1 Introduction
The main thrust of ENFIN is to achieve a new paradigm in our ability to understand biological systems using computational methods. There are three orthogonal aspects to this work: the semantic definition of the data being generated, the distributed network aspects of the data generation and storage, and then appropriate analysis of the data.
A major missing component worldwide is the deployment of “GRID-like” and webservice technologies in modest bioinformatics groups and in standard molecular biology laboratories. Currently the focus for much of the GRID and ontology work has quite rightly been towards the large data providers, in particular the publicly accessible archive databases. However, smaller groups are being excluded from this development for both reasons of documentation and accessibility to competency and computational resources and also because the scientific drivers on the larger projects are focused towards solving problems of interest to the large databases, which rarely overlap with the problems being faced by smaller research groups. ENFIN developed a computational infrastructure appropriate to small research-level bioinformatics groups and everyday molecular biology laboratories. The ENFIN core layer (called EnCORE) therefore aims to provide a lightweight set of standard computational interfaces where the output document from one analysis service can easily be provided in as the input to the next analysis. These software systems are built from open source components and have been written to work in a peer-to-peer fashion, utilizing XML- and GRID-based technologies wherever possible. We also leverage the extensive investment in GRID technology from European programs such as EMBRACE to provide progressively better interoperability between data installations in geographically diverse locations. The aspects that ENFIN aims to provide is the development of a turnkey system using these components, and then the necessary training, documentation and proof of concept of using this system to communicate between bioinformatics and experimental laboratories. We will introduce the latest development in analysis pipelines, FuncNet, a distributed protein function analysis network designed to provide an open platform for the computational prediction and comparison of protein function.
Besides the EnCORE platform development, a panel of informatics tools (EnSUITE) forms the analysis layer of ENFIN and we develop them in tightly coordinated iterative loops between experimental and computational groups. There is a large array of potential methods to apply in this area, all with their own requirements for inputs and their own strengths and weaknesses. Our approach is focused on a limited number of techniques, split into three classes: discrete function prediction, network reconstruction and systems-level modeling. Research topics cover areas such as metabolic and signaling pathways related to cancer or diabetes (Fig. 1).
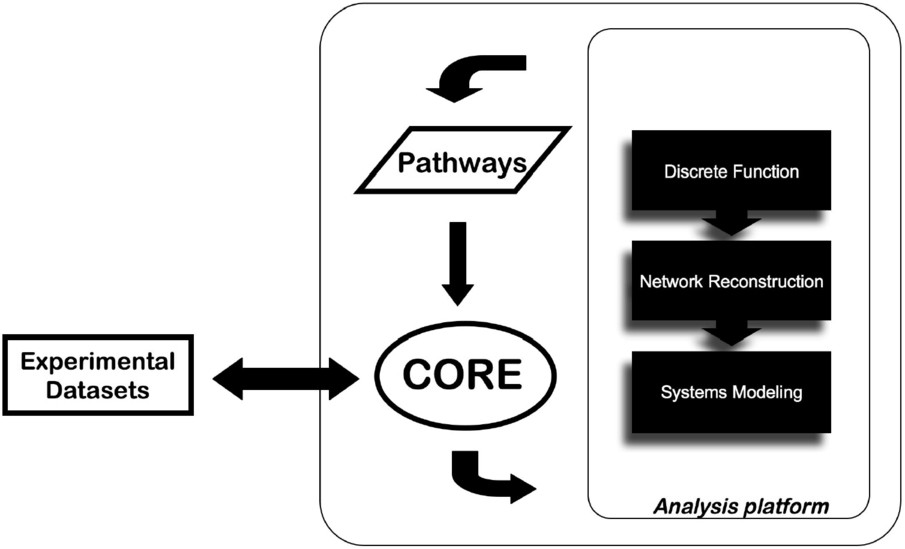
The approach of data integration and analysis by ENFIN. The data provided by the user are integrated via the EnCORE platform and channeled to the analysis platform. Results can be integrated to existing databases and return to the user.
With the foundation of the EnCORE platform and the availability of the computational tools developed in EnSUITE over various disciplines and data types, we are exploring the possible integration depth of webservices workflow technology for research at a systems level.
2 The data integration platform
The EnCORE system is implemented as a growing set of web services, providing open access and a modular, extensible structure (Fig. 2). All services follow the Simple Object Access Protocol (SOAP), to ensure platform and language independence. At the heart of EnCORE is enXml, an XML schema, used as the standardized data exchange format between the web services. It describes the core components of the ENFIN data model: experiments, sets and molecules. Experiments include both wet lab experiment results and bioinformatics data transformations and comprise one or more sets. Sets provide a convenient model to allow set-oriented bioinformatics operations on molecules or other sets. Molecules represent biological molecules such as proteins, which are traceable through multiple conversion steps, even when converting between data types in potentially ambiguous ways.

The EnCORE platform used at first the ArrayExpress [25], IntAct [26] and Reactome [27] software components. During the last 3 years, up to 13 webservices have been progressively added to the platform. These webservices give access to databases, analysis tools and ID converters, which are fully compatible by the use of the enXml schema. For example, it would be possible to map a given set of protein identifiers from various identifier name-spaces to UniProt accessions and retrieve all the pathways from the Reactome database that contain any of the proteins of the set. The proteins involved in one or more pathways could then be passed on to the EnCORE-IntAct service, to obtain sets of experimentally-verified interaction partners for each protein. Finally, the EnCORE-UniProt service could retrieve information for all the resulting proteins, such as the sequence, sequence length and keywords amongst others.
EnCORE web services take enXml-schema-conform XML documents as input and produce modified documents as output by only adding to its content and thus preserving an audit trail within the document. This standardization of the input and output parameters of the EnCORE web services also makes it very easy to chain the services into workflows by just passing-on the XML document. Creating such a workflow using workflow management tools such as Taverna [1] becomes a simple task.
The advantages of a structured XML data exchange format are not exempt of side effects, however. One common issue is that data has to be sent between the workflow executing client and the respective servers running the used services. For a large number of services or large datasets, this results in increased network traffic, which can represent a bottleneck for the performed analysis. The nature of XML, providing a clear structure and readability at the expense of efficiency, increases this risk. For smaller datasets and workflows (for example a standard workflow containing 5 services and run with a few hundred protein accessions) the ever increasing and improving network resources can easily counter-balance this effect. However, for large datasets, especially with respect to the increasing importance and number of high-throughput experiments, this process can become a limiting factor. In an attempt to reduce this effect and still keep the advantages of the XML format, EnCORE services concentrate on the essential data, saving were possible only IDs in the data exchange files with reference information to the original records in the source database. This way the amount of data stored in the exchanged XML files are kept at a minimum, but the records can be linked back to the original resource for complete information retrieval. Additionally projects such as Taverna are developing methods to reduce the network traffic and overcome the limitation presented by large datasets and workflows. One method consists in running a central workflow execution engine at the location where many of the used services of a workflow are hosted. Executing the workflow on it would enable the engine to make full use of the local network capabilities, and traffic from and to the client would be minimized. In implementing standards such as WS-I [2] and being compatible with tools like Taverna, EnCORE benefits from the most advanced efforts in this field.
Development of EnCORE has so far focused on EBI hosted databases, but the flexibility afforded by the generic enXml schema to integrate new data types will facilitate the addition of non-EBI private and public data sources in the future.
EnCORE web services are currently available for PRIDE [3], IntAct, Reactome, UniProt [4], PICR [5], ArrayExpress, and an EnsEMBL-based mapping between AffyMetrix probe set IDs and UniProt accessions. These services provide information on protein identifications, protein interactions, microarray experiments, pathway information, protein annotations, protein identifier mappings and probe set ID to protein identifier mapping. Recent additions to the list of EnCORE web services have been resources outside the EBI, such as the KEGG pathway database [6] or CellMint (http://mint.bio.uniroma2.it/CellMINT/), a protein localization database. We also started to incorporate analysis tools such as g:GOSt [7], a functional profiling tool, where a service is not simply a database lookup, but rather a data processing step. Since such tools can take considerable time to execute, we had to extend the synchronous service call model to an asynchronous model. The later allows a theoretically unlimited service run time. The data submission and immediate result retrieval of the synchronous service invocation are therefore split into three separate parts, the data submission which will immediately return a submission specific ticket number, the status check where a client repeatedly checks the status of a job given by its ticket number and finally the result retrieval of finished jobs. This model is optimal for a workflow environment, since it can be fully automated and does not require manual intervention as other models do which are based on email notification.
The primary recipient of data from EnCORE web services will be EnSUITE, the ENFIN analysis layer. Sample applications using EnCORE web services are available in Java, Perl, Python and Taverna.
The web application EnVISION has been developed as a more end-user friendly interface to the EnCORE web services. It allows any number of services to be applied in any order to any enXml document, and for ease of usability it can create an initial enXml document from a given set of protein identifiers. EnVISION converts the resulting enXml document into a human-readable form using a XSLT script, which can also be used independent from the web application. EnVISION is available at the following Internet address: http://www.ebi.ac.uk/enfin-srv/envision. The possibility to store and share existing workflows is under study.
Recently an enhanced interface EnVISION II has been made public (http://www.ebi.ac.uk/enfin-srv/envision2). This fully-fledged Java Server Faces (JSF)-based web application now offers more functionality and a better result representation than the older EnVISION. It supports multiple datasets to be submitted and run in parallel and for each dataset various information pages are generated allowing a topic centric view of the results. These views can present single web service results in a clear and easy to understand tabular format, but can also provide data that spans more than one web service, like a length distribution graph or a dynamically expandable tree of the GO annotations for all proteins of the final workflow result. Additionally for convenience, these pages provide outgoing links to the source databases to allow a more detailed view of single entries in their original context. For example, the page presenting the result of a protein ID mapping to UniProt will show an overview of the submitted protein IDs and their mapped UniProt accession numbers together with additional information about the protein, such as its name and keywords. For each mapping it will also provide a link back to the original record for that protein in the UniProt website and the full wealth of its information. Other existing pages include views for the Reactome, IntAct and PRIDE services. Work is currently ongoing to refine the documentation and to provide more possibilities to customize the analysis workflow and its parameters.
3 Dry–wet collaborations in practice
Integration is at the core of systems biology, where the study of a system requires associating various disciplines and methods of analysis. From the start, ENFIN has worked as a prototype not only to develop technologies, but also to assess working methods between computational (dry) and experimental (wet) laboratories (Fig. 3). We established various collaborative projects joining multiple biological disciplines with computational scientists and mathematicians, who coordinated their work, to both produce new scientific discoveries and develop adapted bioinformatics tools.
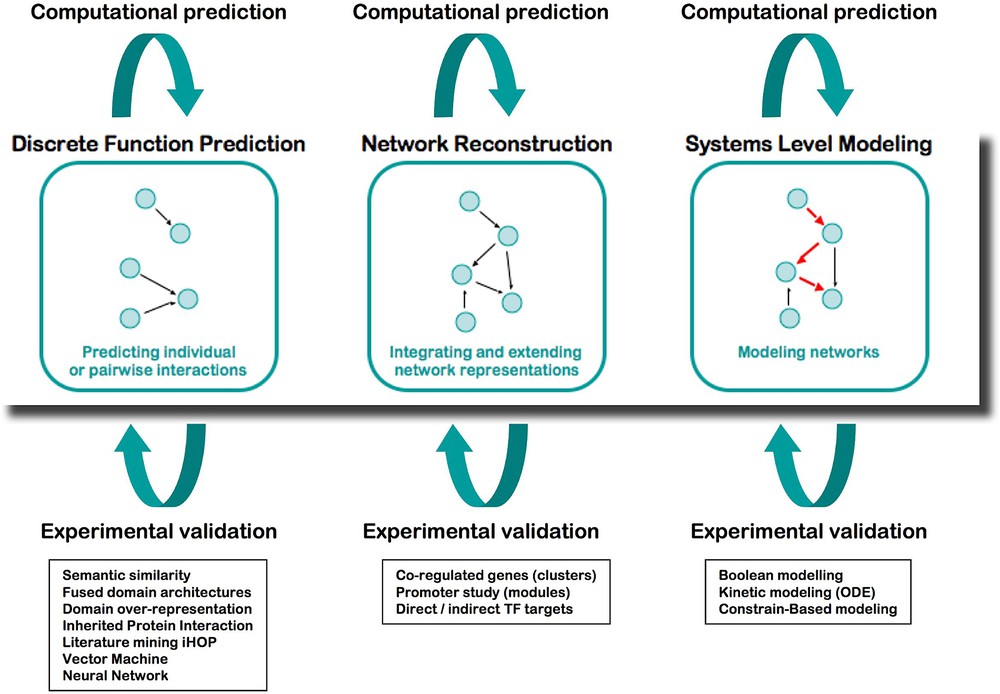
The analysis platform of ENFIN, based on cycling between wet and dry laboratories.
Experiments that inherently handle many data points require their own software and databases specific to the experimental system. Most equipment (e.g. microarray platforms, proteomics, automated microscopes amongst others) comes with its own computer and software bundled with the instrument. The standardization of these computational tools is very variable depending on the maturity of the overall industry and the investment by both the instrumentation Company and early adopter sites. Any laboratory using such equipment will need increasing sophistication in computational methods with personnel who are competent in extracting, moving and troubleshooting datasets in a computational setting. We estimate that between 20 to 30% of the salary resource should be dedicated to close-to-data production informatics. These include mostly databases generation and maintenance tools such as Laboratory Information Management Systems (LIMS). In ENFIN, either wet laboratories hired a dedicated bioinformatician to channel their data into analysis platforms, or established tight collaborations with dry laboratories, where the bioinformatician having developed an analysis method collaborated with each wet lab requesting the service. The ideal situation is obtained when a bioinformatician is embedded within the experimental laboratory and bridges the transfer of data and information with the dry laboratory but provides a critical assessment of some of the computational methods (flaws and advantages).
The computational analysis in ENFIN offers three distinct areas to the scientists:
- – Discrete function prediction focuses on determining individual aspects of function for particular proteins, for example, phosphorylation sites or specific functional sub-types of proteins. Having made large-scale predictions of these discrete functions, this data can then be combined with pathway information to provide specific predictions of sites or functions of interest in the context of pathways. Computational techniques in this area include phosphorylation and glycosylation site prediction, protein localization, protein–protein interaction analysis and sub-family function classification.
- – Network reconstruction focuses on determining or extending pathways using all available information, in particular gene expression levels, cis-regulatory elements, protein–protein interactions and comparative information. Using the genome sequence of a number of organisms, in particular human and mouse we integrate cis-regulatory motifs and pathway information using Bayesian networks. We also use protein–protein interactions and cis-regulatory information to develop statistical methods to find new pathway members. Finally we use comparative mapping from well-established model systems (e.g., S. cerevisiae) to map into more complex systems such as human. In each case we predict likely new members of a particular pathway, and potentially partial information of where these members lie in a pathway.
- – Systems-level modeling focuses on understanding how the components of a given system result in the emergent properties of this system. Three main techniques are applied: flux analysis of metabolic pathways, kinetic modeling of limited parts of metabolic and signaling pathways, and Boolean network modeling of larger signaling pathways. Modeling approaches enable for example to in silico test the effect of the knocking-down or overexpressing a gene or a set of genes in a given network, and provide hints to the biologists to select the most critical components to study in detail. All the computational tools developed during this project are available as stand-alone in the toolbox called EnSUITE, and are progressively adapted to function as webservices that can be incorporated into workflows of the EnCORE platform.
Among the research projects undertaken by ENFIN, integration is seen at two levels: (i) the integration of the bioinformatics analysis results proves more efficient than the use of a single method; and (ii) the integration of different types of data increases the accuracy of computational predictions.
The first research case supplied to the ENFIN pipeline consisted in identifying mitotic spindle proteins from a list of a thousand of proteins initially obtained from mass-spectrometry analysis of purified human mitotic spindles by the group of E. Nigg [8]. The different methods of function prediction developed by the groups of A. Valencia, S. Brunak and C. Orengo gave independent predictions, which were integrated into a single ranked list of potential mitotic spindle proteins. The methods include semantic similarity [9], fused domain architectures and domain over-representation [10–12], inherited protein interaction [13], literature mining [14], vector machine learning and neural networks [15,16]. About 70% of the candidates picked amongst the top of the ranked list were experimentally validated [17].
Another example is the exploration of the regulatory network of human stem cells. Gene expression and ChIP-on-chip datasets obtained from human stem cells or embryonic carcinoma cell lines by the group of J. Adjaye [18] where integrated with existing data from the literature into a database, which was used to derive clusters of co-regulated genes. This approach enabled, by integrating a large amount of datasets of different origins, to identify regulatory modules involved in maintenance of pluripotency in human stem cells (personal communication).
Boolean network modeling has been used by the group of I. Xenarios to identify steady states of the TGF-beta pathway and by applying systematic modifications to the network and discover the key components. Kinetic modeling enabled the quantitative representation of metabolic and signaling pathways: Glucose-mediated insulin secretion by the group of J. Hancock and TGF-beta by the groups of E. Klipp and C. Heldin, respectively [19,20]. This quantitative modeling approach allows a very precise representation of limited molecular systems and offers the possibility to predict the range of values of missing parameters, which could not be assessed experimentally. Models have proven very useful to provide hints to the experimentalists to drive further research.
4 The FuncNet package: A distributed protein function analysis network
FuncNet is a novel open platform for the computational prediction and comparison of protein function (http://www.funcnet.eu). This platform can handle the integration of various computational predictions as seen above with the prediction of mitotic spindle proteins, but now in an automated manner. It is designed to use a reference set of proteins that are known to share a given biological function, to search within a second set of proteins the ones the also share that function. FuncNet works by submitting the same protein sets for analysis by several different prediction algorithms, pooling the results, and deriving overall predictions for the proteins within those sets (Fig. 4). This approach takes into account the wide variety of different kinds of evidence. Its guiding scientific principle is that by aggregating the results from these largely orthogonal algorithms, more statistically powerful and biologically meaningful predictions can be made. The prediction task tackled by each algorithm is that of deciding whether a pair of proteins is ‘functionally associated’, where the exact interpretation of functional association varies between algorithms, and the contributions of all the algorithms can help build an overall profile of the relationship between two molecules. However, by analyzing the results of many such predictions, a variety of different questions can be answered.
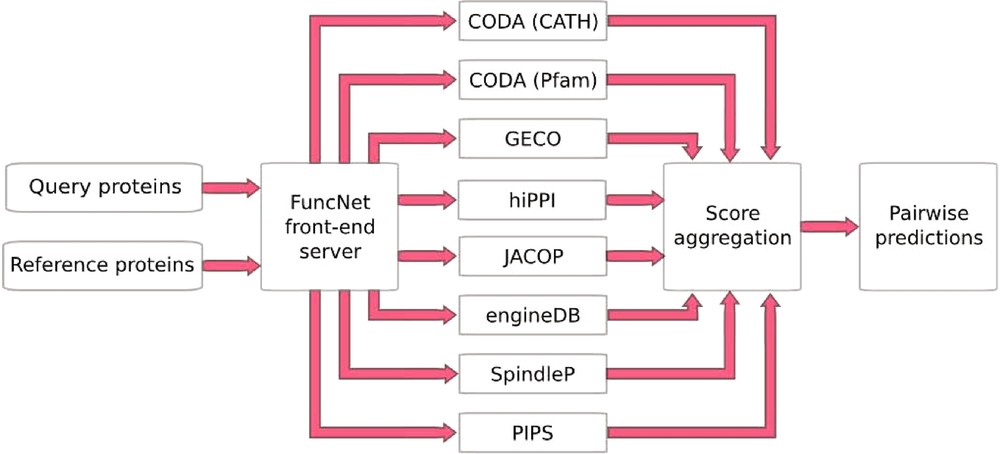
FuncNet. A FuncNet session begins when a user submits two sets of proteins, a query set (under-characterized proteins of interest) and a reference set (proteins with well-characterized function). These two sets are sent to a number of prediction algorithms running in parallel: GECO predicts functional associations based on correlated patterns of gene expression; hiPPI identifies likely protein–protein interactions from domains known to interact in other species; CODA infers functional association from domains found fused together in other species; JACOP performs unsupervised clustering and classification of proteins based on detection of homologous sub-sequences [28]; engineDB is a database of Gene Ontology-based functional similarities between gene products [29]; iHOP contains gene/protein relationships mined from the literature [14]; SpindleP is a neural network trained to identify proteins involved in the formation and function of the mitotic spindle (http://www.cbs.dtu.dk/services/SpindleP/); PIPS uses a naive Bayesian classifier to predict protein–protein interactions [30]. Each predictor returns a set of predicted associations between proteins in the query set and proteins in the reference set, with a p-value for each prediction. FuncNet then applies Fisher's unweighted method to combine all of the predictions for every protein pair, deriving an overall score and p-value for each pair. The final prediction reflects the number of different prediction methods that predicted an association between this pair of proteins and the statistical significance of each prediction.
An additional design principle, which informed the architectural decisions behind FuncNet, is that it should be an open and extensible network using clearly defined and standards-compliant protocols. All communication within the pipeline and with external clients uses common web services standards. This allows its component parts (and its users) to be located anywhere in the world, and to use any software platform. Each of the prediction algorithms accepts the same kind of input data – UniProt primary accessions, currently human-only – and produces the same kind of output data – pairwise predictions of functionally associated protein pairs. This means that the same data format can be used to communicate with each prediction service, with only the location of the predictor changing. There are several benefits to this approach. Firstly, new prediction services can be easily plugged into the network since each satisfies the same service contract. Secondly, service implementation code can be reused between prediction services, enabling early partners in the collaboration to get later joiners up and running. Thirdly, because each predictor exposes a common interface, which is accessible from outside the pipeline, specialized client programs written to directly target individual predictors can be used to submit jobs to any predictor. This means for example that a tool initially designed to retrieve gene expression correlation data from the GECO service could be re-used to extract text-mining results from the iHOP service without modification.
Once the predictors have all completed and the overall scores have been calculated, users or client programs can retrieve the complete score profile for each protein pair. This contains the full set of hits from the predictors, in order to facilitate further data analysis or integration, plus the overall score assigned by FuncNet. An additional score calculation option, currently under development, will use this data to predict which proteins in the query set are functionally related to the reference set as a whole, assuming the user has submitted a consistent reference set representing a well-defined biological system or phenomenon. In addition, queries can be sent directly to the individual prediction services, if a user is only interested in certain kinds of evidence, e.g. text mining or gene expression.
The primary audience for FuncNet is intended to be computational biologists and bioinformaticians, who wish to integrate FuncNet predictions into their data analysis workflows or toolkits, via scientific workbench packages like Taverna, Bioclipse [21] or UTOPIA [22], or by means of programming languages such as Perl or Java.
An important extension under development will integrate FuncNet into the broader EnCORE computational infrastructure, allowing jobs to be submitted to FuncNet via the EnVISION interface, and their results to be integrated with predictions and annotations retrieved from other ENFIN resources. Currently, FuncNet only supports human proteins, since it is limited by the capabilities of its component predictors, but expanding its coverage to other organisms is a high priority.
5 Discussion – conclusion
The ENFIN Network of Excellence, funded by the European Commission until 2011, pioneers the area of data integration by developing tools and methods dedicated to small and middle-sized laboratories.
In the near future, the ENFIN core will be the central provider of data for joint research projects, allowing groups to develop methods that utilize this core infrastructure. Each ENFIN analysis method should become applicable to the core. This will remove a large duplication of effort currently existing in computational groups, which often have to develop bespoke methods to analyze the heterogeneous data for each experimental collaborator they work with. The development of this core infrastructure will enable real two-way communication between bioinformatics and experimental groups. Part of this communication requires both groups to be able to precisely describe the aspects of the biomolecular pathway that they either know (from the experimentalists) or predict (from the computational work). Using the Reactome framework for pathway and IntAct for interaction networks information, we propose to allow storage of more speculative hypotheses, both from experimental and computational work. This aspect will enable integrating speculative data within the EnCORE platform in a near future.
As an important component of a data integration platform, we provide the framework for both the semantic and then syntactic standards around new experimental information. We participate in the international work in biological standards definition and try to adopt emerging standards, for example the community standard controlled vocabulary for the representation of protein modifications PSI-MOD [23].
Another axis in our platform has been the setup of a registry of databases used in ENFIN, which should allow annotating precisely the origin, characteristics and standards of the data they contain and define the availability of an EnCORE-compatible webservice.
Finally, as a parallel research, ENFIN aims at developing critical assessment methodologies to directly and effectively test the accuracy of bioinformatics methods. In collaboration with the coordinators of the DREAM project in the USA (Dialogue for Reverse Engineering Assessments and Methods) [24] we have organized in 2008 the first European conference on assessment of computational methods. Participants of ENFIN along also participate with other researchers to DREAM challenges.
Acknowledgements
The ENFIN (contract number LSHG-CT-2005518254) and the EMBRACE (contract number LHSG-CT-2004-512092) projects are funded by the European Commission within its FP6 Programme, under the thematic area “Life sciences, genomics and biotechnology for health”.
The members of ENFIN are E. Birney (Director of ENFIN) and H. Hermjakob at the European Bioinformatics Institute, United Kingdom (EBI); C. Ouzounis at the Center for Research and Technology Hellas, Greece (CERTH); A. Valencia at the Spanish National Cancer Research Centre, Spain (CNIO); S. Brunak at the Centre for Biological Sequence Analysis, Denmark (DTU); J. Vilo at QureTec, Estonia (QURE); J. Ellenberg at the European Molecular biology Laboratory, Germany (EMBL); V. Schachter at the Genoscope, France; J. Heringa at the Centre for Integrative Bioinformatics at the Free University of Amsterdam, The Netherlands (IBIVU); C.-H. Heldin at the Ludwig Institute for Cancer Research, Sweden (LICR); E. Nigg, E. Klipp and J. Adjaye at the Max-Planck Institute, Germany (MPI); J. Hancock at the Medical Research Council Mammalian Genetics Unit, United Kingdom (MRC MGU); I. Xenarios at the Swiss Institute for Bioinformatics, Switzerland (SIB); D. Schomburg at the Technical University Braunschweig, Germany (TUBS); C. Orengo at the University College London, United Kingdom (UCL); T. Mäkelä at the University of Helsinki, Finland (UH); G. Cesareni at the University Tor Vergata in Rome, Italy (UniTor); G. Barton at the University of Dundee, United Kingdom (UNIVDUN); E. Segal at the Weizmann Institute, Israel (WI).
FuncNet has been developed in collaboration between several groups involved in the ENFIN and EMBRACE European projects, based at UCL, SIB, CNIO, DTU, UNIVDUN, University of Malaga (Spain), Institute for Biomedical Technologies (Bari, Italy).