefore, both ratios should have values close to those of river water of the studied region.
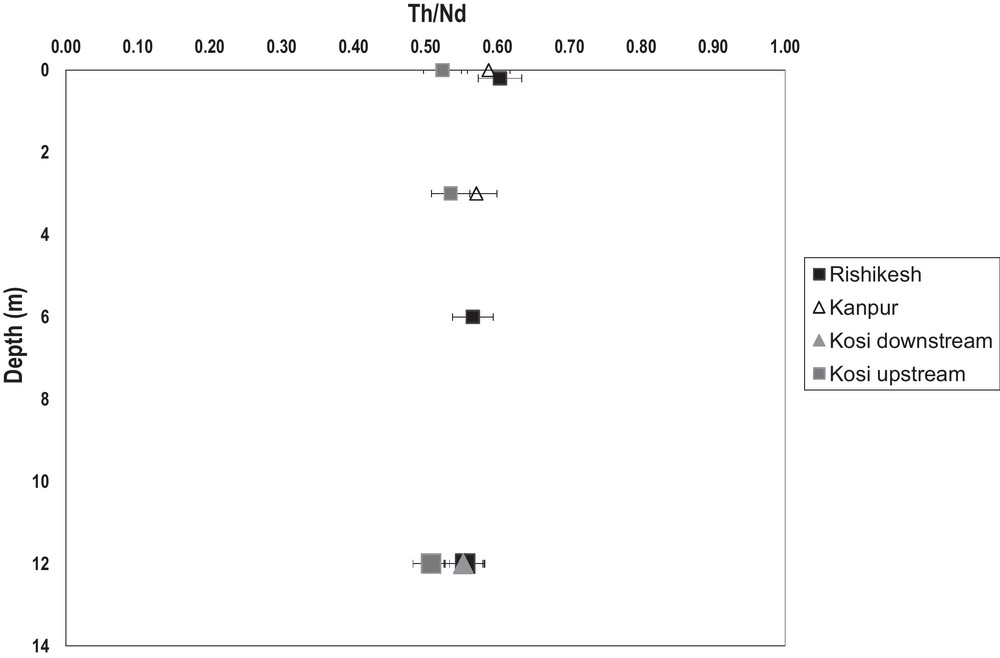
Depth-variations of Th/Nd ratios for the sediments of the Rishikesh (black squares), Kanpur (white triangles), Kosi downstream (grey triangles) and Kosi upstream (grey squares) sampling locations. As no depth has been allocated to the bedload, these latter and bank samples were defined for this graphic at a depth of 12 m.
Variations des rapports Th/Nd en fonction de la profondeur pour les sédiments de rivière collectés à Rishikesh (carrés noirs), Kanpur (triangles blancs), Kosi aval (triangles gris) et Kosi amont (carrés gris). Comme les profondeurs des sédiments de berge et de certains sédiments de fond ne sont pas définies, une valeur arbitraire de 12 m a été utilisée.
Solving Eqs. (1)–(4) with the above intervals of a priori values for the mobility parameters leads to transfer times of 90 ky to 170 ky for bedload sediments collected between Rishikesh and Kanpur (with k238 leaching coefficients ranging from 10.3 × 10−5 y−1 to 0.66 × 10−5 y−1) (Fig. 4, Table 2a), and from 100 to 170 ky for the Kosi river (with a k238 parameter ranging from 4.3 × 10−5 y−1 to 1.3 × 10−5 y−1) (Fig. 4, Table 2b). The results of the model also suggest that the different mobility parameters depend on each other and on the transfer time. Thus, the above values of sediment transfer time for both Ganges and Kosi rivers are linked to the value of the k238 leaching parameters (Fig. 5a), whereas the ratios k238/f238 and k234/f234 are correlated with each other (Fig. 5b).
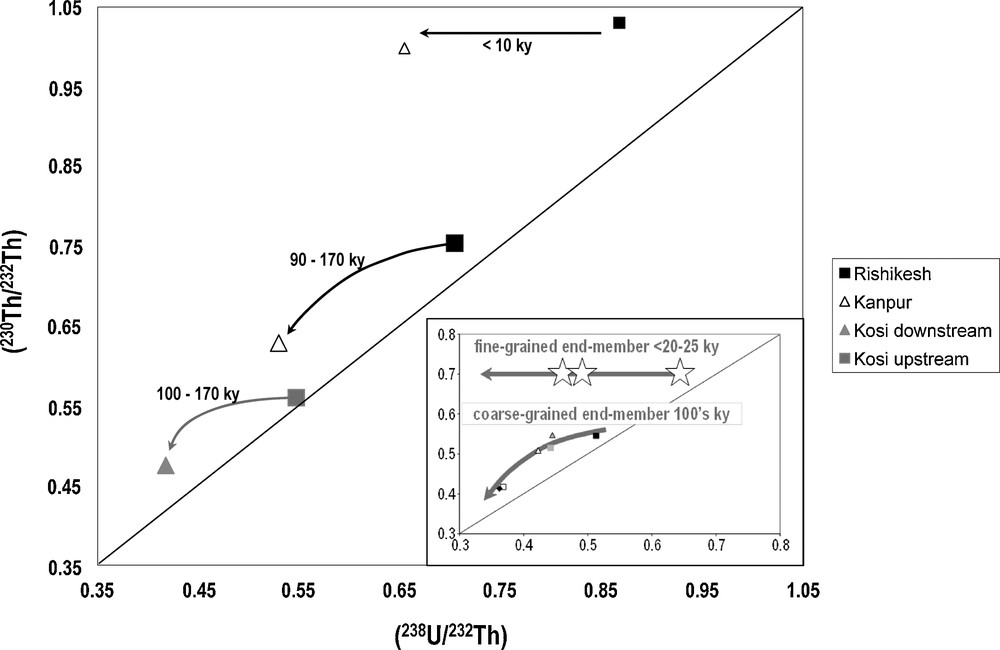
Time interpretation for the U-Th variations of river sediments in the (230Th/232Th) vs (238U/232Th) diagram. Insert: previous estimates based on U-Th data from Gandak and Ganges sediments (Granet et al., 2010).
Interprétation en terme de temps de transfert des variations U-Th des sédiments dans le diagramme (230Th/232Th) versus (238U/232Th). Encart: estimations faites à partir des données U-Th des sédiments de la Gandak et du Ganges (Granet et al., 2010).
Temps de transfert et paramètres de mobilité (ki, fi) obtenus par modélisation pour les sédiments grossiers collectés dans le Ganges amont (a), dans la Kosi (b) et pour les particules fines du Ganges amont (c). La gamme de variation de chacun des paramètres correspond aux valeurs minimales et maximales obtenues à partir de 7500 sets de solutions retenues via l’approche présentée en annexe.
Time (ky) |
k238 (10−5 y−1) |
k234 (10−5 y−1) |
f 238 | f 234 | k234/k238 | f234/f238 |
Upper Ganges between Risikesh and Kanpur (coarse-grained sediments) |
||||||
93 | 10.3 | 11.4 | 7.70 | 8.60 | 1.1 | 1.1 |
169 | 0.66 | 0.87 | 0.42 | 0.60 | 1.3 | 1.4 |
Kosi (Upstream-Downstream) (coarse-grained sediments) |
||||||
104 | 4.3 | 6.0 | 3.2 | 4.6 | 1.4 | 1.4 |
167 | 1.3 | 1.3 | 0.94 | 1.0 | 1.0 | 1.1 |
Upper Ganges between Risikesh and Kanpur (fine-grained sediments) |
||||||
0.38 | 71.9 | 72.8 | 1 × 10−8 | 1 × 10−8 | 1.0 | 1.0 |
5.4 | 14.3 | 18.6 | 7.50 | 11.0 | 1.3 | 1.5 |

(a) Plot of the k238 parameter against transfer time for the 7500 plausible solutions derived for the model applied to the coarse-grained sediments of the Upper Ganges. (b) Plot of the k234/f234 ratio against the k238/f238 ratio for the 7500 plausible solutions derived for the model applied to the coarse-grained sediments of the Upper Ganges.
(a) Variation du paramètre k238 en fonction du temps de transfert pour les 7500 solutions plausibles obtenues à partir du modèle appliqué sur les sédiments grossiers du Ganges amont. (b) Variation du rapport k234/f234 en fonction du rapport k238/f238 pour les 7500 solutions plausibles obtenues à partir du modèle appliqué sur les sédiments grossiers du Ganges en amont.
Even if it is clear that supplementary sediment samples would be required to get a better precision of the coarse-grained sediment transfer time, the important point to stress here, is that the new data obtained for the Upper Ganges and Kosi rivers confirm the long transfer time of bank sediments within alluvial plains of the Ganges river system (Granet et al., 2007, 2010). This result might be surprising in the light of 10Be data for instance (Lupker et al., 2012b), which indicates that the denudation rates are one order of magnitude shorter than the above U/Th transfer times. A specific study comparing the results of the U-Th and 10Be methods is certainly required to constrain the origin of such an apparent discrepancy. This, however, is beyond the scope of this study.
The same solving approach applied to the fine-grained sediment end-member from the upstream part of the Ganges, between Rishikesh and Kanpur, gives a residence time < 10 ky, with a k238 leaching coefficient of about 10−6 y−1 (Table 2, Fig. 4). Once again, this is very similar to the maximum residence time proposed for the fine-grained sediments at the scale of the Narayani-Gandak river (in Granet et al., 2010). It can, however, be noticed that the above calculation has been performed with sediments which are not totally similar in terms of grain size, and hence that this maximum value could be underestimated. This is nevertheless not really important, as it will be shown in section 5.2 that the fine-grained sediment transfer time can be constrained independently.
5.1.2 Origin of the upstream–downstream U and Th variations
In the frame of the above scenario, which assumes that the budget of U and Th nuclides in the sediments of the Indo-Gangetic plain rivers is controlled by both the secondary weathering processes modifying the sediments during their transfer across the plain and the duration of this transfer, the new data imply that: (a) the (230Th/232Th) and (238U/232Th) activity ratios of sediments eroded along the Himalayan range and carried by the rivers can be different from one river to another when entering the plain; and (b) that Th and (238U/232Th) activity ratios of the fine-grained and coarse-grained sediments can also differ within a single river, when entering the plain. If these activity ratios record those of the source rocks, from where the sediments originate, then the data indicate that the lithologies controlling the U-Th budget of the sediments have variable (238U/232Th) and hence (230Th/232Th) activity ratios at the scale of the Himalayan Range. The Sr isotope ratios of the sediments at the outlet of the Himalayan range show much stronger variations than those of Nd (Lupker, 2011; Lupker et al., submitted), and are most likely the result of variable contributions of a “Lesser Himalaya” (LH) component to these sediments, as these rocks are known to be characterized by high Sr isotopic ratios (Singh et al., 2008, and references therein). However, it has been shown that the (238U/232Th) activity ratios of the three main Himalayan structural units (i.e. the High Himalayan Crystalline, the Lesser Himalaya and the Tethys Sedimentary Series) are quite similar (Granet et al., 2007). This implies similar average U/Th and hence Th activity ratios for the source of Himalayan sediments, and hence does not favor the above scenario which assumes that the variations of the Th and U/Th ratios of the sediment at the outlet of the Himalayan Range reflect the U-Th variations of the sediments source rocks. Nevertheless, it is worth noting that the rock database used for constraining the mean U/Th ratios of the three main Himalayan lithologies is probably not entirely representative of the whole Himalayan Range, as it mainly comprises rocks from the Central Nepal Himalaya. In addition, it is not perfectly adapted to estimate the U/Th ratios of these units, as the budget of U and Th in sediments could be significantly affected by the presence of minor lithologies such as leucogranites and black shales, which are U and Th-rich (Chabaux et al., 2001; Singh et al., 2003). The geographical repartition of such specific lithologies is not perfectly known. Their occurrence along the High Range might control the variations of the (238U/232Th) and (230Th/232Th) activity ratios in the river sediments at the outlet of the Range. The analysis of pebbles collected in different Himalayan rivers, as done for the Narayani-Gandak river (Granet et al., 2010), could provide additional information to test this hypothesis. But at this stage and keeping in mind the above limitations, the data base for estimating the average U/Th ratio of the Himalayan structural units rather excludes a scenario that considers the variations of the (238U/232Th) and (230Th/232Th) activity ratios in the Himalayan sediments at the outlet of the High Range to be the result of the variabilities of the U-Th signatures of the source rocks.
Therefore, as an alternative, we propose that the sediments, which arrive in the Indian plain, have been weathered sufficiently long time to allow for a modification of their U-Th values. It has been shown that the sediments carried by the Kali Gandaki river, one of the main Nepalese streams flowing within the Himalayan range, have been weathered for a relatively long period (up to 100 ky) before their removal and transport by the rivers (Granet et al., 2007). These weathering processes can fractionate U and Th and produce sediments with (238U/232Th) and (230Th/232Th) activity ratios different from those of the unaltered Himalayan rocks. In this case, variations in the intensity of these weathering processes along the High Range might explain why the sediments of the different Himalayan rivers have different U/Th ratios when they enter the Indian plain. The difference observed in the U-Th systematics of fine-grained sediments and coarse-grained sediments in the Himalayan rivers at the outlet of the range would then indicate that in function of the granulometry: (1) the sediments originate from different geographical areas or from different pedological horizons; or (2) the sediments are transported over different time-scales. In order to better constrain these hypotheses, it is now of prime importance to study in detail the U-Th systematics of the sediments from the High Range of the Himalaya.
5.2 Variations of the U and Th isotopes ratios in the fine-grained sediments at the outlet of the Ganges river
As for sediments of the two other sampling locations, the Ganges sediments collected at its outlet, before the confluence with the Brahmaputra, display a relatively wide range of variations in their (238U/232Th) and (230Th/232Th) activity ratios, with low values in bedload sediments and high values in the suspended sediments (Table 1, Fig. 6a). In the isochron diagram (Fig. 6a), the data points also define linear trends, which again might reflect mixing (or sorting) relationships between a coarse-grained and a fine-grained sediment end-member. In detail, however, the data points plot along different mixing trends, relatively close to each other for all the suspended sediments collected in 2008, whereas the 2004 data points plot clearly along a different trend. Such a difference suggests that the U-Th characteristic of the fine-grained sediment end-members can vary significantly from one year to another and probably also within a year; significant variations of the (238U/232Th) and (234U/230Th) ratios are observable, whereas the Th and U activity ratios remain more or less invariant for the different sampling periods (2004 and 2008). If the U-Th variations between sediments collected in 2008 and 2004 do not result from a sampling bias, they imply that the transfer of sediments for the Ganges is not stationary over a few years, or even a few months. The immediate corollary is that the transfer time of the fine-grained end-member of the Ganges sediments or at least of one of its components is much shorter than 10 to 20 ky (Granet et al., 2010; previous section), probably of the order of the year or less.

(a) Plot of the sediment data points in the (230Th/232Th) versus (238U/232Th) diagram for Harding bridge samples. Larger symbols are used to indicate bedload and bank river sediments, whereas the same but smaller symbols are used to indicate suspended river materials. (b) Comparison with data points of suspended sediments collected in the Chambal river, the Himalayan rivers at the outlet of the Himalaya range, and the Himalayan river in the plain.
(a) Représentation dans le diagramme (230Th/232Th) versus (238U/232Th) des données des sédiments collectés à Harding Bridge. Les symboles plus gros indiquent les sédiments de fond/sédiments de berge, les mêmes symboles mais plus petits sont utilisés pour les sédiments en suspension. (b) Comparaison avec les données des sédiments en suspension pour la Chambal, les rivières himalayennes en sortie de chaine, et les rivières himalayennes dans la plaine.
Data source: this study–Granet et al., 2010.
The geochemical variations in the fine-grained sediment end-member are not only reflected by the U/Th ratios but also by major element concentrations and deuterium isotope ratios in sediments regularly collected at the outlet of the Ganges over the 2002–2010 years/period (Lupker et al., 2012a). These latter are assumed to be the result of changes in the proportion of sediment contributions from the South Ganges tributaries (mainly the Chambal river) and the Himalayan rivers. Although the number of Chambal sediments analyzed for U and Th is small (this study; Sarin et al., 1990), the position of these sediment data points in the isochron diagram and in the (234U/238U) versus (230Th/238U) diagram (not shown) indicates that this assumption cannot be upheld as an explanation for the U-Th variations in the Ganges sediment end-member. In the isochron diagram for instance, the data points representative of the Chambal suspended sediments plot between the suspended 2008 and 2004 sediments and, therefore, cannot be a mixing end-member (Fig. 6b). An alternative mixing scenario, which could be proposed to explain the difference between 2004 and 2008 suspended sediments (Fig. 6b), would be to interpret the particular U-Th characteristics of the 2004 sediments by a stronger sediment contribution from upstream Ganges tributaries relative to plain sediments (sediments from Gandak and Ghaghara river on the Indian plain for instance). However, again, this scenario fails when looking at the position of the data in the (234U/238U) vs (230Th/238U) diagram (not shown).
Not only for these reasons, but also because the temporal variations are observed for the (238U/232Th) activity ratios (and 230Th/238U ratios) and not for the (230Th/232Th) (nor the (234U/238U) ratios) at the outlet of the Ganges, we suggest that these variations may reflect annual changes in the degree of weathering of the Ganges suspended sediments. In such a scenario the lower U/Th ratios of the 2004 suspended sediments point to a smaller U loss and hence a smaller degree of weathering than in the case of the 2008 suspended sediments. Such an explanation is consistent with the observation that the amount of carbonates in the 2004 sediments (6 to 8%) is higher than in the 2008 sediments (3–4%) (carbonate concentrations in Lupker et al., 2012a). It may be related to the fact that in 2004 the monsoon was much less intense across the whole Indo-Gangetic plain than in 2008 (data from the India Meteorological Department, http://imd.gov.in/section/hydro/distrainfall/districtrain.html). Such a relation between the weathering intensity of sediments and the rainfall intensity is quite possible in view of the relationships between weathering fluxes carried by rivers and the river discharge observed at both regional or local scale (Millot et al., 2002; Olivia et al., 2003; Viville et al., 2012 and references therein).
5.3 U-Th disequilibrium in the bedload sediments of the Ganges outlet
As observed in the isochron diagram (Fig. 6), the (238U/232Th) and (230Th/232Th) activity ratios of the bedload sediments collected at the outlet of the Ganges basin vary significantly over the 2004–2008 period. The range of variation is smaller than observed for the upstream and dowstream sediments of the Upper Ganges or the Kosi river. It is, however, very similar to the upstream-downstream variation in the Gandak river sediments (Granet et al., 2007). Such annual variations within a single location are unexpected for a scenario of long sediment transfer time (≈ 100 ky or more) within the plain and might question the reliability of such estimates.
Looking in more details at the data, one observes that the two samples with the lowest (238U/232Th) and (230Th/232Th) activity ratios are the most enriched in U and Th (Table 1). In addition, these two samples have relatively high Zr and Hf concentrations, whereas the sample with the highest (230Th/232Th) activity ratio has lower Zr content (unpublished data, France-Lanord personal communication). This certainly indicates that the observed variations result from variation in the proportion of minor heavy minerals within the sediments; Garzanti et al. (2010) have indeed shown that Th and U in bedload sediments are mainly contained in minor minerals (zircon, monazite, allanite and xenotime), whereas in the suspended sediments they are mainly carried by clay minerals. The rock dissolution and grinding procedure was modified a few years ago to avoid the existence of residual and undissolved minerals (Granet et al., 2010) and, thus, also applied in this study. It allows for a complete dissolution of minerals; this is validated by U and Th concentration reproducibility tests of Ganges river sediments, but also by the good consistency obtained in this study between the Th concentrations determined by TIMS and by ICP-MS (Fig. 7); the ICP-MS determinations have been performed after a complete digestion of the sample by Li metaborate fusion. We therefore consider that the observed variation of the Th and U/Th activity ratios in the Ganges sediments over the 2004–2008 period is real and not a consequence of analytical bias. Such a variation might point to a very fast transfer of the coarse sediments (or at least a part of it) within the Ganges basin as already proposed for the fine-grained sediment end-member. In this case, the upstream-downstream variation reported for the (230Th/232Th) and (238U/232Th) activity ratios in sediments of the Ganges tributaries (Kosi river, Ghaghara river, Gandak river, Upper Ganges river) can no longer be interpreted in terms of long transfer time. They should rather be interpreted in terms of mineral sorting along the rivers flow path (with logically preferential upstream depositions of heavy minerals). Th and U/Th activity ratio variations in the sediments at the Ganges outlet suggest that the heavy minerals have low (230Th/232Th) and (238U/232Th) ratios. This would imply, in contrast to what is expected, that sediments in the alluvial plain are more enriched in heavy minerals than the upstream sediments. In addition, the Sr and Nd isotopic data in the sediments of the Gandak river do not point to any upstream-downsteam mineral sorting (Granet et al., 2007). Similarly, the REE patterns normalized to Upper Continental Crust values in the sediments of the Ganges tributaries do not really change from upstream to downstream, whereas it should vary if there was mineral sorting involving heavy minerals such as zircon (Fig. 8). Thus, all these observations and reasonings suggest that the (230Th/232Th) and (238U/232Th) activity ratio variations in the sediments from the Ganges tributaries cannot be interpreted in terms of mineral sorting, and hence reflect long transfer time for the sediments within the plain. It is therefore a priori difficult to involve short sedimentary transfer time to account for the U-Th variations observed in the bedload collected at the Ganges basin outlet over the period 2004–2008. We propose for this reason that such variations might simply be linked to the sampling procedure of the bedload by dredging, which would not allow for the collection of a representative sediment sample especially with respect to the amount of minor minerals. The difference in the mineral densities, combined with the local dynamics of stream waters might cause local redistributions of sediments and minerals on the river bottom with formations of “placer” enriched in heavy minerals and “anti-placer” zones depleted in heavy minerals (Garzanti et al., 2010). Thus, dredging might imperfectly sample all these different sediment types and result in an artificial mineralogical and, hence, U-Th variability when different bedload sediments have been collected at different periods. The real impact of such a sampling bias is, however, difficult to correctly assess on the basis of our data alone. It obviously creates an additional uncertainty on the estimated sediment transfer time. However, it seems that estimates based on bank sediment analysis and not on dredged bedloads, as done for the Gandak and Ghaghara rivers, are probably more reliable. Indeed such samples are composite samples (see Section 3), which probably allow one to get a more representative coarse-grained sediment than by dredging.
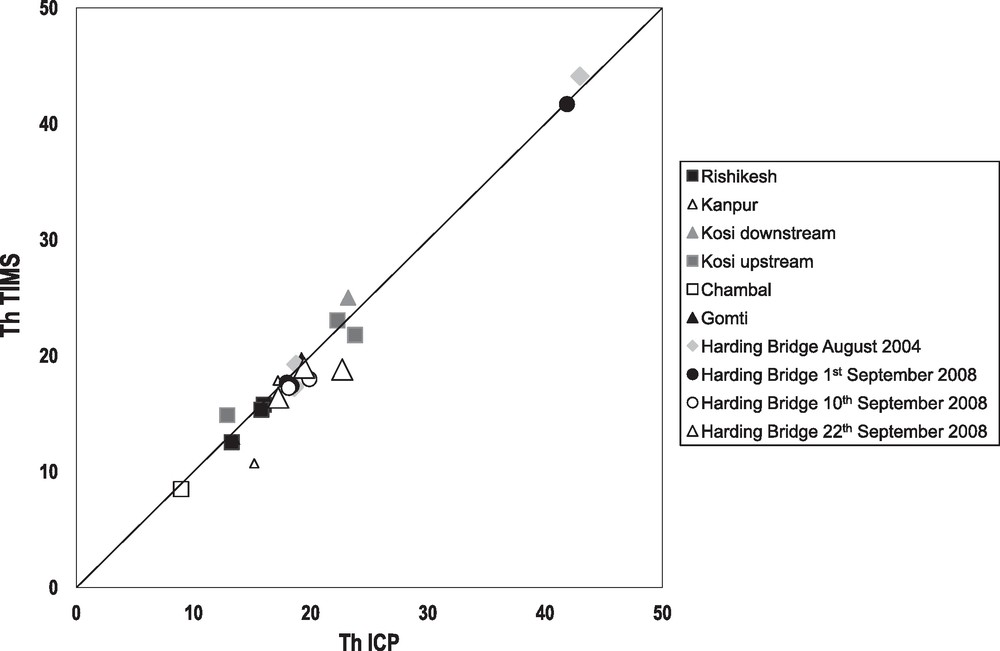
Comparison of the Th concentration measurements of bank and bedload sediments by TIMS and ICP-Ms. TIMS data were obtained by isotope dilution and acid dissolution in Strasbourg (this study). ICP-Ms data were analyzed at Centre de Recherches Pétrographiques et Géochimiques (CRPG) in Nancy (France) after complete digestion of the sample by Li metaborate fusion. (France-Lanord, pers. com.)
Comparaison des concentrations en Th dans les sédiments grossiers mesurées par TIMS et par ICP-MS. Les données par TIMS ont été obtenues après dilution isotopique et dissolution acide à Strasbourg (cette étude). Les données par ICP-MS ont été obtenues au Centre de Recherche Pétrologique et Géochimique (CRPG) à Nancy (France) après fusion au métaborate de Li (France-Lanord, com. pers.).

REE variations normalized to Upper Continental Crust values for the bedload or bank sediments from Rishikesh (black squares), Kanpur (white triangles), Kosi downstream (grey triangles) and Kosi upstream (grey squares) rivers. (REE data: France-Lanord, pers. com.)
Variations des terres rares normalisées aux valeurs de la croûte continentale supérieure pour les sédiments grossiers collectés à Rishikesh (carrés noirs), Kanpur (triangles blancs), Kosi aval (triangles gris) et Kosi amont (carrés gris) (données : France-Lanord, com. pers.).
6 Conclusions
The new U and Th data presented in this study for suspended and coarse-grained sediments (bedload and bank sediments) from the Upper Ganges and the Kosi rivers are entirely consistent with the 238U-234U-230Th-232Th systematics obtained previously for the Ganges main stream and two of its tributaries (Granet et al., 2007, 2010): an upstream–downstream variation of the 238U-234U-230Th disequilibria in the sediments along the rivers within the Ganges plain, with different variation trends for suspended and coarse-grained sediments. Assuming that such trends result from the combination of both radioactive decay and U-Th fractionations associated to the sediment weathering during its transit and storage in the plain, the above data confirm the occurrence of very different sediment transfer times which strongly depend on their grain size: much shorter transfer time for the fine-grained sediment (a few ky or less) than for the coarse-grained sediment (100 ky or more).
The fine-grained sediment end-members collected at the Ganges outlet in 2004 and 2008, however, show significantly different 234U/230Th activity ratios. This difference is most probably an indication of a quick transit for the fine-grained sediment end-member (of the order of the year or less) within the plain. It is proposed that the variation of the U/Th ratio in this sediment end-member results from a significant difference in the intensity of weathering of these sediments during their transfer in the rivers from one year to another. Conversely, the U-Th variations observed in the bedload collected at the Ganges basin outlet over the period 2004-2008 cannot result from short sediment transfer time. They probably are the result of dredging; this kind of sampling might not allow for the collection of a representative sediment sample, especially with respect to minor minerals that carry U and Th. It results in an artificial U-Th variability in the different bedload sediments collected at different periods and certainly induces an uncertainty on the estimated sediment transfer time. This uncertainty, however, could be reduced when bank sediments can be studied instead of bedload sediments.
Acknowledgments
The financial support by the INSU/CNRS, the REALISE network and the University of Strasbourg over the 2001-2010 period has allowed the development of the study of 238U-series disequilibria in sediments and more generally on environmental samples in Strasbourg laboratory. All these French research organizations are warmly thanked for their support. The Simone and Cino Del Duca Foundation is also thanked for support to FC in 2005. Estelle Blaes acknowledges the Region Alsace and the CNRS (BDI), France, for the funding of her Ph.D. scholarship, and the University of Strasbourg for a temporary lecturer (ATER) position in 2010. A part of this study has been performed in the frame of the “Calimero” ANR project. M. Lupker and C. France-Lanord are thanked for the samples provided for this study. Discussions with P. Ackerer, C. France-Lanord and M. Lupker during the course of this study were very appreciated. We are also very grateful to F. Delay for his insightful comments on the manuscript. Review by M. Lupker helped to improve the manuscript. This is a EOST-LHyGeS contribution.
Appendix A Solving approach for model equations
The gain and loss model used to interpret the radioactive disequilibrium variations along the river stream is described by the ordinary differential equation system given in section 2 (Eqs. (1) to (4)), whose analytical solutions can be found in Chabaux et al. (2012). A stochastic bound constrained optimization algorithm based on a particle swarm technique has been constructed and used to perform the calculation of the leaching rate and input flux constants (k238, f238 coefficients) of each nuclide and the transfer time. The optimization algorithm uses the measured (234U/238U), (230Th/238U), (238U/232Th) and (230Th/232Th) activity ratios of each sample as constrains for the model.
A.1 Particle Swarm Optimization (PSO) algorithm
Classical techniques using population-based evolutionary systems such as particle Swarm Optimization (PSO, e.g., Kennedy and Eberhart, 1995) are intended to mimic the evolution of a social organism, in which individuals (also called “particles” or “bees”) describe the candidate solution to a designed problem. Each particle flies through a prescribed and bounded multidimensional space to find the optimal solution. Each individual evaluates its current position with reference to a goal (the objective function at each iteration). Particles are allowed to share memories of their best position in a prescribed local neighborhood. These positions are then used to update particle velocities and positions (see the following Eqs. (5) and (6)). Let M be the swarm size and D the size of the search domain (number of parameters). In the original PSO version, each M-particle moves inside the D-dimensional search space according to:
(5) |
(6) |
In QPSO approach, all particles move under quantum-mechanical rules rather than classical Newtonian random motion. In quantum mechanics, the governing equation is the well-known time-dependent Schrödinger equation
(7) |
The unknown in the previous Schrödinger equation is the wave function . In the QPSO approach, the wave function is a spatially distributed function measuring the probability of a particle motion and obeying to the norm . Clerc and Kennedy, 2002 showed that convergence of the algorithm might be achieved if each i-particle moves toward its local attractor. During the optimization procedure, all “bees” are flying towards the optimum location defined by vector p(t).
Let the ith-particle local attractor be a vector pi with coordinates in the D-dimensional parameter space with:
(8) |
Equation (8) requires only two random numbers and can be seen as a stochastic local attractor of the ith-particle. Here, c1 is called the cognitive parameter and c2 is called the social parameter. The random scalar φ is uniformly distributed between 0 to 1. In the QPSO approach, the original PSO system is considered as a quantum-like system, making that each particle has a quantum behavior with motions ruled by the wave probability density function (pdf). Different attractive potential field functions have been tested. A “reasonable” potential field selection is intended to attract particles towards the center of the potential field. However, such convergence speed is achieved while sacrificing an even search activity over the whole space of parameters.
For the sake of simplicity let us take particles moving in a one-dimensional parameter space and let us define r as r = x-p, where x is the current particle position and p is the average best position. We present here the simplest potential, the so-called delta-well function given by where γ is proportional to the depth of the well. In QPSO optimization, we are only interested in bounds states given by the stationary states i.e. the wave envelope function (Mikky and Kishk, 2005). Assuming the principle of separation for time and space variables in the wave function, the time-independent solution of the Schrödinger equation, i.e., the so-called wave envelope function is given by:
(9) |
It can be shown that following this potential field, we have an analytical expression for the probability density function (pdf) of the particle's motion. The probability density function Q is thus given by
(10) |
(11) |
Now, one needs to link the above quantum description provided by the wave function (Eqs. (10) and (11)) and a Newtonian description of the particle motion. The next step is therefore to measure the position of the particle i.e. “collapsing the quantum state to the classical state”. A classical Monte Carlo method is used to simulate the process of measurement. The procedure of simulation is described as follows. Let v be the random number uniformly distributed in the interval (0, 1). Substituting v for F(r) leads to and because u = 1–v is also a random number of uniform distribution between 0 and 1, it becomes obvious that .
For time dependent Ln (iterative procedure), i.e. and using , one can obtain the random sequence of the position as,
(12) |
For the D-dimensional search space, the particle position is updated at each iteration step by using:
(13) |
(14) |
Hence, one has
(15) |
With exceptions of the swarm size (Number of bees), the number of maximum iterations and the problem dimension D, the most important parameter is the contraction-expansion coefficient which is crucial to the dynamical behavior of each individual and the convergence speed. Results from several stochastic simulations reported in Sun et al. (2004) showed that the particle position either converges to its local optimum when α < 1.78 or diverges when α > 1.8. Tο prevent blowing the swarm apart, we set the contraction-expansion factor to eγ0 ≈ 1.781 where γ0 ≈ 0.577 is the Euler-Mascheroni constant. For practical consideration, the Euler-Maclaurin method is used to generate α. Values for the cognitive parameter c1 and the social parameters c2 are better to be chosen even at c1 = c2 = 2 (see Mikki and Kishk, 2005).
A.2 Optimization of the determination of time-scales of sedimentary transfer using QPSO
For weathering optimization problem solved by QPSO-based method, our objective function is the relative squared error RSE defined by:
(16) |
Choose a suitable potential well (Delta well, Harmonic well, Square well). Solve the Schrödinger equation to get the envelope wave function ψ (r) and then the pdf function of the particle position.
“Collapse” wave function into the bounded region (absolute/relative bounds) using Monte Carlo procedure.
Initialize the swarm of size MaxBee:
- fort = 1 to MaxIter until convergence
- Compute the fitness value of each particle with the objective function defined as RSE
- Update the particle best position Pi
- Update the global best position of the swarm Pg
- Compute the mean best position μ of the swarm
- for each particle in the swarm
- Update each component of the particle's position and adjust the component xi
- end for
- Rescale solutions within absolute and relative bounds
- Evaluate convergence
- end for