1. Introduction
Enzyme design seeks to develop biocatalysts with improved “à la carte” characteristics by altering the amino acid sequences of natural enzymes or generating new sequences and tertiary structures. The interest in enzyme design and engineering stems from the unique potential benefits of these catalysts, such as their ability to work efficiently under mild biological conditions while achieving exceptional efficiency, selectivity, and specificity. Enzyme design is intellectually stimulating, serving as a stringent test of our understanding of enzyme stability, folding, evolution, and catalysis.
The process of enzyme design, whether starting from a natural or computationally generated scaffold, involves selecting specific residues for mutation, creating new variants, and applying screening methods to evaluate the desired properties [1]. There are two primary approaches that can be effectively combined: rational design [2, 3, 4] and directed evolution (DE) [5, 6, 7]. Rational design focuses on predetermined key residues, identified through the analysis of multiple sequence alignments (MSAs), structural evaluation of active sites, substrate-binding tunnels, reaction mechanisms, and key intermediates formed during the reaction, using techniques like quantum mechanics, molecular mechanics, molecular dynamics (MD), and Monte Carlo simulations [3, 8]. Rational methods typically target as mutation hotspots active site residues [9, 10] or those involved in the bottleneck regions of tunnels, using tools like CAVER, AQUA-DUCT, and HotSpot Wizard [8, 11, 12]. Inspired by the deep-learning (DL) AF2 approach [13, 14, 15], recognized with the 2024 Noble Prize in Chemistry, many DL techniques have been recently developed for generating new protein sequences and scaffolds, which then are further refined using sequence design methods such as ProteinMPNN [16, 17, 18, 19, 20, 21, 22]. All these different designs generated by means of traditional methods or DL can also be further improved with DE. Recognized with the 2018 Nobel Prize in Chemistry, DE traditionally utilized random mutagenesis but has advanced to integrate bioinformatics [23, 24, 25, 26, 27, 28], rational methods [29, 30], sequence analysis [31, 32], NMR data [33], and high-throughput screening [34], often guided by machine-learning models [35, 36]. The DE approach is particularly powerful for improving low-activity computational enzyme designs [37, 38, 39] and enhancing side activities [40, 41]. Numerous lab-engineered enzymes have been developed over the years, contributing to drug production, biotherapeutics, and fragrance production [42].
One key advantage of DE is its ability to introduce mutations across the entire protein unlike traditional rational design strategies, which often focus on the active site or substrate tunnels for helping substrate binding/product release and/or altering the water content [8, 43]. The DE studies have shown significant activity increases through mutations distant from the active site, which are difficult to predict computationally [6, 44, 45, 46]. This has been observed in various enzyme families, where in many cases mutations occur around 15 Å from the active site [44]. Intriguingly, the impact of mutations on the enzyme turnover (kcat) is not directly correlated to their proximity to the active site, which contrasts with the more deterministic role of active site mutations in specificity [6]. Such regulation of the enzyme catalytic activity by means of distal mutations suggests that allostery (i.e., regulation of catalytic activity by effector and/or protein binding) plays a key role in many proteins [47]. Molecular dynamics simulations have explained how such mutations influence enzyme conformational dynamics and enhance catalytic activity by altering the network of non-covalent interactions [46, 48], thus regulating the flexibility of dynamical elements such as loops or lids gating the active site access [40, 49, 50]. Although computational models can rationalize these changes, predicting which distal mutations will affect activity remains challenging [46, 48, 51]. The insights gained from DE about the role of distal mutations regulating enzymatic function could help develop computational approaches for designing efficient enzymes with natural-like functionality [44].
The influence of distal mutations in enzyme design parallels the allosteric regulation seen in effector binding or substrate transport within heterocomplexes. These mutations can shift the conformational landscape of the enzyme, favoring a subset of conformations that enhance catalysis. Recognizing this similarity, we explored the application of MD simulations for estimating the enzymes’ conformational landscape and extracting key conformationally relevant positions for their use in enzyme design [2, 48]. We developed the Shortest Path Map (SPM) tool [52], which constructs a graph based on mean distances and correlation values between residues as determined from MD simulations. Unlike previous allosteric studies that identified regions of importance [53], the SPM method identifies specific residue pairs that significantly influence enzyme dynamics (Figure 1). This precise identification of key residues is valuable in enzyme design, enabling the creation of targeted libraries for mutagenesis.
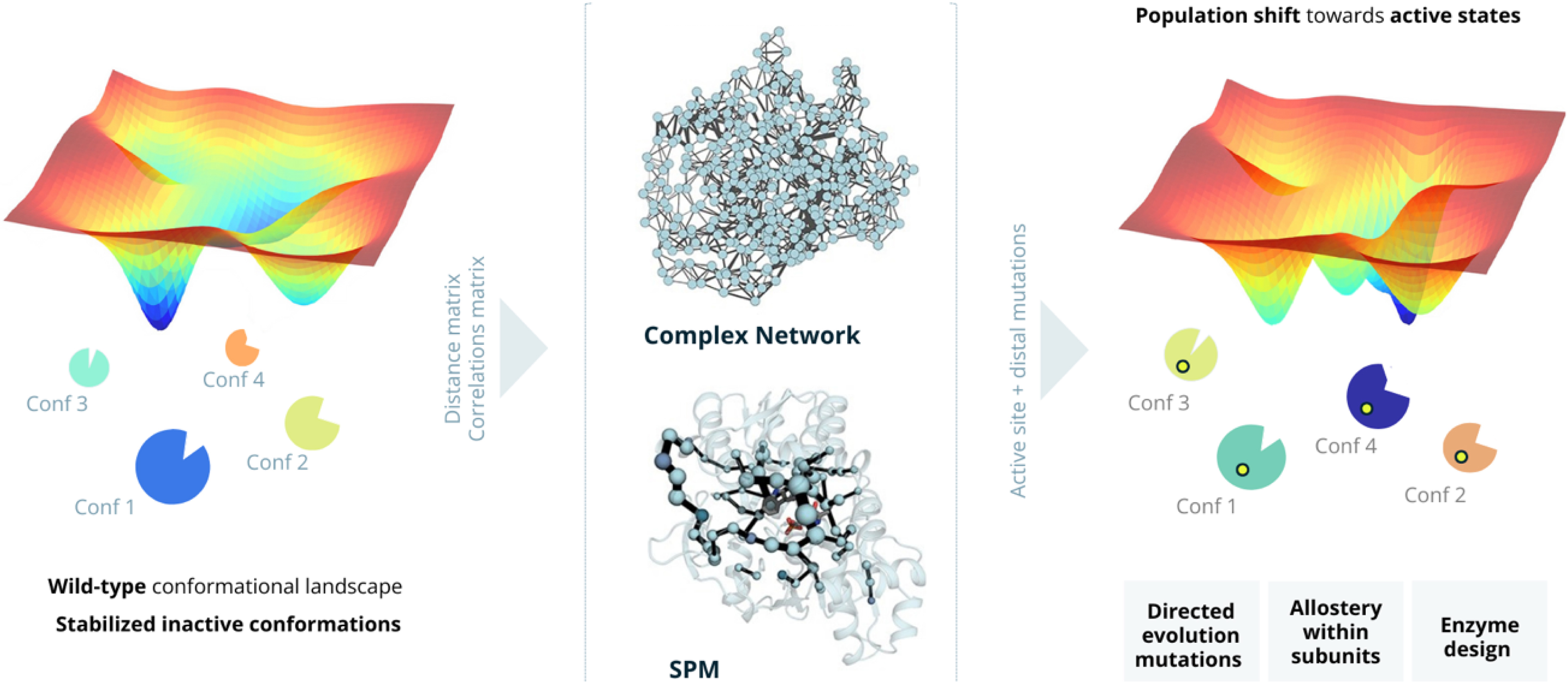
Computational enzyme design based on inducing a population shift on the enzyme conformational landscape towards active states by using the SPM method. Left: The wild-type enzyme conformational landscape features multiple stable states, which are predominantly catalytically inactive (labeled Conf 1, Conf 2, Conf 3, and Conf 4). This represents the enzyme conformational heterogeneity, but the enzyme presents poor catalytic efficiency as active states are poorly populated. Center: By computing the distance and the correlation matrices from the multiple replica MD simulations, the first complex graph network is generated, which is further simplified to obtain the SPM network. SPM identifies potential mutational hotspots that can influence the global conformational landscape and induce a shift in the population distribution towards catalytically active states. Right: By introducing targeted mutations at both active and distal sites, the conformational landscape is reshaped to favor catalytically active states. This approach has a wide range of applications, including directed evolution mutation identification (Section 2.1), elucidation and optimization of allosteric pathways (Section 2.2), and finally, rational enzyme design (Section 2.3). Masquer
Computational enzyme design based on inducing a population shift on the enzyme conformational landscape towards active states by using the SPM method. Left: The wild-type enzyme conformational landscape features multiple stable states, which are predominantly catalytically inactive (labeled Conf 1, Conf 2, ... Lire la suite
2. SPM tool
The SPM builds a graph based on mean distances and correlation values computed from MD simulations, following a strategy similar to the protocol used by Sethi et al. [53]. For each protein residue, a node is created centered on the carbon alpha atom. The next step is to establish edges between node pairs. An edge is formed between pairs of nodes whose Cα atoms remain within 6 Å of each other throughout the MD simulation. The edge distance is then derived from the calculated correlation values, which represent the information transfer along that edge. Residue pairs with higher correlation values (closer to 1 or −1) have shorter edge distances while less correlated pairs (values closer to 0) have longer edge distances. This approach results in a graph with nodes and edges reflecting residue proximity and correlation, which is then simplified as illustrated in Figure 1.
Unlike previous allosteric studies that focus on identifying communities within the first complex graph generated, we compute the shortest path lengths. The SPM identifies which edges of the graph are the shortest (i.e., most correlated) and most frequently used when passing through all the protein residues. After normalizing all edges, only those with the highest contribution are depicted and visualized in the 3D structure. The key advantage of the SPM is that it directly pinpoints critical residues rather than broad regions, making it especially valuable for enzyme design by enabling the construction of small libraries of hotspot positions.
The SPM has proven effective in identifying mutation spots, either within the active site or at distal locations targeted by DE. It has also been applied for elucidating the allosteric regulation existing in multimeric protein structures and more recently for enzyme design. The following sections discuss several cases where SPM has been applied: (1) identification of DE mutation hotspots, (2) rationalization of allosterically relevant residues, and (3) for enzyme design.
2.1. SPM captures DE mutations
The DE approach has become an invaluable strategy in enzyme design engineering, enabling the discovery of enzymes with improved or novel functionalities through iterative rounds of mutation and selection [5, 6, 7]. The key advantage of DE is its ability to identify beneficial mutations across the entire protein, which are often difficult to predict computationally, but it lacks mechanistic insights into how the identified mutations affect enzyme dynamics and conformational changes. Computational models can rationalize DE mutations, but the challenge persists in the prediction of these key mutations.
The SPM’s predictive capabilities have been validated in several key case studies, where it successfully identified mutations that mirrored those obtained through DE. For instance, in the case of mechanistically complex retro-aldolase (RA) enzymes, DE introduced multiple mutations scattered throughout the enzyme, which drastically increased its catalytic activity towards the abiological aldol substrate methodol [23, 45, 48]. The SPM tool could predict most of the mutational hotspots observed in the experimentally evolved enzymes. Specifically, the SPM identified seven of the thirteen mutations introduced by DE while four others were located at adjacent positions and only two were more than six positions away in the sequence (Figure 2a). Of note is that designed RAs are (βα)8-barrel enzymes, which is a fold shared by many enzymes suggesting that the application of the SPM tool might be quite broad. A similar result was observed in the complex homodimeric monoamine oxidase from Aspergillus niger (MAO-N) [49, 54, 55], where DE was employed to increase catalytic activity towards chiral amines and broaden the enzyme’s substrate scope [56]. Five positions were introduced by DE for obtaining the MAO-N D5 evolved variant [57]. The key DE mutations include one active site mutation, one mutation at the entrance tunnel, and three additional distant mutations up to 18–19 Å away from the active site, all of them, despite being computationally challenging to predict, identified by the SPM tool or located at adjacent positions (Figure 2d). The computational evaluation of the conformational changes induced by these mutations by means of Markov state models indicated major changes in beta-hairpin conformation between wild-type and D5 variants. Additionally, the SPM applied to D5 contains the DE positions for generating D9 and D11 variants [57]. This agreement between computational predictions and experimental outcomes underscores the SPM’s effectiveness in identifying functionally relevant distal positions and highlights its ability to predict key residues regulating enzyme activity by analyzing the conformational dynamics of the starting enzyme [44].
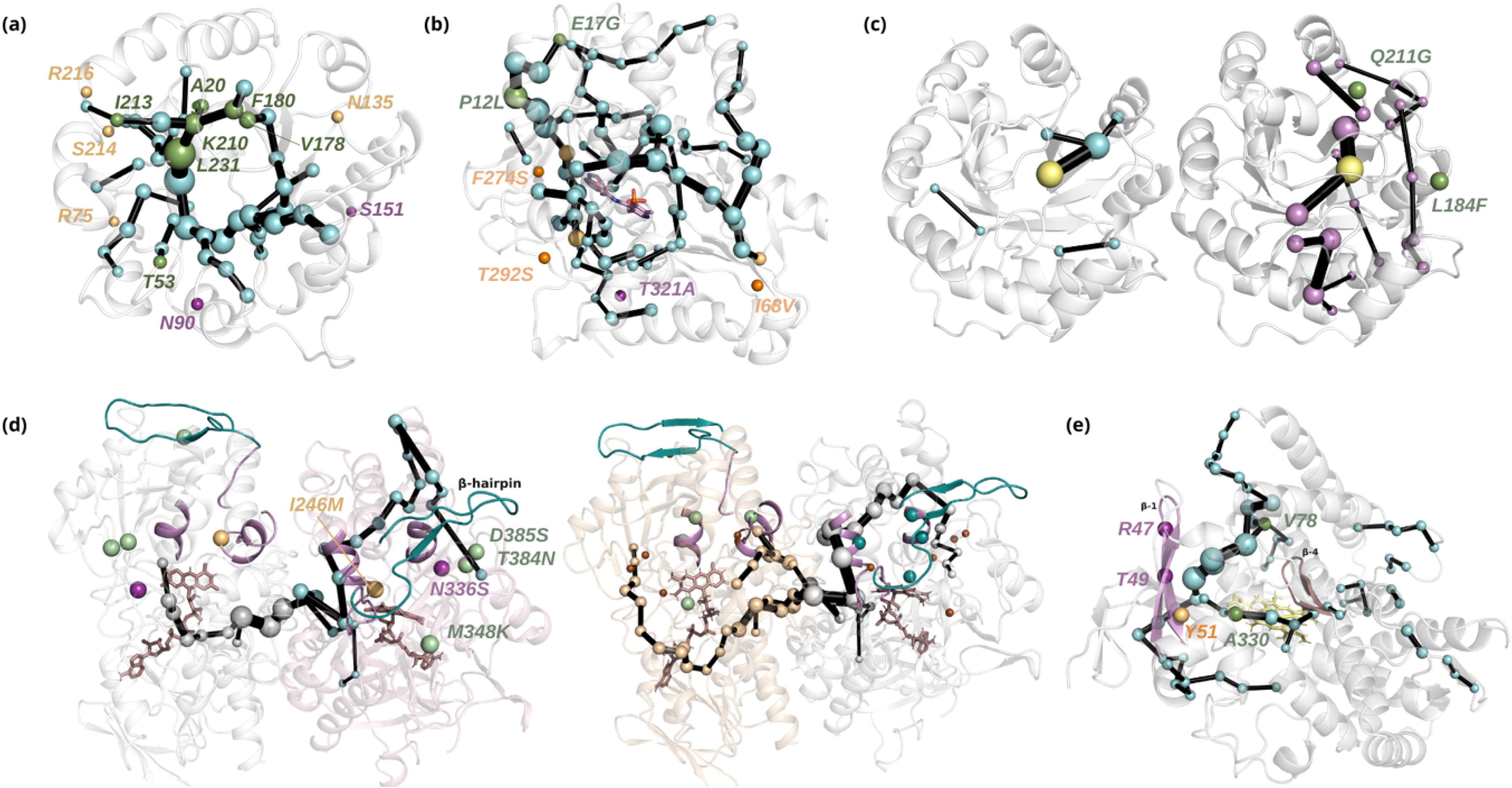
Some examples of the application of the SPM method for capturing DE mutations. (a) SPM is applied to retro-aldolase enzyme. Of the thirteen mutations introduced by DE, seven were directly identified by SPM (green) with an additional four located at adjacent positions (yellow). Only two mutations (purple) were positioned more than six residues away in sequence. (b) Six distal mutations in PfTrpB were introduced by DE to generate a stand-alone PfTrpB-0B2 variant. Of these, five mutations were identified by SPM: two directly captured by SPM (green) and three adjacent to SPM-identified positions (orange). The only distal mutation not captured by SPM is shown in purple. (c) The SPM in 1A53-2 (left) and 1A53-2.5 (right) depicts the interaction network centered on the transition state and extending to the closed state of the protein. The two mutations found by DE are directly involved in the SPM. (d) Right: The five mutations in MAON-WT identified by DE to generate MAON-D5 evolved variant are shown as spheres. The key DE mutations include one active site mutation (purple), one mutation at the entrance tunnel (yellow), and three additional distant mutations (green). Left: The DE mutations on MAON-D5 towards MAON-D9 variant are also captured by SPM and are shown in dark green while previous mutations are shown in brown. The beta-hairpin structure is highlighted in teal, and the entrance loop is represented in violet in both images. The FAD cofactors are depicted as sticks in dark violet. (e) The SPM pathway of the CYP450 enzyme included the previously identified DE mutations V78 and A330 (green). SPM also depicts the long-distance connection between beta-sheet 1 (purple) and beta-sheet 4 (light violet). The key mutation Y51I, identified by SPM as an adjacent position, is shown in yellow. Masquer
Some examples of the application of the SPM method for capturing DE mutations. (a) SPM is applied to retro-aldolase enzyme. Of the thirteen mutations introduced by DE, seven were directly identified by SPM (green) with an additional four located at adjacent ... Lire la suite
The SPM tool was also employed by the Mulholland Laboratory to analyze shifts in dynamical networks within the transition-state (TS) ensemble along the DE of a computationally designed Kemp eliminase, which catalyzes a proton abstraction from carbon by a base [58]. An SPM analysis of the closed states uncovered a dynamical network that connected the active site to more distal regions of the protein, which indicates fine-tuning of its dynamics by remote mutations (Figure 2c). Mutations such as Q211G and L184F, introduced during DE, were also identified by SPM as key contributors to this network, facilitating the global response of the enzyme scaffold to the TS. Mutation Q211G increased flexibility, fine-tuning the dynamic response while L184F enhanced packing by tightening the interactions between neighboring solvent-exposed loops, further stabilizing the TS. These mutations were essential in reducing the structural fluctuations in the TS ensemble, thereby improving catalytic preorganization.
Another striking example of SPM’s utility to identify the mutations introduced by DE comes from work on the heterocomplex tryptophan synthase from Pyrococcus furiosus (PfTrpS). The TrpB complex, a subunit of the tryptophan synthase complex (TrpS), was subjected to DE to create an efficient stand-alone enzyme variant independent of the alpha subunit (TrpA) with improved catalytic activity [59, 60]. The evolved variant, PfTrpB-0B2, contained six distal mutations that restored the enzyme’s ability to access essential conformational states in the absence of its TrpA protein binding partner [61, 62] (Figure 2b). Two of the distal mutations, P12L and E17G, introduced by DE are located near the TrpA–TrpB interface. Another mutation, F247S, is found in a known tunnel while T292S is situated in one of the loops close to the essential COMM domain that covers the active site where the pyridoxal phosphate cofactor is located. Additionally, two more mutations, I68V and T321A, are present in more remote regions of the enzyme. Of the six mutations in PfTrpB-0B2, the first two were directly predicted by SPM and three were making persistent non-covalent interactions with SPM-identified positions. Mutation T292S, which significantly enhanced enzyme activity, modulated COMM domain closure via interactions with D300, supporting SPM predictions. The only residue not captured by the SPM is T321, which is considered to play a minor role in COMM domain conformational dynamics. The comparison of the free energy landscapes between the wild type and the evolved PfTrpB-0B2 indicated that these distal mutations recovered the conformational heterogeneity observed for the wild type when forming complexes with TrpA [61]. This study highlights the potential of the SPM in identifying key residues for improving enzyme function, offering a powerful tool for targeting allosterically regulated systems.
The SPM was also applied to study the epistatic effects and conformational dynamics in the stepwise evolution of a cytochrome P450-BM3 monooxygenase engineered for regioselective and stereoselective hydroxylation of a steroid [63]. The previously identified DE mutations V78 and A330 were successfully included in the SPM pathway of the parent enzyme (Figure 2e). Additionally, combinatorial saturation mutagenesis revealed that the mutant R47I/T49I/Y51I/F87A exhibited improved activity compared to the wild type, achieving 94% 2β-selectivity and 67% substrate conversion. Computational analysis showed that while mutations R47I and T49I alone did not affect selectivity, the Y51I mutation shifted substrate orientation, increasing 2β-selectivity.The SPM successfully identified the Y51 position providing the key residue responsible for both activity and selectivity. Furthermore, the SPM pathway detected a long-distance communication network between β4 and β1, involving positions R47, T49, and Y51.
These selected examples show that the SPM method is a valuable computational tool for capturing the conformational effects of mutations introduced by DE. By pinpointing key residues and pathways that regulate conformational transitions, the SPM can be, in principle, used to enhance the efficiency of enzyme engineering efforts by predicting conformationally relevant positions not strictly located at the active site. The successful application of the SPM in enzymes like RA, monoamine oxidase, tryptophan synthase, Kemp eliminase, and cytochrome P450 illustrates its potential to complement and rationalize DE approaches, accelerating the development of enzymes with optimized functionalities through a deeper understanding of their conformational landscapes.
2.2. SPM identifies allosteric pathways
Allosteric regulation is a fundamental process in which distant residues within a protein communicate to regulate its activity. This long-range communication allows proteins to respond to various signals, adjusting their function accordingly. Understanding the specific pathways through which allosteric signals travel is crucial to enzyme design and for understanding how mutations alter protein function. The SPM has proven effective in mapping allosteric pathways in key proteins, offering insights into how mutations can alter their regulation and function. For instance, the SPM was applied to 5 μs atomistic MD simulations of the K-Ras4B protein embedded in a phospholipid membrane to investigate its allosteric regulation [64]. Allosteric regulation in K-Ras, particularly the oncogenic K-Ras4B, is key to its function in cellular signaling. K-Ras switches between an active GTP-bound state and an inactive GDP-bound state with allosteric changes in key regions of the G domain (switches I and II), enabling effector recruitment and signal transduction. Mutations, especially in Gly12 and Gly13, can disrupt this regulation, locking K-Ras in an active state and driving cancer progression [64]. The SPM identified a prominent allosteric pathway starting at the hypervariable region (HVR), a near-membrane region that might be receiving allosteric messages and transferring them to the major hub, located on helix α5, loop α3–β5, and the N-terminus of sheet β5 (Figure 3a). Branching out to key areas in the G domain, the allosteric route extends to regions crucial to K-Ras function, including the P-loop, Thr58, and Ala59 on switch II and Thr35 on switch I. The analysis of the allosteric communication existing in K-Ras4B was also assessed by means of other measures: distance fluctuation analysis [65, 66], anisotropic thermal diffusion [67], and dynamical non-equilibrium simulations [68], which provided complementary information on how allostery is transmitted when hydrolyzing GTP [64]. The SPM therefore provided useful insights into K-Ras allostery that help in identifying potential allosteric sites for therapeutic targeting, advancing drug design efforts for this protein.
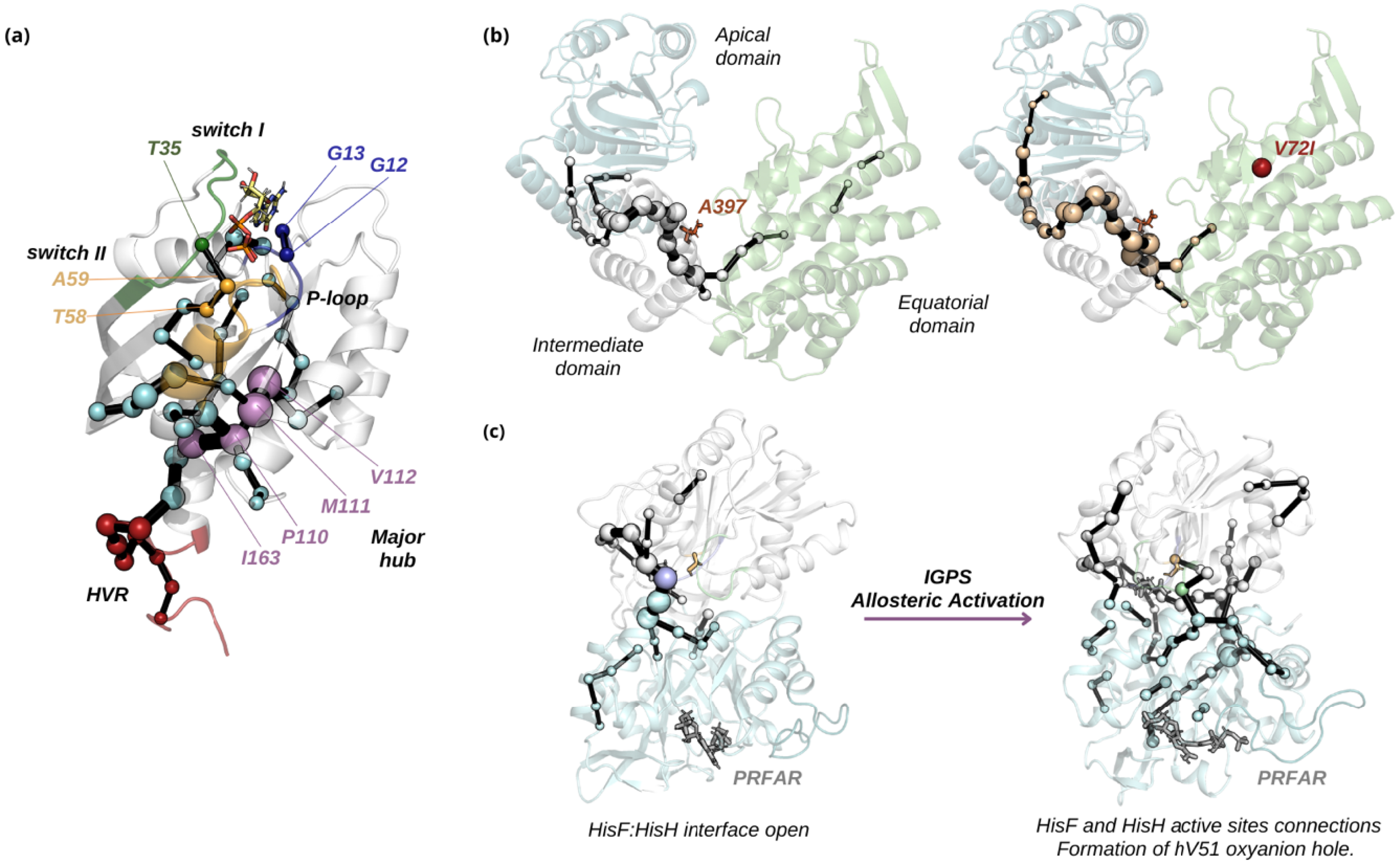
Some examples of the application of the SPM method for identifying allosteric networks. (a) K-Ras4B: SPM reveals the allosteric network connecting the membrane-embedded HVR region (dark red) to the central hub (violet), which extends to switch II (orange) and further connects to switch I (green). Notably, the critical mutations Gly12 and Gly13 in the P-loop (blue) are also captured by SPM. (b) Hsp60: The allosteric network links the equatorial domain (wheat), intermediate domain (white), and apical domain (dark red). Introducing the V72I mutation disrupts this network, disrupting the communication between the equatorial domain and the active site, represented by residue A397 in dark orange. (c) IGPS: SPM analysis shows the progression of IGPS from the open HisH–HisF interface to an allosterically active state, where multiple pathways connect HisF and HisH active sites, facilitating the formation of the hV51 oxyanion hole. Masquer
Some examples of the application of the SPM method for identifying allosteric networks. (a) K-Ras4B: SPM reveals the allosteric network connecting the membrane-embedded HVR region (dark red) to the central hub (violet), which extends to switch II (orange) and further connects ... Lire la suite
In a recent study, the allosteric regulation mechanism of the human mitochondrial heat shock protein (Hsp60), which is responsible for controlling proteostasis, is elucidated using the SPM tool [69]. This study examines the effect of V72I point mutation, linked to hereditary spastic paraplegia SPG13, which is characterized by progressive weakness and spasticity of the lower limbs. An SPM analysis was performed on MD simulations of both the wild-type (M WT) and mutant (M V72I) Hsp60 monomers (Figure 3b). The results indicate that the extensive interdomain allosteric communication present in the wild-type monomer is entirely disrupted in the V72I mutant, leading to decreased efficiency in communication across the intermediate domain, which contains the critical catalytic residue Asp 397. By mapping the allosteric network in the Hsp60 monomer, this work offers valuable insights into the pathogenic effects of the V72I mutation, demonstrating how specific distal mutations can alter protein function and contribute to disease and highlighting the potential applicability of SPM in rationalizing allosterically regulated mechanisms.
Imidazole glycerol phosphate synthase (IGPS) enzyme has been a model for studying allosteric regulation [70, 71, 72]. The enzyme IGPS consists of two subunits, HisH and HisF, with HisH catalyzing glutamine hydrolysis and HisF delivering ammonia through an internal tunnel. Bound 30 Å from the HisH active site, PRFAR acts as an allosteric effector, enhancing glutaminase activity by 4500-fold [73]. In this case, a time-evolution Shortest Path Map (te-SPM) analysis is applied to MD simulations, specifically by examining accelerated MD simulations in 600 ns time intervals, uncovering the progression of allosteric activation [74] (Figure 3c). Initially, correlated motions are limited to the HisH subunit and the HisF–HisH interface. However, as the te-SPM analysis progresses, it reveals concerted motions that are activated upon productive closure of the HisF–HisH interface, extending throughout the entire HisF subunit, the interdomain region, and the HisH active site, leading to the formation of the hV51 oxyanion hole. This dynamic process aligns with μs–ms motions observed by NMR and suggests that IGPS allosteric activation follows a “violin model” of dynamics-based allostery, similar to what is found in some protein kinases [75]. The truncated SPM approach for the time-evolution analysis can be also used to decipher the molecular basis of allosteric mechanisms in related allosterically regulated enzymes.
All the provided examples support the idea that the SPM tool is a useful strategy for uncovering the intricate mechanisms of allosteric regulation in proteins by identifying critical pathways of residue communication. So far, the SPM has offered deep insights into how proteins modulate their functions and how mutations can disrupt this regulation. Therefore, the SPM serves as a promising tool for rational design of targeted therapeutics and enzyme engineering.
2.3. Conformationally driven enzyme design with SPM
The application of the SPM in different unrelated enzymes demonstrates that analyzing an enzyme conformational ensemble can pinpoint key positions that significantly impact its conformational dynamics. Notably, the SPM identifies positions throughout the enzyme, not just in the active site, allowing for the prediction of distal mutations. This suggests a strategy for conformationally driven enzyme design involving the reconstruction of the enzyme conformational landscape, detection of critical positions using the SPM, and identification of the specific amino acid substitution for each mutation through experimental or computational methods.
We engineered a stand-alone functional TrpB by combining the SPM with ancestral sequence reconstruction (ASR) [76]. Interestingly, ASR revealed that the last bacterial common ancestor (LBCA) is allosterically inhibited by TrpA, thus presenting high stand-alone activity [77, 78]. Over the course of evolution, this inhibition shifted towards allosteric activation; specifically ANC3 TrpS is the first ancestral enzyme displaying allosteric activation by TrpA. By coupling SPM and sequence conservation analysis between LBCA and ANC3 TrpB, six key positions were rationally identified, leading to the SPM6 variant, which achieved a sevenfold increase in catalytic activity [76] (Figure 4a). This improvement of activity obtained by the experimental testing of a few (<5) variants was similar to that obtained after multiple rounds of DE in PfTrpB that required the generation and screening of more than 3000 variants [59]. Two additional variants were developed that further validate the approach: SPM3, based on ANC2 TrpB, involved three mutations; SPM8, derived from an additional SPM analysis of ANC3 TrpS instead of TrpB, added two allosterically relevant positions [76]. While SPM8 achieved similar catalytic improvement to SPM6, SPM3 showed a smaller enhancement, which was expected from the reduced number of mutations. An additional comparison of SPM with MSA revealed the complementarity of the two methods, as they identified distinct mutation sites with only one shared residue mutation between them [76].
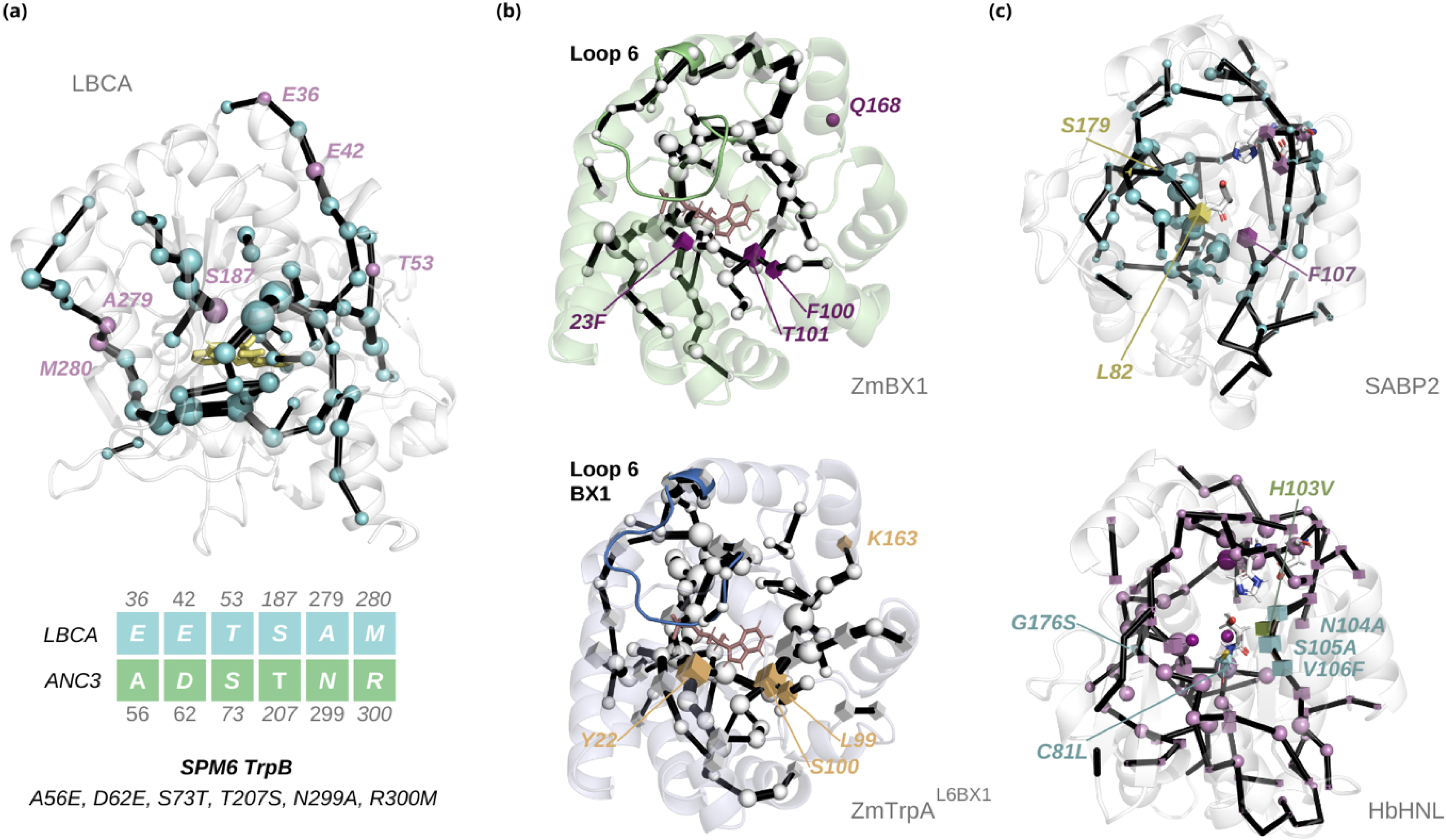
Some examples of the application of the SPM method for rational enzyme design. (a) Designing stand-alone TrpB variants based on the ancestral ANC3-TrpB scaffold: SPM identifies a set of conformationally relevant positions in LBCA-TrpB (violet), which after sequence analysis conservation with ANC3-TrpB residues led to the SPM6 TrpB design exhibiting a sevenfold increase in catalytic activity with respect to ANC3 WT. (b) Designing stand-alone TrpA enzymes: the comparison of the SPM graphs computed considering IGP-bound catalytically activated states of the stand-alone ZmBX1 (top) and ZmTrpA-L6BX1 (bottom) allowed the generation of the ZmTrpA-SPM4-L6BX1 variant. The selected positions mutated are shown in purple for ZmBX1 and in yellow for ZmTrpA-L6BX1. ZmTrpA-SPM4-L6BX1 presents a 178-fold higher stand-alone catalytic efficiency compared to ZmTrpA. (c) Designing efficient esterases from hydroxynitrile lyases: the introduction of the three obvious active site mutations for providing an oxyanion hole pocket to HbHNL and one additional stabilization mutation yielded HNL3V, which shows poor esterase activity. The SPM analysis applied to the reference SABP2 esterase (top) and HNL3V (bottom) is depicted, with spheres indicating residues shared between both proteins and cubes marking differing residues. Four/five SPM positions were selected for mutagenesis, yielding HNL7 and HNL8 that both exhibit a 300-fold enhancement in esterase catalytic activity as compared to HbHNL. The incorporation of evolutionary information into SPM provided HNL7T, which displays a 1450-fold increase in catalytic efficiency, thus surpassing that of the SABP2 esterase taken as reference. Masquer
Some examples of the application of the SPM method for rational enzyme design. (a) Designing stand-alone TrpB variants based on the ancestral ANC3-TrpB scaffold: SPM identifies a set of conformationally relevant positions in LBCA-TrpB (violet), which after sequence analysis conservation ... Lire la suite
In another recent study also focused on a tryptophan synthase, the SPM tool was employed to enhance the stand-alone activity of Zea mays TrpA (ZmTrpA) by identifying key conformationally relevant positions [79] (Figure 4b). Unlike TrpB, where a shift in allosteric regulation was observed along its phylogenetic tree, LBCA TrpA was already allosterically activated by TrpB [77, 78]. This made more difficult the identification of positions for stand-alone activity using traditional methods like MSA. However, the structurally similar stand-alone enzyme ZmBX1 [80] from the secondary metabolism of maize served as a model for designing an enhanced TrpA variant [79].
The initial approach involved engineering the ZmTrpA to the ZmTrpA-L6Bx1 variant by switching the L6 loop with the L6 found in ZmBX1 [80], as L6 closed conformation observed in ZmBX1 was reported to be crucial to TrpA stand-alone activity [79]. This resulted in a significant increase in catalytic turnover (kcat) but at the expense of increasing KM, indicating the need for additional improvements to achieve better catalytic efficiency [80]. To further enhance the stand-alone activity of ZmTrpA, the SPM method was applied to identify key conformationally relevant positions by comparing the intramolecular pathways of ZmTrpA-L6BX1 and ZmBX1 at the catalytically activated state. The motivation for specifically targeting the IGP-bound catalytically activated state was the experimental observation that the conformational transition to achieve the catalytically active state is rate-determining [81]. By comparing the generated SPM for the starting ZmTrpA-L6BX1 scaffold and ZmBX1, a subset of non-conserved SPM-identified positions was selected for mutation, leading to the ZmTrpA-SPM4-L6BX1 variant, which exhibits a 178-fold improvement in catalytic efficiency towards IGP cleavage [79]. With this study, the power of SPM in identifying both active site and distal mutations to boost stand-alone activity is highlighted and paves the way for enzyme design in those enzymatic systems where conformational change is rate-limiting.
The SPM tool was also successfully applied to predict mutations that converted hydroxynitrile lyase (HbHNL) from the rubber tree into an efficient esterase by comparison with tobacco esterase (SABP2) [82] (Figure 4c). Both enzymes are present in the α,β-hydrolase fold and despite the fact that they share the same catalytic Ser–His–Asp triad, they exhibit very different forms of reactivity. Initial efforts to engineer HbHNL into an esterase produced the HNL3V variant, which focused on the three obvious active site mutations (T11G, E79H, K236M) to introduce the oxyanion hole residues and to replace the polar site by a hydrophobic site as observed in SABP2. However, these three obvious mutations only showed limited esterase activity. To further enhance the esterase activity, SPM analysis of HNL3V, a stabilized version of HNL3 for experimental purposes, and SABP2 revealed five additional positions outside the active site, which were connected to the catalytic residues and could potentially affect the oxyanion hole and the catalytic Asp preorganization. By replacing these positions with those from SABP2, the HNL6V, HNL7V, and HNL8V variants were created, enhancing esterase activity by 300-fold. The SPM-guided mutations targeted correlated movements essential for catalysis by removing unwanted movements in HNL3V and adding the missing ones from SABP2. To this end, the residues in HNL3V were replaced with the corresponding ones from SABP2. Notably, most of the engineered mutations were outside the active site, demonstrating the ability of the SPM to identify distal residues contributing to catalytic function.
The combination of SPM analysis with an MSA of esterases resulted in the identification of the most effective variant, HNL7TV. Among the mutations tested, the N104A substitution produced the largest increase in turnover rate (kcat). However, sequence alignment revealed that most esterases naturally have a threonine at this position rather than an alanine. Introducing the N104T substitution led to the best overall variant with approximately twice the catalytic efficiency (kcat/KM) of SABP2, thus increasing the esterase catalytic efficiency of the starting HbHNL scaffold by 1450-fold. Despite this impressive improvement, the turnover rate (kcat) of HNL7TV remained 13-fold lower than SABP2, indicating a need for further refinement. Nevertheless, the dramatic increase in catalytic efficiency demonstrated the ability of the SPM to predict which residues outside the active site contribute to catalytic activity [82].
3. Conclusions
Recognizing the high similarity between allosteric processes and the effect exerted by distal active site mutations on the enzyme catalytic activity, we hypothesized that the computational identification of allosteric networks could bring interesting insights into enzymatic function and design [44]. To that end, we developed the SPM method [44, 52], which identifies a set of conformationally relevant positions based on the application of graph theory to the distance and correlation matrices computed from MD data. Over the years, we (and others) have applied the SPM to identify conformationally relevant positions and compared those with residues mutated in DE experiments [2, 44, 48, 58, 63]. Interestingly, in many different enzyme classes and families, the application of SPM has revealed that some of the DE mutations are introduced in conformationally relevant sites, thus suggesting its potential application for enzyme design.
The SPM has also been used for elucidating the intricate mechanisms of allosteric regulation in different enzymatic and protein systems [64, 69, 74]. The SPM allosteric networks identify critical pathways of residues, some of which are experimentally known to have a large effect on enzyme/protein regulation. We have also tested the applicability of SPM, especially if combined with ASR and/or MSA, to rationally design new improved enzymes with enhancements in catalytic efficiency that range from 7- to 1450-fold [76, 79, 82]. Altogether these examples show the potential of the SPM method for understanding the effect of distal mutations into the enzyme conformational dynamics and how they affect enzymatic function. More importantly, integrating SPM with other computational techniques such as MSA and ASR corresponds to a successful approach for rational enzyme design.
Declaration of interests
The authors do not work for, advise, own shares in, or receive funds from any organization that could benefit from this article, and have declared no affiliations other than their research organizations.