1 Introduction
‘Cancer dormancy’ is an operational term used to describe the phenomenon of a prolonged quiescent state in which tumour cells are present, but tumour progression is not clinically apparent [1–3]. As a condition, cancer dormancy is often observed in breast cancers, neuroblastomas, melanomas, osteogenic sarcomas, and in several types of lymphomas, and is often found ‘accidentally’ in tissue samples of healthy individuals who have died suddenly [4,5]. In some cases, cancer dormancy has been found in cancer patients after several years of front-line therapy and clinical remission. The presence of these cancer cells in the body determines, finally, the outcome of the disease. In particular, age, stress factors, infections, act of treatment itself or other alterations in the host can provoke the initiation of uncontrolled growth of initially dormant cancer cells and subsequent waves of metastases [2,6]. Recently, some molecular targets for the induction of cancer dormancy and the re-growth of a dormant tumour have been identified [7,8]. However, the precise nature of the phenomenon remains poorly understood.
One of the main factors (but not the only one) contributing to the induction and maintenance of cancer dormancy is the reaction of the host immune system to the tumour cells [1,2]. Indeed, tumour-associated antigens can be expressed on tumour cells at very early stages of tumour progression [9] and, as a consequence, during the avascular stage, tumour development can be effectively controlled by tumour-infiltrating cytotoxic lymphocytes (TICLs) [10]. The TICLs may be cytotoxic lymphocytes (CD8+ CTLs), natural killer-like (NK-like) cells and/or lymphokine activated killer (LAK) cells [11–14].
In [15] we developed a mathematical model for the spatio-temporal response of cytotoxic T-lymphocytes to a solid tumour. For a particular choice of parameters the model was able to simulate the phenomenon of cancer dormancy by depicting spatially unstable and heterogeneous tumour cell distributions that were nonetheless characterized by a relatively small total number of tumour cells. This behaviour was consistent with several immunomorphological investigations. However, the alteration of certain parameters of the model was enough to induce bifurcations into the system, which in turn resulted in the existence of travelling-wave-like solutions in the numerical simulations. These travelling waves were of great importance because when they existed, the tumour invaded the healthy tissue at its full potential escaping the host's immune surveillance.
It is worth mentioning that the cancer dormancy solutions were characterized by an irregular evolution, which according to several objective indications, was an actual manifestation of spatio-temporal chaos. In particular, a bifurcation analysis of the ODE kinetics of our system has revealed the existence of oscillatory solutions for the ODE system emerging through a Hopf bifurcation. We have presented these results in [15] and have indicated several connections with spatio-temporal chaotic systems that couple oscillatory kinetics with diffusion (see, for example, the excellent work on λ–ω systems presented in [16]). Furthermore, we have been able to correlate numerically the existence of the stable limit cycle that emerged through the Hopf bifurcation with the irregular spatio-temporal evolution of the PDE system and the onset of cancer dormancy.
In recent years several papers have begun to investigate the mathematical modelling of the various aspects of the immune system response to cancer. The development of models which reflect several spatial and temporal aspects of tumour immunology can be regarded as the first step towards an effective computational approach in investigating the conditions under which tumour recurrence takes place and in the optimising of both spatial and temporal aspects of the application of various immunotherapies. Key papers in this area include [17–22], which focus on the modelling of tumour progression and immune competition by generalized kinetic (Boltzmann) models and [23–26], which focus on the development of tumour heterogeneities as a result of tumour cell and macrophage interactions. Moreover, [27] is concerned with receptor–ligand (Fas–FasL) dynamics, [28] investigates the process of macrophage infiltration into avascular tumours, [15,29,30] focus on the dynamics of tumour cell–TICL interactions, and finally [31] and [32] analyze various immune system and immunotherapy models in the context of cancer dynamics.
In this paper we undertake a travelling-wave analysis of a sub-system of the model presented in [15]. The full system involved some spatially non-uniform kinetic terms through the introduction of a Heaviside function modelling some aspects of the geometry over which the system was solved. This spatial non-uniformity in the kinetics complicates the travelling-wave analysis and for the sake of mathematical simplicity it is not treated here (see also the comments in [15] concerning the effect that the Heaviside function in the kinetics has on the spatio-temporal simulations). Furthermore, we also do not consider the chemotaxis aspect of the full system. Thus the model under consideration will be a nonlinear reaction–diffusion system of partial differential equations.
2 The mathematical model
For the sake of completeness we first of all introduce the complete model as it has been discussed in [15]. Let us consider a simplified process of a small, growing, avascular tumour which elicits a response from the host immune system and attracts a population of lymphocytes. The growing tumour is directly attacked by TICLs [33–35] which, in turn, secrete soluble diffusible factors (chemokines). These factors enable the TICLs to respond in a chemotactic manner (in addition to random motility) and migrate towards the tumour cells. Our model will therefore consist of six dependent variables denoted E, T, C, , and α, which are the local densities/concentrations of TICLs, tumour cells, TICL–tumour cell complexes, inactivated TICLs, ‘lethally hit’ (or ‘programmed-for-lysis’) tumour cells, and a single (generic) chemokine, respectively.
The local interactions between the TICLs and tumour cells may be described by the simplified kinetic scheme given in Fig. 1 (see [30] and [15] for full details). The parameters , and are non-negative kinetic constants: and describe the rate of binding of TICLs to tumour cells and detachment of TICLs from tumour cells without damaging cells; is the rate of detachment of TICLs from tumour cells, resulting in an irreversible programming of the tumour cells for lysis (i.e. death) with probability p or inactivating/killing TICLs with probability . Using the law of mass action the above kinetic scheme can be ‘translated’ into a system of ordinary differential equations. Furthermore, we consider other kinetic interaction terms between the variables and examine migration mechanisms for the TICLs, tumour cells and also consider diffusion of the chemokines. We assume that there is no ‘nonlinear’ migration of cells and no nonlinear diffusion of chemokine i.e. all random motility, chemotaxis and diffusion coefficients are assumed constant.
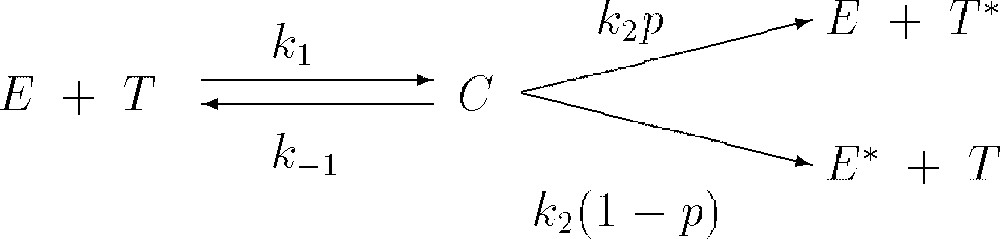
Schematic diagram of local lymphocyte–cancer cell interactions.
We assume that the TICLs have an element of random motility and also respond chemotactically to the chemokines. There is a source term modelling the underlying TICL production by the host immune system, a linear decay (death) term and an additional TICL proliferation term in response to the presence of the tumour cells. Combining these assumptions with the local kinetics (derived from Fig. 1) we have the following PDE for TICLs:
(1) |
We assume that the chemokines are produced when lymphocytes are activated by tumour cell–TICL interactions. Thus we define chemokine production to be proportional to tumour cell–TICL complex density C. Once produced the chemokines are assumed to diffuse throughout the tissue and to decay in a simple manner with linear decay kinetics. Therefore the PDE for the chemokine concentration is:
(2) |
We assume that migration of the tumour cells may be described by simple random motility and that on the kinetic level the growth dynamics of a solid tumour may be described adequately by a logistic term (see [15] for a full discussion concerning the validity of these assumptions). Hence the PDE governing the evolution of tumour cell density is:
(3) |
We assume that there is no diffusion of the complexes, only interactions governed by the local kinetics derived from Fig. 1. The absence of a diffusion term is justified by the fact that formation and dissociation of complexes occurs on a time scale of tens of minutes, whereas the random motility of the tumour cells, for example, occurs on a time scale of tens of hours. Thus, the cell–cell complexes do not have time to move. Therefore the equation for the complexes is given by:
(4) |
We assume that inactivated and ‘lethally hit’ cells (i.e. cells that will die) are quickly eliminated from the tissue (for example, by macrophages) and do not substantially influence the immune processes being analysed. Inactivated cells also do not migrate and therefore we have:
(5) |
(6) |
It is easy to see that Eqs. (5) and (6) are only coupled to the full system through the complexes C and that neither nor have any effect on the variable C. Thus, Eqs. (1)–(4) essentially dictate the behaviour of the complete system.
The system of Eqs. (1)–(4) is closed by applying appropriate boundary and initial conditions. In the one-dimensional case, we define the spatial domain to be the interval and we assume that there are two distinct regions in this interval – one region entirely occupied by tumour cells, the other entirely occupied by the immune cells. We propose that an initial interval of tumour localization is , where . In this framework the function (cf. Eq. (1)) is defined by:
(7) |
(8) |
The closed system is non-dimensionalized by choosing an order-of-magnitude scale for the E, T and C cell densities, of , and , respectively, as suggested by the initial conditions. The chemokine concentration α is normalised through some reference concentration discussed in [15]. Time is scaled relative to the diffusion rate of the TICLs, i.e. and the space variable x is scaled relative to the length of the region under consideration, i.e. .
An estimation of the parameters of the system based on experimental data has been obtained in [15]. The experimental data used were concerned with dormant murine B cell lymphomas [2,36]. The corresponding numerical simulations of the non-dimensionalized system with the estimated values for the parameters under discussion were able to reproduce several characteristics of a tumour in its dormant state. Figs. 2a, 3a and 4a show the initial conditions for the TICL, tumour cell and TICL–tumour cell densities, respectively. Fig. 2b–d shows the evolution of the (non-dimensionalized) spatial distribution of TICL density within the tissue at times corresponding to 700, 1000 and 1300 days, respectively. The time instances depicted in the figures show the formation of an unstable, heterogeneous spatial distribution of TICL density throughout the tissue. Fig. 3b–d shows the spatial distribution of tumour cell density within the tissue at times corresponding to 700, 1000 and 1300 days. The figures show a train of solitary-like waves invading the tissue and subsequently creating a spatially heterogeneous distribution of tumour cell density throughout. Fig. 4b–d shows time instances of the corresponding TICL–tumour cell complex distribution at 700, 1000 and 1300 days, respectively.
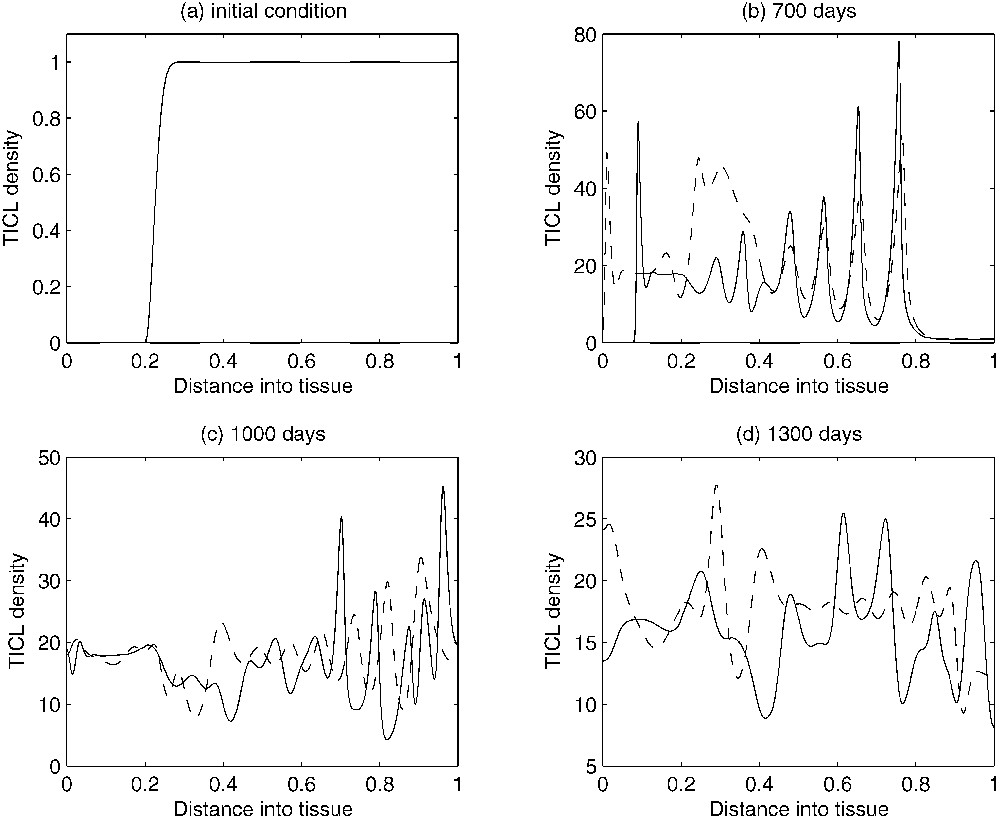
Figures showing plots of the TICL density over time. As time evolves, the complicated spatio-temporal dynamics can be observed. The solid-line curves show results when chemotaxis of the TICLs is incorporated into the system. The dashed line curves show results when there is no chemotaxis (i.e. χ=0), only random motility of TICLs.
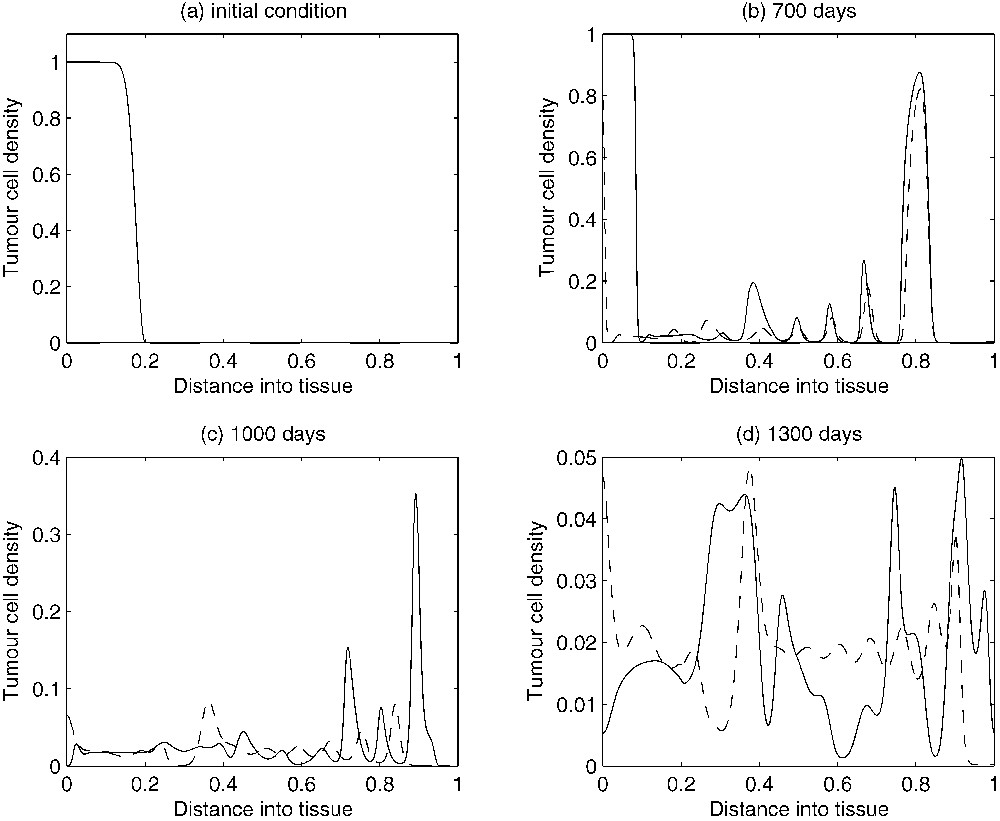
Figures showing plots of the tumour cell density over time. As time evolves, the complicated spatio-temporal dynamics can be observed. The solid-line curves show results when chemotaxis of the TICLs is incorporated into the system. The dashed line curves show results when there is no chemotaxis (i.e. χ=0), only random motility of TICLs.
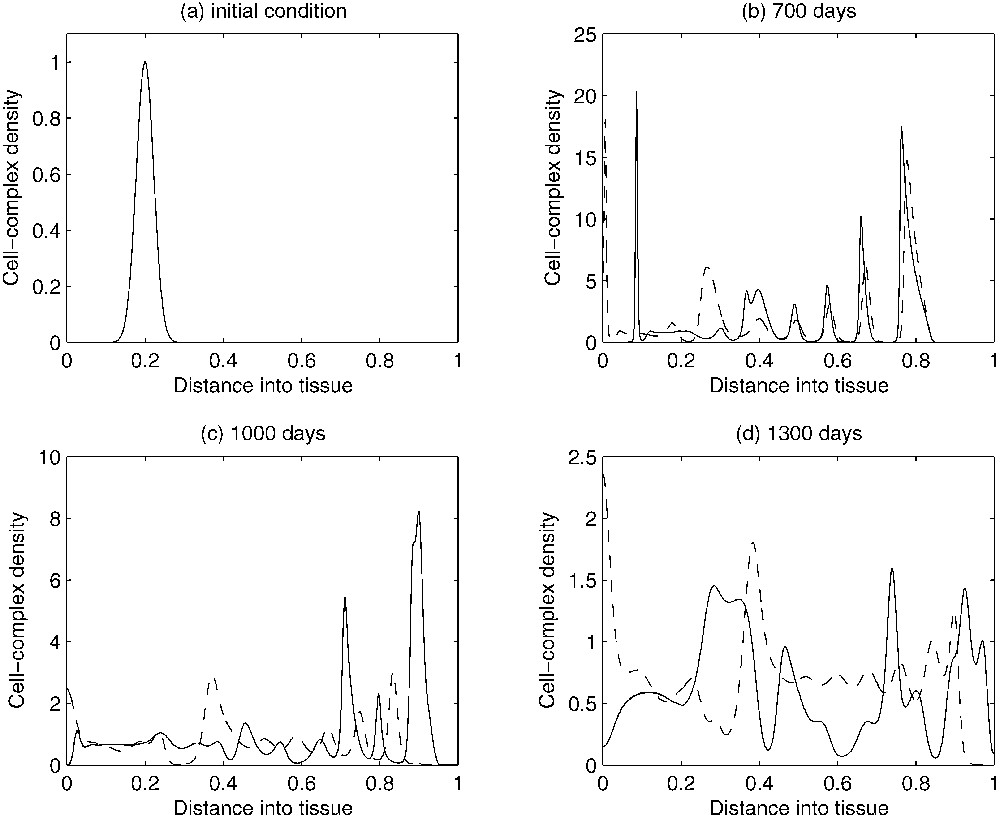
Figures showing plots of the TICL-tumour-cell complex density over time. As time evolves, the complicated spatio-temporal dynamics can be observed. The solid-line curves show results when chemotaxis of the TICLs is incorporated into the system. The dashed line curves show results when there is no chemotaxis (i.e. χ=0), only random motility of TICLs.
In addition to observing the above spatio-temporal distributions of each cell type within the tissue, the temporal dynamics of the overall populations of each cell type (i.e. total cell number) was examined. This was achieved by calculating the total number of each cell type within the whole tissue space using numerical quadrature. Fig. 5a shows the variation in the number of TICLs within the tissue over time (approximately 80 years, an estimated average lifespan). Initially, the total number of TICLs within the tissue increases and then subsequently oscillates around some stationary level (approximately cells). Long-time numerical calculations indicated that this behaviour will persist for all time. A similar scenario is observed for the tumour cell population. From Fig. 5b, we observe that initially, the tumour cell population decreases in number before subsequently oscillating around some stationary value (approximately 107 cells) for all time. Fig. 5c gives the corresponding temporal dynamics of the complexes.
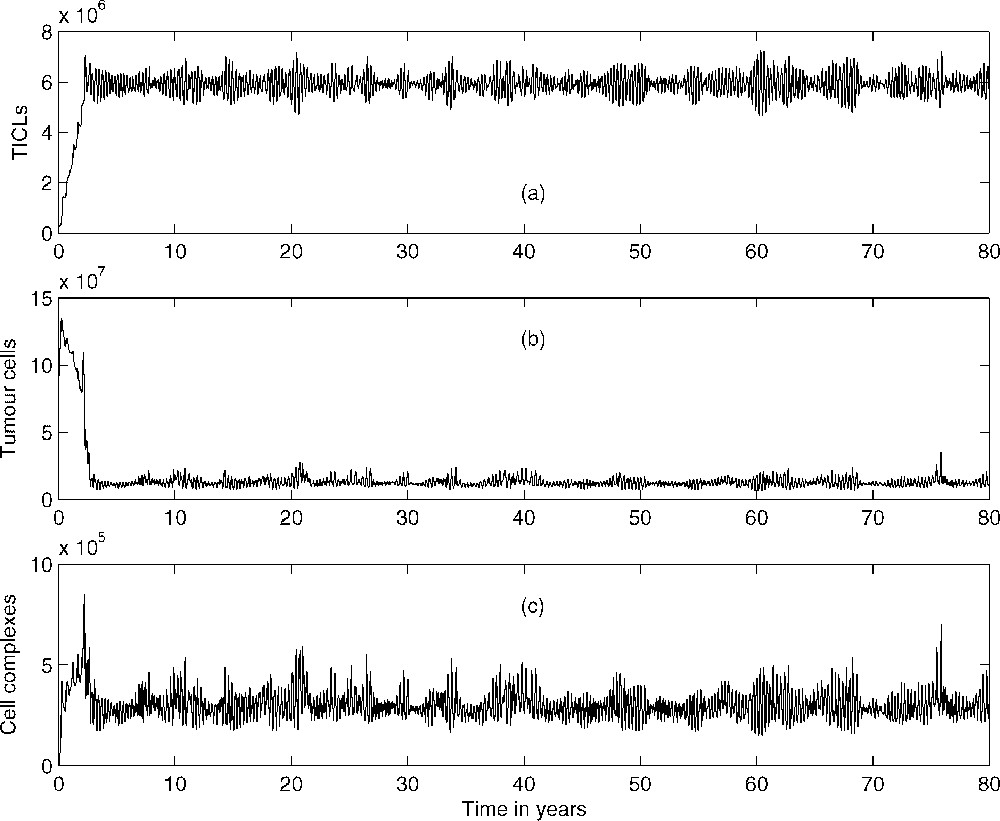
Total number of (a) lymphocytes, (b) tumour cells, and (c) tumour cell–TICL complexes within tissue over a period of 80 years.
In [15] we have been able to correlate numerically the irregular spatio-temporal evolution of the system (1)–(4) depicted in the above simulations with the existence of a stable limit cycle that emerged through a Hopf bifurcation in the spatially homogeneous ODE kinetics. Although the bifurcation analysis presented in [15] is out of the scopes of this article, we would like to correct a previous error by presenting a small (corrected) part of the relevant analysis. In particular, we focus on the bifurcations of the non-dimensionalized homogeneous ODE kinetics of Eqs. (1)–(4) with the Heaviside function omitted (i.e. ) and with respect to parameter , which is crucial in revealing the existence of the Hopf bifurcation under discussion. The bifurcation diagrams presented here have been generated with the version of the AUTO routine that is implemented within the XPP software package [37]. Fig. 6 shows part of the bifurcation diagram of TICL density E versus parameter . In the case of our system, AUTO was able to detect a (super-critical) Hopf bifurcation at . The solid dots represent the maximum and minimum values of the periodic solutions that emerge when lies in a particular interval. Most of the limit cycles that emerge through this bifurcation, including the one that is generated for (the parameter value that is associated with the irregular spatio-temporal simulations presented here), have been characterized by AUTO as stable. However there is a region around where unstable limit cycles exist. Fig. 7 shows a detailed view of this part of the bifurcation diagram. Here the solid dots represent stable limit cycle solutions, whereas the open circles represent unstable limit cycle solutions. As can be seen we have co-existence of stable and unstable limit cycles. Fig. 8, which has been generated with the MATLAB continuation toolbox MATCONT [38,39], reveals the structure of the projections of the limit cycles emerging at the co-existence region to the phase-plane. The existence of a fold or limit-point cycle (LPC) is evident.
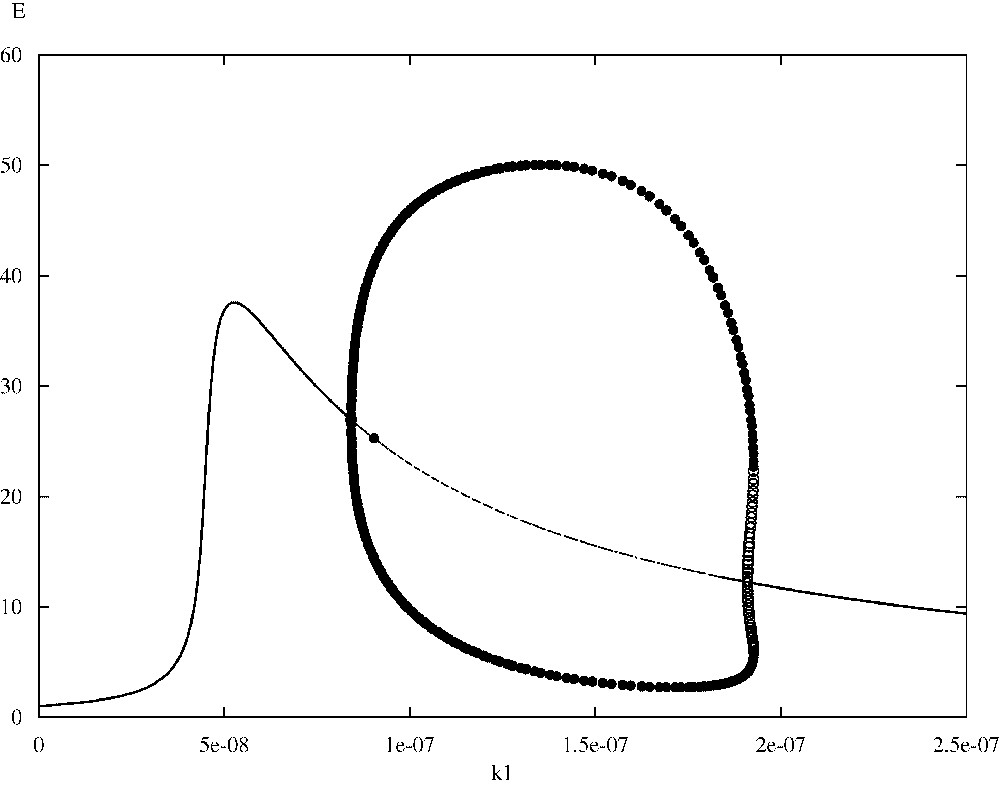
Bifurcation diagram of TICL density E versus the parameter .
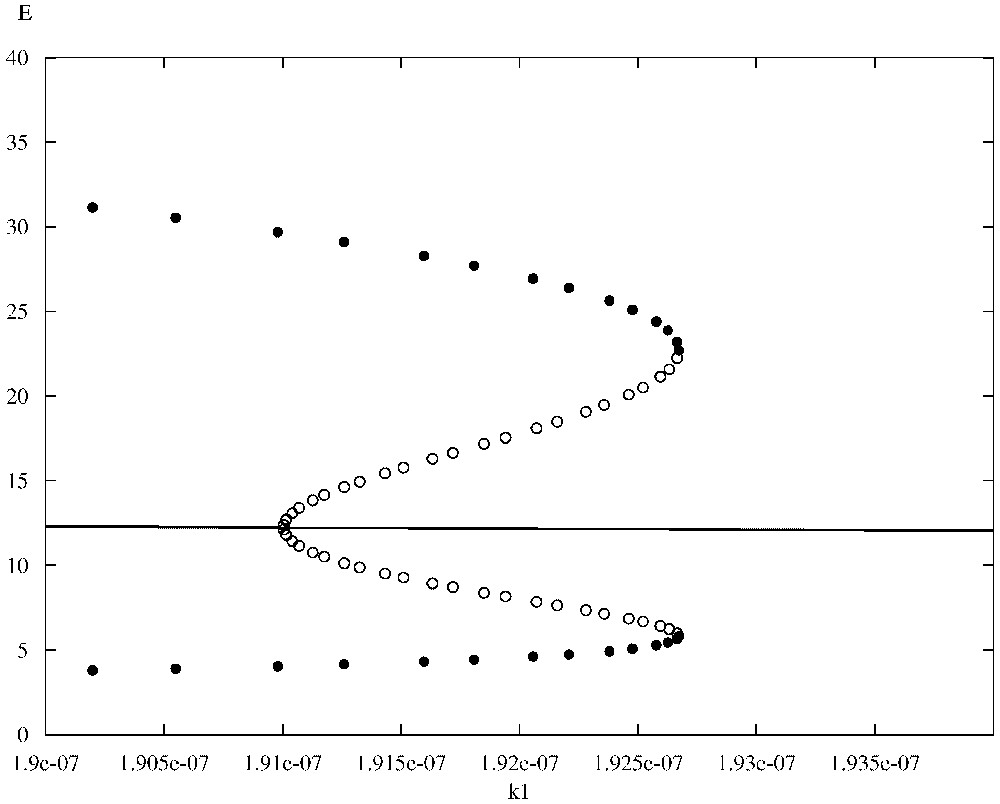
Detailed view of the co-existence region of Fig. 6.
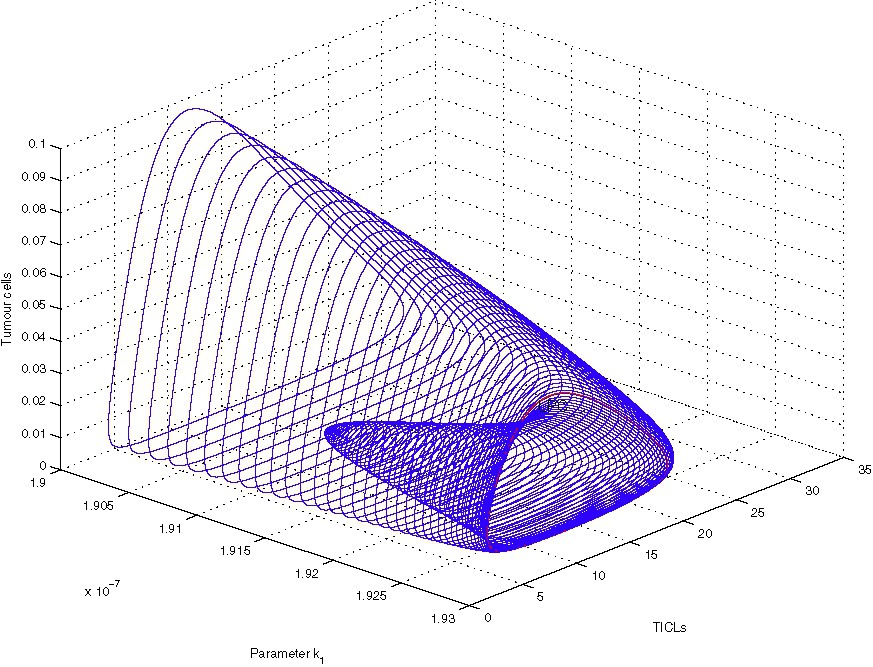
Limit-cycle-projection continuation in the co-existence region.
The above spatio-temporal simulations appear to indicate that eventually the tumour cells develop very small-amplitude oscillations about a ‘dormant’ state, indicating that the TICLs have successfully managed to keep the tumour under control. The numerical simulations demonstrate the existence of cell distributions that are quasi-stationary in time but unstable and heterogeneous in space. However, one would expect that by reducing the probability p of tumour cells being killed by lymphocytes, travelling-wave-like solutions of more canonical nature should emerge. Indeed, reducing the parameter p in the simulations results in the emergence of solutions of a composite type consisting of steady-state and travelling-wave components both in the full system and in the system without chemotaxis (i.e. the system of Eqs. (1), (3) and (4) with ). Figs. 9–11 show the evolution of these composite solutions from the initial conditions for the full system (with chemotaxis incorporated).
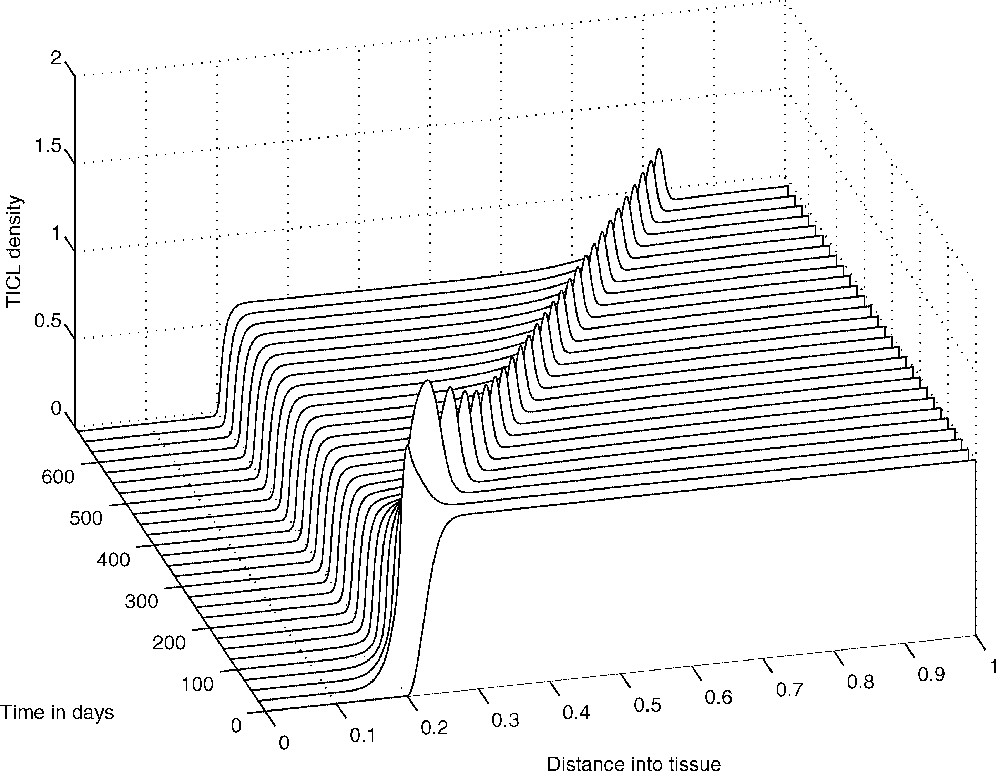
The evolution from the initial conditions of a ‘composite’ solution concerning TICL density E.
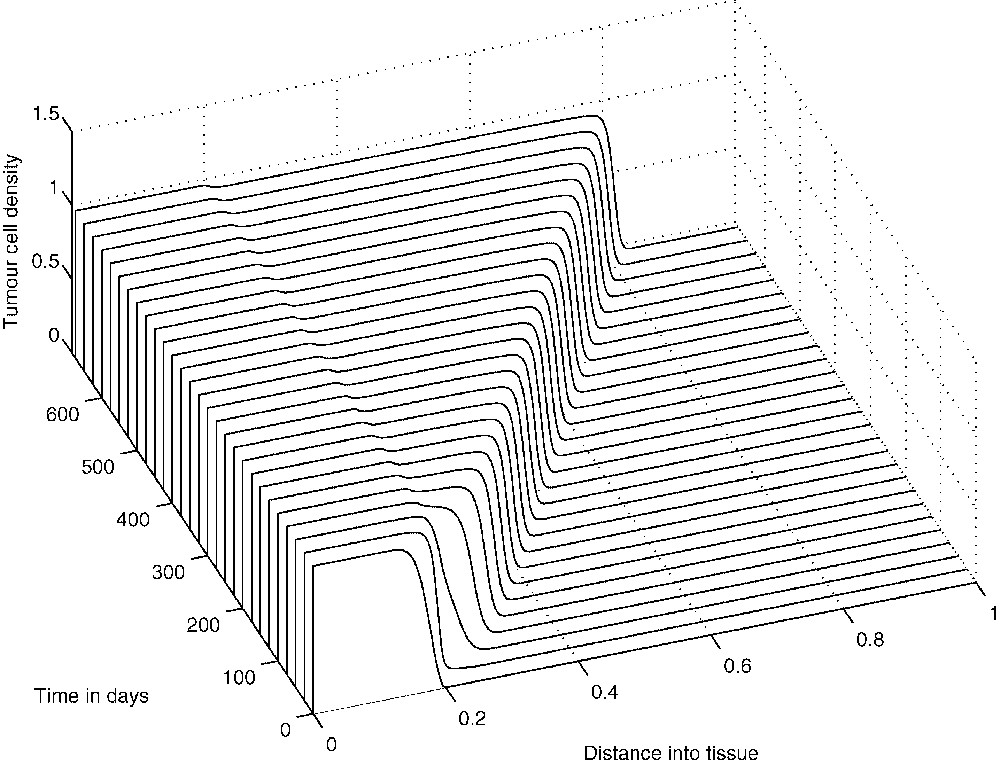
The evolution from the initial conditions of a ‘composite’ solution concerning tumour cell density T.
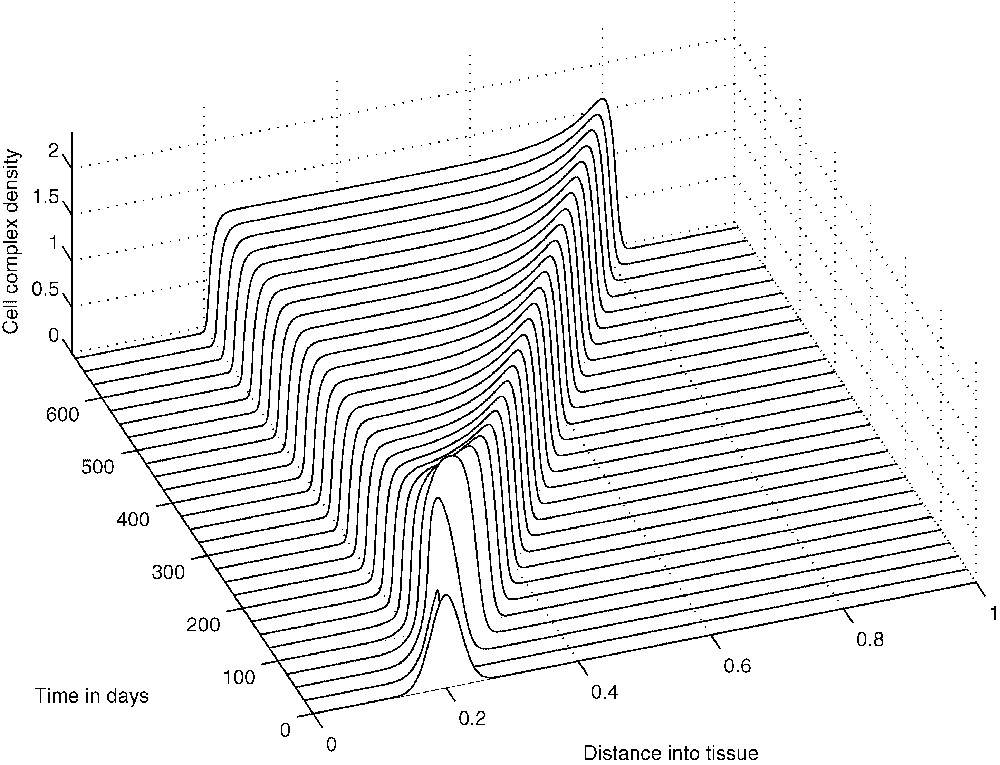
The evolution from the initial conditions of a ‘composite’ solution concerning cell-complex density C.
Our intention in this paper is to investigate the travelling-wave components of the composite solutions that arise for a particular range of values of parameter p (see also the bifurcation analysis undertaken in [15]). For the sake of mathematical simplicity we will not consider the effect of chemotaxis. That is to say we will investigate the solutions of the system of Eqs. (1), (3) and (4) with . We would like to note here that this is a reasonable simplifying assumption since, according to the numerical simulations that follow, the formation of the travelling-wave components is not affected by the chemotaxis term. Furthermore, we omit the Heaviside function, i.e. we set . We note that the Heaviside function is responsible for the formation of the steady-state components of the composite solutions (see also the relevant discussion in [15] concerning the effect that the Heaviside function has on the spatio-temporal simulations) and thus by omitting it we choose to focus on the travelling-wave components. Specifically then, we will focus on the following non-dimensionalized reaction–diffusion system:
(9) |
(10) |
(11) |
Non-dimensionalized parameter values
σ=41 200 | ρ=59 760 | η=0.0404 | μ=6.5×107 |
ɛ=31 128 000.01 | ω=1 | ||
ϕ=42 912.6214 | λ=15 892.19418 | ψ=3.12×107 |
The system of Eqs. (9), (10) and (11) has been solved numerically over the interval with zero-flux boundary conditions imposed and the initial conditions given by the non-dimensionalization of Eqs. (7). Figs. 12–14 show the results of the numerical simulations, which clearly depict the evolution of standard travelling waves from the initial conditions. We note that these travelling waves – and the corresponding composite solutions of the full system – are of great biological importance because, when they exist, the tumour invades the healthy tissue at its full potential. In the next section we undertake a travelling-wave analysis of system (9)–(11).
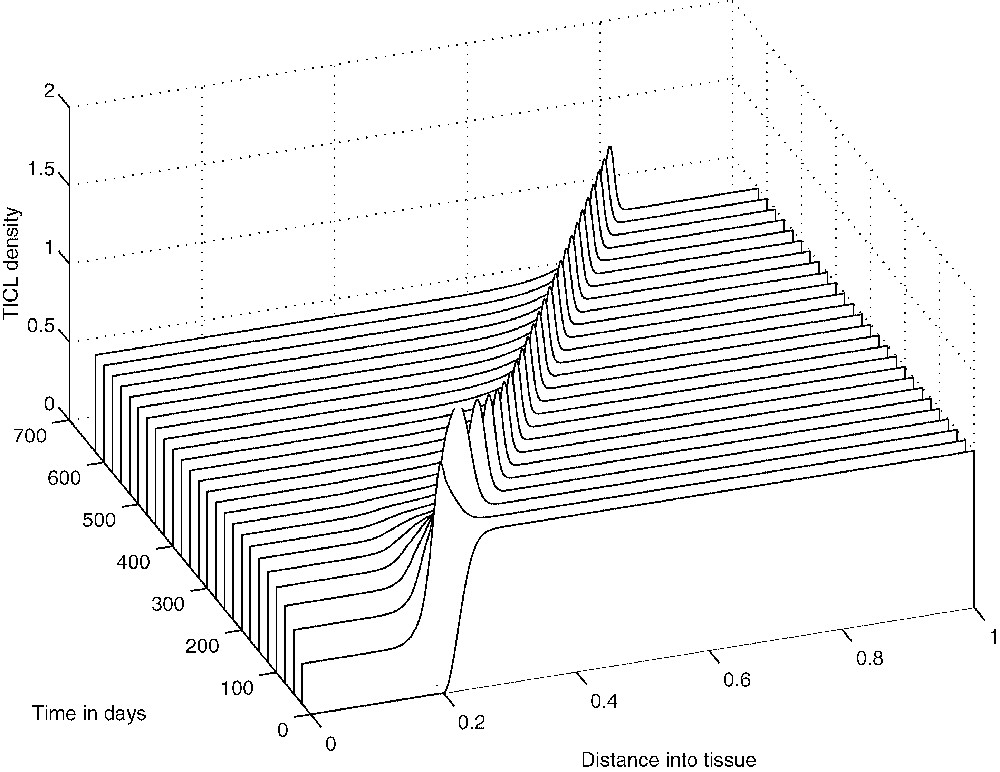
The evolution from the initial conditions of the travelling wave of effector cell density.
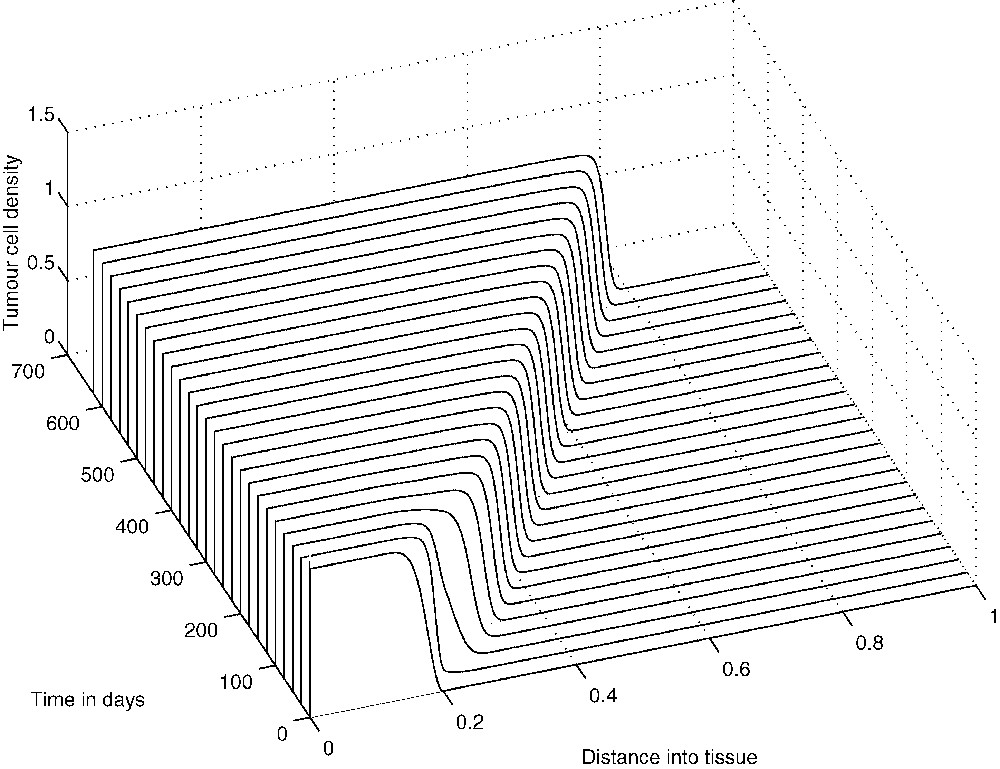
The evolution from the initial conditions of the ‘invasive’ travelling wave of tumour-cell density.
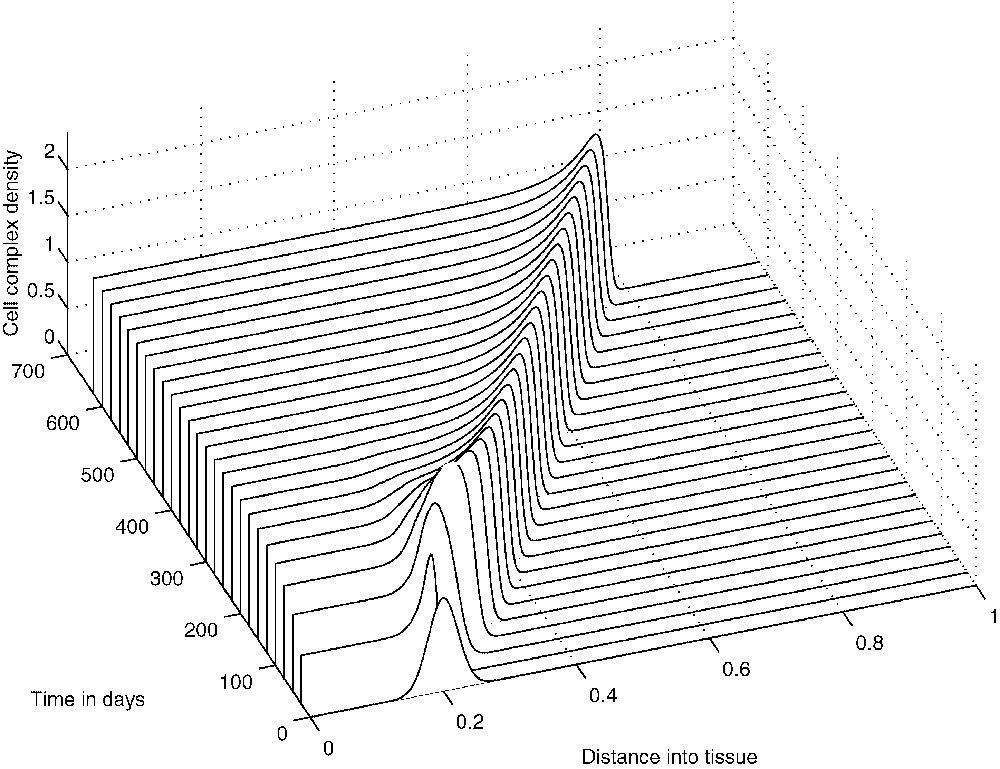
The evolution from the initial conditions of the travelling wave of cell-complex density.
3 Travelling-wave analysis
The numerical simulations of the previous section indicate that the system of Eqs. (9)–(11) exhibits travelling wave solutions for some choice of parameters. Two of the main approaches for establishing travelling-wave solutions for systems of PDEs are: (a) the geometric treatment of an appropriate phase-space, where one essentially is interested in intersections between unstable and stable manifolds and (b) the Leray–Schauder (degree-theoretic) method, which employs homotopy techniques (see e.g. [40,41]). From a numerical analysis point of view, the former approach is used either in conjunction with a shooting method over a truncated domain or by trying to identify a ‘trivial’ heteroclinic connection for some choice of parameters and then follow its deformation as the parameters are changing using numerical continuation.
In all cases the main purpose is to establish the existence of a travelling-wave solution without any available information concerning its nature. Our approach, however, is going to be ‘computer-assisted’ in the sense that we are going to make use of the information that the numerics of the previous section can provide us.
Since we are interested in waves travelling from the left part of the domain to the right, we specify a travelling coordinate , where and we let:
(12) |
(13) |
(14) |
(15) |
(16) |
Since the wave velocity c is unknown, system (15) can be regarded as a nonlinear eigenvalue problem. Several analytical methods have been developed for estimating c in this framework. However, the numerical solutions of Eqs. (9)–(11) readily yield a value of . In the analysis that follows, we therefore use this numerical estimate for c to fix the wave speed at the constant (non-dimensional) value of 850 and hence take c as a fixed parameter.
The steady states of system (15) can be found by solving the (nonlinear) equation . Several numerical optimization methods can be employed for this task. However, for the purposes of the travelling-wave analysis, the numerical simulations of the previous section indicate that we should identify a heteroclinic connection between and , where:
(17) |
We are interested in the existence of an orbit of (15) that satisfies:
(18) |
(19) |
(20) |
The values of the parameters of the system under discussion suggest that an approximation of the connecting orbit by perturbing the system of Eqs. (12)–(14) can be feasible. In particular, we perturb Eqs. (12) and (13) by ignoring the effect of the second derivatives on the system (see also [44]). That is to say, we consider the perturbed system:
(21) |
(22) |
(23) |
Let and be the projections of and onto the phase-space defined by Eqs. (21)–(23). It is obvious that and are steady states of the perturbed system. There is a three-dimensional unstable manifold associated with and a one-dimensional stable manifold associated with . We have used XPP (see [37]) to investigate numerically the phase-space of the system of Eqs. (21)–(23). XPP provides the implementation of numerical algorithms for tracking one-dimensional invariant manifolds and in the case of it was able to confirm that defines a heteroclinic connection between and . Figs. 15–17 show approximations to the connecting orbit defined by in the , and planes, respectively. These compare very well with the results of the spatio-temporal simulations of the full PDE system.
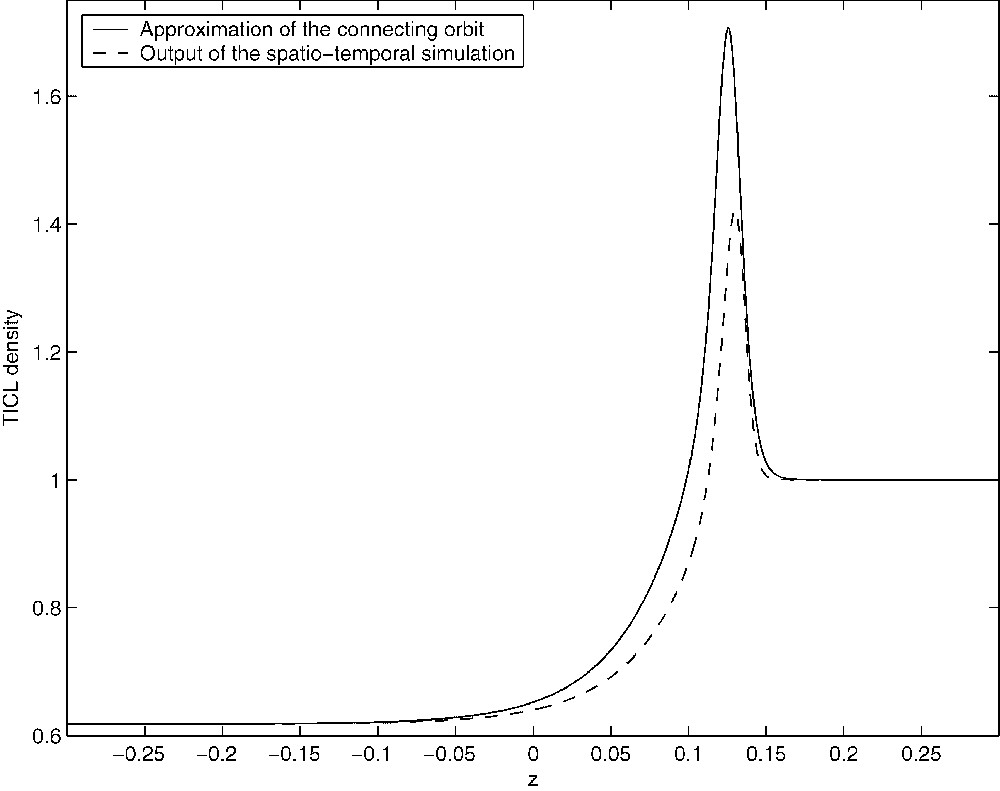
Figure showing the approximation of the connecting orbit in the (E,z)-plane from the travelling-wave analysis (solid line). The orbit was computed over the truncated domain [−0.3,0.3].
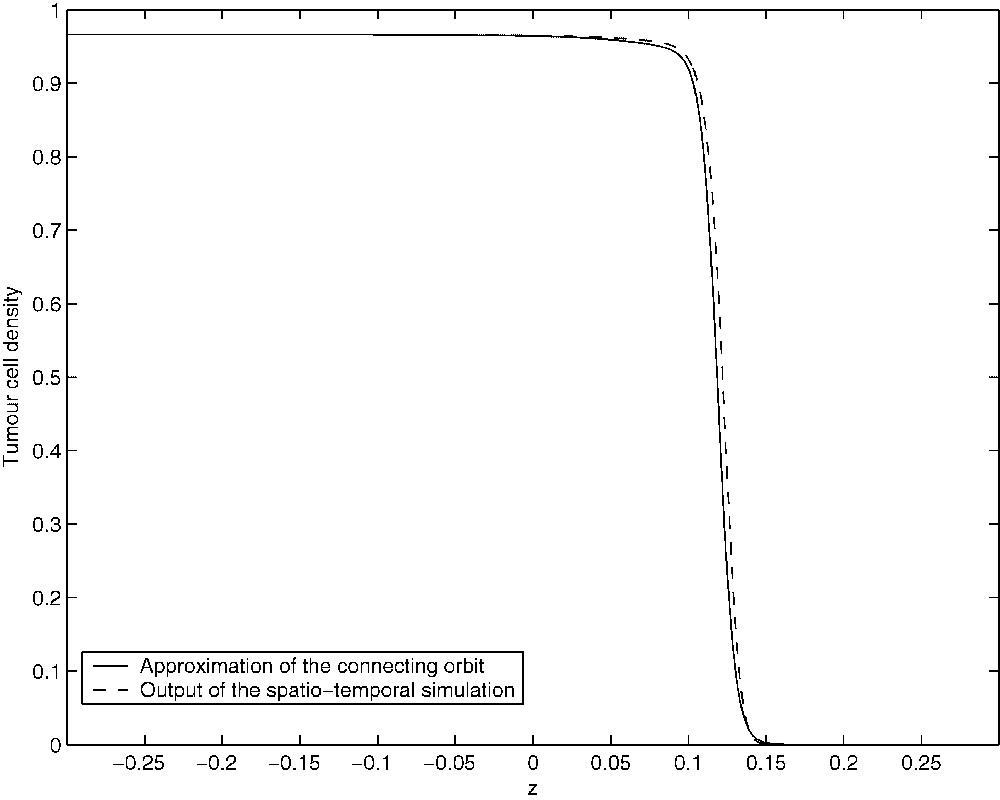
Figure showing the approximation of the connecting orbit in the (T,z)-plane (solid line). The orbit was computed over the truncated domain [−0.3,0.3].

Figure showing the approximation of the connecting orbit in the (C,z)-plane (solid line). The orbit was computed over the truncated domain [−0.3,0.3].
4 Discussion
In this paper we have undertaken a travelling-wave analysis of a mathematical model developed in [15], describing the growth of solid tumours in the presence of an immune system response. For a particular choice of parameters, the model is able to simulate the phenomenon of cancer dormancy; a clinical condition that has been observed in breast cancers, neuroblastomas, melanomas, osteogenic sarcomas, and in several types of lymphomas. The behaviour of the cancer dormancy simulations can be described as highly irregular, depicting unstable and heterogeneous tumour cell distributions that are nonetheless characterized by a relatively low total number of tumour cells. This behaviour is consistent with several immunomorphological investigations with tumour spheroids infiltrated by TICLs.
However, the alteration of certain parameters of the model is enough to induce bifurcations into the system, which in turn result in the evolution of travelling-wave-like solutions in the numerical simulations. These travelling waves are of great importance because, when they exist, the tumour invades the healthy tissue at its full potential. The existence of these travelling waves for a particular choice of parameters was established in this paper for a reduced system, which nonetheless captures the essential elements of the full model presented in [15].
We would like to note here that the first order approximation employed in deriving Eqs. (21)–(23) could be further investigated in the context of geometric perturbation theory. More precisely, we believe that Fenichel's invariant manifold theorem [42,45] has a special role to play here and, as a matter of fact, it seems that one could try to analyse system (15) by employing the relevant techniques discussed in [42]. Nonetheless, several problems arise in this direction with perhaps the most prominent of them being the unavailability of simple analytic expressions for the steady states of the system under discussion.
The work presented here complements the bifurcation analysis undertaken in [15] and seems to provide an adequate framework for studying and identifying critical parameters of the process in which cancer cells are present in a tissue but do not clinically occur for a long period of time, but can begin to grow progressively at a later date. Thus our modelling and analysis offers the potential for quantitative analysis of mechanisms of tumour-cell–host-cell interactions and for the optimisation of tumour immunotherapy and genetically engineered anti-tumour vaccines.
Acknowledgments
We would like to thank Dr. Fordyce Davidson for the helpful and stimulating discussions. Both authors acknowledge support from the EU Network Grant HPRN-CT-2000-00105 ‘Using mathematical modelling and computer simulation to improve cancer therapy’.
1 See also the discussion on p. 120 of [43] concerning the so-called transversality theorem.