La connectivité fonctionnelle est une notion neurobiologique, qui affirme informellement qu’il y aurait une forte dépendance entre neurones et que cette dépendance pourrait être utilisée pour comprendre comment le cerveau encode les stimuli, programme les actions, etc. Cependant, en pratique, ces fortes dépendances sont souvent reconstruites, grâce aux processus de Hawkes, sur un nombre incroyablement faible de neurones, parce que l’observation du réseau sous-jacent est excessivement partielle. Nous prouvons de nouvelles équations qui expliquent comment la reconstruction idéale par processus de Hawkes est liée à la covariance entre neurones observés. Ces équations nous aident à comprendre ce que fait exactement la reconstruction par processus de Hawkes dans deux cadres asymptotiques, la synchronization et le cadre classique des processus ponctuels. De plus, elles pourraient nous permettre de comprendre qualitativement ce qui se passe dans l’immense réseau non observé, ouvrant la voie à une possible définition mathématique de la connectivité fonctionnelle.
Functional connectivity is a neurobiological notion, informally stating that there would be a strong dependence between neurons and that this dependence might be useful in understanding the way the brain encodes stimuli, programs actions, etc. However, in practice such strong dependencies are often reconstructed via Hawkes processes based on an amazingly small number of neurons, because of the very scarce observation of this very complex and huge network. We derive new simple equations, which explain how the ideal Hawkes reconstruction is linked to the covariance between the observed neurons. These equations help us in particular to understand what the Hawkes reconstruction does in two settings, synchronization and classical point process asymptotics. Moreover they might help us to also understand what is qualitatively happening at the scale of the huge unobserved network, paving the path for a possible mathematical definition of functional connectivity.
Accepté le :
Publié le :
DOI : 10.5802/crmath.190
Patricia Reynaud-Bouret 1 ; Alexandre Muzy 2 ; Ingrid Bethus 3
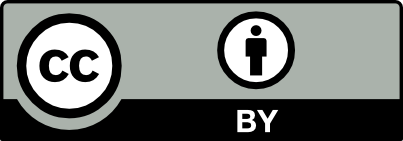
@article{CRMATH_2021__359_4_481_0, author = {Patricia Reynaud-Bouret and Alexandre Muzy and Ingrid Bethus}, title = {Towards a mathematical definition of functional connectivity}, journal = {Comptes Rendus. Math\'ematique}, pages = {481--492}, publisher = {Acad\'emie des sciences, Paris}, volume = {359}, number = {4}, year = {2021}, doi = {10.5802/crmath.190}, zbl = {07362168}, language = {en}, }
TY - JOUR AU - Patricia Reynaud-Bouret AU - Alexandre Muzy AU - Ingrid Bethus TI - Towards a mathematical definition of functional connectivity JO - Comptes Rendus. Mathématique PY - 2021 SP - 481 EP - 492 VL - 359 IS - 4 PB - Académie des sciences, Paris DO - 10.5802/crmath.190 LA - en ID - CRMATH_2021__359_4_481_0 ER -
Patricia Reynaud-Bouret; Alexandre Muzy; Ingrid Bethus. Towards a mathematical definition of functional connectivity. Comptes Rendus. Mathématique, Volume 359 (2021) no. 4, pp. 481-492. doi : 10.5802/crmath.190. https://comptes-rendus.academie-sciences.fr/mathematique/articles/10.5802/crmath.190/
[1] Second order statistics characterization of Hawkes processes and non-parametric estimation, IEEE Trans. Inf. Theory, Volume 62 (2016) no. 4, pp. 2184-2202 | DOI | MR | Zbl
[2] Long-lasting potentiation of synaptic transmission in the dentate area of the anaesthetized rabbit following stimulation of the perforant path, J. Physiol., Volume 232 (1973), pp. 331-356 | DOI
[3] Large-scale recording of neuronal ensembles, Nature Neurosci., Volume 7 (2004) no. 5, pp. 446-451 | DOI | Zbl
[4] Spike Timing–Dependent Plasticity: A Hebbian Learning Rule, Annu. Rev. Neurosci., Volume 31 (2008), pp. 25-46 | DOI
[5] Mean-field limit of generalized Hawkes processes, Stochastic Processes Appl., Volume 127 (2017) no. 12, pp. 3870-3912 | DOI | MR | Zbl
[6] Exact computation of the maximum-entropy potential of spiking neural-network models, Phys. Rev. E, Volume 89 (2014) no. 5, pp. 1262-1292 | Zbl
[7] Massively parallel recording of unit and local field potentials with silicon-based electrodes, J. Neurophys., Volume 90 (2003), pp. 1314-1323 | DOI
[8] Graphical models for marked point processes based on local independence, J. R. Stat. Soc., Ser. B, Stat. Methodol., Volume 70 (2008) no. 1, pp. 245-264 | DOI | MR | Zbl
[9] Estimating the interaction graph of stochastic neural dynamics, Bernoulli, Volume 25 (2019) no. 1, pp. 771-792 | MR | Zbl
[10] Functional connectivity, Brain Mapping, an encyclopedic reference, Volume 2, Academic Press Inc., 2015, pp. 187-201
[11] Neuronal Assemblies, IEEE Trans. Biomed. Eng., Volume 36 (1989), pp. 4-14 | DOI
[12] Unitary events in multiple single-neuron spiking activity: I. detection and significance, Neural Comput., Volume 14 (2002) no. 5, pp. 43-80 | DOI | Zbl
[13] Detecting unitary events without discretization in time, J. Neurosci. Methods, Volume 94 (1999)
[14] Lasso and probabilistic inequalities for multivariate point processes, Bernoulli, Volume 21 (2015) no. 1, pp. 83-143 | MR | Zbl
[15] Accuracy of Tetrode Spike Separation as Determined by Simultaneous Intracellular and Extracellular Measurements, J. Neurophys., Volume 84 (2000) no. 1, pp. 402-413
[16] The organization of behavior: a neuropsychological theory, John Wiley & Sons; Chapman & Hall, 1949
[17] Intracellular features predicted by extracellular recordings in the hippocampus in vivo, J. Neurophys., Volume 84 (2000), pp. 390-400 | DOI
[18] Tetrode technology: advances in implantable hardware, neuroimag-ing, and data analysis techniques, J. Neurosci. Methods, Volume 117 (2002), pp. 141-152 | DOI
[19] et al. Fully integrated silicon probes for high-density recording of neural activity, Nature, Volume 551 (2017), pp. 232-236
[20] Assessment of synchrony in multiple neural spike trains using loglinear point process models, Ann. Appl. Stat., Volume 5 (2011), pp. 1262-1292 | MR | Zbl
[21] An introduction to expander graphs, Contributions in Mathematical and Computational Sciences, Société Mathématique de France, 2019 | MR | Zbl
[22] Reconstructing the functional connectivity of multiple spike trains using Hawkes models, J. Neurosci. Methods, Volume 297 (2018), pp. 9-21 | DOI
[23] Emergence of event cascades in inhomogeneous networks, Nature Sci. Reports, Volume 6 (2016)
[24] Sparse space-time models: concentration inequalities and Lasso, Ann. Inst. Henri Poincaré, Probab. Stat., Volume 56 (2020) no. 4, pp. 2377-2405 | MR | Zbl
[25] How Structure Determines Correlations in Neuronal Networks, PLoS Comput. Biol., Volume 7 (2011) | DOI | MR
[26] Spatio-temporal correlations and visual signalling in a complete neuronal population, Nature, Volume 454 (2008), pp. 995-999 | DOI
[27] Rôle de la connectivité intracorticale dans le traitement des informations sensorielles, Ph. D. Thesis, Université Paris 6 (2017) (sous la direction de R. Lambert)
[28] Dynamical changes and temporal precision of synchronized spiking activity in monkey motor cortex during movement preparation, J. Physiol., Volume 94 (2000) no. 5-6, pp. 569-582
[29] Neuronal coding of prediction errors, Annu. Rev. Neurosci., Volume 23 (2000), pp. 473-500 | DOI
[30] The synaptic organization of the brain, Oxford University Press, 2004
[31] Synchronization of cortical activity and its putative role in information processing and learning, Annu. Rev. Physiol., Volume 55 (1993), pp. 349-374 | DOI
[32] Neuronal assemblies: necessity, signature and detectability, Trends Cogn. Sci., Volume 1 (1997), pp. 252-261 | DOI
Cité par Sources :
Commentaires - Politique