[Reconstruction de mesures discrètes à partir de projections. Conséquences sur la distance de Sliced Wasserstein empirique]
On s’intéresse dans cet article au problème de reconstruction d’une mesure discrète
This paper deals with the reconstruction of a discrete measure
Révisé le :
Accepté le :
Publié le :
DOI : 10.5802/crmath.601
Keywords: Reconstruction, Inverse Problems, Discrete Measures
Mots-clés : Reconstruction, problèmes inverses, mesures discrètes
Eloi Tanguy 1 ; Rémi Flamary 2 ; Julie Delon 1
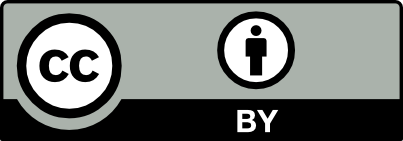
@article{CRMATH_2024__362_G10_1121_0, author = {Eloi Tanguy and R\'emi Flamary and Julie Delon}, title = {Reconstructing discrete measures from projections. {Consequences} on the empirical {Sliced} {Wasserstein} {Distance}}, journal = {Comptes Rendus. Math\'ematique}, pages = {1121--1129}, publisher = {Acad\'emie des sciences, Paris}, volume = {362}, year = {2024}, doi = {10.5802/crmath.601}, zbl = {07939447}, language = {en}, }
TY - JOUR AU - Eloi Tanguy AU - Rémi Flamary AU - Julie Delon TI - Reconstructing discrete measures from projections. Consequences on the empirical Sliced Wasserstein Distance JO - Comptes Rendus. Mathématique PY - 2024 SP - 1121 EP - 1129 VL - 362 PB - Académie des sciences, Paris DO - 10.5802/crmath.601 LA - en ID - CRMATH_2024__362_G10_1121_0 ER -
%0 Journal Article %A Eloi Tanguy %A Rémi Flamary %A Julie Delon %T Reconstructing discrete measures from projections. Consequences on the empirical Sliced Wasserstein Distance %J Comptes Rendus. Mathématique %D 2024 %P 1121-1129 %V 362 %I Académie des sciences, Paris %R 10.5802/crmath.601 %G en %F CRMATH_2024__362_G10_1121_0
Eloi Tanguy; Rémi Flamary; Julie Delon. Reconstructing discrete measures from projections. Consequences on the empirical Sliced Wasserstein Distance. Comptes Rendus. Mathématique, Volume 362 (2024), pp. 1121-1129. doi : 10.5802/crmath.601. https://comptes-rendus.academie-sciences.fr/mathematique/articles/10.5802/crmath.601/
[1] Sliced and Radon Wasserstein barycenters of measures, J. Math. Imaging Vis., Volume 51 (2015) no. 1, pp. 22-45 | DOI | MR | Zbl
[2] Random projections, marginals, and moments (https://djalil.chafai.net/docs/projections.pdf)
[3] Probability distributions with given multivariate marginals and given dependence structure, J. Multivariate Anal., Volume 42 (1992) no. 1, pp. 51-66 | DOI | MR | Zbl
[4] Some Theorems on Distribution Functions, J. Lond. Math. Soc., Volume 11 (1936) no. 4, pp. 290-294 | DOI | MR | Zbl
[5] Advances in probability distributions with given marginals. Beyond the copulas, Mathematics and its Applications, 67, Springer, 2012 | DOI
[6] Generative Modeling Using the Sliced Wasserstein Distance, 2018 IEEE Conference on Computer Vision and Pattern Recognition, CVPR 2018, Salt Lake City, UT, USA, June 18-22, 2018, Computer Vision Foundation / IEEE Computer Society (2018), pp. 3483-3491 | DOI
[7] Determining point distributions from their projections, 2017 International Conference on Sampling Theory and Applications (SampTA) (2017), pp. 164-168 | DOI
[8] Uniqueness and complexity in discrete tomography, Discrete tomography (Applied and Numerical Harmonic Analysis), Birkhäuser, 1999, pp. 85-113 | DOI | MR | Zbl
[9] On multistochastic Monge-Kantorovich problem, bitwise operations, and fractals, Calc. Var. Partial Differ. Equ., Volume 58 (2019) no. 5, 173, 33 pages | DOI | MR | Zbl
[10] On the determination of probability distributions of more dimensions by their projections, Acta Math. Acad. Sci. Hung., Volume 7 (1956), pp. 403-410 | DOI | MR | Zbl
[11] Parametric families of multivariate distributions with given margins, J. Multivariate Anal., Volume 46 (1993) no. 2, pp. 262-282 | DOI | MR | Zbl
[12] Progressive Growing of GANs for Improved Quality, Stability, and Variation, International Conference on Learning Representations (2018)
[13] Verteilungsfunktionen mit gegebenen Marginalverteilungen, Z. Wahrscheinlichkeitstheor. Verw. Geb., Volume 3 (1964), pp. 247-270 | DOI | MR | Zbl
[14] On a construction of multivariate distributions given some multidimensional marginals, Adv. Appl. Probab., Volume 51 (2019) no. 2, pp. 487-513 | DOI | MR | Zbl
[15] Sliced-Wasserstein distance for large-scale machine learning: theory, methodology and extensions, Ph. D. Thesis, Institut polytechnique de Paris (2021)
[16] Computational Optimal Transport: With Applications to Data Science, Found. Trends Mach. Learn., Volume 11 (2019) no. 5-6, pp. 355-607 | DOI | Zbl
[17] Wasserstein Barycenter and Its Application to Texture Mixing, Scale Space and Variational Methods in Computer Vision (Alfred M. Bruckstein; Bart M. ter Haar Romeny; Alexander M. Bronstein; Michael M. Bronstein, eds.), Springer, 2012, pp. 435-446 | DOI
[18] On projections of probability distributions, Acta Math. Acad. Sci. Hung., Volume 3 (1952), pp. 131-142 | DOI | MR | Zbl
[19] Convergence of sgd for training neural networks with sliced Wasserstein losses (2023) (https://arxiv.org/abs/2307.11714)
[20] Sensing theorems for unsupervised learning in linear inverse problems, J. Mach. Learn. Res., Volume 24 (2023), 39, 45 pages | DOI | MR | Zbl
[21] Properties of discrete sliced Wasserstein losses (2023)
[22] Sliced Wasserstein Generative Models, 2019 IEEE/CVF Conference on Computer Vision and Pattern Recognition (CVPR) (2019), pp. 3708-3717 | DOI
Cité par Sources :
Commentaires - Politique