Version française abrégée
L'explosion d'intérêt pour les graphes non aléatoires comme représentation des réseaux réels, constitués de nœuds interconnectés, a généré une importante et croissante littérature, aussi bien à partir de l'observation de ces réseaux qu'à partir de la construction de modèles spécifiques. Ces réseaux, pouvant représenter des réactions métaboliques, des régulations génétiques, des relations linguistiques, des graphes sociaux, des connexions Internet, etc., sont approchés par une relation générale entre la connectivité des nœuds, cette dernière étant définie comme le nombre de connexions (non dirigées) liées à un nœud. Cela permet ainsi de caractériser les modèles par la distribution de leurs degrés de connectivité. Par exemple, le nombre de nœuds nr de connectivité r pour les réseaux scale free est donné par une loi de puissance nr∝r−β. Bien qu'il existe plusieurs moyens d'obtenir des exemples spécifiques de réseaux non aléatoires, basés sur différentes méthodes d'évolution des connexions, il n'existe pas encore de voie systématique et générale permettant d'obtenir une distribution donnée, les modèles courants étant trouvés largement par un processus d'essai erreur. De plus, nombre de modèles dynamiques d'évolution de réseaux peuvent mener à une même distribution des degrés de connectivité, ces derniers ne se rapportant donc pas forcément à l'évolution présente.
Dans cet article, nous allons montrer par une approche thermodynamique qu'il est possible de caractériser un réseau présentant une configuration de nœuds ayant une entropie maximale et soumis à des contraintes. Ceci n'a pas un intérêt seulement technique : la contrainte peut en effet être interprétée comme une fonction de coût qui, en principe, représente le coût en énergie libre pour l'établissement du réseau et dont la forme contient implicitement des informations sur l'évolution du système. Nous espérons donc que cette approche permettra de mettre en évidence la structure du réseau, avec des applications possibles aux réseaux métaboliques, génétiques et protéiques. Pour construire un réseau présentant une distribution donnée des degrés de connectivité, nous avons besoin de déterminer la contrainte appropriée menant à cette distribution. Nous ferrons alors évoluer les connexions du réseau, choisi arbitrairement, en en maximisant l'entropie. Le tout repose sur une dynamique d'évolution respectant la contrainte.
Nous illustrons cette approche avec une application au cas simple des réseaux exponentiels, qui sont d'une certaine manière analogues à un gaz parfait. Comme nous allons le montrer, cette analogie n'est pas complète, car la probabilité d'avoir une connexion entre nœuds de degrés donnés n'est pas indépendante des degrés. Nous estimons cet effet sur l'entropie du réseau en montrant qu'elle diffère de celle des réseaux aléatoires d'une petite quantité – ce qui, dans un sens, fait que les connexions sont presque aléatoires. Enfin, nous montrons comment la relation entre les variables thermodynamiques intensives et extensives du réseau apparaı̂t naturellement par cette approche. Nous émettons l'idée que la variable intensive des réseaux exponentiels – et par extension pour tout réseau de classe similaire – est une mesure de la complexité du réseau, que nous avons appelé complexité β. Nous montrons comment ce type d'analyse des réseaux dans les organismes vivants promet de fournir les variables macroscopiques appropriées à la vie.
Considérons un système de N nœuds, avec nr de degré r et ayant la probabilité prs qu'un nœud de degré r soit connecté à un nœud de degré s. L'entropie des nœuds du réseau (à une constante additive près) est :
Pour construire un réseau ayant une contrainte donnée, nous partons d'un réseau arbitraire, un réseau aléatoire ou ordonné, par exemple. Nous faisons alors évoluer les connexions, tout en maintenant la contrainte, et nous vérifions si cette action a augmenté l'entropie. Si c'est le cas, nous acceptons le nouveau réseau et nous continuons le processus. Sinon, nous acceptons la nouvelle configuration avec une probabilité correspondant à , où k est une constante établie par essai erreur afin d'améliorer la convergence de la méthode. Ce processus continue jusqu'à ce que l'entropie, soumise à la contrainte, soit maximisée.
Il est à noter que la similarité avec l'algorithme de Metropolis n'est que superficielle. Nous ne suggérons pas que les propriétés de l'état stable du réseau sont déterminées par une moyenne de l'ensemble. Cette approche permet plutôt d'empêcher le réseau de se fixer dans un état correspondant à un maximum local d'entropie. Cependant, la solution n'est pas attendue pour être unique, dans la mesure où la distribution des degrés de connectivité ne caractérise pas complètement le réseau.
Pour des réseaux exponentiels :
(3.1) |
Il est intéressant de comparer la valeur du modèle « entropie » ∑nrlognr avec la vraie valeur statistique de l'entropie prenant en compte les corrélations entre nœuds. Désignons nrs le nombre de nœuds de degré r connectés aux nœuds de degrés s. Si les connexions étaient aléatoires, la probabilité pour des liens d'un nœud fortement connecté d'être reconnecté à tout autre nœud et la probabilité pour des liens d'un nœud de faible degré de connectivité d'être reconnecté à tout autre nœud devraient être les mêmes :
(4.1) |
(4.2) |
La fonction de coût joue le rôle d'une énergie interne pouvant être changée de deux manières : en reconnectant (ajout de chaleur), ce qui changera la distribution des nœuds, ou par croissance du réseau, sans changer la distribution des nœuds (lors d'un travail) :
(5.1) |
L'approche thermodynamique fournit donc une caractérisation naturelle des variables macroscopiques associées à tout réseau. D'une certaine manière, c'est la fin de l'histoire, mais nous aimerions clairement acquérir une intuition quant à la nature des variables macroscopiques tout comme pour la pression d'un gaz ou la magnétisation d'un milieu.
Un nombre d'approches a été proposé pour définir la complexité des réseaux. Dans la théorie des graphes, elle est dérivée de la propriété des arbres. La complexité structurale peut être définie en fonction du nombre de paramètres requis pour définir le réseau. La complexité des liens a été quant à elle définie comme la variabilité du second plus court chemin entre nœuds. Toutes ces définitions ont en commun, en contraste avec la notion de complexité algorithmique en informatique, le fait que les systèmes ordonnés et aléatoires ont une complexité nulle.
Nous avons proposé une approche plutôt différente, issue de la considération suivante. En effet, une autre caractéristique commune aux réseaux aléatoires et ordonnés est que l'entropie par nœuds est indépendante du nombre de ceux-ci. Ceci peut être paraphrasé en disant que l'information locale est suffisante pour déterminer la large structure d'échelle des graphes. Nous voulons que la notion de complexité capture l'étendue où ce n'est pas le cas. Ainsi, cet aspect de la complexité des graphes est encodé dans la relation entre la distribution des nœuds liés par une connexion, deux connexions, etc. Les graphes aléatoires et ordonnés restent aléatoires et ordonnés par cette sorte de renormalisation. De manière similaire, les graphes exponentiels et scale free restent approximativement les mêmes par cette transformation. Cela confirme que la complexité de tels graphes peut être captée par n'importe quelle paire de nœuds. En d'autres termes, cette forme de complexité de réseaux peut être exprimée par un simple paramètre. Par exemple, nous pouvons nous concentrer sur les premiers et les derniers liens, à savoir le paramètre de voisinage C et la longueur caractéristique L. Par exemple, pour le réseau de small world de Watts et Strogatz, nous montrons que le paramètre C/L a en effet le caractère d'une mesure de la complexité, que nous appelons la complexité β.
Pour les réseaux exponentiels, nous montrons que la complexité β varie selon β−3. Si nous interprétons β comme l'inverse d'une température, cela signifie que la complexité décroı̂t quand la température décroı̂t. Ceci est attendu, car, pour de faibles valeurs de β, la distribution des degrés des nœuds approche une constante inférieure à 1/β. De manière équivalente, le « coût » par nœud, tel qu'il est mesuré par la valeur de la contrainte, décroı̂t comme β−1.
Nous voulons maintenant comparer ceci avec la définition thermodynamique de la complexité des réseaux, qui, d'après nos hypothèses précédentes, sera de la forme :
En résumé, nous avons montré que la méthode thermodynamique nous permettait de construire des réseaux ayant n'importe quelle distribution des degrés de connectivité. Nous donnerons d'autres exemples par ailleurs. Pour établir si cette approche permet de comprendre les propriétés des réseaux, nous avons besoin de regarder des applications correspondant des systèmes explicites. Par exemple, de nombreux processus biologiques peuvent être décrits par la loi canonique simplifiée (SCL) de Mandelbrot :
Dans le langage des réseaux thermodynamiques, cette distribution correspond à une fonction de coût, qui décrit le système dans lequel l'information est transmise sous forme de mots avec un coût constant par bit. Cela suggère une correspondance avec le coût de synthèse d'une enzyme. Par ailleurs, le coût du réseau exponentiel croı̂t linéairement avec le degré, ce qui exprime la conservation du nombre de liens dans un réseau. Cette approche pourrait nous permettre d'expliquer, par exemple, la connectivité différentielle dans la régulation des réseaux génétiques de la levure. L'approche thermodynamique nous permet de caractériser les variables macroscopiques d'un réseau et donc, par extension, de n'importe quel système pouvant être décrit en terme de réseau. En particulier donc, l'approche nous offre la possibilité d'une caractérisation macroscopique de la vie.
1 Introduction
The explosion of interest in non-random graphs as representations of real-world networks of connections between nodes has generated a large and growing literature, both in the characterisation of observed networks and on methods of construction of specific models [1]. In all of these cases, the specific contexts, in which networks may represent metabolic reactions [2], genetic regulation [3], linguistic relations [4], social graphs [5], internet connections [6] and so on, are abstracted into a general relation between the connectivity of the nodes. To this end, we define the degree of a node as the number of (undirected) connections to or from that node. The models can then be characterised by the distribution of nodal degrees. For example, in the now well-known, scale-free networks, the number of nodes nr with r connections is given by a power law nr∝r−β. Although many ways are known to obtain specific examples of non-random networks by evolving the connections according to various schemes [1], there is as yet in general no systematic way of constructing the connections in a network to obtain a given nodal distribution, the current models being found largely by trial and error. Furthermore, since many dynamical models for the evolution of a network can lead to the same nodal distribution [7,8], the dynamical models may be unrelated to the actual evolution.
In this paper we shall show that a thermodynamic approach allows us to characterise a network as a maximum entropy configuration of nodes subject to an external constraint. This is not only of technical interest: the constraint can be interpreted as a cost function, which in principle represents the cost in free energy of establishing the network. The form of the cost function therefore implicitly contains information on the evolution of the network. We therefore expect this approach to illuminate the structure of networks with possible applications in metabolic, genetic and protein networks.
To construct a network with given nodal distribution, we need to derive the relevant constraints that will lead to the given distribution. This is done in section 2. Starting from a given arbitrary network, we then evolve the connections to maximise the entropy of the network. The trick here is to find an evolutionary dynamics that respects the constraints.
We illustrate the approach with an application to the simple case of exponential networks, which are somewhat analogous to a perfect gas. As we shall show, the analogy is not complete, because the probability of a connection between nodes of given degrees is not independent of the degrees. We estimate the effect of this on the network entropy, showing that it differs from random by a relatively small amount (i.e. that in this sense the connections are close to random). Finally, we show how a relation between intensive and extensive thermodynamic variables for the network arises naturally from this approach. We speculate that the intensive variable for exponential networks (and, by extension, for any similar classes of networks) is a measure of the complexity of the network that we have called β-complexity. We show how this type of analysis of the networks in living organisms this approach promises to provide us with the macroscopic variables appropriate to life.
2 The thermodynamic method
Let us consider a system of N nodes with nr of degree r and with probability prs that a node of degree r is connected to a node of degree s. The entropy of the nodes of the network (up to an additive constant) is:
The usual method of Lagrange multipliers gives
Thus, for any distribution nr, we can construct appropriate constraints.
To avoid confusion, note that the nr values are not all independent, so the integration cannot be carried out unless they are known explicitly. For example, if we want an exponential network, we have , hence (up to a constant) as expected.
To construct a network with given constraint, we begin from an arbitrary network, for example a random network or an ordered one. We then make some rewiring, subject to maintenance of the constraints, and test to see if the entropy is increased by this action. If it is, we accept the new network and continue. If it is not, then we accept the new configuration with probability , where k is a constant set by trial and error to improve the convergence of the process. The rewiring is continued until the constrained entropy is maximised.
Note that the similarity here to the Metropolis algorithm [9] is superficial only. We are not suggesting that the steady-state properties of the network are determined by an average over this ensemble. Rather the approach is intended to prevent the network settling into configuration with a shallow local maximum of entropy. Even so, the solution is not expected to be unique in those cases where the distribution of nodal degrees does not completely characterise the network. (For example, various different networks with the same scale free node distribution are known [10].)
3 Application to exponential networks
For exponential networks
(3.1) |

Evolution of the entropy of the network during the process of maximising the entropy. The number of rewiring trials, after a pair of linked nodes has been randomly chosen, is about 1% of the total of the possible new linkages for one of those nodes. This condition is set to improve the convergence without affecting the random process. Then the total number of rewiring trials is about 1 600 000.
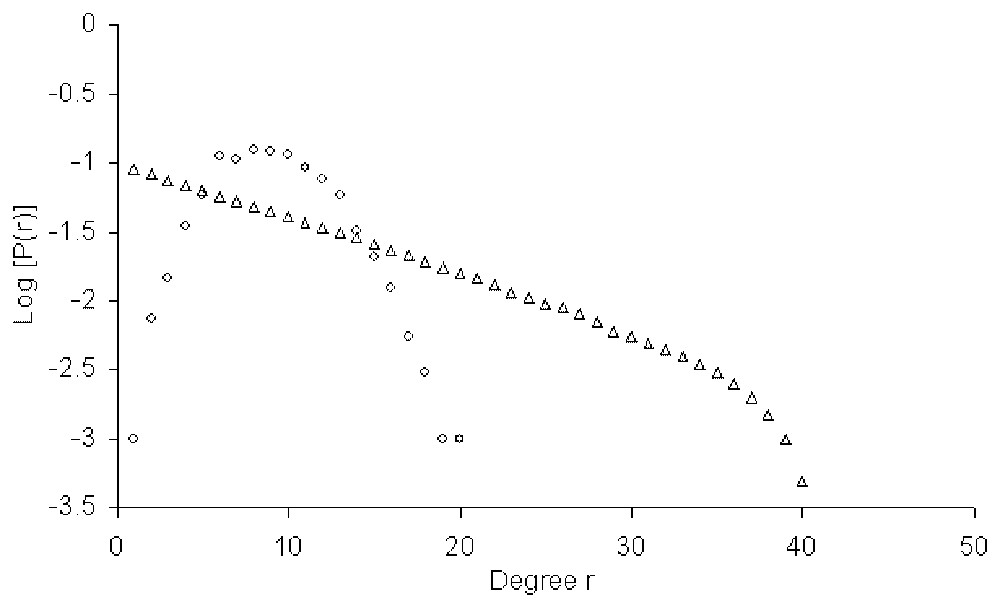
The degree distribution of the nodes before and after the process of maximisation. The network contains 2000 nodes and is constructed as explained in the text. The empty circles are for the random network (prior to entropy maximisation) and the empty triangles are for the exponential network.
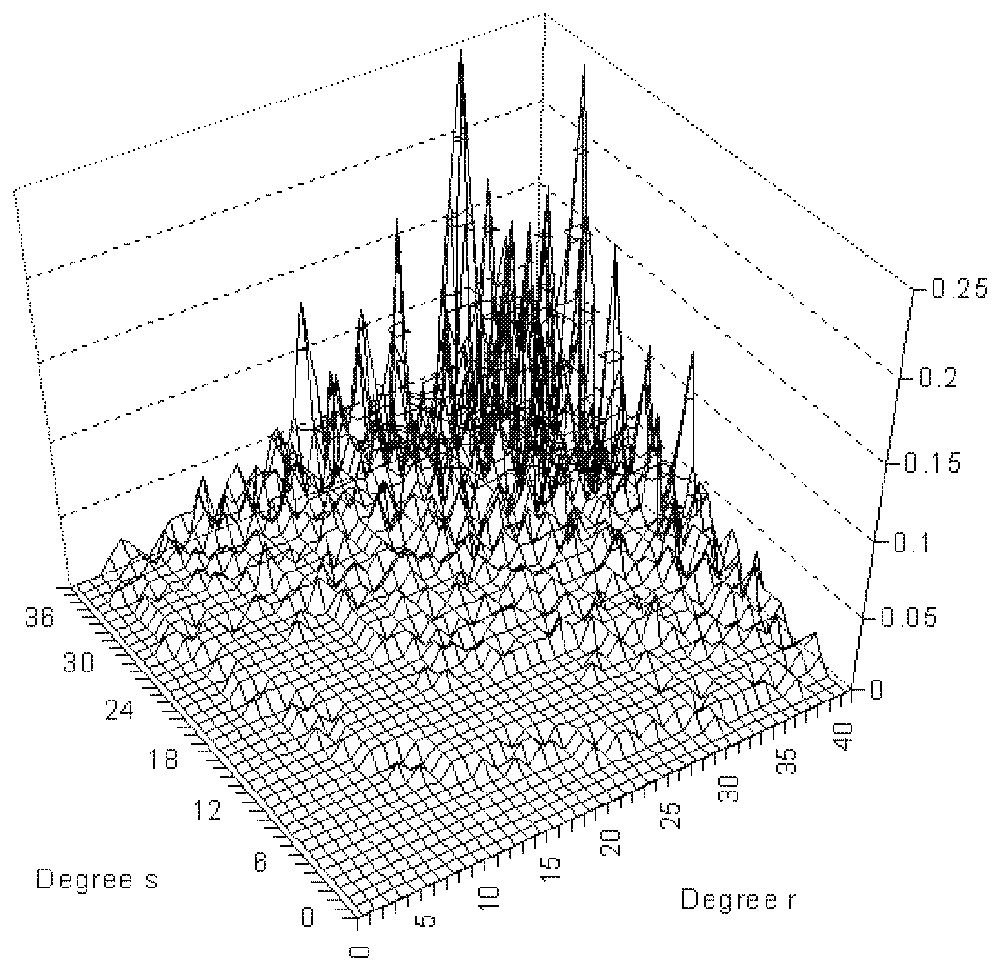
Distribution of the connection probability in an exponentially connected network between nodes of degree r and s.
4 Entropy of an exponential network
It is of interest to compare the value of the model entropy ∑nrlognr associated with the degree distribution of the nodes with the true statistical entropy of the network, taking into account correlations between the connections of the nodes. Let nrs be the number of nodes of degree r that are connected to nodes of degree s. The probability of finding a connection between a node of degree r and a randomly selected node of degree s is nrs/ns. If the connections were made randomly, this probability is independent of the degree of the node to which the connection is made, so we should have:
(4.1) |
(4.2) |
For a general network, the entropy is still given by (4.2) but with (4.1) now used to define:
Fig. 4 shows how the ratio of the entropy contributed by the nodes to that of the links depends on the size and mean connectivity of the exponential network. The ratio is defined in such a way that it is unity for a random network, namely as:

Ratio of the entropy contributed by the nodes to that of the links plotted against the mean connectivity. This ratio is 1 for random networks.
5 Intensive and extensive variables
The cost function plays the role of an internal energy, which can be changed in two ways: by rewiring (adding heat) which will change the node distribution, or by growth of the network without changing the node distribution (doing work):
(5.1) |
The first term on the right is just the change in entropy of the nodes (multiplied by β−1). The second term on the right represents, in some sense, the cost per node associated with varying the external parameters. The external parameter might be, for example, the total number of nodes, or the total number of connections; for each choice, X, there will be an associated intensive variable, x, defined by (5.1):
The thermodynamic approach therefore provides a natural characterisation of the macroscopic variables associated with any network. In a sense, this is the end of the story, but we would clearly like to acquire an intuitive feeling for the nature of the macroscopic variables associated with a network, just as we derive an intuitive understanding of the pressure of a gas or the magnetisation of a medium. In the next section, we argue the case for regarding the quantity x as a measure of the complexity of a network, related to what we have called β-complexity [11].
6 Network complexity
A number of approaches to defining the complexity of networks have been proposed. In graph theory the complexity is derived from the properties of the spanning trees. Structural complexity [12] can be defined in terms of the number of parameters required to define the network. Edge complexity [13] has been defined in terms of the variability of the second shortest path between nodes. What all of these definitions have in common, and in contrast to the notion of algorithmic complexity in computer science, is that both ordered systems and random ones have zero complexity.
We have proposed a rather different approach to network complexity, which arises from the following consideration. Another feature that random and ordered networks have in common is that the entropy per node is independent of the number of nodes. This can be paraphrased by saying that local information is sufficient to determine the large-scale structure of the graphs. We want the notion of complexity to capture the extent to which this fails to be the case. Thus this aspect of the complexity of a graph is encoded in the relation between the distributions of nodes linked by one connection, two connections and so on. Random graphs and ordered graphs remain random and ordered under these ‘renormalisation’ transformations. Similarly, exponential graphs and scale-free graphs remain approximately exponential or scale-free respectively under these transformations. This suggests that the complexity of such graphs can be captured by any pair of links in this chain of connections. In other words, the complexity of networks of this form can be expressed by a single parameter. For example, we can concentrate on the first and last links in the chain, namely the cliqueiness parameter C and the characteristic path length L [13]. For example, for the small world network of Watts and Strogatz [14], we show that the parameter C/L does indeed have the character of a measure of complexity (Fig. 5), which we have called β-complexity.

The ratio of clustering coefficient to characteristic length scale (or diameter) of the small world network of Watts and Strogatz [13] behaves as a complexity parameter. The coefficients are scaled to 1 for p=0.
For the exponential network we show the average value of C/L as a function of the mean connectivity ν of the nodes in Fig. 6. In terms of the parameter β, the curve is fitted fairly closely by C/L∝β−3. If we interpret β as an inverse temperature, then this says that the complexity decreases with decreasing temperature. This is to be expected since for small β the distribution of the degrees of the nodes approaches a constant for degrees <1/β. Equivalently, the ‘cost’ per node, as measured by the value of the constraint, decreases with β−1.
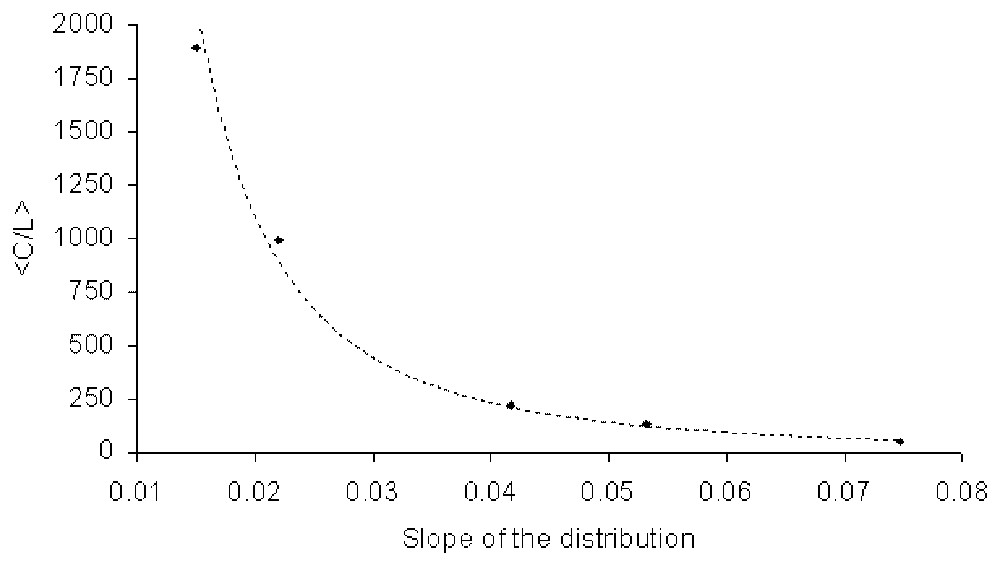
Value C/L against the slope of the exponential distribution (in the log-linear plot of Fig. 2). The graph is plotted for the following mean connectivities, respectively 6, 8, 10, 16, 20. The curve is fitted closely by a power function with exponent close to −2.25.
We now want to compare this with the thermodynamic definition of network complexity, which, according to our hypothesis above, will be of the form:
7 Conclusions
We have shown that the thermodynamic method allows us to construct networks of any given node distribution. We shall give some other examples elsewhere. To establish whether the thermodynamic ideas in this paper can provide any further insight into network properties, we need to look at applications to explicit systems. For example, many biological processes can be described by the Mandelbrot simplified canonical distribution (SCL) [15]:
In the language of thermodynamic networks, this distribution corresponds to a cost function that describes a system in which information is transmitted in words with a constant cost per bit. This suggests a correspondence with the cost of synthesising enzymes. On the other hand, the exponential network has a cost that grows linearly with rank expressing the conservation of the number of links in a network. This approach may enable us to explain, for example, the different connectivity in the regulation of the genetic network in yeast [3].
The thermodynamic approach allows us to characterise the macroscopic variables of a network, and, hence, by extension, of any system that can be described in network terms. In particular, therefore, the approach holds out the possibility of a macroscopic characterisation of living systems.
Acknowledgements
We have benefited from conversations with Camille Ripoll, Jeremy Ramsden and Steve Gurman.