1 Introduction
A discrete dynamical system has the same definition as the continuous ones [1–9]. It involves a flow function f defined on , where T is a discrete time space (in general N) and E a discrete state space ( in the Boolean case and more generally a countable subset of ), representing, for each state e and time t, the state reached after time t by the trajectory starting in state e at time 0. We denote in general by , which permits to have a coherent notation for all the states of a trajectory. The set of such states is called the orbit of . Following [10], we can now define the discrete time derivative for the state vector of an automaton by: , which reduces to , if . By using the same formula, we can also define:
- – the space derivative: , if
- – the partial state derivative: .
A discrete automaton is defined by a transition function F: , where depends only on coordinates , being a neighbourhood of i in the space set S (in general the Manhattan – or – unit ball of centred on i and having a radius equal to 1), with conditions defining V on the boundary of S (e.g., periodic) and with constraints on the discrete velocity ensuring that the flow remains in E.
The aim of the paper is to find a discrete analogous for the definition of a potential (or gradient) discrete dynamical system (called here potential automaton) similar to those known in continuous systems. Then we will study as biological application a potential automaton whose orbits are the synonymy classes of the genetic code, the potential deriving only from simple physicochemical properties of the amino-acids corresponding to these synonymy classes.
2 Definition of a potential automaton
A continuous potential differential equation on is defined by: , , where P is a real continuously differentiable function (e.g., a polynomial with real coefficients) on . In the same way, a potential automaton on the discrete state space E is defined by:
(1) |
In the Boolean case, if , where is an interaction tensor, with as marginal matrices and , is an interaction matrix and a threshold line vector, we have, for the partial space derivatives of P:Example
Then the potential automaton associated to P is defined by:(2)
Hence we have:
and , where H is the Heaviside function. From (2), we derive:(3)
3 Properties of a potential automaton
Let us suppose that the state space E equalsand that P is defined on E by: ,, where A, W, B are respectively an integer tensor, an integer matrix and an integer line vector. Let suppose also that: ,. Consider now the potential automaton defined by: , if, and by boundary conditions, if, such that the flow remains in E. Then, if the tensor A is symmetrical with vanishing diagonal (i.e. if , and ), and if each sub-matrix on any subset J of indices in of and of W is non-positive with vanishing diagonal, P decreases on the trajectories of the potential automaton, for any mode of implementation of the dynamics (sequential, block sequential and parallel). Hence the stable fixed configurations of the automaton correspond to the minima of its potential P.Proposition 1
We have:Proof
In a potential automaton, we have , , hence:
(1) In the sequential updating, only one component changes its value between t and , hence:
(2) In the block-sequential updating, let denote by J the subset of reached by the sequential iteration. Then only the 's for k in J can change their value between t and , hence:
In the Boolean case, let us suppose that,, withand each sub-matrix on any subset J of indices inof W is non-positive. Then P decreases on the trajectories of the potential automaton defined byfor any mode of implementation of the dynamics (sequential, block sequential, and parallel). This automaton is a Hopfield-like neural network, whose stable fixed configurations correspond to the minima of P.Proposition 2
It is easy to check that from (2): , and from (3) and from :Proof
Then, for the block sequential iteration: , the result coming from the non-positivity of the sub-matrices of W or from [9]. □
The interest of Proposition 2 is to show that the Hopfield-like automaton, defined by:
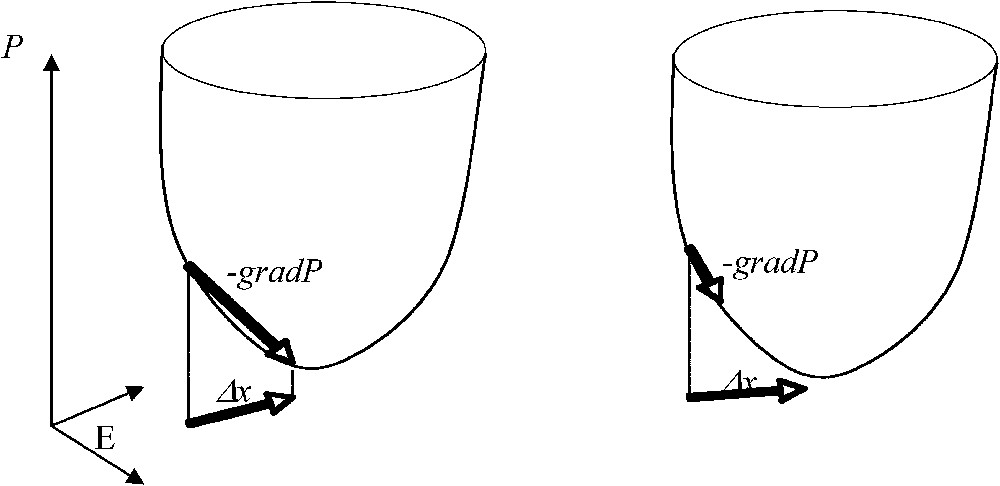
Potential automaton with Δx=−gradP (on the left) and automaton with a Lyapunov function decreasing on its trajectories (on the right).
4 Application to the genetic code
We will now use the notion of potential automaton introduced above in order to show what kind of biological significance can have the attraction basins of the stable fixed configurations (i.e. corresponding to the minima of the potential) of such an automaton (see also [11]).
The stereochemical hypothesis belongs to a theory about the origin of the genetic code for which the first complexes between amino-acids (AA) and nucleic acids (NA) have been the start of the autopoietic origin of life. This theory claims that, from a physicochemical similarity on three (dependent) dimensions: (i) the ability L of binding (in particular through hydrogen bounds), (ii) the hydrophobicity H, and (iii) the molecular weight P, codons are associated in an unique and degenerate way to their AA [13,14]. This correspondence can be summarized in Fig. 2, in which the variables H, L and P are given for each amino-acid showing a structure into synonymy classes with sizes from 1 to 6.
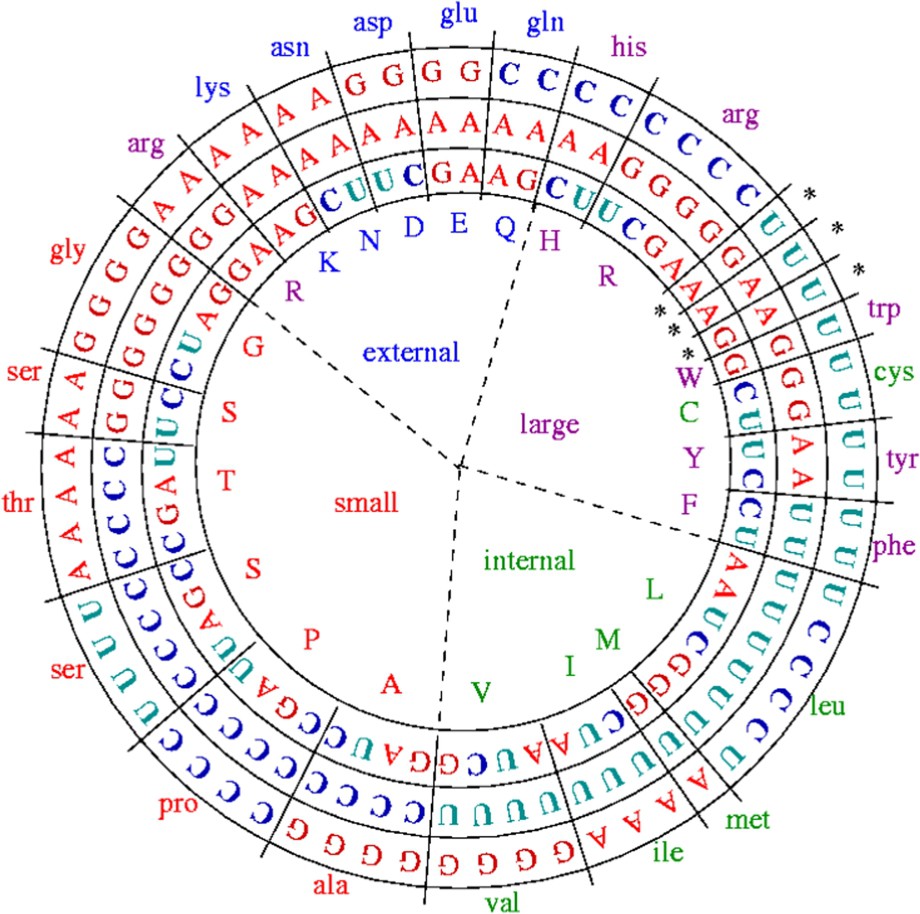
Repartition of the amino-acids and of their codons following two criteria, size (small or large) and hydrophobicity (high/internal and low/external) (after [12]).
According to the wobble hypothesis by Crick, the first two bases of a codon are essential for its affectation to a given amino-acid: we observe that the second base permits to share the codon space into classes (Fig. 3) associated with hydrophobic AA (central base uridine U or cytosine C) or heavy AA (central base U or adenine A), or able of binding AA (central base C or guanine G). Then we could represent each base by a Boolean number of three binary digits (e.g., 110 for U, the first 1 for the hydrophobicity, the second 1 pour the heavy weight and the last 0 for a weak ability of binding), summarizing their characteristics on the H, L and P axes. For the sake of simplicity, we will in the following reduce the coding to two binary digits, retaining only hydrophobicity and steric volume, redundant with P and L. We will show that it will suffice to explain the essential of the degeneracy of the genetic code, by taking into account optimality properties of the genetic code [15] and using a classical coding for the nucleic bases [1], i.e. , , et . We will also define a potential automaton SUSY (SUrrogate SYstem), linking codons and AA having similar physicochemical properties like hydrophobicity (resp. hydrophily ) et big (resp. small) steric volume, positively correlated to P and L.
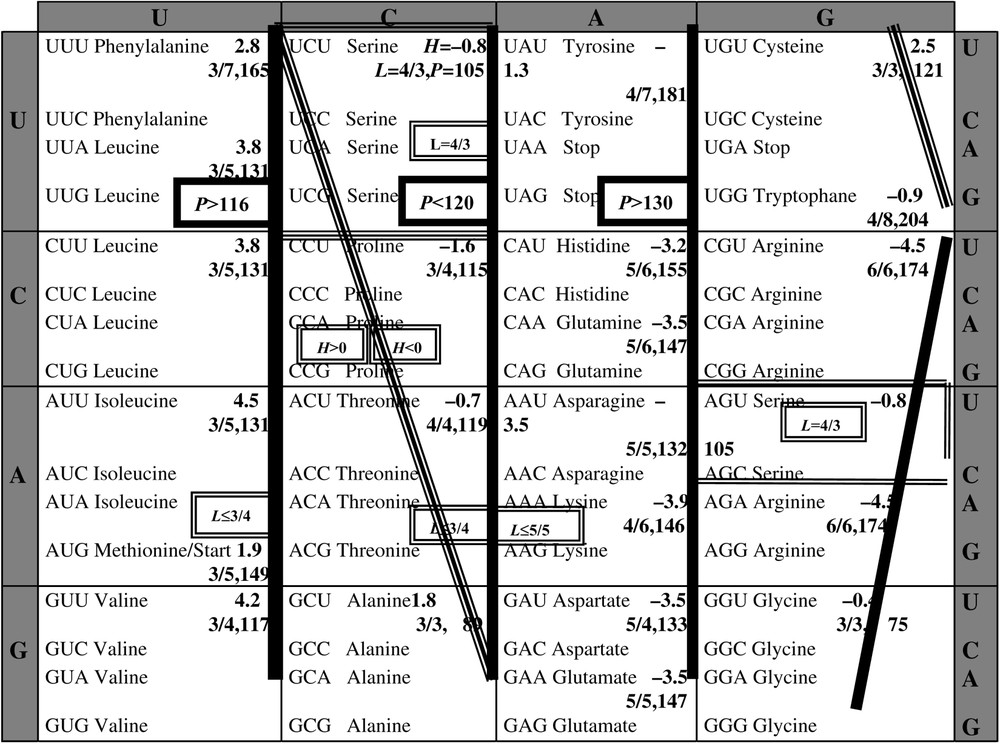
Table of the genetic code, indicating the hydrophobicity H, the maximal number L of possible hydrogen bounds divided by the length of the longest carbonate chain and molecular weight P, for each amino-acid of a synonymy class of codons.
Let us define, for each configuration W in , the set of Boolean numbers of six binary digits, the subset and :
- –
where D is the Hamming distance, weighted following the Crick's wobble, which defines the codons ordering O (given in Fig. 3 from 1 to 64, for the Ws varying between 111111 and 000000):
- – S is a similarity function between codons X and Y: ,
- –
coefficients and are fixed by electrostatic (hydrophobicity H) and steric (molecular weight P and ability of binding L) properties of the amino-acids [16]:
Then the potential automaton SUSY is defined on by:
The attraction basins can be identified in the framework of the genetic code table in Fig. 4.
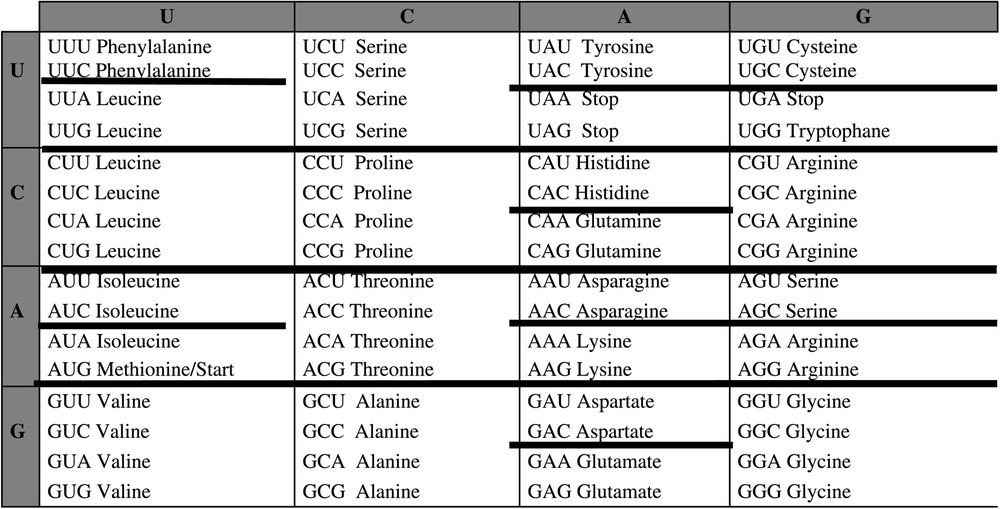
Identification of the attraction basins of the automaton SUSY in the genetic code table.
If we except codon 111100, whose similarity with 101100, the only codons having an incorrect affectation [17,18] are:
- • 011101, to relate to the block (),
- • 110001, to relate to the block (),
- • 010000, whose block has to be related to those of 100000 (),
- • 010011, whose block has to be related to those of 111011 ().
For the two last codons above, the three digits coding with a complement of similarity equal to 1 for the equality between the third digit of the two first bases of a codon would relate 010001001 to the block of 101001001 () and 010001110 to the block of 110101110 (). In any case of incorrect affectation we would be then very near to the above critical threshold of 10 defined for the similarity between the five first digits, in coherence with the stereochemical hypothesis and with the previous observations of the Gray code regularities [12,19,20].
5 Variational properties of the system
The automaton SUSY not only maximizes a similarity function, but it gives final synonymy classes verifying two other variational properties, one based on a maximal information principle and the second showing a maximal congruence with present nucleic ‘fossils’ existing in many types of cells.
5.1 A maximal information principle
The easy way shown above to obtain a sketch of the synonymy classes through the potential automaton SUSY reinforces the stereochemical hypothesis. Apart from the amino-acids with six codons, the result is particularly convincing for the amino-acids the most frequent both in the Miller experiment and in the human genome as recalled in the table in Fig. 5 [21,22]. The mutual benefit taken by the RNA and AA worlds from a possible primitive direct association as predicted by the stereochemical theory (four out of the AA in Fig. 5, Leu, Glu, Val and Arg have preferential affinities with their codons and anti-codons [24]) is compatible with their Darwinian co-evolution well summarized by de Duve [25]: “The theory considered most likely today supposes a historical, co-evolutionary process in which the anticodons and the corresponding amino-acids were progressively recruited together under the control of natural selection. Several arguments support this hypothesis. The most convincing lies in the structure of the code, which, far from being random, happens to be such as to minimize the deleterious consequences of mutations.”

Table giving the frequencies of occurrences of the AA in human genome [23] and in the genetic code, and giving their rank in a consensus chronology [22] and in the Miller experiment [22].
We can interpret in a very schematic way this progressive adaptation by using a simple variational criterion, based on the dual principle of the minimization of the mutation function M and of the maximization of the genetic code information I.
Fig. 6 gives the optimal classes repartition frequency for a code with 2 AA, obtained at the intersection of the graphs of the functions
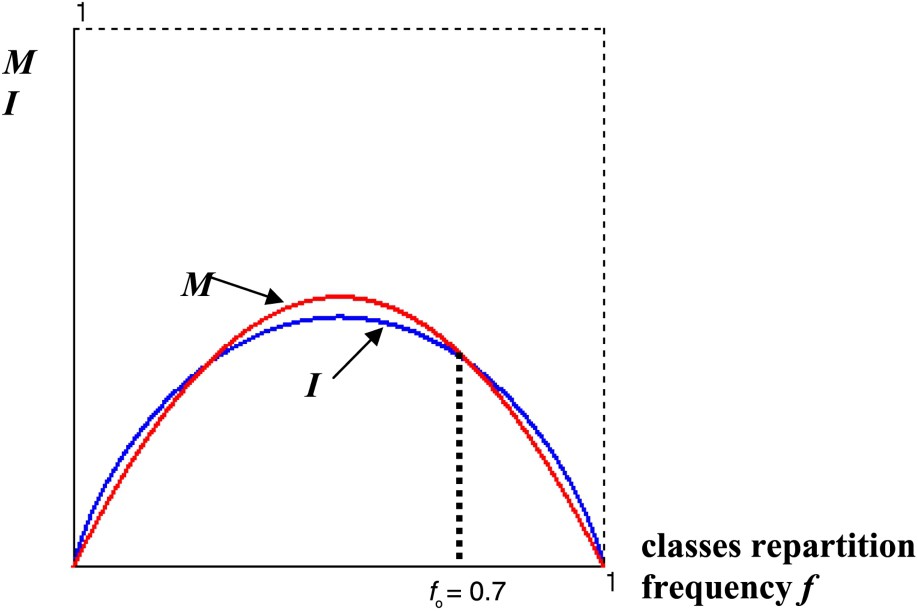
Graph of the mutation function M and of the information I showing the optimal frequency .
If now we renormalize the repartition of the genetic code in 21 synonymy classes or clusters: 3 of 6 codons, 5 of 4, 2 of 3, 9 of 2 and 2 of 1, following at each step the rule such as we roughly respect the optimal proportion for one of the bigger renormalized class, we get a coherent tree (Fig. 7), satisfying at each bifurcation the above variational criterion. For example, the first renormalization concerns clusters of size 1 and 2, we combine for obtaining clusters of size 3 and 4 (see the bottom line of the tree) and secondly with clusters of size 4 and 6 for obtaining the third line from the bottom, which corresponds to the affectation of codons to only 8 amino-acids (e.g., the 8 first in Miller's experiment). This short proof explains the classical properties of resistance to the mutations shown by the genetic code [26,27] compatible with the stereochemical hypothesis used in the automaton SUSY.
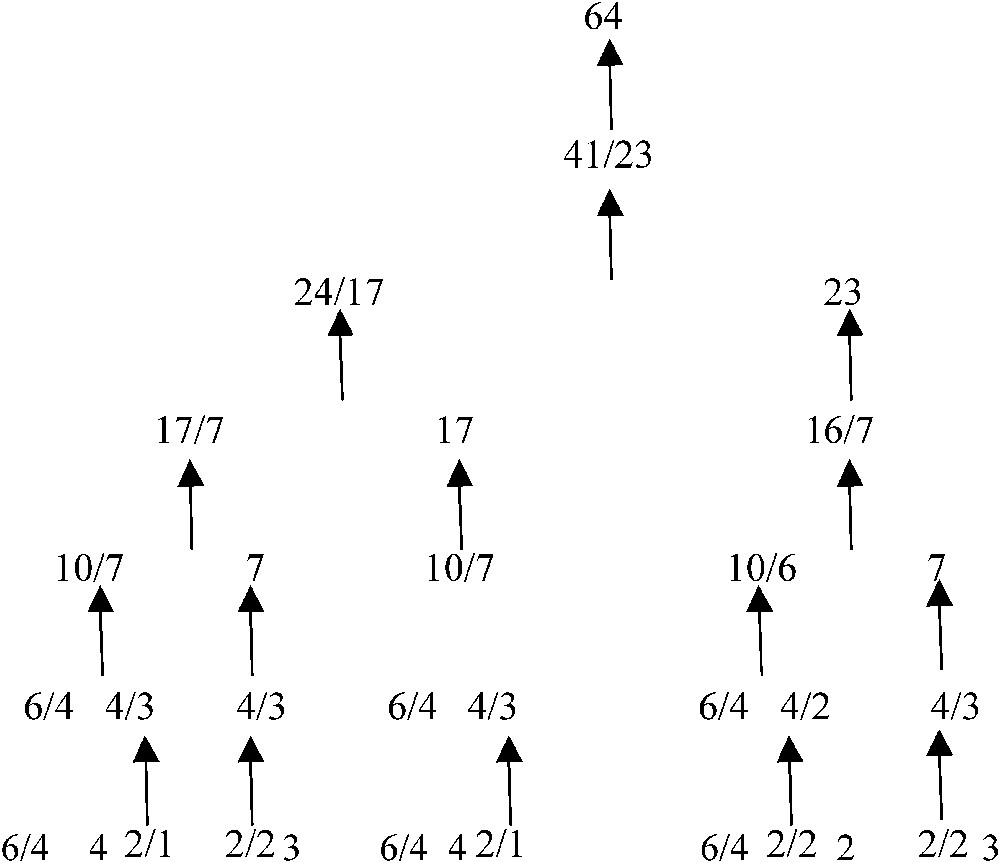
Renormalization tree respecting the optimal frequency for the synonymy classes of the genetic code.
5.2 A maximal congruence with present nucleic ‘fossils’
Some RNA relics exist in practically all eukaryote and prokaryote cells: tRNAs and miRNAs represent such fossils preserved during the evolution. We have already proved [1,2] that the most conserved part of tRNAS (their loops) were similar to primitive circular RNAs made of 1 and only 1 representative of each codon class, obtained by maximizing their affinity to AAs and minimizing their length. In the presently known functional miRNAS, we have the same similarities. For example, there is 222 known human miRNAs, plus 76 supplementary human miRNAs recently discovered [28], similar to a primitive circular RNA called AB: CAAGACUAUGAAUGGUGCCAUU, with a significativity p less than 21 – as shown in Fig. 8, which presents consensus sequences for these 76 miRNAs.
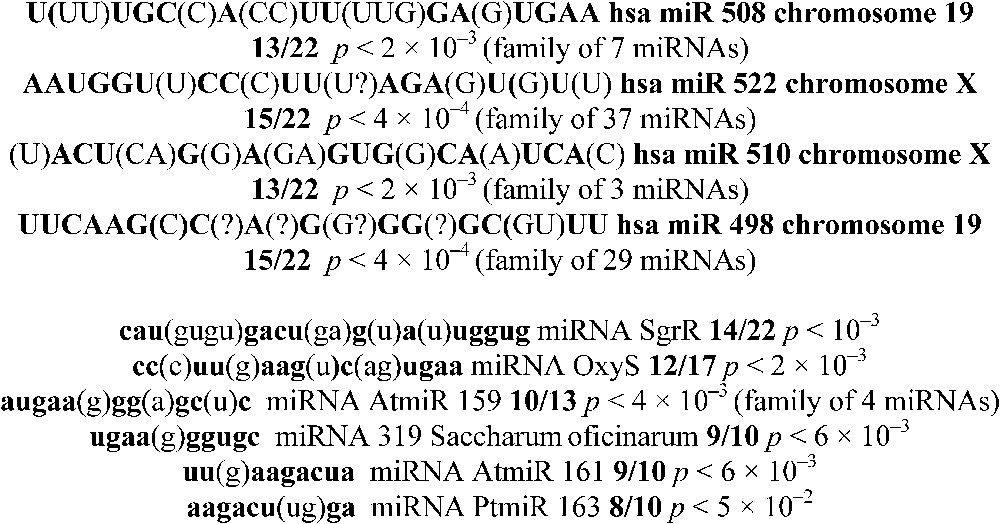
Similarities between miRNA consensus sequences and a circular primitive RNA called AB.
Fig. 8 presents also consensus sequences for miARNs coming from bacteria and plants [29,30] showing the same similarities. Further statistical studies [31] confirm this fact and the miRNA world can then be considered as a fossil reservoir coming from primitive RNAs built with respect to the synonymy classes obtained from the automaton SUSY.
6 Conclusion
We have introduced in this paper the notion of potential automaton, which gives a natural framework to previous discrete systems like Hopfield neural networks, well studied in the past for the existence of Lyapunov energy functions. In a further study, we will introduce the notion of Hamiltonian automaton and propose new directions of research towards the potential-Hamiltonian decomposition of discrete dynamical systems (cf. [5,6] for the continuous case). An application of this new notion of potential automaton concerns the genetic code for which we proved that the degeneracy in synonymy classes of codons can be partly explained by the action of a potential automaton based on the stereochemical properties of both the amino-acids and their codons.
Acknowledgements
We are very indebted to L. Segel and J. Maynard-Smith (died in 2004) for inviting us to pursue researches in the field of theoretical biology and for showing and signalling the road.