1 Introduction
The developments of space geodesy have allowed the establishment of global observing systems. Doppler Orbitography and Radio-positioning Integrated by Satellite (DORIS) (Barlier, 2005; Willis et al., 2006) is one of these systems, as Satellite Laser Ranging (SLR) (Pearlman et al., 2002), Very Long Baseline Interferometry (VLBI) (Schlueter and Behrend, 2007; Schlueter et al., 2002) and Global Positioning System (GPS) (Beutler et al., 1999; Dow et al., 2009). The large number of measurements collected by these systems permit today to represent the displacement of the ground stations in terms of coordinates time series.
The analysis of coordinate time series allows one to better apprehend the temporal variability of the physical phenomena (deformations of the Earth's crust, mass transfers, geodynamic local phenomena, etc.). Pioneering studies were undertaken in the late 1990s in the GPS system by King et al. (1995), Langbein and Johnson (1997), Zhang et al. (1997), Mao et al. (1999) and Williams et al. (2004). These authors implemented standard statistical methods, e.g. spectral density or maximum likelihood estimation (MLE), separately on the North, East and Up components (NEU) of the time series, in order to determine the noise characterization (type and level) of each component. In the DORIS system, to characterize the type and level of noise in weekly coordinates (NEU) time series, Le Bail (2006) and Feissel-Vernier et al. (2007) have used the Allan variance (Allan, 1966), while Williams and Willis (2006) have employed the MLE method. The main advantage of MLE method is to determine the type of noise (white noise, flicker noise and random walk noise) and its amplitude. However, its limitation is that several combinations should be tested, since the method does not identify directly the type of noise (iterative process), while the Allan variance can directly and rapidly detect the type and level of noise, but requires that the time series should be regular (gaps should be filled) and stationary (elimination of trend and all periodic components). This study is a contribution to these methodological developments. We apply the wavelet and the Singular Spectrum Analysis (SSA) methods on the weekly position time series of DORIS stations to extract the noise from the signal, in order to provide certain information useful to later geodynamic interpretations such as the trend, seasonal components and residual noise contained in these time series.
The wavelet and SSA are well-known methods already applied in a variety of disciplines (geophysics, meteorology, biosciences, economics, etc.). The wavelet technique (Daubechies, 1992) permits to study the signal at different resolutions using functions (wavelets) well localized in both physical space (time) and spectral space (frequency), generated from each other by translation and dilation which is well suited for investigating the temporal evolution of periodic and transient signals. The SSA (Broomhead and King, 1986) technique permits to decompose the original time series into the sum of a small number of independent and interpretable components such as a slowly varying trend, oscillatory components and a structureless noise.
The article is organized as follows. For the convenience of the reader we briefly recall the wavelet and SSA approaches in Sections 2 and 3, respectively. Section 4 describes the data analysed in this study. Results and discussion are reported in Section 5, and finally the conclusion is drawn in Section 6.
2 Wavelet transform
A wavelet is a function ψ(t) of L2 (R) (L2(R): continuous functions of real variable and square integrable) which satisfies the following properties (Daubechies, 1992; Mallat, 1999; Ranta, 2003):
- • zero mean:
; - • unitary norm:
; - • centred around t = 0.
We introduce the factor of translation (or position) u and the scale factor (or dilatation) s; therefore, we define a family of functions ψu,s called wavelets, deriving all of the mother wavelet ψ, such as:
(1) |
The Wavelet transform can be defined as the projection of the signal X(t) on the basis of wavelet functions ψu,s:
(2) |
The original signal can be reconstructed from its wavelet coefficients WT(u,s) with consideration that the wavelet ψ checks the admissibility condition (Daubechies, 1992; Holschneider, 1998):
(3) |
The inverse wavelet transform uses the standardization coefficient Cψ given by:
(4) |
2.1 De-noising steps
The majority of wavelet algorithms use a decimated discrete decomposition of the signal (Daubechies, 1992; Holschneider, 1998; Mallat, 1989). This decomposition has the characteristic to be orthogonal and to concentrate information in some great wavelet coefficients. The de-noising idea is to conserve only the greatest coefficients and put the others (corresponding to the noise) at zero before reconstruction of the signal.
Assume that the observed data vector X = [X1, X2,…, XN]t is given by:
(5) |
Let W(.) and W−1(.) denote the forward and inverse discrete wavelet transform operators. Let D(., λ) denote the de-noising operator with threshold λ.
The de-noising procedure proceeds in three steps:
- • decomposition: choose a wavelet and choose a level l. Compute the wavelet decomposition of the signal X at level l; W = W(X);
- • thresholding of the wavelet coefficients: Z = D(W,λ);
- • reconstruction: from the coefficient thresholding, one reconstructs the signal: S = W−1(Z).
2.2 Strategies of thresholding
Donoho and Johnstone (Donoho, 1995; Donoho and Johnstone, 1994) propose two types of thresholding functions denoted Tλ:
- • hard thresholding:
- • soft thresholding:
2.3 Determination of the threshold
The thresholding method suggested in this work is the VisuShrink (Donoho and Johnstone, 1994) based on the mean square error minimization. This method proposes a universal threshold λ, which depends only on the number of measurements N and the noise variance σ2. It is used when the noise is independent and identically distributed according to a centred normal law; its value is given by:
(6) |
The noise variance σ2 can be calculated by a robust estimator from the median Med of the absolute values of the wavelet coefficients at the first level of decomposition as follows:
(7) |
3 Singular spectrum analysis
The SSA method allows one to extract significant components from time series (trends, periodic signals and noise) (Broomhead and King, 1986; Ghil et al., 2002; Vautard et al., 1992). The method is based on the computation of the eigenvalues and the eigenvectors of a covariance matrix C formed from the time series {Xt, t = 1,…, N} and the reconstruction of this time series based on a number of selected eigenvectors associated with the significant eigenvalues of the covariance matrix. The algorithm of SSA includes the following steps.
3.1 Step (1): choice of the embedding dimension M
The time series {Xt, t = 1,…, N} is embedded into a vector space of dimension M. The embedding dimension M must be sufficiently long to include the assumed periodicity of the time series without exceeding half of the length of the time series.
3.2 Step (2): computation of the M × M covariance matrix C
Computation of the M × M covariance matrix C given by:
(8) |
3.3 Step (3): study of the eigenvalues of the covariance matrix C
The M eigenvalues of the covariance matrix C once determined are ordered by decreasing value. Each eigenvalue λk gives the Partial Variance (PV), given by Eq. (9), of the original time series in the direction specified by the corresponding eigenvectors Ek.
(9) |
If we arrange and plot the ordered eigenvalues, one can often distinguish an initial steep slope, representing the true signal, and a (more or less) “flat floor” representing the noise (Vautard and Ghil, 1989). Thus, the theory of the SSA allows one to conclude that (Le Bail, 2004):
- • the signal has a trend if the diagram contains an isolated eigenvalue;
- • the signal is periodic if there are two close eigenvalues that have the same dominant frequency;
- • the small eigenvalues constitute the noise of the signal.
3.4 Step (4): projection of the original time series onto the k-th eigenvectors and its reconstruction
By the eigenvectors {Ek, 1 ≤ k ≤ M}, called Empirical Orthogonal Functions (EOFs), we can construct the time series of length N′ (N′ = N – M + 1) given by:
(10) |
Ak(t) called the k-th principal component (PC). It represents the projection of the original time series onto the k-th EOF (with 1 ≤ k≤ M). The sum of the power spectral of the PC is identical to the power spectral of the time series X(t) (Vautard et al., 1992) and therefore, we can study separately the spectral contribution of the various components.
The PCs, however, have length N′, not N, and do not contain phase information. In order to extend the time series to length N, it is necessary to choose a subset of EOFs on which the reconstruction is based, the associated PCs are combined to form the partial reconstruction Rк(t) of the original time series X(t).
(11) |
These series Rк(t) of length N are called the reconstructed components (RCs). They have the important property of preserving the phase of the time series; therefore, X(t) and Rк(t) can be superimposed. No information is lost in the reconstruction process, since the sum of all individual RCs gives back the original time series.
4 Data used
For this study, we have used the weekly position residuals of DORIS stations (solution ign09wd01) in STation Coordinate Difference (STCD) format (Noll and Soudarin, 2006), available on the International DORIS Service (IDS) (Willis et al., 2010a) website at http://ids-doris.org/network/ids-station-series.html.
The data are provided by Institut géographique national/Jet Propulsion Laboratory (IGN, France/JPL, USA) (Willis et al., 2010b), using the GIPSY-OASIS II software package (Zumberge et al., 1997), referred to ITRF2005 (Altamimi et al., 2007) and expressed in the local geodetic reference frame (dN: North component, dE: East component and dH: Vertical component). The observing satellites are SPOT series from 2 to 5, TOPEX/Poseidon, Envisat, Jason-2 and Cryosat-2. The Jason-1 data are not used in the ign09wd01 solution, as these data are affected by a large error related to the extreme and unexpected sensitivity of the on-board receiver to radiation over the South Atlantic Anomaly (Lemoine and Capdeville, 2006; Willis et al., 2004). This DORIS/IGN solution is the latest one from this group. It was prepared in for the IDS combination (Valette et al., 2010) in view of ITRF2008 (Altamimi et al., 2011) and includes the latest available data processing strategies to mitigate errors in solar radiation pressure models (Gobinddass et al., 2009a, 2009b) as well as high-frequency errors in atmospheric drag corrections (Gobinddass et al., 2010).
We should point out that DORIS time series in STCD format are also provided by two other analysis centres: Institute of Astronomy Russian Academy of sciences (INASAN) (Kuzin et al., 2010) and Laboratoire d’études en géophysique et océanographie spatiales/Collecte et localisation par Satellite (LEGOS/CLS) (Soudarin and Cretaux, 2006). We chose to use the IGN/GPL solution (ign09wd01) because it is the most recent one and because it is regularly updated (on average every week).
The time series selected in this application, as seen in Table 1 and Fig. 1, are the coordinate residuals of 18 stations with data gaps less than 3 months and their observing period is longer than 4 years. For this study, we had to remove some outliers, which are not included in the tolerance interval, given by:
Stations DORIS sélectionnées dans cette étude.
Acronym | Site | Country | Latitude (°) |
Longitude (°) |
Data span |
ADFB | Terre Adélie | Antarctica (French base) | –66.67 | 140.00 | 2008.2–2011.3 |
ARFB | Arequipa | Peru | –16.47 | –71.50 | 2006.6–2011.3 |
CADB | Cachoeira Paulista | Brazil | –22.68 | –45.00 | 2004.3–2011.3 |
DIOB | Dionysos | Greece | 38.01 | 23.93 | 2006.4–2011.3 |
DJIB | Djibouti | Djibouti | 11.53 | 42.85 | 2006.6–2011.3 |
HBMB | Hartebeesthoek | South Africa | –25.88 | 27.70 | 2007.2–2011.3 |
HEMB | St-Helena | UK (South Atlantic Ocean) | –15.95 | –5.67 | 2003.2–2011.3 |
KOLB | Kauai | USA (Hawaii) | 22.12 | –159.67 | 2002.9–2011.3 |
LICB | Libreville | Gabon | 0.35 | 9.67 | 2005.9–2011.3 |
MIAB | Miami | USA | 25.73 | –80.17 | 2005.2–2011.3 |
REZB | Reykjavik | Iceland | 64.15 | –21.98 | 2004.8–2011.3 |
RIQB | Rio Grande | Argentina | –53.78 | –67.75 | 2008.3–2011.3 |
ROUB | Rothera | Antarctica (UK base) | –67.57 | –68.12 | 2007.9–2011.1 |
SANB | Santiago | Chile | –33.15 | –70.67 | 2001.5–2011.3 |
SYPB | Syowa | Antarctica (Japanese base) | –69.00 | 39.58 | 1999.3–2011.3 |
TLSB | Toulouse | France | 43.55 | 1.48 | 2007.5–2011.3 |
YASB | Yarragadee | Australia | –29.01 | 115.35 | 2003.9–2011.3 |
YEMB | Yellowknife | Canada | 62.48 | –114.48 | 2007.6–2011.3 |

Map of the 18 sites selected in this study.
Carte des 18 sites sélectionnés dans cette étude.
Table 2 shows the percentages of outliers and interpolated points (data gaps and values considered as aberrant) relative to the total number of points (number of observations). We remark that the time series ADFB, HBMB and YEMB present the largest proportions of data gaps (relatively to their observation period) over the periods respectively: (2010.69–2010.88), (2010.75–2010.94) and (2010.75–2010.94). In general, the cause of these data gaps could be related to equipment problem affecting either the data quality (oscillator problem) or the physical location of the antenna (antenna tilt), as discussed in Willis et al. (2009). It could also be linked to a lesser amount of DORIS data in the first weeks after first data availability or to a local geophysical phenomenon (earthquake, landslide, etc.). We note that the difference between the percentages of outliers and interpolated points in the North component of ARFB station is due to the elimination of the two first points deemed aberrant and therefore not taken into account in the interpolation of data gaps. We note the same thing for the components North and Vertical of DIOB station. Consequently, the first point has been removed from each component.
Problèmes rencontrés lors de l’analyse des 18 séries temporelles STCD DORIS.
Station | Series (mm) |
Obs. nbr. | Gaps (week) |
Perc. outliers | Perc. interp. | Station | Series (mm) |
Obs. nbr. | Gaps (week) |
Perc. outliers | Perc. interp. |
ADFB | dN | 152 | 9 | 2.0 | 11.8 | MIAB | dN | 320 | 0 | 0.6 | 0.6 |
dE | 1.3 | 11.2 | dE | 0.3 | 0.3 | ||||||
dH | 3.3 | 13.2 | dH | 1.9 | 1.9 | ||||||
ARFB | dN | 246 | 0 | 1.2 | 0.4 | REZB | dN | 340 | 0 | 0 | 0 |
dE | 2.9 | 2.9 | dE | 0 | 0 | ||||||
dH | 2.9 | 2.9 | dH | 0.6 | 0.6 | ||||||
CADB | dN | 345 | 9 | 0.3 | 7.3 | RIQB | dN | 160 | 0 | 0.6 | 0.6 |
dE | 1.2 | 7.8 | dE | 0.6 | 0.6 | ||||||
dH | 0 | 7.0 | dH | 0.6 | 0.6 | ||||||
DIOB | dN | 257 | 0 | 0.4 | 0 | ROUB | dN | 169 | 0 | 0.6 | 0.6 |
dE | 1.2 | 1.2 | dE | 0.6 | 0.6 | ||||||
dH | 2.0 | 1.6 | dH | 3.0 | 3.0 | ||||||
DJIB | dN | 249 | 0 | 0 | 0 | SANB | dN | 506 | 7 | 0.4 | 1.8 |
dE | 0.4 | 0.4 | dE | 0.6 | 2.0 | ||||||
dH | 2.0 | 2.0 | dH | 1.6 | 3.0 | ||||||
HBMB | dN | 190 | 9 | 0.5 | 15.3 | SYPB | dN | 626 | 2 | 1.8 | 2.4 |
dE | 2.1 | 16.8 | dE | 2.2 | 2.9 | ||||||
dH | 1.6 | 16.3 | dH | 2.6 | 3.2 | ||||||
HEMB | dN | 420 | 2 | 0 | 3.1 | TLSB | dN | 201 | 0 | 0.5 | 0.5 |
dE | 0 | 3.1 | dE | 0.5 | 0.5 | ||||||
dH | 1.0 | 4.1 | dH | 1.5 | 1.5 | ||||||
KOLB | dN | 441 | 1 | 0 | 0.2 | YASB | dN | 387 | 1 | 0 | 0.3 |
dE | 0 | 0.2 | dE | 0 | 0.3 | ||||||
dH | 1.6 | 1.8 | dH | 1.8 | 1.3 | ||||||
LICB | dN | 274 | 9 | 0.4 | 3.7 | YEMB | dN | 175 | 5 | 1.1 | 14.3 |
dE | 0.4 | 3.7 | dE | 0.6 | 13.7 | ||||||
dH | 1.1 | 4.4 | dH | 1.7 | 14.9 |
5 Results and discussion
5.1 Trend
As previously mentioned, the SSA technique requires, a priori, the choice of the embedding dimension M which depends on the periodicity of the time series without exceeding half of the length of the time series. In this application, we have taken arbitrarily M = 52 weeks which corresponds to one year.
Fig. 2 represents the diagrams of the logarithms of the 52 eigenvalues for the components (North: dN, East: dE and Vertical: dH) of stations at high latitude. The first eigenvalue, which is clearly visible from the other remaining values, indicates the signature of a dominant trend represented by the Reconstructed Component RC 1 as seen in Fig. 3.
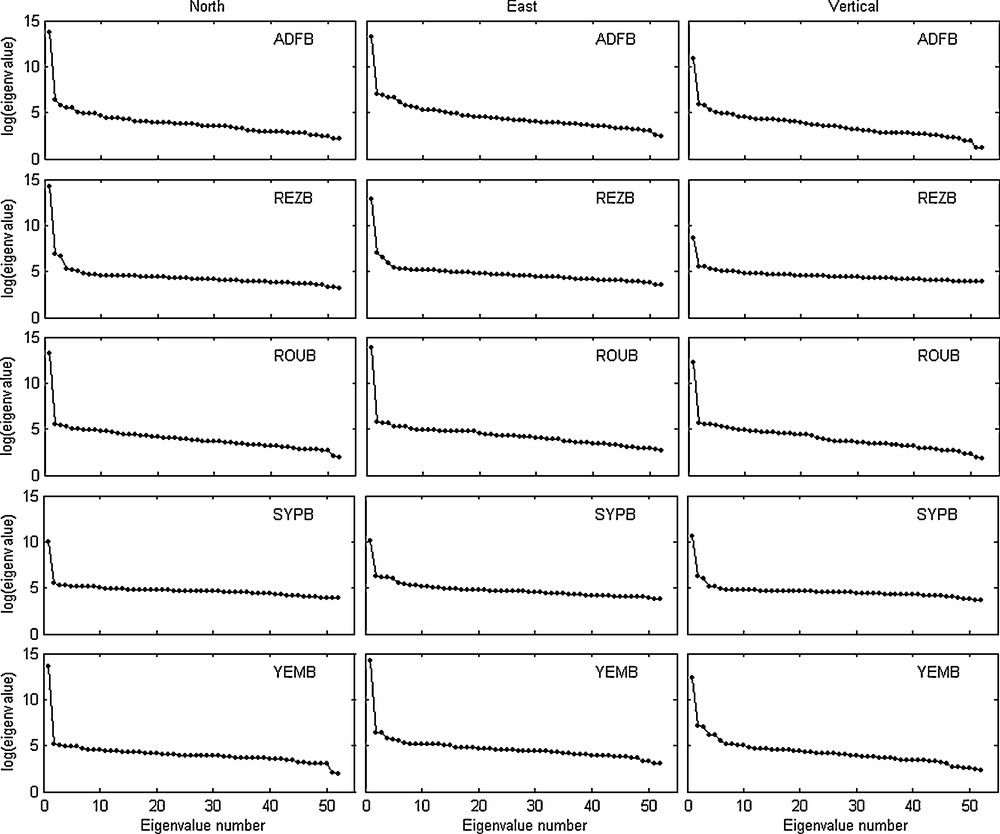
Diagrams of the 52 eigenvalues for the components (North, East and Vertical) of stations at high latitude.
Diagrammes des 52 valeurs propres pour les composantes (Nord, Est et Verticale) des stations de haute latitude.

Superposition of the original components (North, East and Vertical) of stations at high latitude with their non-linear trend (reconstructed component RC1).
Superposition des composantes originales (Nord, Est et Verticale) des stations de haute latitude, avec leur tendance non linéaire (composante reconstruite RC1).
The results provided in Table 3 show that the partial variance of trend in the most horizontal components (North and East) of studied stations explains over 90% of the total signal and it is more important compared to the Vertical component. Globally, the horizontal displacements of the stations are usually due to plate tectonics (Argus et al., 2010) and the vertical displacements may be due to local subsidence or postglacial rebound (King et al., 2010; Willis et al., 2009).
Les variances partielles et les pentes (obtenues par la méthode des moindres carrés) des tendances non linéaires (composante reconstruite RC1) dans les composantes Nord, Est et Verticale, et les vitesses GEODVEL2010 issues du site web de UNAVCO dans les composantes horizontales (Nord et Est).
Station | Series (mm) |
Partial variance | Slope (mm/yr) |
GEODVEL velocity | Station | Series (mm) |
Partial variance | Slope (mm/yr) |
GEODVEL velocity |
ADFB | dN | 99.59 | –14.95 ± 0.15 | –12.16 | MIAB | dN | 88.37 | 2.05 ± 0.09 | 1.94 |
dE | 98.54 | 11.02 ± 0.15 | 7.79 | dE | 96.62 | –12.70 ± 0.07 | –10.26 | ||
dH | 94.90 | 2.77 ± 0.10 | dH | 14.67 | 1.61 ± 0.03 | ||||
ARFB | dN | 89.57 | 6.07 ± 0.22 | 10.34 | REZB | dN | 99.65 | 21.63 ± 0.07 | 19.28 |
dE | 76.89 | 11.35 ± 0.29 | –2.24 | dE | 98.29 | –15.28 ± 0.13 | –9.23 | ||
dH | 20.15 | 6.50 ± 0.21 | dH | 54.46 | 2.64 ± 0.04 | ||||
CADB | dN | 98.09 | 15.15 ± 0.07 | 11.94 | RIQB | dN | 99.55 | 16.36 ± 0.09 | 10.69 |
dE | 70.85 | –4.78 ± 0.22 | –3.49 | dE | 77.00 | 6.26 ± 0.07 | 1.87 | ||
dH | 80.18 | 16.65 ± 0.58 | dH | 87.40 | 0.11 ± 0.14 | ||||
DIOB | dN | 98.65 | –14.41 ± 0.05 | a | ROUB | dN | 99.40 | 9.96 ± 0.07 | 10.45 |
dE | 94.69 | 5.78 ± 0.06 | a | dE | 99.57 | 17.13 ± 0.04 | 13.78 | ||
dH | 11.33 | –1.78 ± 0.06 | dH | 98.11 | 4.65 ± 0.11 | ||||
DJIB | dN | 99.68 | 16.49 ± 0.06 | 15.15 | SANB | dN | 93.77 | 7.01 ± 0.46 | 10.42 |
dE | 99.78 | 31.61 ± 0.07 | 23.62 | dE | 82.27 | 2.49 ± 0.87 | –0.24 | ||
dH | 91.21 | 1.25 ± 0.02 | dH | 53.76 | –0.67 ± 0.04 | ||||
HBMB | dN | 99.30 | 16.50 ± 0.09 | 17.28 | SYPB | dN | 78.56 | 2.63 ± 0.04 | 2.77 |
dE | 98.56 | 15.34 ± 0.19 | 17.30 | dE | 79.11 | –3.18 ± 0.03 | –3.52 | ||
dH | 71.03 | 2.17 ± 0.07 | dH | 88.77 | 3.04 ± 0.07 | ||||
HEMB | dN | 99.36 | 20.18 ± 0.08 | 17.66 | TLSB | dN | 99.52 | 15.56 ± 0.07 | 15.50 |
dE | 99.07 | 21.13 ± 0.05 | 22.46 | dE | 99.46 | 14.86 ± 0.04 | 19.26 | ||
dH | 45.02 | 4.06 ± 0.07 | dH | 9.73 | 1.72 ± 0.04 | ||||
KOLB | dN | 99.74 | 33.16 ± 0.07 | 34.15 | YASB | dN | 99.93 | 57.01 ± 0.08 | 57.06 |
dE | 99.90 | –61.09 ± 0.09 | –62.98 | dE | 99.83 | 39.88 ± 0.08 | 38.61 | ||
dH | 15.46 | 0.92 ± 0.06 | dH | 82.43 | 0.57 ± 0.05 | ||||
LICB | dN | 99.66 | 15.86 ± 0.05 | 18.25 | YEMB | dN | 99.61 | –10.50 ± 0.05 | –10.51 |
dE | 99.12 | 21.02 ± 0.08 | 22.04 | dE | 99.58 | –16.63 ± 0.07 | –17.24 | ||
dH | 46.97 | 6.20 ± 0.10 | dH | 97.21 | 8.23 ± 0.10 |
a The Aegean Sea plate (location of DIOB station) is not included in the model GEODVEL2010.
Our results (Table 3) show that the velocities values of the North and East components for most DORIS stations are consistent with those of GEODVEL (for GEODesy VELocity) model (Argus et al., 2010) provided by UNAVCO at http://www.unavco.org/community_science/science-support/crustal_motion/dxdt/model.html. We note a particularity in the velocity values of Arequipa (ARFB) and Rio Grande (RIQB) stations. For Arequipa, this is due to post-seismic relaxation after the June 23 2001 (magnitude 8.1) earthquake (Willis et al., 2009). For Rio Grande, this can be explained that this site is moving east relative to the South America plate at a significant velocity (2.2 ± 1.3 mm/yr) (Argus at al., 2010), which is consistent with the hypothesis (Smalley et al., 2003) that left-lateral shear across a wide east-striking zone in Patagonia causes the region several tens of kilometres north of the Magallenes-Fagano fault to be moving east relative to the interior of the South America plate. However, the GEODVEL model does not solve for the motion of all tectonic plates; the small Aegean Sea plate is not included in this model. For this reason, the horizontal velocities of Dionysos station (DIOB), which is located on the Aegean Sea plate, are not provided in Table 3.
However, the low partial variances in the Vertical component for the stations ARFB, DIOB, KOLB, MIAB and TLSB compared to other stations can be explained by the fact that these sites are experiencing a small vertical deformation as a result of Glacial Isostatic Adjustment (GIA). In the ICE-5G (VM2) model (Peltier, 2004), ARFB station has a predicted vertical rate of 0.8 mm/yr, DIOB 0.1 mm/yr, KOLB –0.3 mm/yr, MIAB –1.5 mm/yr and TLSB –0.2 mm/yr. Furthermore, the high velocity in the Vertical component of the station CADB is particularly significant; which can be explained by the fact that the position and velocity solutions for this station could be affected by errors related to the South Atlantic Anomaly (SAA) effect on SPOT-5 (Bock et al., 2010; Stepanek et al., 2010), especially as this station is located just in the centre of the SAA and at least for stations located just close to the SAA as ARFB, HEMB and SANB. Another possibility is also that this station presents a positive vertical discontinuity around mid 2008 that has not been previously detected by any DORIS group. Using the wavelet transform (multiresolution analysis) (Khelifa et al., 2012), the approximation signal at level 5 (Fig. 4) shows a discontinuity of 13 mm and a change in velocity on 3 June 2008. To identify this discontinuity, we have used the wavelet of Daubechies “db2” which is more appropriate for this kind of application (Mallat and Hwang, 1992). YEMB results present the largest amplitude for the vertical velocity (8.23 mm/yr). This value is very close to the value predicted by the ICE-5G (VM2) model (i.e. 8.3 mm/yr).

Highlighted by the signal of approximation 5 (Khelifa et al., 2012) a discontinuity of 13 mm in the Vertical component of CADB station, on 3 June 2008.
Mise en évidence par le signal d’approximation 5 (Khelifa et al., 2012) d’une discontinuité de 13 mm dans la composante Verticale de la station CADB, le 3 juin 2008.
5.2 Seasonal signals
The main skill of SSA is its ability to automatically and easily detect the dominant periodic signals in the time series; near equality of a great eigenvalues pair is associated with a dominant seasonal signal activity. Figs. 5–7 show a few examples of seasonal signals contained in the studied time series and their periodicities identified by periodogram. The most important peaks on the periodogram indicate the values of the periodicities contained in the signal such as annual and semi-annual signals, 117, 59, and 20.3 days. In Fig. 5, the reconstructed component RC 2-3 of the North component of HEMB station, based on the second and third EOFs (eigenvectors), reveals a clear annual signal with a partial variance of 0.20%, and the reconstructed components RC 4-5 and RC 6-7 in the East component illustrate, respectively, a periodic signals of 59 and 20.3 days with a partial variance of 0.10% and 0.08%, respectively. Fig. 6 reveals an evident semi-annual signal contained in the Vertical component of SANB station with a partial variance of 3.45%, and the Fig. 7 shows a seasonal signal of 117 days in the Vertical component of ADFB and TLSB stations with a partial variance of 1.23% and 5.01%, respectively.

Seasonal signals in the North and East components of HEMB station with their Power Spectral Density PSD (periodogram). The annual signal in the North component is represented by the reconstructed component RC 2-3, and the seasonal signals of 59 and 20.3 days in the East component are represented respectively by RC 4-5 and RC 6-7 over the last two years (2009–2011).
Signaux saisonniers dans les composantes Nord et Est de la station HEMB, avec leur densité spectrale de puissance DSP (périodogramme). Le signal annuel dans la composante Nord est représenté par la composante reconstruite RC 2-3, et les signaux saisonniers de 59 et 20,3 jours dans la composante Est sont représentés respectivement par RC 4-5 et RC 6-7 au cours des deux dernières années (2009–2011).

The semi-annual signal (represented by the reconstructed component RC 4-5) and its periodogram in the Vertical component of SANB station over the last five years (2006–2011).
Le signal semi-annuel (représenté par la composante reconstruite RC 4-5) et son périodogramme dans la composante Verticale de la station SANB au cours des cinq dernières années (2006–2011).

The seasonal signal of 117 days and its periodogram in the Vertical component of ADFB (represented by the reconstructed component RC 2-3) and TLSB (represented by the reconstructed component RC 5) stations.
Le signal saisonnier de 117 jours et son périodogramme dans la composante Verticale de la station ADFB (représentés par la composante reconstruite RC 2-3) et de la station TLSB (représenté par la composante reconstruite RC 5).
The annual and semi-annual errors and the term of 117 days were previously detected by several authors (Le Bail, 2006; Mangiarotti et al., 2001; Williams and Willis, 2006). The annual periodicity may be connected to the draconitic period of the sun-synchronous SPOT satellites (Gobinddass et al., 2009b) or to un-modelled effects in atmospheric density models used for satellite drag correction (Gobinddass et al., 2010), while the semi-annual periodicity could probably be related to the atmosphere perturbation (Stepanek et al., 2010). However, the periodicity of 117 days is the draconitic period of the TOPEX satellite (Gobinddass et al., 2009a, 2009b), while the periodicity of 20.3 days could be connected to the hydrological phenomena and atmospheric loading (Williams and Willis, 2006), and the periodicity of 59 days could be associated to the 117.3 days (–3.0679 degree/day) period of the angle between the TOPEX orbital plane and the sun (Williams and Willis, 2006).
We should point out that the error of 120 days was supposed to be removed or at least mitigated in the ign09wd01 solution (Gobinddass et al., 2009a, 2009b), as the latest ign09wd01 solution is the result of a computation which takes into account a better strategy for the computation of the solar radiation pressure. For this reason, we have tried to check the noise remaining at 120 days in the analysed solution (ign09wd01) and to compare it with that in the previous solution (ign07wd01). For example, this error was detected in the ign03wd01 solution by (Bessissi et al., 2009) using the wavelets in the Vertical component of SYPB station. In the frame of this work, this error was also detected using the SSA on the same component (Fig. 8) in the ign07wd01 solution, but not in the case of ign09wd01 solution. The same error was also detected in the North component of CADB station (Fig. 8) in the ign07wd01 solution, but not in the case of ign09wd01 solution. Taking the stations which have a common period of observation longer than 4 years for both solutions (ign07wd01 and ign09wd01), we have found this error in the two solutions in the Vertical component of KOLB, East component of SYPB and North component of YASB (Fig. 9). The results show that the variance of this error, in respectively ign07wd01 and ign09wd01 solutions, was decreased from 3.27% to 2.90% for KOLB, 7.42% to 4.83% for SYPB and 0.04% to 0.01% for YASB. The amplitude of this error, computed as the average of amplitudes of this periodicity over the same observation period for the two solutions, is also decreased; passing for the solution ign07wd01 to the solution ign09wd01 from respectively: 3.2 to 1.6 mm for KOLB, 5.6 to 4.8 mm for SYPB and 3.0 to 1.9 mm for YASB. However, our results are in agreement with the results derived by (Gobinddass et al., 2009a, 2009b) which stipulate that the new solution ign09wd01 is an improvement over the previous ign07wd01 solution.

The seasonal signal of 120 days and its periodogram in the Vertical component of SYPB station (represented by the reconstructed component RC 5) and the North component of CADB station (represented by the reconstructed component RC 5-6) for the ign07wd01 solution.
Le signal saisonnier de 120 jours et son périodogramme dans la composante Verticale de la station SYPB (représentés par la composante reconstruite RC 5) et la composante Nord de la station CADB (représentés par la composante reconstruite RC 5-6) pour la solution ign07wd01.
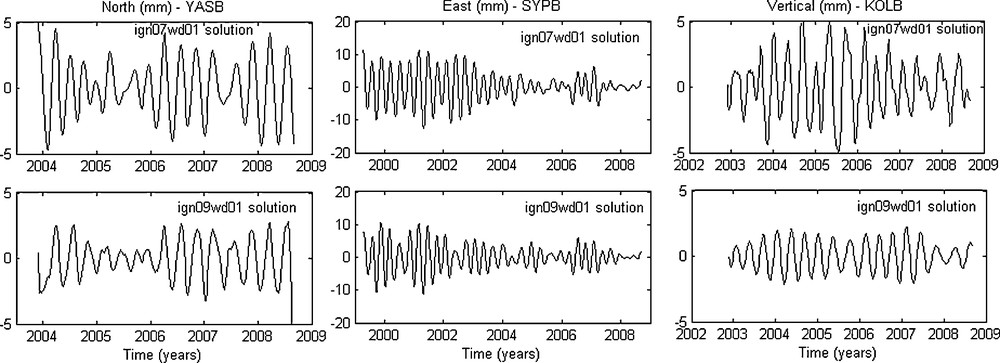
The seasonal signal of 120 days in the components: North of YASB station (represented by the reconstructed component RC 4), East of SYPB (represented by the reconstructed component RC 3-4) and Vertical of KOLB (represented by the reconstructed component RC 3-4) for the two solutions ign07wd01 and ign09wd01.
Le signal saisonnier de 120 jours dans les composantes : Nord de la station YASB (représenté par la composante reconstruite RC 4), Est de SYPB (représenté par la composante reconstruite RC 3-4) et Verticale de KOLB (représenté par la composante reconstruite RC 3-4) pour les deux solutions ign07wd01 et ign09wd01.
5.3 Noise determined by Singular Spectrum Analysis and wavelet
The SSA allows one to assess the noise affecting the time series by extracting the reconstructed components from the initial time series. In the diagram of the eigenvalues, the noise is characterized by much lower values that form a flat floor or a mild slope (Pike et al., 1984; Vautard and Ghil, 1989). In our case, the noise is extracted from the original components (North, East and Vertical) after removing their reconstructed components (de-noised time series) based on the first EOFs associated to the largest eigenvalues which correspond to trends (linear or non-linear) and various oscillatory components.
The critical point in wavelet analysis is the choice of optimal wavelet function which depends on the kind of applications (Meyer, 1992). In our application, we have used the Meyer wavelet as in Bessissi et al. (2009). The wavelet coefficients used in this analysis are calculated from a decomposition of the signal at level 4 using the VisuShrink method with soft thresholding.
Figs. 10 and 11 represent the noise and the de-noised components (dN, dE and dH) for the stations at high latitude determined by SSA and wavelet, respectively. The obtained results show that the de-noised components determined by wavelet are more regular and smooth relatively to those determined by SSA which is probably related to the continuous character of soft thresholding function.
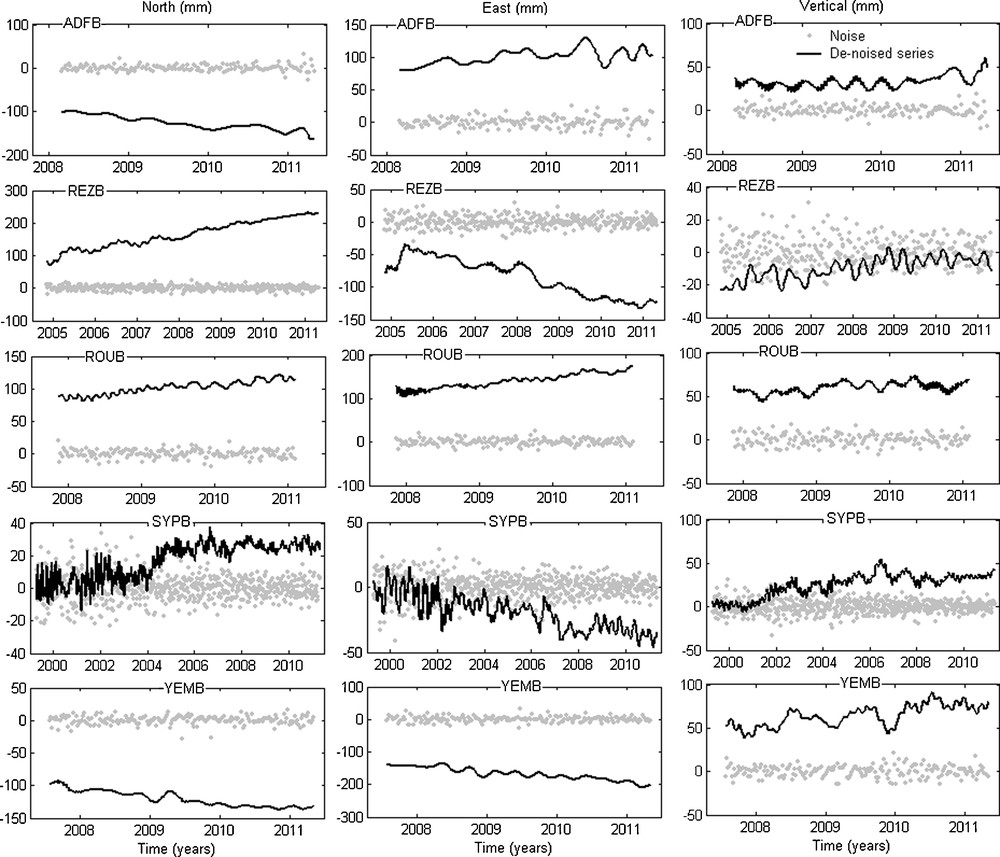
The noise and the de-noised components (North, East and Vertical) determined by Singular Spectrum Analysis for the stations at high latitude.
Le bruit et les composantes dé-bruitées (Nord, Est et Verticale) déterminés par l’analyse du spectre singulier pour les stations de haute latitude.
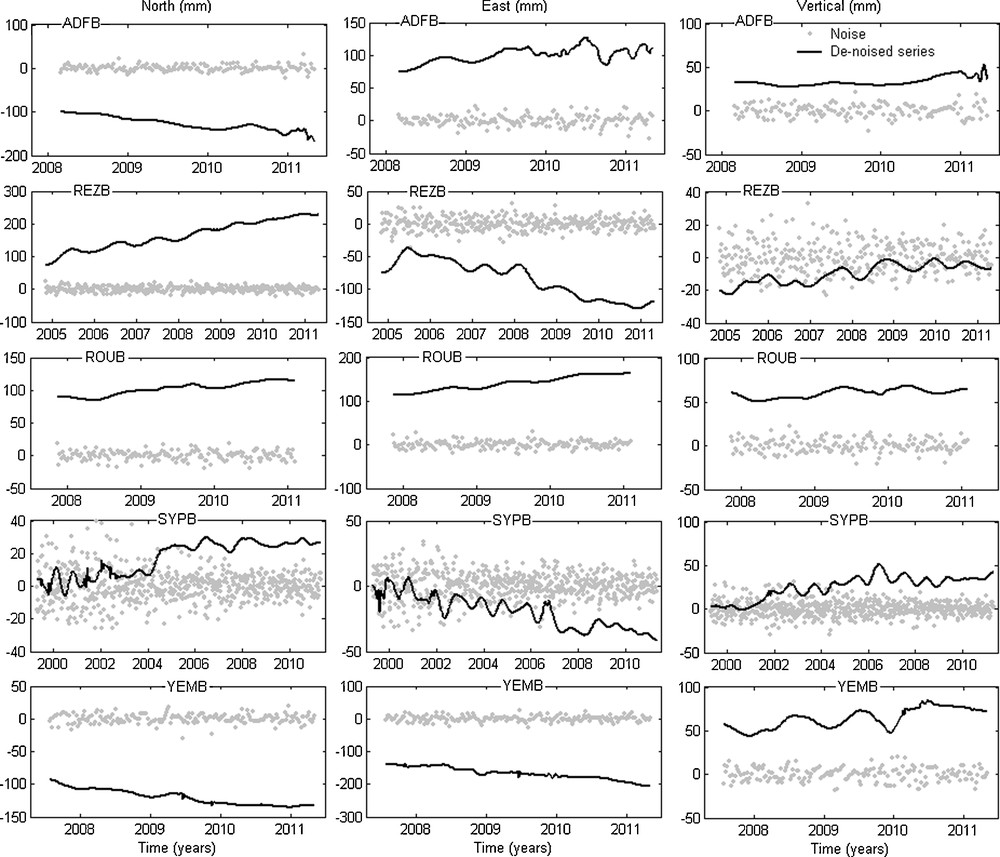
The noise and the de-noised components (North, East and Vertical) determined by wavelet for the stations at high latitude.
Le bruit et les composantes dé-bruitées (Nord, Est et Verticale) déterminés par ondelettes pour les stations de haute latitude.
Table 4 shows that the standard deviation (STD) of the noise determined by SSA in the North, East and Vertical components ranges between 7–13, 8–24 and 7–14 mm respectively, and that determined by wavelet is about 7–14, 8–27 and 7–16 mm, respectively. Therefore, the both techniques give almost the same results in term of standard deviation.
Déviation standard (STD) du bruit déterminée par ondelettes et analyse du spectre singulier (SSA) dans les composantes Nord, Est et Verticale.
Station | Technique | STD – North (mm) |
STD – East (mm) |
STD – Vertical (mm) |
ADFB | Wavelet | 7.2 | 8.2 | 7.1 |
SSA | 7.2 | 8.2 | 5.7 | |
ARFB | Wavelet | 14.3 | 27.6 | 15.8 |
SSA | 11.9 | 24.0 | 14.9 | |
CADB | Wavelet | 13.6 | 22.2 | 15.5 |
SSA | 13.1 | 19.5 | 13.2 | |
DIOB | Wavelet | 10.3 | 11.8 | 9.2 |
SSA | 9.0 | 11.0 | 7.4 | |
DJIB | Wavelet | 8.0 | 13.0 | 9.4 |
SSA | 7.6 | 12.2 | 8.8 | |
HBMB | Wavelet | 9.7 | 15.4 | 14.0 |
SSA | 9.8 | 13.0 | 11.6 | |
HEMB | Wavelet | 9.9 | 15.8 | 10.4 |
SSA | 9.4 | 14.9 | 9.1 | |
KOLB | Wavelet | 10.2 | 12.2 | 8.9 |
SSA | 9.5 | 11.4 | 7.7 | |
LICB | Wavelet | 9.1 | 15.1 | 10.5 |
SSA | 8.5 | 14.5 | 9.1 | |
MIAB | Wavelet | 10.6 | 14.9 | 8.4 |
SSA | 10.2 | 14.2 | 7.6 | |
REZB | Wavelet | 8.1 | 9.9 | 9.1 |
SSA | 7.3 | 8.8 | 8.3 | |
RIQB | Wavelet | 8.4 | 12.7 | 10.0 |
SSA | 7.5 | 9.9 | 7.8 | |
ROUB | Wavelet | 7.2 | 8.6 | 7.6 |
SSA | 6.4 | 7.3 | 6.5 | |
SANB | Wavelet | 12.4 | 21.7 | 12.5 |
SSA | 11.6 | 20.6 | 10.8 | |
SYPB | Wavelet | 9.8 | 10.2 | 9.0 |
SSA | 8.3 | 8.6 | 7.9 | |
TLSB | Wavelet | 8.4 | 12.3 | 9.0 |
SSA | 7.5 | 10.4 | 7.4 | |
YASB | Wavelet | 10.4 | 12.0 | 7.8 |
SSA | 9.2 | 10.8 | 6.9 | |
YEMB | Wavelet | 7.0 | 8.8 | 7.6 |
SSA | 6.4 | 8.5 | 6.5 |
The East component is generally less well determined than the two others; most DORIS satellites have high inclinations, resulting in roughly north-south orientation of the satellite passes over the stations. This pass geometry implies a poor determination of the East station coordinates (Le Bail, 2006). This is confirmed by our results, which show that the noise in the East direction is more important compared to the North and the Vertical ones, while it is small in the stations at high latitude (ADFB, REZB, ROUB, SYPB and YEMB) compared to the other stations. However, it is dependent of the latitude of station which is simply a result of the near polar orbit of the SPOT and Envisat satellites (98° inclination) (Le Bail, 2006; Soudarin et al., 1999; Williams and Willis, 2006). As a result, the high latitude stations are globally more observed than the others by SPOT and Envisat satellites and less observed by TOPEX/Poseidon (66.04° inclination). Therefore, the number of observations for stations at high latitude is larger than for the other ones.
6 Conclusions
The main purpose of this article is to apply the wavelet transform and the SSA approaches in the analysis of the DORIS stations coordinate time series, in order to extract maximum information on their “noise” which allows one to investigate the stability of the stations and on their “signal” that allows one to determine the systematic signals such as trends and periodic components.
The non-linear trends and the seasonal signals (annual and semi-annual, 120, 59 and 20.3 days) contained in the studied time series have been well extracted and quantified by the SSA. The results show that the dominant signal present in these time series is mainly of geophysical nature (plate tectonics); the trend represents over 90% of the total signal in the horizontal components. We have also found through the studied stations that the error of 120 days in the ign09wd01 solution relatively to the previous solution ign07wd01 was removed from some coordinates and mitigated for the others. Using the wavelet analysis, this study has also allowed us to identify for CADB station a vertical discontinuity of 13 mm on 3 June 2008.
The noise determination by SSA approach is based on the removing of the trend and various seasonal components from the original time series, while the wavelet analysis, using the VisuShrink method with soft thresholding, performs well in the smoothing of the signal and in the simultaneous extracting of the noise from the original time series. The study presented here shows that the two approaches give approximately the same results for the noise level in term of standard deviation. Indeed, the standard deviation of the noise determined by SSA according to the North, East and Vertical components ranges between 7–13, 8–24 and 7–14 mm respectively, while those determined by wavelet are about 7–14, 8–27 and 7–16 mm, respectively. The noise level in the East component is more important compared to the North and the Vertical ones, and it is small in the stations at high latitude compared to the other ones. This is due to the near polar orbit of the SPOT and Envisat satellites (98° inclination). For sites at high latitude, the satellite passes are less orthogonal to the East component.
However, in the noise analysis of DORIS stations coordinate time series, the two approaches adopted throughout this work allow to give the signals of the true signal (de-noised time series) and of the noise, compared for example to MLE and Allan variance methods which give the information on the level and type of noise, without giving the filtered signals (signal and noise). Furthermore, the noise determination by wavelet analysis is rapid and direct, without any hypothesis on the properties (regularity and stationarity) of studied time series.