Uniaxial compressive strength (UCS) is an important mechanical parameter for stability assessments in rock mass engineering. In practice, obtaining the UCS simply, accurately and economically has attracted substantial attention. In this paper, studies related to UCS estimation using indirect tests were reviewed, it was found that regression techniques and soft computing techniques were mainly used to evaluate the UCS value, and theses soft computing techniques can accurately and effectively predict the UCS. To select the proper indirect parameters to predict the UCS, statistical analysis was performed on the relationships between UCS and indirect parameters, and based on the analysis, two indirect parameters (the Schmidt hammer rebound value (L-type) and ultrasonic P-wave velocity) were deemed adequate to predict UCS. To establish the UCS predictive model, the random forest algorithm was employed, the predictive model was verified by data collected from references. To further verify the validity of the predictive model, laboratory tests were performed, and the predictive results were consistent with the measured results, thus the UCS value predictive model can be applied to the fields of rock mechanics and engineering geology.
Révisé le :
Accepté le :
Publié le :
Min Wang 1 ; Wen Wan 2 ; Yanlin Zhao 3
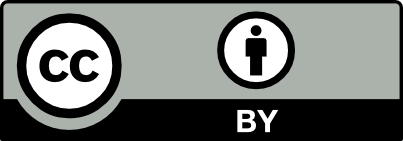
@article{CRMECA_2020__348_1_3_0, author = {Min Wang and Wen Wan and Yanlin Zhao}, title = {Prediction of the uniaxial compressive strength of rocks from simple index tests using a random forest predictive model}, journal = {Comptes Rendus. M\'ecanique}, pages = {3--32}, publisher = {Acad\'emie des sciences, Paris}, volume = {348}, number = {1}, year = {2020}, doi = {10.5802/crmeca.3}, language = {en}, }
TY - JOUR AU - Min Wang AU - Wen Wan AU - Yanlin Zhao TI - Prediction of the uniaxial compressive strength of rocks from simple index tests using a random forest predictive model JO - Comptes Rendus. Mécanique PY - 2020 SP - 3 EP - 32 VL - 348 IS - 1 PB - Académie des sciences, Paris DO - 10.5802/crmeca.3 LA - en ID - CRMECA_2020__348_1_3_0 ER -
%0 Journal Article %A Min Wang %A Wen Wan %A Yanlin Zhao %T Prediction of the uniaxial compressive strength of rocks from simple index tests using a random forest predictive model %J Comptes Rendus. Mécanique %D 2020 %P 3-32 %V 348 %N 1 %I Académie des sciences, Paris %R 10.5802/crmeca.3 %G en %F CRMECA_2020__348_1_3_0
Min Wang; Wen Wan; Yanlin Zhao. Prediction of the uniaxial compressive strength of rocks from simple index tests using a random forest predictive model. Comptes Rendus. Mécanique, Volume 348 (2020) no. 1, pp. 3-32. doi : 10.5802/crmeca.3. https://comptes-rendus.academie-sciences.fr/mecanique/articles/10.5802/crmeca.3/
[1] Drillability prediction: geological influences in hard rock drill and blast tunneling, Geol. Rundsch., Volume 86 (1997), pp. 426-438 | DOI
[2] Standard test method for unconfined compressive strength of intact rock core specimens, Soil and Rock, Building Stones: Annual Book of ASTM Standards 4.08, ASTM, Philadelphia, Pennsylvania, 1984
[3] Rock Characterisation Testing and Monitoring (E. T. Brown, ed.), Pergamon Press, Oxford, 1981
[4] The complete ISRM suggested methods for rock characterization, testing and monitoring: 1974–2006, Suggested Methods Prepared by the Commission on Testing Methods, International Society for Rock Mechanics (R. Ulusay; J. A. Hudson, eds.), ISRM Turkish National Group, Ankara, Turkey, 2007
[5] A fuzzy model to predict the uniaxial compressive strength and the modulus of elasticity of a problematic rock, Eng. Appl. Artif. Intell., Volume 17 (2004), pp. 61-72 | DOI
[6] Estimation of uniaxial compressive strength based on P-wave and Schmidt hammer rebound using statistical method, Arab. J. Geosci., Volume 6 (2013), pp. 1925-1931 | DOI
[7] The determination of uniaxial compressive strength from point load strength for pyroclastic rocks, Eng. Geol., Volume 170 (2014), pp. 33-42 | DOI
[8] Estimation of elastic constant of rocks using an ANFIS approach, Appl. Soft Comput., Volume 12 (2012) no. 1, pp. 40-45 | DOI
[9] A neuro-genetic network for predicting uniaxial compressive strength of rocks, Geotech. Geol. Eng., Volume 30 (2012) no. 4, pp. 1053-1062 | DOI
[10] Predicting the uniaxial compressive strength and elastic modulus of a fault breccia from texture coefficient, Rock Mech. Rock Eng., Volume 42 (2009), pp. 117-127 | DOI
[11] Models to predict the uniaxial compressive strength and the modulus of elasticity for Ankara agglomerate, Int. J. Rock Mech. Min. Sci., Volume 41 (2004) no. 5, pp. 717-729 | DOI
[12] Estimating the uniaxial compressive strength of a volcanic bimrock, Int. J. Rock Mech. Min. Sci., Volume 43 (2006) no. 4, pp. 554-561 | DOI
[13] Application of neural networks for the prediction of the unconfined compressive strength (UCS) from Equotip hardness, Int. J. Rock Mech. Min. Sci., Volume 36 (1999) no. 1, pp. 29-39 | DOI
[14] Prediction of compression strength from other rock properties, Q. Colo. Sch. Mines, Volume 59 (1964) no. 4b, pp. 623-640
[15]
(“Engineering classification and index properties for intact rock”, Air Force Weapons Lab. Tech. Report, AFWL-TR 65-116., Kirtland Base, New Mexico, 1966)[16] Point-load strength test, Int. J. Rock Mech. Min. Sci., Volume 9 (1972) no. 6, pp. 669-697 | DOI
[17] Point load test in geotechnical practice, Eng. Geol., Volume 9 (1975) no. 1, pp. 1-11 | DOI
[18] Application of point-load index test to strength determination of rock and proposals for new size-correction chart, Proc. 21st US Symp. Rock Mech., Rolla, Missouri (D. A. Summers, ed.), 1980, pp. 543-553
[19] A rational approach to the point load test, Proc. 3rd Australian-New Zealand Geomechanics Conference, Volume 2, 1980, pp. 35-39
[20] Determination of some engineering properties of weak rocks, Proc. Int. Symp. Weak Rock, Tokyo, 1981, pp. 21-24
[21] The influence of core sample geometry on the axial point-load test, Int. J. Rock Mech. Min. Sci. Geomech. Abstr., Volume 20 (1983) no. 6, pp. 291-295 | DOI
[22] Rock compressive strength, Colliery Eng., Volume 41 (1964), pp. 287-292
[23] A systematic determination of engineering criteria for rocks, Bull. Assoc. Eng. Geol., Volume 11 (1973), pp. 235-245
[24] Assessment of the degree of weathering in granite using petrographic and physical index tests, Proc. Int. Symp. On Deterioration and Protection of Stone Monuments. Unesco, Paris, 1978, pp. 1-35
[25] Correlations of Rock Index Values with Engineering Properties and the Classification of Intact Rock, FHWA, Washington, DC, 1979
[26] Bursting liability indices of coal, Int. J. Rock Mech. Min. Sci. Geomech. Abstr., Volume 17 (1980), pp. 157- 161
[27] The application of strength and deformation index testing to the stability assessment of coal measure excavations, Proc. 24th US Symp. Rock Mech., Texas A&M University, Texas, 1983, pp. 599-609
[28] Schmidt hammer rebound data for estimation of large-scale in situ coal strength, Int. J. Rock Mech. Min. Sci., Volume 21 (1984), pp. 39-42 | DOI
[29] Suggested method for determining point load strength, Int. J. Rock Mech. Min. Sci. Geomech. Abstr., Volume 22 (1985) no. 2, pp. 53-60
[30] Use of the Schmidt hammer for rock and coal testing, 26th US Symp. on Rock Mech., Rapid City, 1985, pp. 549-555
[31] Empirical strength indices of Indian coals-an investigation, Proc. 27th US Symp. Rock Mech., Balkema, Rotterdam, 1986, pp. 59-61
[32] Correlation between unconfined compressive and point load strength for Appalachian rocks, Proc. 30th US Symp. Rock Mech., Morgantown, 1989, pp. 461-468
[33] Rock index properties for geo-engineering in underground development, Min. Eng., Volume 41 (1989) no. 2, pp. 106-110
[34] Evaluation of empirical methods for measuring the uniaxial compressive strength of rock, Int. J. Rock Mech. Min. Sci., Volume 27 (1990) no. 6, pp. 495-503 | DOI
[35] Correlating Schmidt hardness with compressive strength and young’s modulus of carbonate rocks, Bull. Int. Assoc. Eng. Geol., Volume 42 (1990), pp. 75-84 | DOI
[36] Use of Schmidt hammer for estimating mechanical properties of weak rock, Proc. 6th Int. Assoc. Eng. Geol. Congr., Balkema, Rotterdam, 1990, pp. 511-519
[37] Point load-uniaxial compressive strength correlation, Proc. 7th ISRM Congress, Aachen, Germany, Volume 1, 1991, pp. 637-639
[38] Point-load strength: an index for classification of rock material, Bull. Int. Assoc. Eng. Geol., Volume 44 (1991), pp. 27-33 | DOI
[39] Problems and promises of index testing of rocks, Proc. 33rd US Symp. Rock Mech., Sante Fe, NM, Balkema, Rotterdam, 3–5, 1992, pp. 879-888
[40] An example of artificial neural network (ANN) application for indirect estimation of rock parameters, Rock Mech. Rock Eng., Volume 41 (2008) no. 5, pp. 781-795 | DOI
[41] Correlation between point load index and uniaxial compressive strength for different rock types, Rock Mech. Rock Eng., Volume 45 (2012), pp. 259-264 | DOI
[42] Prediction of uniaxial compressive strength of sandstones using petrography-based models, Eng. Geol., Volume 96 (2008) no. 3–4, pp. 141-158 | DOI
[43] Prediction of uniaxial compressive strength and modulus of elasticity for Travertine samples using regression and artificial neural networks, Int. J. Rock Mech. Min., Volume 20 (2010), pp. 41-46
[44] Application of neural network technique for prediction of uniaxial compressive strength using reservoir formation properties, Int. J. Rock Mech. Min. Sci., Volume 56 (2012), pp. 100-111 | DOI
[45] Prediction of unconfined compressive strength of carbonate rocks using artificial neural networks, Environ. Earth Sci., Volume 68 (2012) no. 3, pp. 807-819 | DOI
[46] Artificial neural networks and nonlinear regression techniques to assess the influence of slake durability cycles on the prediction of uniaxial compressive strength and modulus of elasticity for carbonate rocks, Int. J. Numer. Anal. Methods, Volume 36 (2012), pp. 1636-1650 | DOI
[47] Prediction of the strength and elasticity modulus of gypsum using multiple regression, ANN, and ANFIS models, Int. J. Rock Mech. Min. Sci., Volume 46 (2009) no. 4, pp. 803-810 | DOI
[48] An intelligent approach to predict unconfined compressive strength of rock surrounding access tunnels in longwall coal mining, Neural Comput. Appl., Volume 24 (2012) no. 1, pp. 233-241 | DOI
[49] Estimation of uniaxial compressive strength of rock materials by index tests using regression analysis and fuzzy inference system, Eng. Geol., Volume 160 (2013), pp. 54-68 | DOI
[50] Prediction of uniaxial compressive strength of some sedimentary rocks by fuzzy and regression models, Geotech. Geol. Eng., Volume 36 (2018), pp. 401-412 | DOI
[51] Knowledge-based model of material behaviour with neural networks, J. Eng. Mech., Volume 117 (1991) no. 1, pp. 132-153 | DOI
[52] Artificial Neural System: Foundation, Paradigms, Applications and Implementations, Pergamon, New York, 1990
[53] Prediction of strength properties of some schistose rocks from petrographic properties using artificial neural networks, Int. J. Rock Mech. Min. Sci., Volume 38 (2001) no. 2, pp. 269-284 | DOI
[54] Predicting of compressive and tensile strength of limestone via genetic programming, Expert Syst. Appl., Volume 35 (2008), pp. 111-123 | DOI
[55] Improving the prediction of the UCS by Equotip readings using statistical and neural network models, Mem. Centre Eng. Geol. Net, Volume 162 (1997), 127 pages
[56] Estimating the unconfined compressive strength and elastic modulus of a fault breccia mixture of weak rocks and strong matrix, Int. J. Rock Mech. Min. Sci., Volume 43 (2006), pp. 1277-1287 | DOI
[57] Estimation of strength parameters of rock using artificial neural networks, Bull. Eng. Geol. Environ., Volume 69 (2010), pp. 599-606 | DOI
[58] Numerical methods in rock mechanics, Int. J. Rock Mech. Min. Sci., Volume 39 (2010), pp. 409-427 | DOI
[59] The effect of initial weights on premature saturation in back-propagation learning, Proc. IEEE Int. Joint Conf. on Neural Networks, Seattle, WA, USA, 18–21, 1991, pp. 765-770 | DOI
[60] Prediction of airblast-overpressure induced by blasting using a hybrid artificial neural network and particle swarm optimization, Appl. Acoust., Volume 80 (2014), pp. 57-67 | DOI
[61] Fuzzy sets, Inf. Control, Volume 8 (1965), pp. 338-353 | DOI | Zbl
[62] ANFIS: adaptive-network-based fuzzy inference system, IEEE Trans. Syst. Man Cybern., Volume 23 (1993), pp. 665-685 | DOI
[63] Neuro-Fuzzy and Soft Computing, Prentice-Hall, Upper Saddle River, 1997
[64] Modeling tunnel boring machine performance by neuro-fuzzy methods, Tunn. Undergr. Space Technol., Volume 15 (2000) no. 3, pp. 260-269
[65] Prediction of ground vibrations resulting from the blasting operations in an open-pit mine by adaptive neuro-fuzzy inference system, Environ. Geol., Volume 56 (2008), pp. 97-107 | DOI
[66] An assessment on producing synthetic samples by fuzzy C-means for limited number of data in prediction models, Appl. Soft Comput., Volume 24 (2014), pp. 126-134 | DOI
[67] A new optimizer using particle swarm theory, Proc 6th Int. Symp. on Micro Machine and Human Science, Nagoya, Japan, 4–6, 1995, pp. 39-43 | DOI
[68] Particle swarms for feed forward neural net training, Proc. IEEE Int. Joint Conf. on Neural Networks, Honolulu, HI, USA, 12–17, 2002, pp. 1895-1899
[69] Blasting-induced flyrock and ground vibration prediction through an expert artificial neural network based on particle swarm optimization, Arab. J. Geosci., Volume 7 (2014) no. 12, pp. 5383-5396 | DOI
[70] Where and why artificial neural networks are applicable in civil engineering, J. Comput. Civ. Eng., Volume 8 (1994), pp. 129-130
[71] Prediction of uniaxial compressive strength of granitic rocks by various nonlinear tools and comparison of their performances, Int. J. Rock Mech. Min. Sci., Volume 62 (2013) no. 9, pp. 113-122 | DOI
[72] Prediction of the strength of mineral admixture concrete using multivariable regression analysis and an artificial neural network, Expert Syst. Appl., Volume 38 (2011), pp. 9609-9618 | DOI
[73] Development of optimal fuzzy models for predicting the strength of intact rocks, Comput. Geosci., Volume 54 (2013), pp. 107-112 | DOI
[74] A novel approach for blast-induced flyrock prediction based on imperialist competitive algorithm and artificial neural network, Sci. World J., Volume 2014 (2014) (article ID 643715) | DOI
[75] Suggested methods for the quantitative description of discontinuities in rock masses, Int. J. Rock Mech. Min. Sci. Geomech. Abstr., Volume 15 (1978), pp. 319-368
[76] Gene selection and classification of micro array data using random forest, BMC Bioinforma., Volume 7 (2006), 3 pages | DOI
[77] Random forests, Mach. Learn., Volume 45 (2001), pp. 5-32 | DOI | Zbl
[78] Bagging predictors, Mach. Learn., Volume 24 (1996), pp. 123-140 | DOI | Zbl
[79] An assessment of the effectiveness of a random forest classifier for land-cover classification, ISPRS J. Photogramm. Remote Sens., Volume 67 (2012), pp. 93-104 | DOI
[80] Refining a reconnaissance soil map by calibrating regression models with data from the same map (Normandy, France), Geoderma Regional., Volume 1 (2014), pp. 21-30 | DOI
[81] Soil organic carbon concentrations and stocks on Barro Colorado Island - digital soil mapping using random forests analysis, Geoderma, Volume 146 (2008), pp. 102-113 | DOI
[82] Digital mapping of soil organic matter stocks using random forest modeling in a semi-arid steppe ecosystem, Plant Soil, Volume 340 (2011), pp. 7-24 | DOI
[83] Newer classification and regression tree techniques: bagging and randomforests for ecological prediction, Ecosystems, Volume 9 (2006), pp. 181-199 | DOI
[84] Random forests for classification in ecology, Ecology, Volume 88 (2007), pp. 2783-2792 | DOI
[85] Random forest: a classification and regression tool for compound classification and QSAR modeling, J. Chem. Inf. Comput. Sci., Volume 43 (2003), pp. 1947-1958 | DOI
[86] Novel solutions for an old disease: diagnosis of acute appendicitis with random forest, support vector machines, and artificial neural networks, Surgery, Volume 149 (2011) no. 1, pp. 87-93 | DOI
[87] Prediction and analysis of cell-penetrating peptides using pseudo-amino acid composition and random forest models, Amino Acids, Volume 47 (2015) no. 7, pp. 1485-1493 | DOI
[88] Using random forest to model the domain applicability of another random forest model, J. Chem. Inf. Model., Volume 53 (2013) no. 11, pp. 2837-2850 | DOI
[89] Predition of RNA-binding residues in proteins from primary sequence using an enriched random forest model with a novel hybrid feature, Proteins-Struct. Funct. Bioinform., Volume 79 (2011) no. 4, pp. 1230-1239 | DOI
[90] Fault diagnosis in spur gears based on genetic algorithm and random forest, Mech. Syst. Signal Process., Volume 70–71 (2016), pp. 87-103 | DOI
[91] Test Methods for Ultra Violet Velocities Determination, American Society for Testing and Materials, 1983, D2845 pages
[92] Suggested methods for determining the uniaxial compressive strength and deformability of rock materials, Int. J. Rock Mech. Min. Sci. Geomech. Abstr., Volume 16 (1979), pp. 135-140
[93] Study on uniaxial compressive strength, point load strength index, dynamic and physical properties of serpentinites from Central Greece: test results and empirical relations, Eng. Geol., Volume 108 (2009), pp. 199-207 | DOI
[94] A fuzzy triangular chart to predict the uniaxial compressive strength of the Ankara agglomerates from their petrographic composition, Eng. Geol., Volume 66 (2002), pp. 39-51 | DOI
[95] Applying soft computing methods to predict the uniaxial compressive strength of rock from schmidt hammer rebound values, Comput. Geosci., Volume 21 (2017) no. 1, pp. 1-17 | MR
[96] A comparative evaluation of rock strength measures, Int. J. Rock Mech. Min. Sci., Volume 21 (1984), pp. 233-248 | DOI
[97] Prediction of engineering properties of a selected litharenite sandstone from its petrographic characteristics using correlation and multivariate statistical techniques, Eng. Geol., Volume 38 (1994), pp. 135-157 | DOI
[98] Genetic algorithms and simulated annealing: a marriage proposal, Int. Symp. Neural Netw., Volume 2 (1993), pp. 1104-1109 | DOI
[99] Point load test on meta-sedimentary rocks and correlation to UCS and BTS, Rock Mech. Rock Eng., Volume 46 (2013) no. 4, pp. 889-896 | DOI
[100] Rock strength as a metric of welding intensity in pyroclastic deposits, Eur. J. Mineral., Volume 15 (2003), pp. 855-864 | DOI
[101] Considerations on strength of intact sedimentary rocks, Eng. Geol., Volume 72 (2004), pp. 261-273 | DOI
[102] The influence of porosity on tensile and compressive strength of porous chalk, Rock Mech. Rock Eng., Volume 37 (2004) no. 4, pp. 331-341 | DOI
[103] Development of a new method for estimating the indirect uniaxial compressive strength of rock using Schmidt hammer, BHM Berg- Huettenmaenn Monatsh, Volume 156 (2011) no. 4, pp. 142-146 | DOI
[104] Uniaxial compressive strength and point load strength, Int. J. Rock Mech. Min. Sci., Volume 33 (1996), pp. 183-188 | DOI
[105] Schmidt sertlik cekici kullanılarak tahmin edilen tek eksenli basınç dayanımı verilerinin guvenirligi uzerine bir degerlendirme, Jeol. Muh., Volume 48 (1996), pp. 78-81
[106] Correlating uniaxial compressive strength with Schmidt hammer rebound number, point load index, Young’s modulus, and mineralogy of gabbros and basalts (Northern Greece), Bull. Eng. Geol., Volume 54 (1996), pp. 3-11
[107] Basınc direnci tahmininde Schmidt venokta yuk indeksi kullanmanın guvenirligi, KTU Jeoloji Muhendisligi Bolumu 30. Yıl Sempozyumu BildirilerKitabı, Trabzon (S. Korkmazve; M. Akcay, eds.), 1996, pp. 362-369
[108] The point load test for weak rock in dredging applications, Int. J. Rock Mech. Min. Sci., Volume 34 (1997) no. 3–4, 702 pages
[109] Correlation of mineralogical and textural, characteristics with engineering properties of selected granitic rocks from Turkey, Eng. Geol., Volume 51 (1999), pp. 303-317 | DOI
[110] Evaluation of mechanical rock properties using a Schmidt hammer, Int. J. Rock Mech. Min. Sci., Volume 37 (2000), pp. 723-728 | DOI
[111] Evaluation of the block punch index test with particular reference to the size effect, failure mechanism and its effectiveness in predicting rock strength, Int. J. Rock Mech. Min. Sci., Volume 38 (2001), pp. 1091-1111 | DOI
[112] Evaluation of simple methods for assessing the uniaxial compressive strength of rock, Int. J. Rock Mech. Min. Sci., Volume 38 (2001), pp. 981-994 | DOI
[113] Correlation of Schmidt hardness with unconfined compressive strength and Young’s modulus in gypsum from Sivas (Turkey), Eng. Geol., Volume 66 (2002), pp. 211-219 | DOI
[114] Estimation of rock physicomechanical properties using hardness methods, Eng. Geol., Volume 71 (2004), pp. 281-288 | DOI
[115] Correlating sound velocity with the density, compressive strength and Young’s modulus of carbonate rocks, Int. J. Rock Mech. Min. Sci., Volume 5 (2004), pp. 871-875 | DOI
[116] Correlation between Schmidt hardness, uniaxial compressive strength and Young’s modulus for andesites, basalts and tuffs, Bull. Eng. Geol. Environ., Volume 63 (2004), pp. 141-148 | DOI
[117] The Schmidt hammer in rock material characterization, Eng. Geol., Volume 81 (2005), pp. 1-14 | DOI
[118] The relationship between effective porosity, uniaxial compressive strength and sonic velocity of intact Borrowdale volcanic group core samples from Sellafield, Geotech. Geol. Eng., Volume 23 (2005) no. 6, pp. 793-809 | DOI
[119] The effect of porosity on the relation between uniaxial compressive strength and point load index, Int. J. Rock Mech. Min. Sci., Volume 42 (2005) no. 4, pp. 584-589 | DOI
[120] A comparative evaluation of indirect methods to estimate the compressive strength of rocks, Rock Mech. Rock Eng., Volume 38 (2005) no. 4, pp. 329-343 | DOI
[121] Predicting uniaxial compressive strength by point load test: significance of cone penetration, Rock Mech. Rock Eng., Volume 39 (2006) no. 5, pp. 483-490 | DOI
[122] Correlation between uniaxial compressive strength and point load index for salt-range rocks, Pak. J. Eng. Appl. Sci., Volume 1 (2007), pp. 1-8
[123] Estimation of rock engineering properties using hardness tests, Eng. Geol., Volume 90 (2007), pp. 138-147 | DOI
[124] The uniaxial compressive strength of soft rock, Civ. Eng. Dimens., Volume 9 (2007), pp. 9-14
[125] Estimation of uniaxial compressive strength from point load strength, Schmidt hardness and P-wave velocity, Bull. Eng. Geol. Environ., Volume 67 (2008), pp. 491-498 | DOI
[126] A correlation between P-wave velocity, impact strength index, slake durability index and uniaxial compressive strength, Bull. Eng. Geol. Environ., Volume 67 (2008), pp. 17-22 | DOI
[127] Determination of mechanical properties of rocks using simple methods, Bull. Eng. Geol. Environ., Volume 67 (2008), pp. 237-244 | DOI
[128] Predicting uniaxial compressive strength, modulus of elasticity and index properties of rocks using the Schmidt hammer, Bull. Eng. Geol. Environ., Volume 68 (2009), pp. 55-63 | DOI
[129] Index properties and strength variation controlled by microstructure for sedimentary rocks, Eng. Geol., Volume 97 (2009), pp. 80-90 | DOI
[130] Predicting the uniaxial compressive strength and static Young’s modulus of intact sedimentary rocks using the ultrasonic test, Int. J. Geomech., Volume 9 (2009) no. 1, pp. 14-19 | DOI
[131] Non-destructive testing of some higher Himalayan rocks in the Satluj Valley, Bull. Eng. Geol. Environ., Volume 68 (2009), pp. 409-416 | DOI
[132] Correlating static properties of coal measures rocks with P-wave velocity, Int. J. Coal Geol., Volume 79 (2009), pp. 55-60 | DOI
[133] Predicting the relationships between brittleness and mechanical properties (UCS, TS and SH) of rocks, Sci. Res. Essays, Volume 5 (2010), pp. 2107-2118
[134] Application of Schmidt rebound number for estimating rock strength under specific geological conditions, J. Min. Sci., Volume 1 (2010) no. 2, pp. 1-8
[135] P-wave velocity test for assessment of geotechnical properties of some rock materials, Bull. Mater. Sci., Volume 34 (2011) no. 4, pp. 947-953 | DOI
[136] Physical and mechanical properties of Gokcseda: Imbros (NE Aegean Sea) Island andesites, Bull. Eng. Geol. Environ., Volume 69 (2011), pp. 321-324 | DOI
[137] Correlating wave velocities with physical, mechanical properties and petrographic characteristics of peridotites from the central Greece, Geotech. Geol. Eng., Volume 29 (2011) no. 6, pp. 1049-1062 | DOI
[138] Correlations Between Index Properties and Unconfined Compressive Strength of Weathered Ocala Limestone, University of North Florida, Jacksonville, 2011, 142 pages
[139] Predicting the uniaxial compressive and tensile strengths of gypsum rock by point load testing, Rock Mech. Rock Eng., Volume 45 (2012) no. 2, pp. 265-273 | DOI
[140] Use of the block punch test to predict the compressive and tensile strengths of rocks, Int. J. Rock Mech. Min. Sci., Volume 51 (2012), pp. 119-127 | DOI
[141] Relationship between point load strength index and uniaxial compressive strength of hydrothermally altered soft rocks, Int. J. Rock Mech. Min. Sci., Volume 50 (2012), pp. 147-157 | DOI
[142] Predicting the compressive and tensile strength of rocks from indentation hardness index, J. South. Afr. Ins. Min. Metall., Volume 112 (2012) no. 5, pp. 331-339
[143] Correlating P-wave velocity with the physico-mechanical properties of different rocks, Pure Appl. Geophys., Volume 170 (2013), pp. 507-514 | DOI
[144] Correlation between unconfined compressive strength and indirect tensile strength of limestone rock samples, Electr. J. Geotech. Eng., Volume 18 (2013), pp. 1737-1746
[145] Statistical method for assessing the uniaxial compressive strength of carbonate rock by Schmidt hammer tests performed on core samples, Rock Mech. Rock Eng., Volume 46 (2013) no. 1, pp. 199-206 | DOI
[146] Using the Schmidt hammer on rock mass characteristic in sedimentary rock at Tutupan Coal Mine, Procedia. Earth Planet. Sci., Volume 6 (2013), pp. 390-395 | DOI
[147] Prediction of the unconfined compressive strength of soft rocks: a PSO-based ANN approach, Bull. Eng. Geol. Environ., Volume 74 (2015) no. 3, pp. 745-757 | DOI
[148] An adaptive neurofuzzy inference system for predicting unconfined compressive strength and Young’s modulus: a study on Main Range granite, Bull. Eng. Geol. Environ., Volume 74 (2015) no. 4, pp. 1301-1319 | DOI
[149] Correlation of Schmidt rebound hardness with uniaxial compressive strength and P-wave velocity of rock materials, Arab. J. Sci. Eng., Volume 40 (2015) no. 7, pp. 1897-1906 | DOI
[150] Prediction of the strength and elasticity modulus of granite through an expert artificial neural network, Arab. J. Geosci., Volume 9 (2016), 48 pages | DOI
[151] Rock strength assessment based on regression tree technique, Eng. Comput., Volume 32 (2016), pp. 343-354 | DOI
[152] Application of statistical methods for predicting uniaxial compressive strength of limestone rocks using nondestructive tests, Acta Geotechnica, Volume 12 (2017) no. 2, pp. 1-13 | DOI
[153] Estimation of uniaxial compressive strength of North Algeria sedimentary rocks using density, porosity, and Schmidt hardness, Arab. J. Geosci., Volume 10 (2017), 383 pages | DOI
[154] A validation study for the estimation of uniaxial compressive strength based on index tests, Rock Mech. Rock Eng., Volume 51 (2018), pp. 2289-2297 | DOI
[155] Fuzzy and multiple regression modelling for evaluation of intact rock strength based on point load, Schmidt hammer and sonic velocity, Rock Mech. Rock Eng., Volume 39 (2006) no. 1, pp. 45-57 | DOI
[156] Predicting intact rock strength for mechanical excavation using multivariate statistics, artificial neural networks, and regression trees, Eng. Geol., Volume 99 (2008), pp. 51-60 | DOI
[157] Estimating the uniaxial compressive strength of some clay-bearing rocks selected from Turkey by nonlinear multivariable regression and rule-based fuzzy models, Expert Syst., Volume 26 (2009), pp. 176-190 | DOI
[158] Prediction of compressive and tensile strength of Gaziantep basalts via neural networks and gene expression programming, Neural Comput. Appl., Volume 18 (2009), pp. 1031-1041 | DOI
[159] Modeling of the uniaxial compressive strength of some clay-bearing rocks using neural network, Appl. Soft Comput., Volume 11 (2011), pp. 2587-2594 | DOI
[160] Application of genetic programming to predict the uniaxial compressive strength and elastic modulus of carbonate rocks, Int. J. Rock Mech. Min. Sci., Volume 63 (2013), pp. 159-169 | DOI
[161] Modeling uniaxial compressive strength of building stones using non-destructive test results as neutral networks input parameters, Constr. Build. Mater., Volume 47 (2013), pp. 1010-1019 | DOI
[162] Selection of regression models for predicting strength and deformability properties of rocks using GA, Int. J. Min. Sci. Technol., Volume 23 (2013), pp. 495-501 | DOI
[163] Application of support vector machines and relevance vector machines in predicting uniaxial compressive strength of volcanic rocks, J. Afr. Earth Sci., Volume 100 (2014), pp. 634-644 | DOI
[164] Application of artificial neural networks and multivariate statistics to predict UCS and E using physical properties of Asmari limestones, Arab. J. Geosci., Volume 8 (2015) no. 5, pp. 2889-2897 | DOI
[165] Prediction of uniaxial compressive strength of rock samples using hybrid particle swarm optimization-based artifical neural networks, Measurement, Volume 60 (2015), pp. 50-63 | DOI
[166] Prediction of the uniaxial compressive strength of sandstone using various modeling techniques, Int. J. Rock Mech. Min. Sci., Volume 85 (2016), pp. 174-186 | DOI
[167] Predicting elastic properties of intact rocks from index tests using multiple regression modelling, Int. J. Rock Mech. Min. Sci., Volume 42 (2005), pp. 323-330 | DOI
[168] The effect of Schmidt hammer type on uniaxial compressive strength prediction of rock, Int. J. Rock Mech. Min. Sci., Volume 44 (2007), pp. 299-307 | DOI
[169] Estimating the unconfined compressive strength of intact rocks from Equotip hardness, Bull. Eng. Geol. Environ., Volume 67 (2008), pp. 23-29 | DOI
[170] Nail penetration test for determining the uniaxial compressive strength of rock, Int. J. Rock Mech. Min. Sci., Volume 47 (2010) no. 2, pp. 265-271 | DOI
[171] Function identification for the intrinsic strength and elastic properties of granitic rock via genetic programming (GP), Comput. Geosci., Volume 37 (2011) no. 9, pp. 1318-1323 | DOI
[172] Relationship between textural, petrophysical and mechanical properties of quartzites: a case study from northwestern Himalaya, Eng. Geol., Volume 135–136 (2012), pp. 1-9 | DOI
[173] Soft computing method for assessment of compressional wave velocity, Scientia Iranica, Volume 19 (2012) no. 4, pp. 1018-1024 | DOI
[174] Regression analysis and ANN models to predict rock properties from sound levels produced during drilling, Int. J. Rock Mech. Min. Sci., Volume 58 (2013), pp. 61-72 | DOI
[175] Assessment of relationships between drilling rate index and mechanical properties of rocks, Tunn. Undergr. Space Tech., Volume 33 (2013), pp. 46-53 | DOI
[176] Predictive model for uniaxial compressive strength for Grade III granitic rocks from Macao, Eng. Geol., Volume 199 (2015), pp. 28-37 | DOI
[177] Drilling rate prediction of an open pit mine using the rock mass drillability index, Int. J. Rock Mech. Min. Sci., Volume 73 (2015), pp. 130-138 | DOI
[178] Soft computing methods for estimating the uniaxial compressive strength of intact rock from index tests, Int. J. Rock Mech. Min. Sci., Volume 80 (2015), pp. 418-424 | DOI
[179] Estimation of strength characteristics of different Himalayan rocks from Schmidt hammer rebound, point load index, and compressional wave velocity, Bull. Eng. Geol. Environ., Volume 74 (2015), pp. 521-533 | DOI
[180] Correlating physico-mechanical properties of intact rocks with P-wave velocity, Acta Geod. Geophys., Volume 51 (2016), pp. 571-582 | DOI
[181] Influences of petrographic and textural properties on the strength of very strong granite rocks, Environ. Earth Sci., Volume 75 (2016) no. 22, 1461 pages | DOI
[182] Application of several non-linear prediction tools for estimating uniaxial compressive strength of granitic rocks and comparison of their performances, Eng. Comput., Volume 32 (2016), pp. 189-206 | DOI
[183] The use of index tests to determine the mechanical properties of crushed aggregates from precambrian basement complex rocks, Ado-Ekiti, SW Nigeria, J. Afr. Earth Sci., Volume 129 (2017), pp. 659-667 | DOI
[184] Prediction of mechanical behaviour from mineralogical composition of Sakesar limestone, Central Salt Range, Pakistan, Bull. Eng. Geol. Environ., Volume 76 (2017), pp. 601-615 | DOI
[185] Model tree approach for predicting uniaxial compressive strength and Young’s modulus of carbonate rocks, Bull. Eng. Geol. Environ., Volume 77 (2018), pp. 331-343 | DOI
- Optimized Gradient Boosting Models for Adaptive Prediction of Uniaxial Compressive Strength in Carbonate Rocks Using Drilling Data, ACS Omega, Volume 10 (2025) no. 11, p. 11016 | DOI:10.1021/acsomega.4c09603
- A Comparative Study of UCS Results Obtained from Triaxial Tests Under Multiple Failure State Conditions (Test Type II), Applied Sciences, Volume 15 (2025) no. 6, p. 3176 | DOI:10.3390/app15063176
- Evaluation of rock pillar failure mechanisms under uniaxial compression: impact of joint number and joint angle, Computational Particle Mechanics, Volume 12 (2025) no. 1, p. 205 | DOI:10.1007/s40571-024-00787-y
- A review of test methods for uniaxial compressive strength of rocks: Theory, apparatus and data processing, Journal of Rock Mechanics and Geotechnical Engineering, Volume 17 (2025) no. 3, p. 1889 | DOI:10.1016/j.jrmge.2024.05.003
- Relationship Between Schmidt Hammer Rebound Hardness Test and Concrete Strength Tests for Limestone Aggregate Concrete Based on Experimental and Statistical Study, Materials, Volume 18 (2025) no. 6, p. 1388 | DOI:10.3390/ma18061388
- Estimation of Uniaxial Strength of Rock: A Comparison between Bayesian-Optimized Machine Learning Models, Mining, Metallurgy Exploration, Volume 42 (2025) no. 1, p. 133 | DOI:10.1007/s42461-024-01168-y
- Influence of rock properties on thrust and torque during rock coring operations a case study, Petroleum Science and Technology, Volume 43 (2025) no. 4, p. 426 | DOI:10.1080/10916466.2023.2294158
- A Novel Hybrid Bayesian-Group-Based Machine Learning (HB-GML) Method for Predicting Uniaxial Compressive Strength (UCS) of Rock, Rock Mechanics and Rock Engineering (2025) | DOI:10.1007/s00603-024-04375-7
- Analysis of the roof damage range in close-proximity gently inclined coal seams mining and the feasibility of upward mining, Scientific Reports, Volume 15 (2025) no. 1 | DOI:10.1038/s41598-025-89808-1
- Overlying strata movement law and rockburst prevention control for fully mechanized top coal caving face in extra-thick coal seams, Scientific Reports, Volume 15 (2025) no. 1 | DOI:10.1038/s41598-025-89133-7
- Study on the initiation and propagation of mining induced fractures by underground coal mining in the loess gully region, Scientific Reports, Volume 15 (2025) no. 1 | DOI:10.1038/s41598-025-93142-x
- Uniaxial Compressive Strength Prediction for Rock Material in Deep Mine Using Boosting-Based Machine Learning Methods and Optimization Algorithms, Computer Modeling in Engineering Sciences, Volume 140 (2024) no. 1, p. 275 | DOI:10.32604/cmes.2024.046960
- Artificial Intelligence Prediction of One-Part Geopolymer Compressive Strength for Sustainable Concrete, Computer Modeling in Engineering Sciences, Volume 141 (2024) no. 1, p. 525 | DOI:10.32604/cmes.2024.052505
- Hybrid PSO with tree-based models for predicting uniaxial compressive strength and elastic modulus of rock samples, Frontiers in Earth Science, Volume 12 (2024) | DOI:10.3389/feart.2024.1337823
- Bayesian optimization-enhanced ensemble learning for the uniaxial compressive strength prediction of natural rock and its application, Geohazard Mechanics, Volume 2 (2024) no. 3, p. 197 | DOI:10.1016/j.ghm.2024.05.002
- Investigating the Relationship Between Failure Patterns and Mechanical Properties of Rock, Journal of Physics: Conference Series, Volume 2907 (2024) no. 1, p. 012026 | DOI:10.1088/1742-6596/2907/1/012026
- Assessment of the uniaxial compressive strength of intact rocks: an extended comparison between machine and advanced machine learning models, Multiscale and Multidisciplinary Modeling, Experiments and Design, Volume 7 (2024) no. 4, p. 3301 | DOI:10.1007/s41939-024-00408-4
- Prediction of Uniaxial Strength of Rocks Using Relevance Vector Machine Improved with Dual Kernels and Metaheuristic Algorithms, Rock Mechanics and Rock Engineering, Volume 57 (2024) no. 8, p. 6227 | DOI:10.1007/s00603-024-03849-y
- Characteristics of deformation and damage of surrounding rock along the top roadway in the working face of an isolated island and its evolution law, Scientific Reports, Volume 14 (2024) no. 1 | DOI:10.1038/s41598-024-63246-x
- An exploration of improving stability of gob-side entry retained with graded and zoned pressure relief technology: a case study, Scientific Reports, Volume 14 (2024) no. 1 | DOI:10.1038/s41598-024-79844-8
- Study on fissure evolution of overlying rock in lower protective mining, Scientific Reports, Volume 14 (2024) no. 1 | DOI:10.1038/s41598-024-68483-8
- Comprehensive study on the Python-based regression machine learning models for prediction of uniaxial compressive strength using multiple parameters in Charnockite rocks, Scientific Reports, Volume 14 (2024) no. 1 | DOI:10.1038/s41598-024-58001-1
- Numerical investigation on the fracture and mechanical behaviors of marble containing two parallel fissures under triaxial compression conditions using FDEM, Theoretical and Applied Fracture Mechanics, Volume 131 (2024), p. 104408 | DOI:10.1016/j.tafmec.2024.104408
- Assessment of Uniaxial Strength of Rocks: A Critical Comparison Between Evolutionary and Swarm Optimized Relevance Vector Machine Models, Transportation Infrastructure Geotechnology, Volume 11 (2024) no. 6, p. 4098 | DOI:10.1007/s40515-024-00433-3
- Study on Crack Propagation of Rock Bridge in Rock-like Material with Fractures under Compression Loading with Sudden Change Rate, Applied Sciences, Volume 13 (2023) no. 7, p. 4354 | DOI:10.3390/app13074354
- Computational intelligence approaches for estimating the unconfined compressive strength of rocks, Arabian Journal of Geosciences, Volume 16 (2023) no. 1 | DOI:10.1007/s12517-022-11085-3
- Feasibility of Artificial Intelligence Techniques in Rock Characterization, Artificial Intelligence in Mechatronics and Civil Engineering (2023), p. 93 | DOI:10.1007/978-981-19-8790-8_4
- Comparison of artificial intelligence and multivariate regression methods in predicting the uniaxial compressive strength of rock during the specific resistivity monitoring, Bulletin of Engineering Geology and the Environment, Volume 82 (2023) no. 11 | DOI:10.1007/s10064-023-03415-w
- Estimation of unconfined compressive strength of marine clay modified with recycled tiles using hybridized extreme gradient boosting method, Construction and Building Materials, Volume 393 (2023), p. 131992 | DOI:10.1016/j.conbuildmat.2023.131992
- Modeling of Tunnel Boring Machine Performance Employing Random Forest Algorithm, Geotechnical and Geological Engineering, Volume 41 (2023) no. 7, p. 4205 | DOI:10.1007/s10706-023-02516-3
- Investigating average infrared radiation temperature characteristics during shear and tensile cracks in sandstone under different water contents, Infrared Physics Technology, Volume 135 (2023), p. 104968 | DOI:10.1016/j.infrared.2023.104968
- Prediction of CSG splitting tensile strength based on XGBoost-RF model, Materials Today Communications, Volume 34 (2023), p. 105350 | DOI:10.1016/j.mtcomm.2023.105350
- Prediction of Coal Dilatancy Point Using Acoustic Emission Characteristics: Insight Experimental and Artificial Intelligence Approaches, Mathematics, Volume 11 (2023) no. 6, p. 1305 | DOI:10.3390/math11061305
- Prediction of uniaxial compressive strength of rock based on lithology using stacking models, Rock Mechanics Bulletin, Volume 2 (2023) no. 4, p. 100081 | DOI:10.1016/j.rockmb.2023.100081
- Machine learning models for predicting unconfined compressive strength: A case study for Precambrian basement complex rocks from Ado-Ekiti, Southwestern Nigeria, Scientific African, Volume 20 (2023), p. e01715 | DOI:10.1016/j.sciaf.2023.e01715
- Study on strength characteristics and thickening characteristics of classified-fine cemented backfill in gold mine, Scientific Reports, Volume 13 (2023) no. 1 | DOI:10.1038/s41598-023-35254-w
- HYFIS vs FMR, LWR and Least squares regression methods in estimating uniaxial compressive strength of evaporitic rocks, Scientific Reports, Volume 13 (2023) no. 1 | DOI:10.1038/s41598-023-41349-1
- Appraisal of Different Artificial Intelligence Techniques for the Prediction of Marble Strength, Sustainability, Volume 15 (2023) no. 11, p. 8835 | DOI:10.3390/su15118835
- Performance of Statistical and Intelligent Methods in Estimating Rock Compressive Strength, Sustainability, Volume 15 (2023) no. 7, p. 5642 | DOI:10.3390/su15075642
- Experimental Study of Rock-like Materials with Two Unequal Parallel Preexisting Fissures under Uniaxial Compression, Applied Sciences, Volume 12 (2022) no. 20, p. 10404 | DOI:10.3390/app122010404
- Prediction of Rock Abrasivity Index (RAI) and Uniaxial Compressive Strength (UCS) of Granite Building Stones Using Nondestructive Tests, Geotechnical and Geological Engineering, Volume 40 (2022) no. 6, p. 3343 | DOI:10.1007/s10706-022-02095-9
- Machine Learning Prediction of the Load Evolution in Three-Point Bending Tests of Marble, Mining, Metallurgy Exploration, Volume 39 (2022) no. 5, p. 2037 | DOI:10.1007/s42461-022-00674-1
- Estimation of Unconfined Compressive Strength (UCS) of Carbonate Rocks by Index Mechanical Tests and Specimen Size Properties: Central Alborz Zone of Iran, Rock Mechanics and Rock Engineering, Volume 55 (2022) no. 1, p. 125 | DOI:10.1007/s00603-021-02532-w
- Several machine learning techniques comparison for the prediction of the uniaxial compressive strength of carbonate rocks, Scientific Reports, Volume 12 (2022) no. 1 | DOI:10.1038/s41598-022-25633-0
- Investigation of Some Property Changes of Light-Colored Turkish Natural Stones after High-Temperature Treatments, Sustainability, Volume 14 (2022) no. 16, p. 10298 | DOI:10.3390/su141610298
- Application of Machine Learning and Multivariate Statistics to Predict Uniaxial Compressive Strength and Static Young’s Modulus Using Physical Properties under Different Thermal Conditions, Sustainability, Volume 14 (2022) no. 16, p. 9901 | DOI:10.3390/su14169901
- Prediction Compressive Strength of Concrete Containing GGBFS using Random Forest Model, Advances in Civil Engineering, Volume 2021 (2021) no. 1 | DOI:10.1155/2021/6671448
- Prediction of uniaxial compressive strength of rock samples using density weighted least squares twin support vector regression, Neural Computing and Applications, Volume 33 (2021) no. 22, p. 15843 | DOI:10.1007/s00521-021-06204-2
- A preliminary application of a machine learning model for the prediction of the load variation in three-point bending tests based on acoustic emission signals, Procedia Structural Integrity, Volume 33 (2021), p. 251 | DOI:10.1016/j.prostr.2021.10.031
Cité par 49 documents. Sources : Crossref
Commentaires - Politique
Vous devez vous connecter pour continuer.
S'authentifier