1 Introduction
In seismic exploration, random noise has various causes, not only during data acquisition, but also during various procedures in data processing such as deconvolution, migration, or even filtering. In Northeast China, countermeasures are hard to apply due to the complex geology (which leads to complicated coherent noise), and to severe winter storms for more than 5 months a year (which leads primarily to powerful random noise). Therefore, many available methods (Deng et al., 2010, 2011; Naghizadeh and Sacchi, 2009; Satish and Nazneen, 2003) do not produce satisfactory results. Time-frequency peak filtering (TFPF) and its extension in the temporal and spatial domain would be a good choice (Lin et al., 2007) to eliminate the strong random noise.
The conventional TFPF algorithm proposed by B. Boashash and M. Mesbah has found its application in seismic record denoising and achieved some good results in the Daqing oil basin in Northeast China, because of its advantages in non-stationary signal estimation without prior knowledge, even under low signal-to-noise ratio (SNR) (Lin et al., 2007, 2008; Liu et al., 2013). To achieve better performance, in the past 2 years, Wu et al. have analyzed the bias condition of conventional TFPF and developed the radial-trace TFPF (RT-TFPF) by utilizing the correlation between adjacent seismic channels (Wu et al., 2011). The RT-TFPF can stretch the valid signal (Henley, 2001) to make it as linear as possible within the window, so that the unbiased condition is better satisfied and a more satisfactory result could be obtained for both noise suppression and signal enhancement.
In RT-TFPF, the most crucial factor is to find the radial trace direction along which the linearity of the effective signals is most enhanced. To begin, a cluster of parallel radial traces with a fixed angle of 45 degree are used because no interpolation needs to be involved and all the effective signals could be stretched, although not to the greater extent. Then other angles of radial traces, parabolic traces (Tian and Li, 2014) and hyperbolic traces (Tian et al., 2014) are constructed to find the direction that approximately aligns with the reflection event according to its time-distance curve equation. In this paper, we propose a temporally and spatially varied radial trace TFPF based on a new trace transform to improve the performance of the above RT-TFPF and deal with seismic trace ensembles including, but not limited to, CMP trace ensembles.
The rest of this paper is organized as follows. In section II, we present the principle of the conventional TFPF and derive the bias reduction condition with the help of a trace transform, and then we illustrate, using a synthetic model, both advantages and disadvantages of the 2D TFPF idea. Section III develops a trace functional to keep track of the temporally and spatially varied seismic event and derives the corresponding trace-transform-based 2D TFPF strategy, which is then applied to both a synthetic record and field data from Northeast China in Section IV. Finally, we present our conclusions in Section V.
2 The filter methodology
2.1 Conventional time-frequency peak filtering
TFPF has been proved to successfully reconstruct the reflected signals from observations corrupted by additive random noise (Lin et al., 2007). It may be divided into two steps, where the first consists in encoding the noised signal as the instantaneous frequency (IF) of a frequency-modulated analytic one, and the second in recovering the desired signal by IF estimation of the analytic one (Boashash and Mesbah, 2004).
The above two procedures can be expressed as follows:
(1) |
(2) |
(3) |
This means that, if the desired signal is linear (or we can say low frequency), conventional TFPF would give an unbiased estimation of the pure signal. For example, for seismic signals whose dominant frequencies are around 20 Hz, TFPF could recover the effective signal with almost no distortion of the amplitudes. However, for intermediate frequency (around 40 Hz) seismic record discrimination, conventional TFPF would cause serious amplitude attenuation, even for the shortest window in PWVD (Wu et al., 2014). In other words, sometimes there is no guarantee of linearity (Yu et al., 2015) of the desired signal, even for extreme values of the parameter. The limitation of the conventional TFPF is that it views the signal only along the time direction where the rapid variation may cause serious distortion. Furthermore, the correlation (Jiang et al., 2014) between adjacent channels is not considered and utilized in conventional TFPF.
2.2 Parallel radial trace TFPF based on radial trace transform
To take advantage of the amplitude correlation within a 2D seismic record, a linear trace transform is a good choice. One type of linear trace transform does not calculate any functional, but only extracts the amplitudes from the 2D trace ensemble and then interpolates them onto another 2D panel. This is also known as the radial trace transform (Li et al., 2013). Though only a point-to-point mapping, the radial trace transform can greatly reduce the frequency of a signal whose wavefront aligns with the transform trajectories.
The reduced-frequency signal is useful for TFPF because the unbiased estimation is based on the premise that the desired signal is linear in time (approaches DC). Therefore, if the direction of the trajectories could be selected to increase the linearity (or decrease the frequency) as much as possible, the 2D TFPF that filters the record along the trajectories would provide a much better performance than the conventional TFPF.
For 2D TFPF, the first step is to transform the original noisy record into the radial trace transform domain , i.e.:
(4) |
The second step is to find the optimal direction along which the dominant frequency is highly reduced and encode as the IF of a frequency-modulated analytic signal,
(5) |
The next step consists in estimating the spatiotemporal signal in the R–T domain with the TFPF algorithm
(6) |
(7) |
2D TFPF filters a record with the help of the radial trace transform to better satisfy the condition of equation (3), rather than conventional TFPF, which filters each channel along the time direction. Therefore, the key problem is to find the optimal radial trace directions to reduce the frequency to a certain extent. In particular, when the radial trace direction coincides with direction of the reflection event, the signal frequency will be reduced to the greatest extent and fulfil the unbiased condition of TFPF.
In parallel with radial trace TFPF, the optimal direction could be obtained only by visual inspection for a record, which may not suit for every reflection event or even any segment of one reflection event. To further improve the performance of 2D TFPF, we construct a linear trace-transform-based algorithm which calculates temporal and spatial varied radial trace direction for 2D TFPF.
2.3 Co-centroid trace-functional-based temporal-spatial varied 2D TFPF
Unlike the radial trace transform, here we use another kind of trace transform that calculates various functionals along trajectories cross-crossing the original X–T domain. For example, the well-known Radon transform (Tau-p Transform or Slant-Stack) calculates the integral of samples along the trajectory and transforms a 2D record into the Radon domain (Alpatov et al., 2015). Besides, these trajectories are not limited to the velocity direction in the radial trace transform, but can assume any direction through the data.
Consider Fig. 1 as a 2D seismic record , the origin could be located at any point and the trajectory is any of the straight lines that pass through this record (Kadyrov and Petrou, 2001). Each has two parameters, and , where is the perpendicular distance from the fixed origin O, and is the angle formed by the horizontal x-axis and the normal . Parameter is defined along the trajectory with its origin at the foot Q.
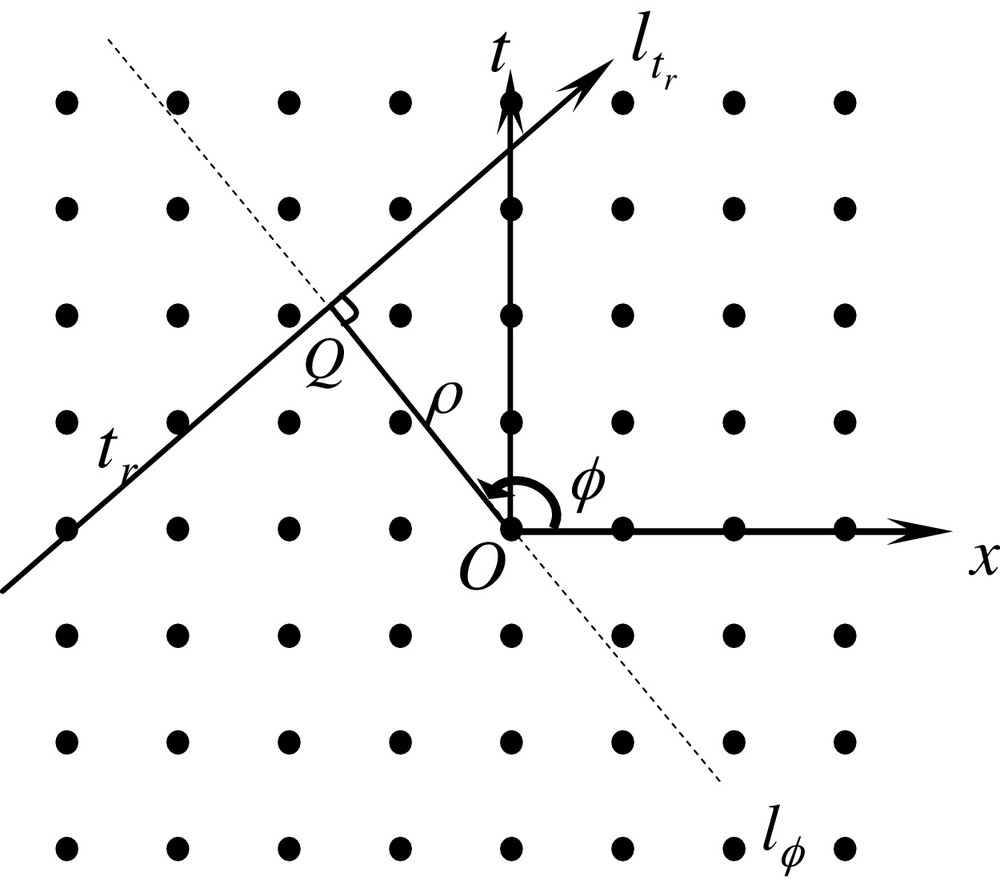
Definition of the linear trace transform.
In trace transform, various kinds of trace functionals can be calculated along the trajectories to manifest different properties of a record in the trace transform domain, such as the integral, the maximum\minimum, or the phase of first harmonic (Kadyrov and Petrou, 2001), etc. During our investigation of different functionals, we notice that some of them emphasize the common amplitude by significantly increasing its proportion in the transform domain. These particular trace functionals share one thing in common: they calculate the centroid along the trajectory. This property is very useful in finding the local direction of a reflection event, because when a trajectory coincides with part of a reflection event, it may capture mainly the common amplitude of the reflected signal, causing the trace functional value to peak.
In this paper, we construct a co-centroid (CC) trace functional and use it to find the temporal–spatial seismic event direction for 2D TFPF automatically. The CC trace functional along trace line is defined as:
(8) |
(9) |
In equation (8), the square of the distance is used to discriminate the reflection event direction, and is used as a weight to ensure not only temporal and spatial information of both the reflected signals and the random noise, but also their energy distribution are considered to avoid incorrect judgment of the reflection events in noise circumstance.
The CC trace functional could identify the optimum temporal and spatial varied trajectory direction according to the shape of the reflection event on the record. Meanwhile, applying TFPF along these directions could also satisfy the unbiased condition of the input signal in the TFPF model.
As we mentioned, the largest energy in the CC transform domain corresponds to constant amplitudes along the trajectory direction. Therefore, to compute a temporal-spatial radial trace direction , one only needs to find the direction which corresponds to the locally largest in , that is,
(10) |
The procedure of CC trace-transform-based 2D TFPF is as follows:
- • for each data point, draw a square on the record with the origin at this data point;
- • search for the direction which corresponds to the largest CC trace functional within each square;
- • apply the 2D TFPF based on the above temporal–spatial trajectory directions.
A synthetic seismic model with two reflection events is shown in Fig. 2, where the optimal temporal–spatial radial trace directions are about and , respectively. Fig. 3 shows the detected temporal-spatial directions with the application of the CC trace transform, which are consistent with the presets by comparison with the color bar on the right.

Synthetic model with events of different directions.
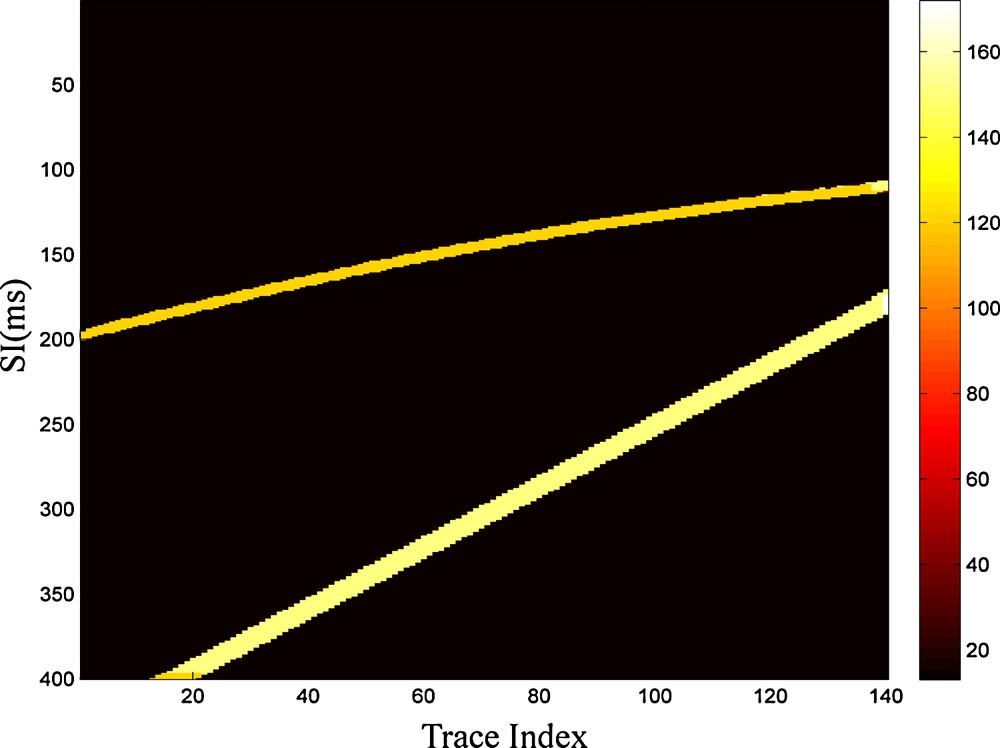
Temporal–spatial direction detected via the CC trace transform.
This test illustrates that by using the CC trace transform, not only the edges of the event could be detected, but also the temporal-spatial radial trace directions could be identified accurately.
3 Implementation and applications
3.1 Example on synthetic records
To attenuate the strong seismic random noise, the CC trace transform is used in the radial trace TFPF to identify the optimal filtering directions. Fig. 4 show a synthetic record with three reflection events where the dominant frequencies are 35 Hz, 30 Hz, and 25 Hz, respectively, and a noisy version with some real seismic noise from Northeast China (SNR = –4.8 dB).
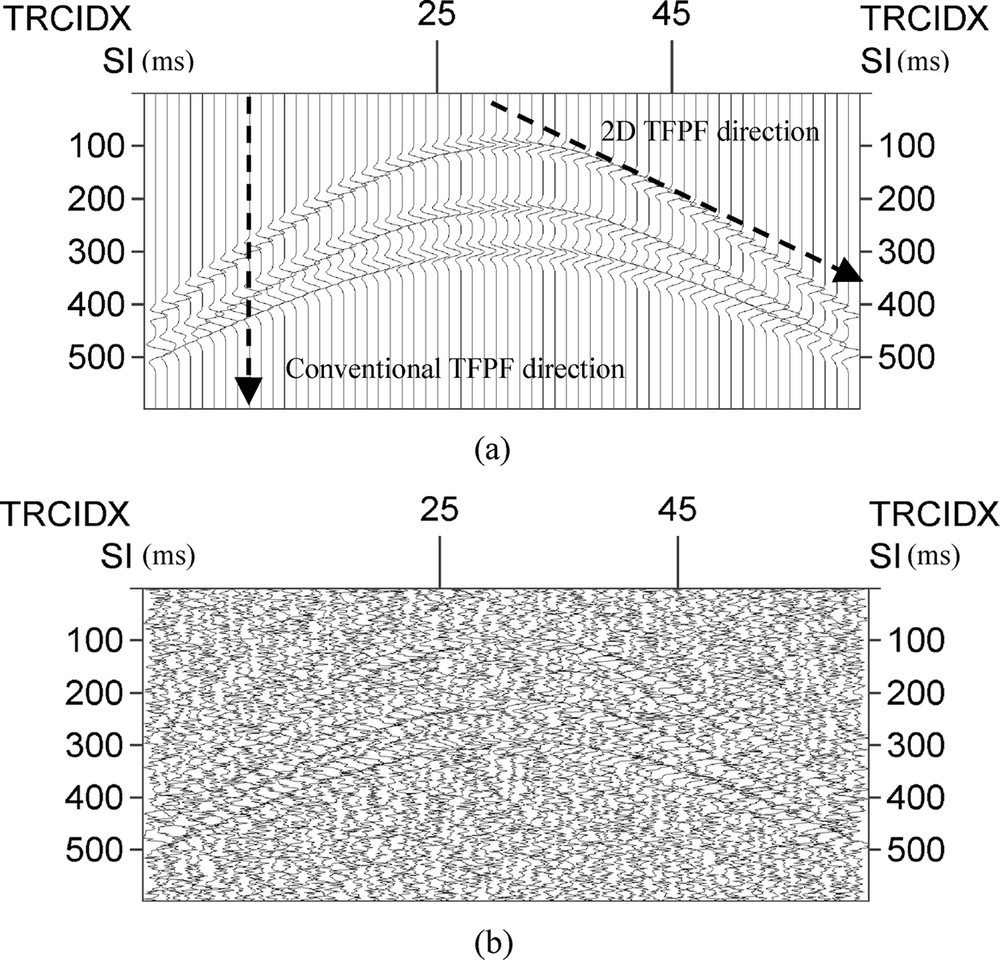
(a): a synthetic record (dominant frequency = 35, 30, and 25 Hz); (b): noised version (SNR = −4.8 dB).
The conventional TFPF that filters the entire record along the time direction would result in Fig. 5a, even with the optimal window length (Lee and Bien, 2011) and the SNR arises to –1.05 dB. Other non-optimal windows would result in pictures such as Fig. 5b, where more background noise as well as some reflected information is reduced, especially for the 35-Hz event in the shallow layer.
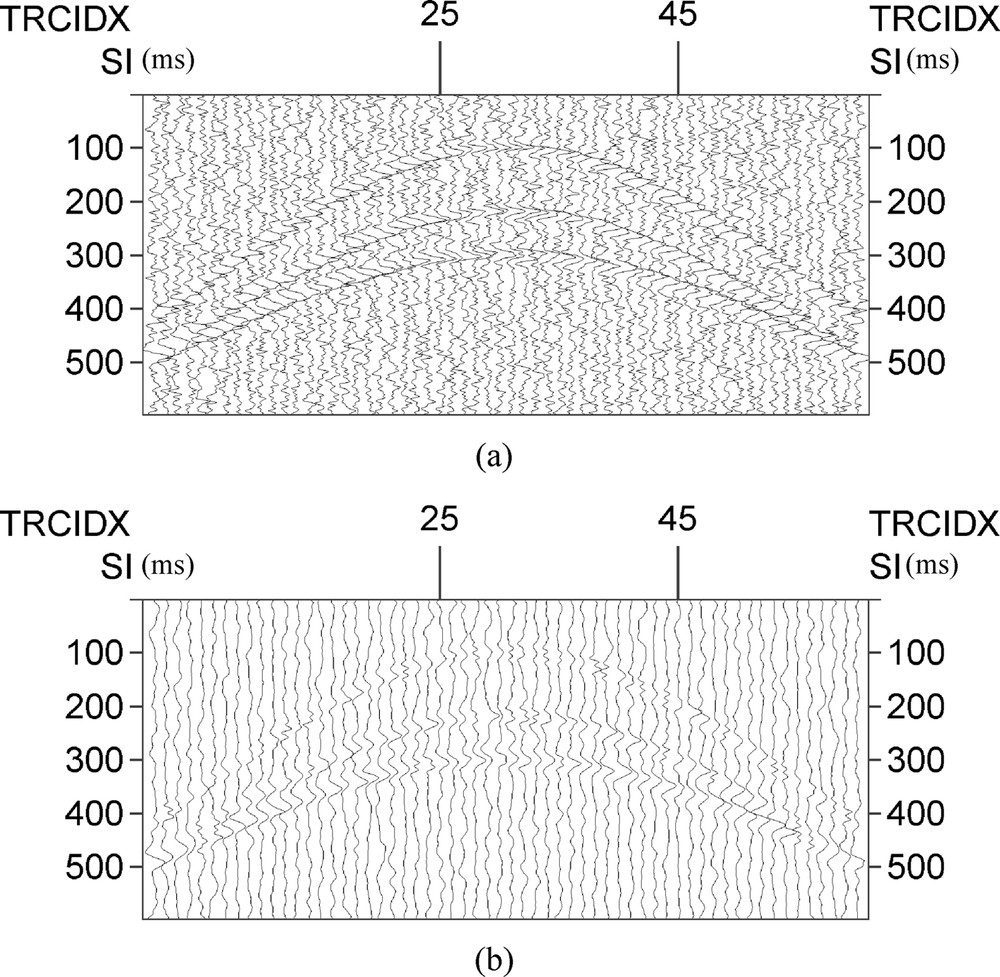
Filtered record: (a): conventional TFPF with optimal parameter; (b): conventional TFPF with non-optimal parameter.
The commonly used parallel radial trace TFPF with global angles , 45°, and 135° does not involve complex resampling techniques such as proximity or interpolation, but may be incompatible with all parts of the reflection event direction. As seen in Fig. 6, for a seismic record in which local directions of the reflection event are rapidly changing, the parallel radial trace TFPF with a global parameter could only recover parts of the reflection event, depending on which direction of the radial trajectories is chosen. Fig. 6a corresponds to 0°; therefore, only horizontal events are recovered. Fig. 6b, c correspond to 45° and 135°, respectively, hence, the corresponding portions of the original reflections are recovered. However, it should be noted that although for each of these cases, portions of the reflection are lost, the background noise is reduced to a certain degree. Thus, if a temporally and spatially varying radial trace direction could be found, the result would be improved.

Parallel 2D TFPF with global filtering directions: (a): ; (b): ; (c): .
Fig. 7a shows the CC trace-transform-based 2D TFPF result, where the SNR rises to 1.26 dB. Compared with Figs. 5 and 6, the recovered reflection event is more complete and clear than both the conventional TFPF and the parallel RT-TFPF result. Fig. 7b shows the difference with the case where the proposed method is used, in which almost no reflected energy is lost in this filtering procedure.

(a, b): filtered result and difference with the case when CC transform-based 2D TFPF is used (Fig. 4b).
We also show the results of some classical denoising methods such as wavelet transform filter (Herrmann and Hennenfent, 2008) and F–X deconvolution as well as the differences with the situation where these two methods are used. The discrete wavelet transform (DWT) tends to concentrate the energy of the desired signal into a small number of coefficients; hence, the DWT of the noisy record consists of a small number of coefficients with high SNR (which are kept) and a large number of coefficients with low SNR (which are discarded). After discarding the noisy coefficients, the record is reconstructed using the inverse DWT. Here, we decompose the original record at level 2, using Daubechies wavelets (db4) and hard threshold to obtain the wavelet method results in Fig. 8a, b. According to Fig. 8, both methods could effectively attenuate the random noise, but each one has its merits and deficiencies.
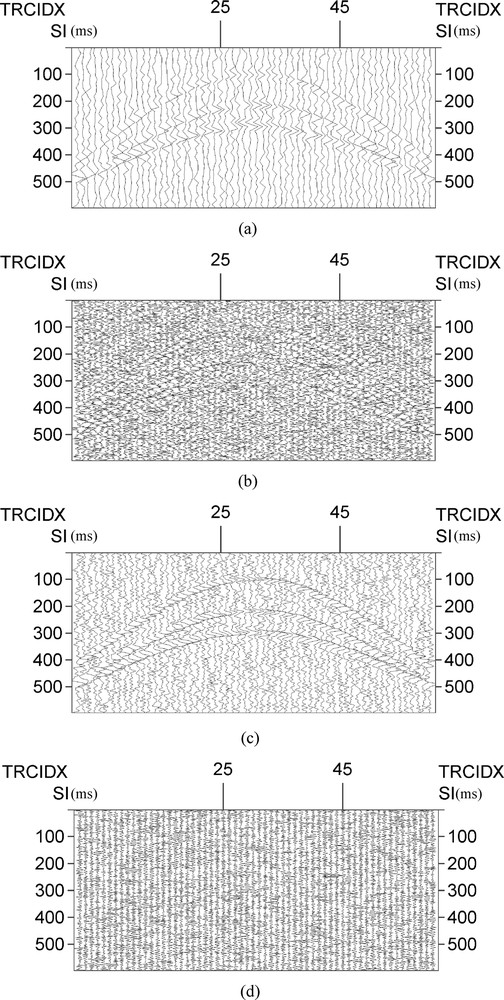
(a, b): filtered result and difference with the case when a wavelet transform filter is used (Fig. 4b); (c, d): filtered result and difference with the case when F–X deconvolution is used (Fig. 4b).
The wavelet filter could almost cleanly attenuate the background random noise, but most energy of the reflection event is badly lost, which can be seen clearly from the difference in Fig. 8b; the F–X method could also identify the reflected signal from the random noise circumstance, and the recovered reflection event is comparatively continuous. Table 1 lists the SNR of different methods, from which we can see that the proposed method increases the SNR to the greater extent.
SNR of different filtered results.
Methods | Results (dB) |
Original Record | –4.8 |
Conventional TFPF (optimal parameter) | –1.05 |
Conventional TFPF (non-optimal parameter) | 0.20 |
Parallel Radial Trace TFPF (global parameter) | –2.90 |
Wavelet transform filter | –1.46 |
F–X deconvolution | 0.13 |
Proposed method | 1.26 |
We also draw the waveform and spectrum of a single channel (the 16th) in Fig. 9. The proposed CC trace-based 2D TFPF nearly recovered the peak amplitude values of the three Ricker wavelets from the background noise, and behaved well in low-frequency (0–10 Hz) random noise attenuation and intermediate frequency seismic signal preservation from the spectrum.
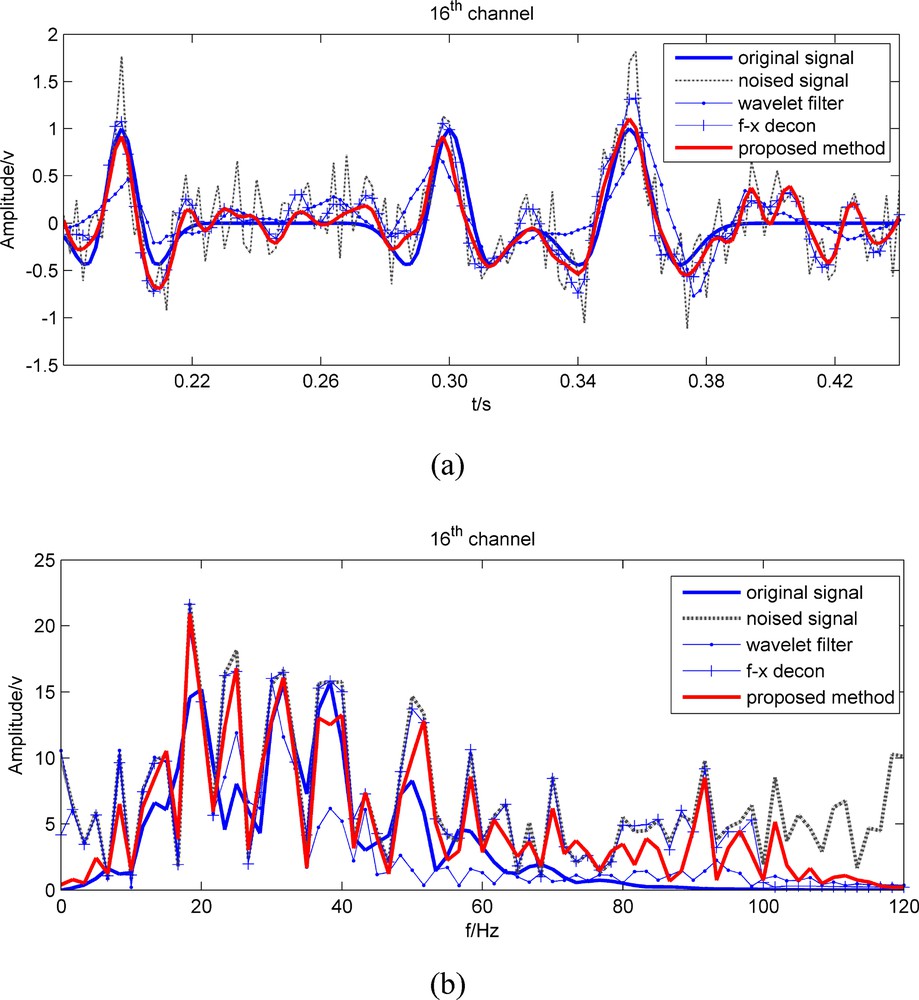
Comparison of single channel signals: (a): waveform; (b): spectrum.
3.2 Example on field data
In practical seismic data processing, details such as the frequencies or event wavefront orientations are usually not well known. Therefore, temporally and spatially varying 2D TFPF can be of importance for the discrimination and signal enhancement of the reflection events. Fig. 10a shows field data from Lamadian Territories in Northeast China. The sample frequency is 500 Hz. This segment is near the shot point so that the dominant frequency of the seismic wavelet is comparatively high. From it, we can see that the presence of random noise (caused by both severe weather and badly aliased coherent noise whose characteristics are similar to random noise) seriously obscures the reflection events and degrades the continuities of the events.
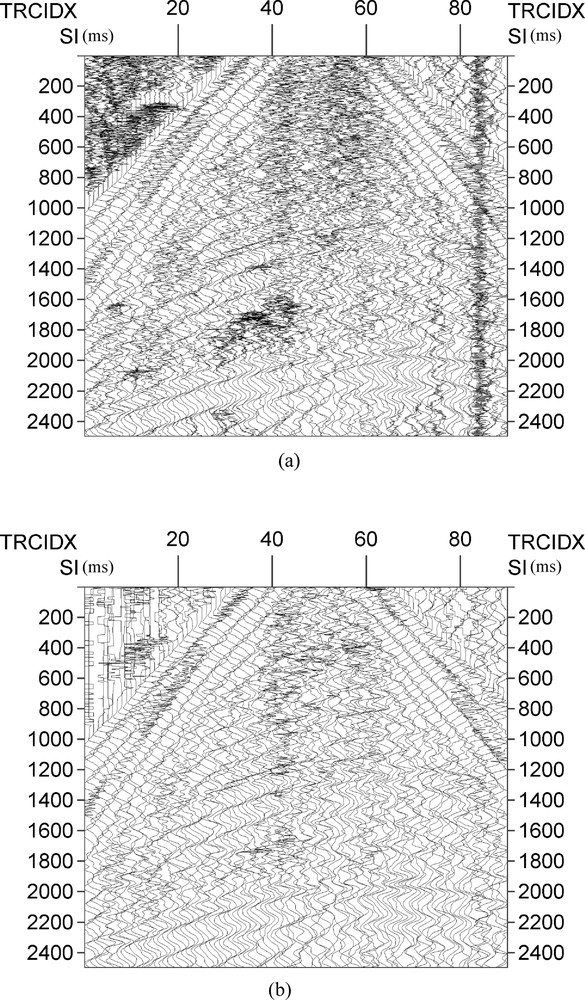
(a): single-shot gather from Lamadian Territories, Northeast China; (b): filtered result from using conventional TFPF.
A comparison of the results obtained by using the conventional TFPF, F–X deconvolution and the CC trace-transform-based 2D TFPF is shown in Figs. 10b, 11a and 12a, respectively. The conventional TFPF and F–X deconvolution can suppress large amounts of random noise, but the coherent events are still not well resolved with either of these two methods. It is to be noted that the shallow layer events in Figs. 10b and 11a can still be hardly identified, whereas with the proposed CC trace based 2D TFPF in Fig. 12a, a larger amount of noise has been removed as well, while more reflection events are discriminated from the background noise. The difference results from the use of the F–X deconvolution, and the proposed methods are shown in Figs. 11b and 12b, respectively, which also illustrate that CC trace transform could identify a more accurate filtering direction for 2D TFPF, resulting in little reflected energy loss in the filtering procedure.
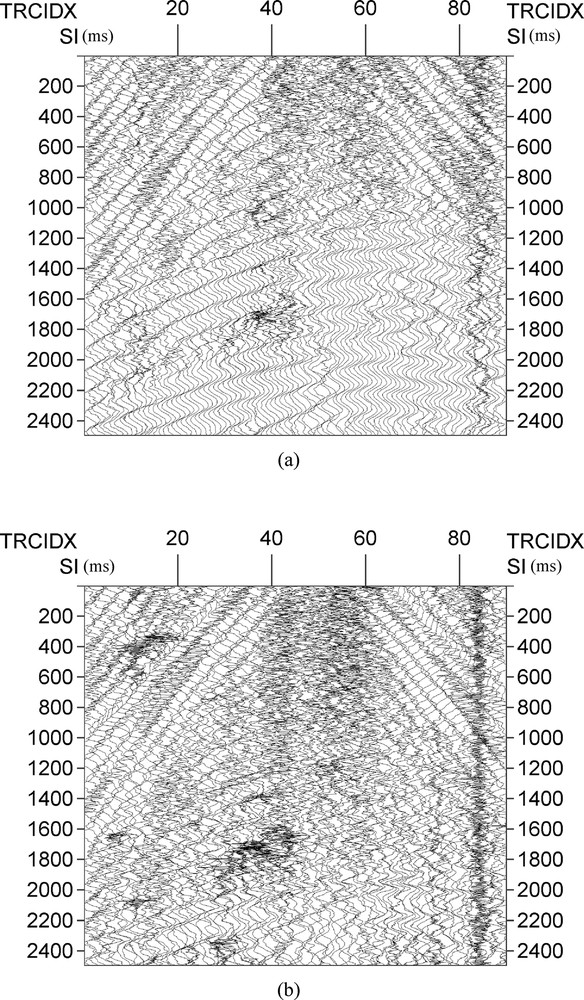
(a): filtered result when using F–X deconvolution; (b): difference result when using F–X deconvolution.

(a): filtered result when using CC trace-transform-based 2D TFPF; (b): difference result when using CC trace-transform-based 2D TFPF.
4 Conclusion
This paper focuses on varying the filtering direction of the 2D TFPF and proposes a CC trace transform to find the optimal temporal–spatial radial traces in the seismic record. In the CC trace transform, we utilize the similarity of data on the reflection event and construct a particular format to significantly enhance the energy when the radial trace is aligned with the reflection event. The sharp energy contrast makes it possible to track the temporal–spatial direction by seeking the local maximum value point and the corresponding parameter in the CC trace transform domain. Both synthetic and field data demonstrate that the proposed CC trace-transform-based 2D-TFPF performs better in the discrimination of the reflected energy, the preservation of the peak amplitudes, and the continuity of the reflection event, in addition to the principal objective of random noise attenuation, making the seismic record more reliable for further interpretation.
Acknowledgment
This paper is supported by National Natural Science Foundation of China (No. 41304085, No. 41130421, and No. 41404081).