It is necessary to make an accurate assessment of uniaxial compressive strength (UCS) for rock mass classification and rock engineering design. However, there are many shortcomings in the conventional tests for UCS of rocks. The aim of this study is to present a hybrid model by integrating the genetic algorithm (GA) into the least-squares support vector machine (LSSVM) to predict the UCS of rock materials. The GA technique was utilized to improve the forecasting accuracy of the proposed LSSVM. To develop the proposed hybrid GA–LSSVM model, four main factors including the block punch index, point load strength, Schmidt rebound hardness and ultrasonic P-wave velocity were considered as input variables, while the UCS of rock materials was the output. A comparison was conducted among the proposed GA–LSSVM, the adaptive neuro-fuzzy inference system, the fuzzy inference system, the artificial neural network and the statistical method in accordance with three statistical indexes. The results of the comparisons show that the developed GA–LSSVM model has great potential to accurately estimate the UCS of rock materials.
Révisé le :
Accepté le :
Publié le :
Xinhua Xue 1 ; Yufeng Wei 2
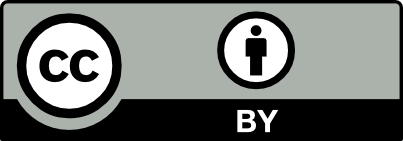
@article{CRMECA_2020__348_3_235_0, author = {Xinhua Xue and Yufeng Wei}, title = {A hybrid modelling approach for prediction of {UCS} of rock materials}, journal = {Comptes Rendus. M\'ecanique}, pages = {235--243}, publisher = {Acad\'emie des sciences, Paris}, volume = {348}, number = {3}, year = {2020}, doi = {10.5802/crmeca.17}, language = {en}, }
Xinhua Xue; Yufeng Wei. A hybrid modelling approach for prediction of UCS of rock materials. Comptes Rendus. Mécanique, Volume 348 (2020) no. 3, pp. 235-243. doi : 10.5802/crmeca.17. https://comptes-rendus.academie-sciences.fr/mecanique/articles/10.5802/crmeca.17/
[1] Predicting uniaxial compressive strength by point load test: significance of cone penetration, Rock Mech. Rock Eng., Volume 39 (2006) no. 5, pp. 483-490 | DOI
[2] A new testing method for indirect determination of the unconfined compressive strength of rocks, Int. J. Rock Mech. Min. Sci., Volume 46 (2009), pp. 1349-1357 | DOI
[3] Brazilizan tensile strength tests on some anisotropic rocks, Int. J. Rock Mech. Min. Sci., Volume 58 (2013), pp. 1-7 | DOI
[4] Soft computing methods for estimating the uniaxial compressive strength of intact rock from index tests, Int. J. Rock Mech. Min. Sci., Volume 80 (2015), pp. 418-424 | DOI
[5] Assessing the uniaxial compressive strength of extremely hard cryptocrystalline flint, Int. J. Rock Mech. Min. Sci., Volume 113 (2019), pp. 310-321 | DOI
[6] Determination of instantaneous breaking rate by geological strength index, block punch index and power of impact hammer for various rock mass conditions, Tunn. Undergr. Space Tech., Volume 26 (2011), pp. 534-540 | DOI
[7] An example for estimation of rock mass deformations around an underground opening by using numerical modeling, Int. J. Rock Mech. Min. Sci., Volume 47 (2010), pp. 272-278 | DOI
[8] Prediction of strength properties of some schistose rocks from petrographic properties using artificial neural networks, Int. J. Rock Mech. Min. Sci., Volume 38 (2001), pp. 269-284 | DOI
[9] An example of artificial neural network (ANN) application for indirect estimation of rock parameters, Rock Mech. Rock Eng., Volume 41 (2007) no. 5, pp. 781-795 | DOI
[10] Prediction of compressive and tensile strength of Gaziantep basalts via neural networks and gene expression programming, Neural Comput. Appl., Volume 18 (2009), pp. 1031-1041 | DOI
[11] Modelling of the uniaxial compressive strength of some clay-bearing rocks using neural network, Appl. Soft Comput., Volume 11 (2011), pp. 2587-2594 | DOI
[12] Artificial neural networks and nonlinear regression techniques to assess the influence of slake durability cycles on the prediction of uniaxial compressive strength and modulus of elasticity for carbonate rocks, Int. J. Numer Anal. Methods Geomech., Volume 36 (2012), pp. 1636-1650 | DOI
[13] Estimation of uniaxial compression strength of rock materials by index tests using regression analysis and fuzzy inference system, Eng. Geol., Volume 160 (2013), pp. 54-68 | DOI
[14] An application of adaptive neuro fuzzy inference system for estimating the uniaxial compressive strength of certain granitic rocks from their mineral contents, Expert Syst. Appl., Volume 40 (2013), pp. 921-928 | DOI
[15] Prediction of uniaxial compressive strength of granitic rocks by various nonlinear tools and comparison of their performances, Int. J. Rock Mech. Min. Sci., Volume 62 (2013), pp. 113-122 | DOI
[16] Comparative evaluation of artificial intelligence models for prediction of uniaxial compressive strength of travertine rocks, Case study: Azarshahr area, NW Iran, Model. Earth Syst. Environ., Volume 2 (2016), 76 | DOI
[17] Comparison of models for estimating uniaxial compressive strength of some sedimentary rocks from Qom Formation, Environ. Earth Sci., Volume 76 (2017), 753 | DOI
[18] Application of fuzzy inference system to predict uniaxial compressive strength and elastic modulus of migmatites, Environ. Earth Sci., Volume 78 (2019), 208 | DOI
[19] Comparasion of three updating schemes using artificial neural network in flow forecasting, Hydrol. Earth Syst. Sci., Volume 8 (2004), pp. 247-255 | DOI
[20] Application of artificial neural networks in groundwater table forecasting-a case study in a Singapore swamp forest, Hydrol. Earth Syst. Sci., Volume 20 (2016), pp. 1405-1412 | DOI
[21] Evaluation of soil-concrete interface shear strength based on LS-SVM, Geomech. Eng., Volume 11 (2016) no. 3, pp. 361-372 | DOI
[22] Optimal control by least squares support vector machines, Neural Networks, Volume 14 (2001), pp. 23-35 | DOI
[23] Functions of positive and negative type and their connection with the theory of integral equations, Phil. Trans. R. Soc., Volume 209 (1909), pp. 415-446 | Zbl
[24] Model induction with support vector machines: introduction and applications, J. Comput. Civ. Eng., Volume 15 (2001), pp. 208-216 | DOI
Cité par Sources :
Commentaires - Politique