We give convergence and cost estimates for a data-driven system identification method: given an unknown dynamical system, the aim is to recover its vector field and its flow from trajectory data. It is based on the so-called Koopman operator, which uses the well-known link between differential equations and linear transport equations. Data-driven methods recover specific finite-dimensional approximations of the Koopman operator, which can be understood as a transport operator. We focus on such approximations given by classical finite element spaces, which allow us to give estimates on the approximation of the Koopman operator as well as the solutions of the associated linear transport equation. These approximations are thus relevant objects to solve the system identification problem.
We then analyze the convergence of a variant of the generator Extended Dynamic Mode Decomposition (gEDMD) algorithm, one of the main algorithms developed to compute approximations of the Koopman operator from data. We find however that, when combining this algorithm with classical finite element spaces, the results are not satisfactory numerically, as the convergence of the data-driven approximation is too slow for the method to benefit from the accuracy of finite element spaces. In particular, for problems in dimension 1 it is less efficient than direct interpolation methods to recover the vector field. We provide some numerical examples to illustrate this last point.
Accepté le :
Première publication :
Publié le :
Christophe Zhang 1 ; Enrique Zuazua 1, 2, 3
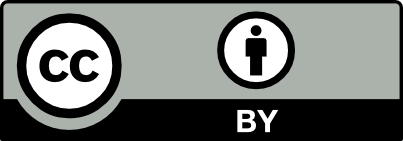
@article{CRMECA_2023__351_S1_721_0, author = {Christophe Zhang and Enrique Zuazua}, title = {A quantitative analysis of {Koopman} operator methods for system identification and predictions}, journal = {Comptes Rendus. M\'ecanique}, pages = {721--751}, publisher = {Acad\'emie des sciences, Paris}, volume = {351}, number = {S1}, year = {2023}, doi = {10.5802/crmeca.138}, language = {en}, }
TY - JOUR AU - Christophe Zhang AU - Enrique Zuazua TI - A quantitative analysis of Koopman operator methods for system identification and predictions JO - Comptes Rendus. Mécanique PY - 2023 SP - 721 EP - 751 VL - 351 IS - S1 PB - Académie des sciences, Paris DO - 10.5802/crmeca.138 LA - en ID - CRMECA_2023__351_S1_721_0 ER -
%0 Journal Article %A Christophe Zhang %A Enrique Zuazua %T A quantitative analysis of Koopman operator methods for system identification and predictions %J Comptes Rendus. Mécanique %D 2023 %P 721-751 %V 351 %N S1 %I Académie des sciences, Paris %R 10.5802/crmeca.138 %G en %F CRMECA_2023__351_S1_721_0
Christophe Zhang; Enrique Zuazua. A quantitative analysis of Koopman operator methods for system identification and predictions. Comptes Rendus. Mécanique, Volume 351 (2023) no. S1, pp. 721-751. doi : 10.5802/crmeca.138. https://comptes-rendus.academie-sciences.fr/mecanique/articles/10.5802/crmeca.138/
[1] System Identification: Theory for the User, PTR Prentice Hall, Upper Saddle River, NJ, 1999, pp. 1-14
[2] Identification of linear parameter varying models, Int. J. Robust Nonlinear Control, Volume 12 (2002) no. 9, pp. 841-853 | DOI | MR | Zbl
[3] Nonlinear System Identification: From Classical Approaches to Neural Networks and Fuzzy Models, Springer Science & Business Media, 2013
[4] On an inverse boundary value problem, Comput. Appl. Math., Volume 25 (2006) no. 2–3, pp. 133-138 | MR | Zbl
[5] Inverse Problems for Partial Differential Equations, 127, Springer, 2006
[6] Carleman estimates and an inverse heat source problem for the thermoelasticity system, Inverse Probl., Volume 27 (2010) no. 1, 015006 | DOI | MR
[7] Nouvelles méthodes pour la détermination des orbites des comètes, F. Didot, 1805
[8] Universal algorithms for learning theory part I: Piecewise constant functions, J. Mach. Learn. Res., Volume 6 (2005), pp. 1297-1321 | Zbl
[9] Universal algorithms for learning theory. Part II: Piecewise polynomial functions, Constr. Approx., Volume 26 (2007) no. 2, pp. 127-152 | DOI | Zbl
[10] Deep Learning, MIT Press, 2016 http://www.deeplearningbook.org
[11] Numerical Approximation of Partial Differential Equations, Springer Series in Computational Mathematics, 23, Springer-Verlag, Berlin, 1994 | DOI
[12] Discovering governing equations from data by sparse identification of nonlinear dynamical systems, Proc. Natl. Acad. Sci. USA, Volume 113 (2016) no. 15, pp. 3932-3937 | DOI | MR | Zbl
[13] Data-driven discovery of coordinates and governing equations, Proc. Natl. Acad. Sci. USA, Volume 116 (2019) no. 45, pp. 22445-22451 | DOI | MR | Zbl
[14] Weak SINDy: Galerkin-based data-driven model selection, Multiscale Model. Simul., Volume 19 (2021) no. 3, pp. 1474-1497 | DOI | MR | Zbl
[15] Ordinary differential equations, transport theory and Sobolev spaces, Invent. Math., Volume 98 (1989) no. 3, pp. 511-547 | DOI | MR | Zbl
[16] Deux remarques sur les flots généralisés d’équations différentielles ordinaires, C. R. Math., Volume 344 (2007) no. 12, pp. 759-764 | DOI | Zbl
[17] Hamiltonian systems and transformation in Hilbert space, Proc. Natl. Acad. Sci. USA, Volume 17 (1931) no. 5, pp. 315-318 | DOI | Zbl
[18] Proof of the quasi-ergodic hypothesis, Proc. Natl. Acad. Sci. USA, Volume 18 (1932) no. 1, pp. 70-82 | DOI | Zbl
[19] Dynamical systems of continuous spectra, Proc. Natl. Acad. Sci. USA, Volume 18 (1932) no. 3, pp. 255-263 | DOI | Zbl
[20] Spectral properties of dynamical systems, model reduction and decompositions, Nonlinear Dyn., Volume 41 (2005) no. 1, pp. 309-325 | DOI | MR | Zbl
[21] Analysis of fluid flows via spectral properties of the Koopman operator, Annu. Rev. Fluid Mech., Volume 45 (2013) no. 1, pp. 357-378 | DOI | MR | Zbl
[22] Linearization in the large of nonlinear systems and Koopman operator spectrum, Physica D, Volume 242 (2013) no. 1, pp. 42-53 | DOI | MR | Zbl
[23] Global stability analysis using the eigenfunctions of the Koopman operator, IEEE Trans. Automat. Contr., Volume 61 (2016) no. 11, pp. 3356-3369 | DOI | MR | Zbl
[24] Spectrum of the Koopman operator, spectral expansions in functional spaces, and state-space geometry, J. Nonlinear Sci., Volume 30 (2019), pp. 2091-2145 | DOI | MR
[25] Existence and uniqueness of global Koopman eigenfunctions for stable fixed points and periodic orbits, Physica D, Volume 425 (2021), 132959 | DOI | MR | Zbl
[26] Koopman invariant subspaces and finite linear representations of nonlinear dynamical systems for control, PLoS One, Volume 11 (2016) no. 2, pp. 1-19 | DOI
[27] Linear predictors for nonlinear dynamical systems: Koopman operator meets model predictive control, Automatica, Volume 93 (2018), pp. 149-160 | DOI | MR | Zbl
[28] Data-driven approximation of the Koopman generator: Model reduction, system identification, and control, Physica D, Volume 406 (2020), 132416 | DOI | MR | Zbl
[29] Application de la théorie des équations intégrales linéaires aux systèmes d’équations différentielles non linéaires, Acta Math., Volume 59 (1932), pp. 63-87 | DOI | MR | Zbl
[30] Learning Koopman invariant subspaces for dynamic mode decomposition, Advances in Neural Information Processing Systems 30 (I. Guyon; U. V. Luxburg; S. Bengio; H. Wallach; R. Fergus; S. Vishwanathan; R. Garnett, eds.), Curran Associates, Inc., 2017, pp. 1130-1140
[31] Dynamic mode decomposition for analytic maps, Commun. Nonlinear Sci. Numer. Simul., Volume 84 (2020), 105179 | DOI | MR | Zbl
[32] Data-driven discovery of Koopman eigenfunctions for control, Mach. Learn.: Sci. Technol., Volume 2 (2021) no. 3, 035023
[33] Optimal construction of Koopman eigenfunctions for prediction and control, IEEE Trans. Automat. Contr., Volume 65 (2020) no. 12, pp. 5114-5129 | DOI | MR | Zbl
[34] On the approximation of Koopman spectra for measure preserving transformations, SIAM J. Appl. Dyn. Syst., Volume 18 (2019) no. 3, pp. 1454-1497 | DOI | MR | Zbl
[35] Data-driven spectral analysis of the Koopman operator, Appl. Comput. Harmon. Anal., Volume 48 (2020) no. 2, pp. 599-629 | DOI | MR | Zbl
[36] Rigorous data-driven computation of spectral properties of Koopman operators for dynamical systems, 2021 (preprint) | arXiv
[37] A data-driven approximation of the Koopman operator: Extending dynamic mode decomposition, J. Nonlinear Sci., Volume 25 (2015) no. 6, pp. 1307-1346 | DOI | MR | Zbl
[38] On convergence of extended dynamic mode decomposition to the Koopman operator, J. Nonlinear Sci., Volume 28 (2018) no. 2, pp. 687-710 | DOI | MR | Zbl
[39] Deep learning for universal linear embeddings of nonlinear dynamics, Nat. Commun., Volume 9 (2018) no. 1, pp. 1-10 | DOI
[40] Kernel-based approximation of the Koopman generator and Schrödinger operator, Entropy, Volume 22 (2020) no. 7, 722 | DOI
[41] Coarse-grained protein models and their applications, Chem. Rev., Volume 116 (2016) no. 14, pp. 7898-7936 | DOI
[42] A data-driven Koopman model predictive control framework for nonlinear partial differential equations, 2018 IEEE Conference on Decision and Control (CDC) (2018), pp. 6409-6414 | DOI
[43] Deep Koopman model predictive control for enhancing transient stability in power grids, Int. J. Robust Nonlinear Control, Volume 31 (2021) no. 6, pp. 1964-1978 | DOI
[44] Power grid transient stabilization using Koopman model predictive control, IFAC-PapersOnLine, Volume 51 (2018) no. 28, pp. 297-302 (10th IFAC Symposium on Control of Power and Energy Systems CPES 2018) | DOI
[45] Linearly recurrent autoencoder networks for learning dynamics, SIAM J. Appl. Dyn. Syst., Volume 18 (2019) no. 1, pp. 558-593 | DOI | MR | Zbl
[46] Learning deep neural network representations for Koopman operators of nonlinear dynamical systems, 2019 American Control Conference (ACC) (2019), pp. 4832-4839 | DOI
[47] Koopman-based lifting techniques for nonlinear systems identification, IEEE Trans. Automat. Contr., Volume 65 (2020) no. 6, pp. 2550-2565 | DOI | MR | Zbl
[48] Dynamic Programming, Princeton University Press, Princeton, NJ, USA, 1957
[49] Nonlinear approximation, Acta Numer., Volume 7 (1998), pp. 51-150 | DOI | Zbl
[50] Breaking the curse for uniform approximation in Hilbert spaces via Monte Carlo methods, J. Complex., Volume 48 (2018), pp. 15-35 | DOI | MR | Zbl
[51] The curse of dimension and a universal method for numerical integration, Multivariate Approximation and Splines, Springer, 1997, pp. 177-187 | DOI | Zbl
[52] Sparse grids, Acta Numer., Volume 13 (2004), pp. 147-269 | DOI | MR | Zbl
[53] Breaking the curse of dimensionality in sparse polynomial approximation of parametric PDEs, J. Math. Pures Appl., Volume 103 (2015) no. 2, pp. 400-428 | DOI | MR | Zbl
[54] Numerical differentiation of noisy, nonsmooth, multidimensional data, 2017 IEEE Global Conference on Signal and Information Processing (GlobalSIP), IEEE (2017), pp. 244-248 | DOI
[55] Extending data-driven Koopman analysis to actuated systems, IFAC-PapersOnLine, Volume 49 (2016) no. 18, pp. 704-709 | DOI
[56] On the numerical approximation of the Perron–Frobenius and Koopman operator, J. Comput. Dyn., Volume 3 (2016) no. 1, pp. 51-79 | DOI | MR | Zbl
[57] Introduction to Data Mining, Addison-Wesley Longman Publishing Co., Inc., USA, 2005
[58] Monte Carlo and quasi-Monte Carlo methods, Acta Numer., Volume 7 (1998), pp. 1-49 | DOI | MR | Zbl
[59] A generalized inverse for matrices, Math. Proc. Cambridge Philos. Soc., Volume 51 (1955) no. 3, pp. 406-413 | DOI | Zbl
[60] Equivalence constants for matrix norms: a problem of Goldberg, Linear Algebra Appl., Volume 306 (2000) no. 1, pp. 1-13 | DOI | MR | Zbl
[61] Data-driven spectral decomposition and forecasting of ergodic dynamical systems, Appl. Comput. Harmon. Anal., Volume 47 (2019) no. 2, pp. 338-396 | DOI | MR | Zbl
[62] Ergodic theory, dynamic mode decomposition, and computation of spectral properties of the Koopman operator, SIAM J. Appl. Dyn. Syst., Volume 16 (2017) no. 4, pp. 2096-2126 | DOI | MR | Zbl
[63] The rate of convergence in ergodic theorems, Russ. Math. Surv., Volume 51 (1996) no. 4, pp. 653-703 | DOI | MR | Zbl
[64] Optimal error bounds for cubic spline interpolation, J. Approx. Theory, Volume 16 (1976) no. 2, pp. 105-122 | DOI | MR
[65] Spacings (with discussion), J. Roy. Statist. Soc. Ser. B, Volume 27 (1965), pp. 395-449 https://www.jstor.org/stable/2345793 | MR | Zbl
[66] Computational complexity of spline interpolation, Int. J. Syst. Sci., Volume 18 (1987) no. 5, pp. 945-954 | DOI | MR | Zbl
[67] Generative stochastic modeling of strongly nonlinear flows with non-Gaussian statistics, 2020 (preprint) | arXiv
[68] A deep learning framework based on Koopman operator for data-driven modeling of vehicle dynamics, 2020 (preprint) | arXiv
[69] Numerical Analysis and Optimization: An Introduction to Mathematical Modelling and Numerical Simulation, Oxford University Press, 2007
Cité par Sources :
Commentaires - Politique