[Bornes d’erreur pour la réduction de modèles sur des variétés construites par des applications polynomiales]
For projection-based linear-subspace model order reduction (MOR), it is well known that the Kolmogorov
Pour la réduction de l’ordre des modèles basée sur la projection d’un sous-espace linéaire, il est bien connu que la largeur de Kolmogorov n décrit la meilleure erreur possible pour un modèle d’ordre réduit de taille
Révisé le :
Accepté le :
Publié le :
DOI : 10.5802/crmath.632
Keywords: Model Order Reduction, nonlinear manifolds, polynomial mappings, polynomial
Mots-clés : Réduction de l’ordre des modèles, variétés non linéaires, transformations polynomiales,
Patrick Buchfink 1, 2 ; Silke Glas 2 ; Bernard Haasdonk 1
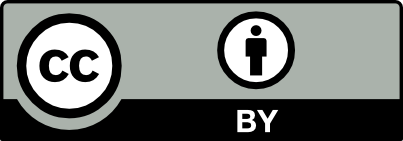
@article{CRMATH_2024__362_G13_1881_0, author = {Patrick Buchfink and Silke Glas and Bernard Haasdonk}, title = {Approximation {Bounds} for {Model} {Reduction} on {Polynomially} {Mapped} {Manifolds}}, journal = {Comptes Rendus. Math\'ematique}, pages = {1881--1891}, publisher = {Acad\'emie des sciences, Paris}, volume = {362}, year = {2024}, doi = {10.5802/crmath.632}, zbl = {07962937}, language = {en}, }
TY - JOUR AU - Patrick Buchfink AU - Silke Glas AU - Bernard Haasdonk TI - Approximation Bounds for Model Reduction on Polynomially Mapped Manifolds JO - Comptes Rendus. Mathématique PY - 2024 SP - 1881 EP - 1891 VL - 362 PB - Académie des sciences, Paris DO - 10.5802/crmath.632 LA - en ID - CRMATH_2024__362_G13_1881_0 ER -
%0 Journal Article %A Patrick Buchfink %A Silke Glas %A Bernard Haasdonk %T Approximation Bounds for Model Reduction on Polynomially Mapped Manifolds %J Comptes Rendus. Mathématique %D 2024 %P 1881-1891 %V 362 %I Académie des sciences, Paris %R 10.5802/crmath.632 %G en %F CRMATH_2024__362_G13_1881_0
Patrick Buchfink; Silke Glas; Bernard Haasdonk. Approximation Bounds for Model Reduction on Polynomially Mapped Manifolds. Comptes Rendus. Mathématique, Volume 362 (2024), pp. 1881-1891. doi : 10.5802/crmath.632. https://comptes-rendus.academie-sciences.fr/mathematique/articles/10.5802/crmath.632/
[1] Kolmogorov widths and low-rank approximations of parametric elliptic PDEs, Math. Comput., Volume 86 (2017) no. 304, pp. 701-724 | DOI | MR | Zbl
[2] Quadratic approximation manifold for mitigating the Kolmogorov barrier in nonlinear projection-based model order reduction, J. Comput. Phys., Volume 464 (2022), 111348, 20 pages | DOI | MR | Zbl
[3] Mitigating the Kolmogorov Barrier for the Reduction of Aerodynamic Models using Neural-Network-Augmented Reduced-Order Models, AIAA SCITECH 2023 Forum (2023) (article no. 0535) | DOI
[4] A quadratic decoder approach to nonintrusive reduced-order modeling of nonlinear dynamical systems, PAMM, Volume 23 no. 1, e202200049 | DOI
[5] Convex optimization, Cambridge University Press, 2004, xiv+716 pages | DOI | MR | Zbl
[6] Analytic regularity and polynomial approximation of parametric and stochastic elliptic PDE’s, Anal. Appl., Singap., Volume 9 (2011) no. 1, pp. 11-47 | DOI | MR | Zbl
[7] Nonlinear compressive reduced basis approximation for PDE’s, C. R. Méc. Acad. Sci. Paris, Volume 351 (2023) no. S1, pp. 357-374 | DOI
[8] Model Reduction of Nonlinear Dynamical Systems by System-Theoretic Methods, Dissertation, Technische Universität München (2020)
[9] Optimal nonlinear approximation, Manuscr. Math., Volume 63 (1989) no. 4, pp. 469-478 | DOI | MR | Zbl
[10] POD-DL-ROM: enhancing deep learning-based reduced order models for nonlinear parametrized PDEs by proper orthogonal decomposition, Comput. Meth. Appl. Mech. Eng., Volume 388 (2022), 114181, 27 pages | DOI | MR | Zbl
[11] Learning Latent Representations in High-Dimensional State Spaces Using Polynomial Manifold Constructions, 2023 62nd IEEE Conference on Decision and Control (CDC), IEEE (2023), pp. 4960-4965 | DOI
[12] Model order reduction of nonlinear dynamical systems, Dissertation, University of California (2011)
[13] Decay of the Kolmogorov N-width for wave problems, Appl. Math. Lett., Volume 96 (2019), pp. 216-222 | DOI | MR | Zbl
[14] Operator inference for non-intrusive model reduction with quadratic manifolds, Comput. Meth. Appl. Mech. Eng., Volume 403 (2023), 115717, 24 pages | DOI | MR | Zbl
[15] Reduced basis methods for parametrized PDEs – a tutorial introduction for stationary and instationary problems, Model reduction and approximation (Comput. Sci. Eng.), Volume 15, Society for Industrial and Applied Mathematics, 2017, pp. 65-136 | DOI | MR
[16] Predicting solar wind streams from the inner-heliosphere to Earth via shifted operator inference, J. Comput. Phys., Volume 473 (2023), 111689, 25 pages | DOI | MR | Zbl
[17] A quadratic manifold for model order reduction of nonlinear structural dynamics, Comput. & Structures, Volume 188 (2017), pp. 80-94 | DOI
[18] Über die beste Annäherung von Funktionen einer gegebenen Funktionenklasse, Ann. Math., Volume 37 (1936) no. 1, pp. 107-110 | DOI | Zbl
[19] A priori convergence theory for reduced-basis approximations of single-parameter elliptic partial differential equations, J. Sci. Comput., Volume 17 (2002) no. 1-4, pp. 437-446 | DOI | MR | Zbl
[20] Global a priori convergence theory for reduced-basis approximations of single-parameter symmetric coercive elliptic partial differential equations, C. R. Math. Acad. Sci. Paris, Volume 335 (2002) no. 3, pp. 289-294 | DOI | Numdam | MR | Zbl
[21] Reduced Basis Methods: Success, Limitations and future Challenges, Proc. ALGORITMY (2016), pp. 1-12
[22] n-Widths in Approximation Theory, Ergebnisse der Mathematik und ihrer Grenzgebiete, 7, Springer, 1985 | DOI | MR | Zbl
[23] Generalization of quadratic manifolds for reduced order modeling of nonlinear structural dynamics, Comput. & Structures, Volume 192 (2017), pp. 196-209 | DOI
[24] Symplectic model reduction of Hamiltonian systems using data-driven quadratic manifolds, Comput. Methods Appl. Mech. Eng., Volume 417 (2023) no. part A, 116402, 28 pages | DOI | MR | Zbl
Cité par Sources :
Commentaires - Politique