1 Introduction
According to recent definitions [1], self-organization is a basic emergent behaviour. Plants and animals assemble and regulate themselves independent of any hierarchy for planning or management.
Emergence describes the way unpredictable patterns arise from innumerable interactions between independent parts.
Let us quote some historical main publications about this topic:
- – feedback loops described in Cybernetics [2,3] which give one of the first models of the living beings' organization. Wiener himself [4] makes the parallelism between the cerebellar tremor and an unadjusted retroactive loop;
- – the works [5,6] studying the entropy of self-organizing systems. Prigogine defined dissipative structures systems which continuously export entropy in order to maintain their organization [7];
- – modelling the organization of living systems by dynamical systems. René Thom proposes catastrophe theory [8] as a model of embryological differentiation. This model uses continuous differentiable dynamical systems;
- – automata theory that allows us to very easily represent interactions between parts of systems. Von Neumann self-reproducing automata [9] is the main example of machines building themselves. Stuart Kaufman models genetic regulatory networks with networks of combinatorial Boolean automata [10,11];
- – Von Foerster and later Henri Atlan [12] try to explain the self-organizing properties of the living beings by the paradigm of the complexity by the noise;
- – Maturana, Varela [13] and Zeleny [14] develop the concepts of the closure property of all the autonomous systems and autopoiesis.
Yet Ashby [15] argues that self-organization cannot be the result of a function because this function ought to be the result of self-organization and so forth.
On the other hand, computer science proposes to this date a lot of models to design adaptive systems, but all these models depend on the comparison between a goal and the results and ingenious choices of parameters by programmers, whereas there are no programmer's intention nor choice in the living systems. Let us mention some examples:
- – for the simulated annealing model, choice of parameters: initial temperature, how many iterations are performed and how much the temperature is decremented at each step [16–18];
- – in neural networks, back propagation of the difference between the target value and the output is used for learning the target [19,20];
- – in evolutionary programming, each individual in the population receives a measure of its fitness to the environment [21,22];
- – the adaptive filter, using the least mean-square algorithm, which is the most widely used adaptive filtering algorithm, measures the difference between the output of the adaptive filter and the output of the unknown system [23,24].
One cannot simulate with all these previous models the adaptive properties of the nervous system and the immune system such as those found in the following examples:
- (1) if we cut the opposite muscles of a monkey and re-attach them in a crossed position of one eyeball [25] or of the limb [26], after some weeks, the eyeballs again move together and the movements of the limb are co-ordinated;
- (2) in an experiment of rats switching between two different environments (morph square and morph circle), it was shown that neurons states got stabilized into two different configurations [27];
- (3) B cells can distinguish the change of a single amino acid in the epitope of an antigen [28] and elaborate new antibodies directed against the new epitope.
Nervous system and immune system are different [29]. The immune system comprises different types of cells and there are no equivalents of the Hodgkin, Huxley equations giving a model of the neuron [30,31].
Yet these systems have in common the property to be able to rewrite their organization whenever the external conditions vary.
All present theories of synaptic learning refer to the general rule introduced by the Canadian psychologist Donald Hebb in 1949 [32]. He postulated that repeated activation of one neuron [33] by another, across a particular synapse, increases its strength.
B cells make specific immuno globulins during their differentiation by recombination, splicing and removal of V, D and J gene segments [34].
These systems having ‘the rewriting property’ operate as self-organizing machines, which self-organize by modifying their internal functions, i.e. self-programming machines.
This problem was raised in genetics: “Molecular biology itself must acknowledge that there is a fundamental difficulty, since it is obliged to admit that the famous ‘genetic program’ is a ‘program which programs itself’ or a program which needs the result of its reading and execution so as to be read and executed” [35].
So through this very fast review of literature, we see that there are fundamental difference between the classical model approach and self-programming machines. Table 1 summarizes these differences.
Differences between the classical model approach and self-programming machines
Model | Self-programming machines | |
Implementation | Is a fixed function solution of differential system or a transition function of automata network | Is an organization modified by environmental data |
Properties of the organization | Distribution of transient and cycles length. Diameter of attraction basins | Only one trajectory can be studied since the trajectory modifies the organization as it goes. Fixed points are pervasive |
Properties of the cycle | Each point of a cycle is gone through only once | The self-programming machine can go through a point more than once during a cycle (in case of n-cycle) since the internal function will be different on the next occurrence |
Probability to stabilize into a fixed point when the input set increases | Less probable [36] | Very probable |
We will define, in this paper, an Adaptive System as a system that changes its behaviour in response to its environment, by varying its own parameters or even, in our case, its own structure: it thus retains information about the environment in a form that can influence its future behaviour [37]. However, an Adaptive System does not have a stated or implicit goal or objective, and thus does not aim at improving its performance. Yet, adaptation results in a seemingly goal-directed behaviour, if the environment repeats patterns of inputs.
We will show that the mechanism proposed here and the adaptive systems of living beings share similar properties:
- – all functions that compare results to a goal (feed-back, gradient, fitness to the environment, etc.) and all parameters choices are not to be allowed;
- – initial states and functions are randomly chosen at the beginning and once and for all;
- – ability to stabilize into a fixed point if the environment is fixed, this stabilization being more probable when the input set size increases or the machines are interconnected;
- – ability to find another stabilization (ultra stability) [38] if the conditions vary, in case of small perturbations or intentional massive breakdowns;
- – ability to run in parallel, each machine having its local clocks, not studied in this paper.
2 Self-programming machines. Description and functioning
We define here self-programming machine formalism and show the properties of their dynamics.
2.1 Components
Let be given:
- – , with p prime,
- – , the set of polynomials which map into itself, ,
- – ,
- – , a set of functions that map into ,
- – an index .
2.2 Definition and functioning
A self-programming machine (or msp) is a sextuplet where:Definition
Functioning: Msp performs the following operations (Fig. 1):
(1) |
(2) |
(3) |
(4) |
(5) |
(6) |

The equalities (5) and (6) define the transition function:
(7) |

From (5) and (6) and (8), we obtain (Fig. 2):
(8) |
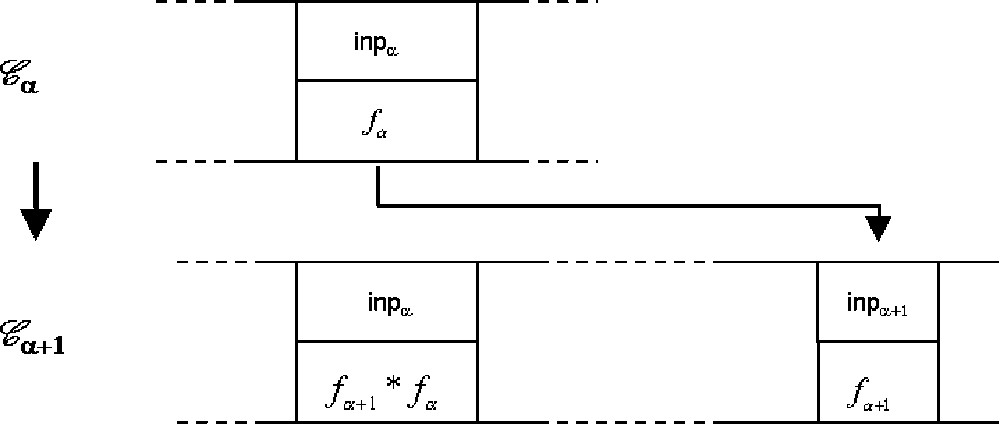
2.3 Initialization and trajectory
Initial conditions of a machine msp () are given by initial inputs and initial function :
and are chosen at random at the beginning and once for all from two laws of probability defined respectively by its sample spaces for and for and its uniform distributions:Initialization
The trajectory of msp corresponds to successive points the machine goes through, starts at and will be denoted:Trajectory
A self-programming machine is a deterministic device, and the cardinality of state set is finite. Therefore such a machine necessarily converges.
Theorem 1
A msp converges whenever a point of its trajectory is identical to a previous one . It then indefinitely goes through a limit cycle or n-cycle composed of the n points:Definitions
Thus dynamics of msp is characterized by the transient length τ and the length n of the limit cycle (i.e. its period).
2.4 Connection to external processes g and h: Machine msp1 and msp2
In order to show the msp adaptive properties:
(1) the msp is connected (Fig. 3) to a combinatorial automaton defined by some external function . Let us call this machine msp1.
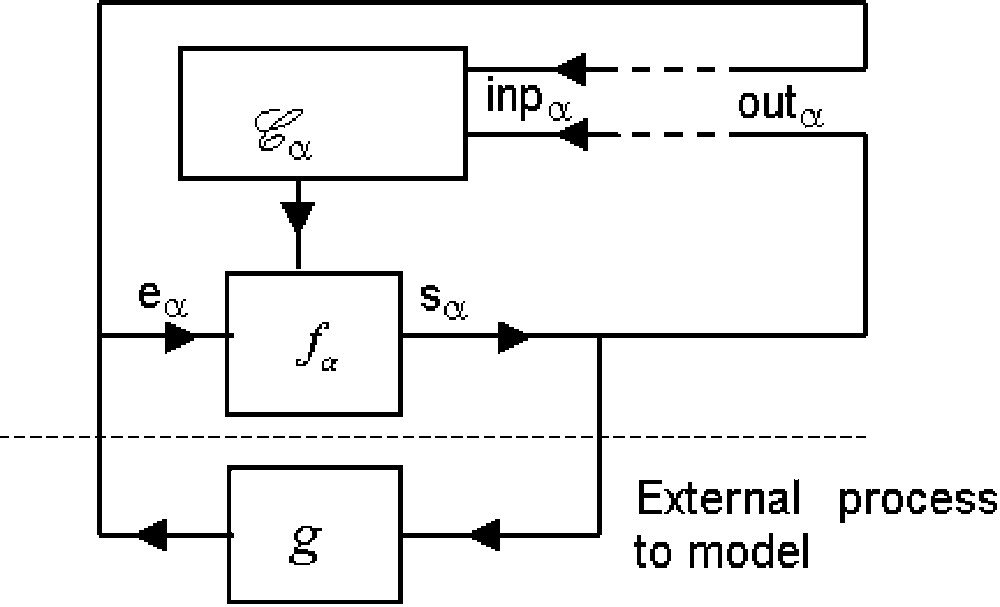
1. The equality (3) defining the msp is replaced by for the msp1.
Let us study under which conditions a msp that we call m′spis identical to msp1, i.e. conditions under which a msp1 is a self-programming machine msp.
The equality (2) defining msp is replaced by for the m′sp.
The equality (6) by .
The equality (8) by .
Operation ‘○’ is left distributive with ‘∗’, so we can write:
(9) |
In this case:
m
′
sp
and
m
sp1
are identical and hence have the same trajectory.
Theorem 2
2. Msp1 is connected (Fig. 4) to another combinatorial automaton defined by any external function . Let us call this machine msp2.
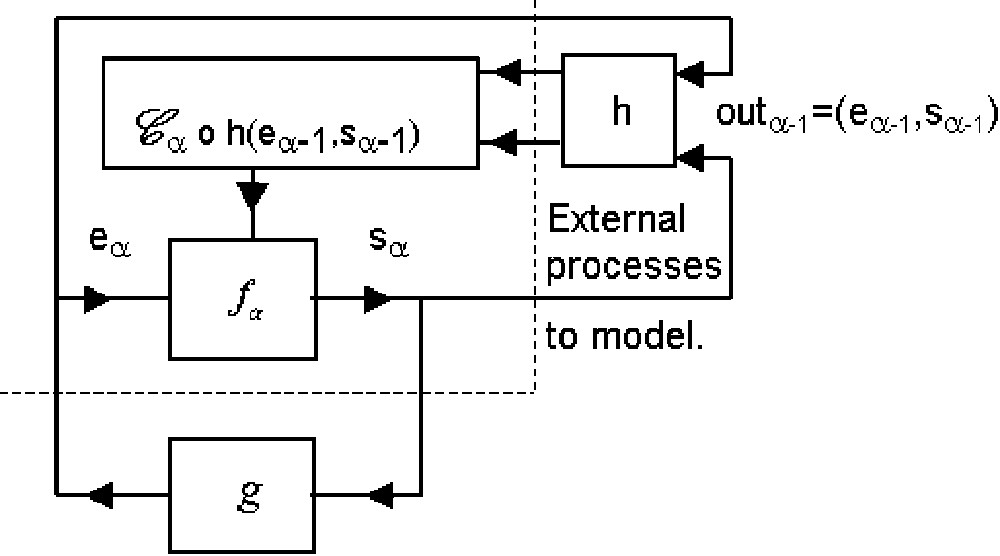
The equality (4) defining the msp is replaced by for the msp2.
Let us study under which conditions a msp1 that we call m″sp identical to msp2:
Then the equality (2): defining msp1 is replaced by for the machine m″sp, the equality (6): is replaced by , and the equality (9) is replaced by .
The function h is injective. In this case: m″spandmsp2are identical and hence have the same trajectory.Theorem 3
From now on, we will only study msp2 and we call msp this machine.
3 Cyclic groups
Let us suppose a msp is going through a n-cycle points of which are: , .
Cyclic group of the states
From the equality (7), we can write , , , therefore ∃s such as
Cyclic group of the n-cycles
The function g being fixed at the beginning, one point of the cycle is only defined by the function . If we have a s-cycle for , we obtain an n-cycle for with (hence ).
It therefore depends uniquely on the membership of to the equivalence class of polynomials having the same image for the value . Therefore the order n of is a divisor of s.
4 Conditions of n-cycles
4.1 Linear operator
In the case of a linear operator ∗, equality (9) becomes: . For the sake of simplicity, we will simplify this to , which not does influence the distribution of n-cycles or transient length.Definition
Let m be the number () of different input values of the n-cycle. The matrix corresponding to transition from state to state is the matrix:
(10) |
(11) |
Equality (11) implies that the conditions for a msp to go through a n-cycle, defined as some vector , is that this vector is an eigenvector associated to eigenvalue 1 of .
Inversely, if we have a product of n () matrices where has eigenvalue 1 and are matrices such as in the left-hand side of Eq. (10), then we can find a linear msp such as these matrices are transition matrices of msp and mspc goes through a n-cycle corresponding to the 1-eigenvector and to the n transition matrices .
A
m
sp
with linear operator goes through a n-cycle
,
if 1 is an eigenvalue of
and
its associated eigenvector.
Theorem 4
4.2 Polynomial operator
In preliminary unpublished work [39], we have studied the case: , where ○ is the composition of functions, thus corresponding to the case where . We found that, in this case, trajectories always ended in fixed points. We have introduced before the linear case, we now introduce a more general polynomial case.
A polynomial operator ∗ is defined, for some polynomial , by:Definition
(12) (13) (14)
We do not have theoretical results in that case and can only give simulations results for such msp: all theoretical results presented in the following Sections 5 and 6 only apply to linear machines. Yet, simulations show very similar results for both linear and polynomial operators (see results in §6.1).
5 Association of machines
5.1 Association with a combinatorial automaton
5.1.1 Definition
A combinatorial automaton defined by a function is modified by a msp (Fig. 5).
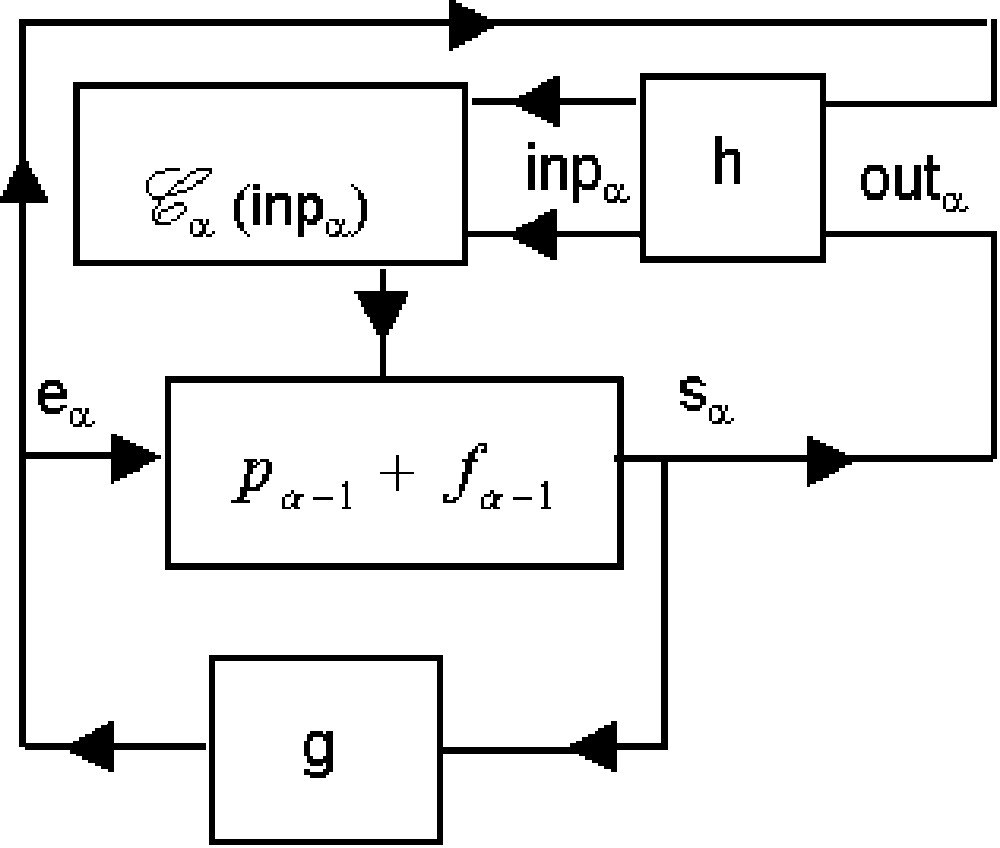
The functioning of this machine (mspc) differs from a msp uniquely by the equality (3) replaced by with .
In this paper, we consider for simplicity that the functions h and g are identity functions (which implies and ) and the operation ‘∗’ is linear. Apparently, in general case, we cannot obtain theoretical results.
5.1.2 Conditions of n-cycle
A
m
spc
goes through a n-cycle if
Theorem 5
Let us suppose this machine goes through a n-cycle, i.e. .
We have:
(15) |
Let P be the matrix defined by the equality (11), let be the line vector such as is the line vector equal to the ith line of P, let V the column vector elements of which are , from the equality (15):
(16) |
These n equalities give the following homogenous system:
(17) |
Repeating the same reasoning for , the determinant of the system is equal to and we obtain
(18) |
(19) |
In addition,
(20) |
5.1.3 Distribution of τ and n
Let be given identical machines mspc differing uniquely by their initial conditions.
If , , a sequence with elements chosen at random can be a trajectory of some mspc if all the values are different: and being given, we have only to choose a function that satisfies the equality . We make the same reasoning for the following points of the sequence.Remark
But, if two points in the sequence are equal (for example, and ), if the value is such that , the sequence cannot be the trajectory of a mspc.
The probability that an arbitrary sequence has all its values different is:
.
Lemma
The following matrix given in Fig. 6 establishes a one-to-one correspondence between the coefficients of polynomial and the values of this polynomial.

The determinant of this matrix is a Vandermonde determinant equal to and different from 0 since p is prime.
The number of functions is , each function has p coefficients, the number of coefficients of the functions of is and , coefficients are equal to x.
The fact that coefficients are equal to x and the correspondence one to one between the coefficients of polynomials and the values of these polynomials imply that it exists pairs such as .
Therefore, if the random variable and have respectively its sample space equal to and , and have uniform distributions:
In the following, we try to find a way to calculate the probability of the event that a mspc goes through a n-cycle. For that, we should work on the space of all the possible trajectories of mspc differing by its initial conditions, but it is not a finite space. To get rid of this problem, for fixed n and , we consider just the set of all the n-sequence of elements which is a finite set. In this space, we are looking for the n-cycles. I mean to find a mspc that goes through these n-elements like a n-cycle. From now on, for n fixed and , as shows our remark, we consider that all elements of are on a trajectory of a mspc which allows us to give the following results:
Let us consider the sequence which elements are chosen at random . This sequence corresponds to a n-cycle of mspc if the three events (18), (19) and (20) occur:
We have demonstrated that [35]:
The probability that a point in the trajectory of this machine belongs to a n-cycle is the probability that a point belongs to a cycle is:
Now, let us evaluate the probability for a point to be a transient point.
Under the assumption that the point is in the trajectory of some machine, then obviously:Theorem 6
and the probability amspcconverges after τ transient points (the th point is on a cycle) is .
τ is a geometric random variable that has expected value and variance
The fast convergence of mspc explains the concordance between the computations and the simulations (Tables 3 and 4).
Mean and variance of τ for different values of |Input|
Expected value of τ: | Variance of τ: | |||||
Simulations (linear) | Theoretical value | Simulations (polynomial) | Simulations (linear) | Theoretical value | Simulations (polynomial) | |
9 | 9.5 | 9 | 8.2 | 68.8 | 72 | 57.5 |
25 | 26.6 | 25 | 32.9 | 621.3 | 600 | 183.3 |
49 | 51.8 | 49 | 60.7 | 2467.6 | 2352 | 1214.72 |
121 | 125.2 | 121 | 132.4 | 14 974.1 | 14 520 | 5528.4 |
Results of simulations and comparison to the theoretical result for ,
T | |Input|=9 | |Input|=25 | |Input|=49 | |Input|=121 |
Nb of 1-cycles | 31,310 | 31,809 | 31,916 | 31,977 |
Nb of 2-cycles | 686 | 191 | 84 | 23 |
Nb of 3-cycles | 4 | 0 | 0 | 0 |
Frequency of n-cycle n>1 | 2.15% | 0.59% | 0.26% | 0.07% |
(simulation) | 45.6 | 166.5 | 379.9 | 1390.3 |
(theoretical value) | 40.5 | 156.2 | 400.1 | 1464.1 |
Because of the remark in §5.1.3, notice that for m small enough so that we can find different points, these points together with one mth point will be on a trajectory of some mspc machine. Our assumption is thus very light.
5.2 Association with another
Fig. 7a and b shows the two ways of binding together two .

In this paper, we focus on a sequential updating scheme: machines change states one at a time. Other updating regimes (parallel, asynchronous) could also be studied but will not be reported here.
The method to demonstrate the equivalence between one msp and a network of n msp consists in replacing each term defining one msp by a n-tuplet.
For example, in the case of Fig. 7a (Fig. 7b would be similar), equations can be rewritten as:
(21) |
(22) |
(23) |
(24) |
(25) |
6 Results
We now present the results of simulations we have run for machines, linear and polynomial. Each result is obtained by 32 000 simulations of the same machine with different initial conditions chosen at random. . For each value of p, the machine is chosen at random, i.e. parameter λ for the linear case and polynomial are chosen at random.
6.1 with operation ‘∗’ linear and polynomial
The similarity between msp with operations ‘∗’ linear and polynomial is striking: we show in Table 2 results of simulations, both for the linear and polynomial cases, indicating that both linear and polynomial cases seem to be in agreement with the theoretical value.
Results of simulations for linear and polynomial cases
‘∗’ linear | ‘∗’ polynomial | |||
% n-cycle | Expected value of τ | % n-cycle | Expected value of τ | |
9 | 3.28 | 8.28 | 3.01 | 11.82 |
25 | 2.63 | 85.50 | 1.84 | 32.92 |
49 | 0.55 | 188.99 | 0.21 | 149.46 |
121 | 0.052 | 299.98 | 0.056 | 302.34 |
6.2 associated with a combinatorial automaton
6.2.1 Transient length
Each figure (Figs. 8–11) compares simulations (points) and computation (theoretical value) from (smooth curve) for a given value of and for 32 000 simulations, τ is on the X axis, the number of initial conditions on the Y axis.
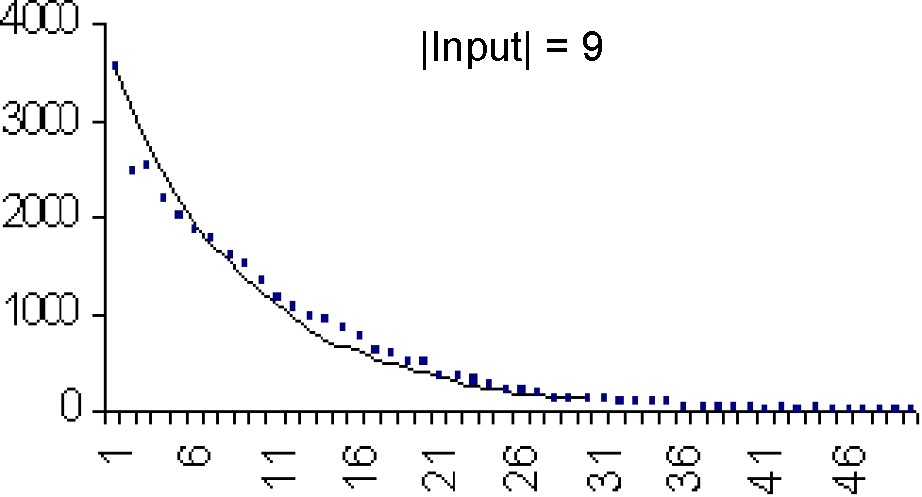
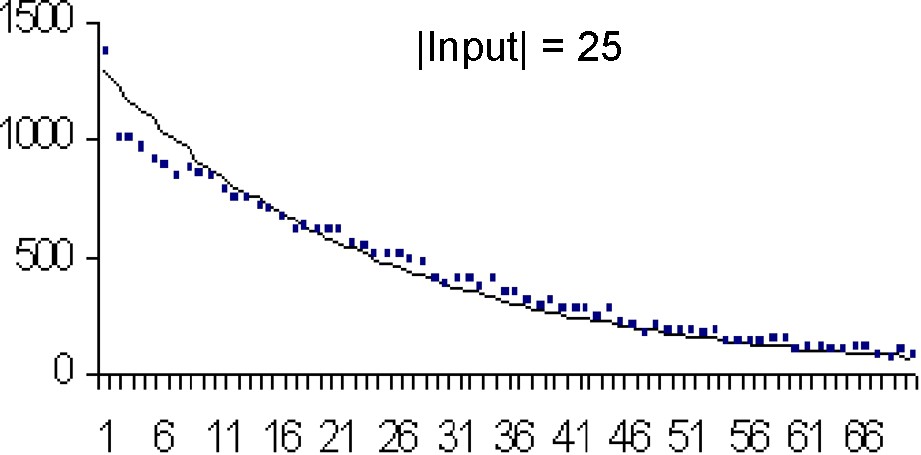

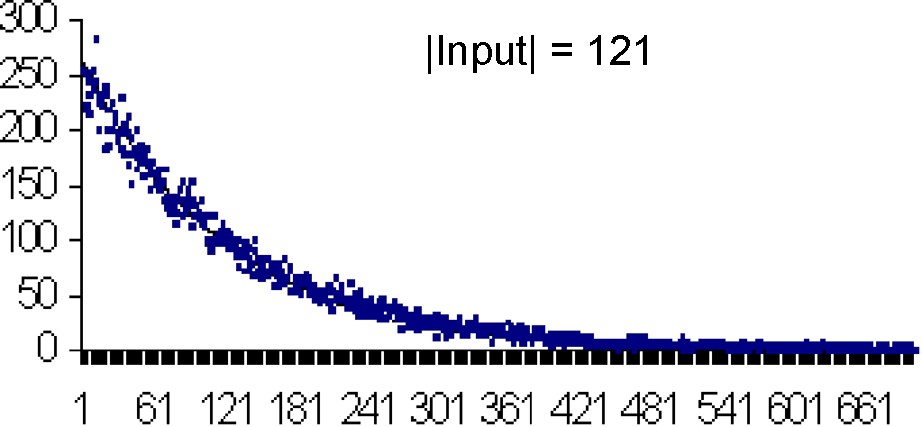
In Table 3, we compare the mean and variance of τ for different values of :
6.2.2 Frequency of cycles of length n
Table 4 shows the results of simulations and comparison to the theoretical result obtained in §6.1 for . The results show that fixed points (1-cycles) are the most common cycles, and increasingly so with increasing p. Again simulation results are in agreement with the expected theoretical value.
7 Conclusion
We have shown in the two well-known examples of adaptive systems in living beings, the nervous system and the immune system that they share a common property: External signals modify the rewriting of their organization and therefore work as stimuli to modify the ‘program’ of the system.
To get better understanding of this very common feature in the living beings, we have devised the concept of Self-programming machines or msp.
These machines have a finite set of inputs, are based on a recurrence and are able to rewrite their internal organization whenever external conditions vary. They have striking adaptation properties:
- – they stabilize almost exclusively into 1-cycles;
- – the proportion of 1-cycles to all possible n-cycles goes to 1 as the number of inputs increases (i.e. 1-cycles are almost certain);
- – they have similar properties whatever the operation defining the recurrence may be (linear or polynomial);
- – they will stabilize again in case of small perturbations or intentional massive breakdowns and have proportion of 1-cycles to all possible n-cycles closer to 1 when they are connected in networks.
These results bring us to make the following statement: adaptive properties of living systems can be explained by their ability to rewrite their internal organization whenever external conditions vary under the only assumption that the rewriting mechanism be a deterministic constant recurrence in a finite state set.