We start from the contractive functional equation proposed in [4], where it was shown that the polynomial solution of functional equation can be used to initialize a Neural Network structure, with a controlled accuracy. We propose a novel algorithm, where the functional equation is solved with a converging iterative algorithm which can be realized as a Machine Learning training method iteratively with respect to the number of layers. The proof of convergence is performed with respect to the
Accepté le :
Accepté après révision le :
Publié le :
Bruno Després 1
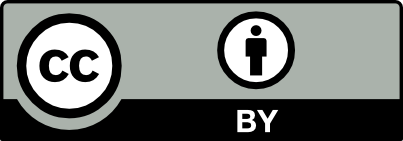
@article{CRMATH_2023__361_G6_1029_0, author = {Bruno Despr\'es}, title = {A convergent {Deep} {Learning} algorithm for approximation of polynomials}, journal = {Comptes Rendus. Math\'ematique}, pages = {1029--1040}, publisher = {Acad\'emie des sciences, Paris}, volume = {361}, year = {2023}, doi = {10.5802/crmath.462}, language = {en}, }
Bruno Després. A convergent Deep Learning algorithm for approximation of polynomials. Comptes Rendus. Mathématique, Volume 361 (2023), pp. 1029-1040. doi : 10.5802/crmath.462. https://comptes-rendus.academie-sciences.fr/mathematique/articles/10.5802/crmath.462/
[1] Deep Learning with Python, Manning Shelter Island, 2018
[2] Nonlinear Approximation and (Deep) ReLU Networks, Computing, Volume 55/1 (2022), pp. 127-172 | Zbl
[3] Neural Networks and Numerical Analysis, Walter de Gruyter, 2022 | DOI
[4] A functional equation with polynomial solutions and application to Neural Networks, C. R. Math. Acad. Sci. Paris, Volume 358 (2020) no. 9-10, pp. 1059-1072 | Numdam | MR | Zbl
[5] Nonlinear Approximation by Deep ReLU Neural Networks (2019) (https://devore2019.sciencesconf.org/resource/page/id/1)
[6] Constructive Approximation, Grundlehren der Mathematischen Wissenschaften, 303, Springer, 1993 | DOI
[7] Deep Learning, MIT Press, 2016 (http://www.deeplearningbook.org)
[8] The Takagi Function and Its Generalization, Japan J. Appl. Math., Volume 1 (1984) no. 1, pp. 83-199 | MR | Zbl
[9] ReLU Deep Neural Networks and Linear Finite Elements, J. Comput. Math., Volume 38 (2020) no. 3, pp. 502-527 | MR | Zbl
[10] Convex analysis and minimization algorithms. I, 305, Springer, 1993
[11] Quand la machine apprend, Odile Jacob, 2019
[12] Better approximations of high dimensional smooth functions by deep neural networks with rectified power units, Commun. Comput. Phys., Volume 27 (2020) no. 2, pp. 379-411 | MR | Zbl
[13] Deep ReLU networks and high-order finite element methods, Anal. Appl., Singap., Volume 18 (2020) no. 5, pp. 715-770 | DOI | MR | Zbl
[14] The convergence of the Stochastic Gradient Descent (SGD) : a self-contained proof (2021) | arXiv
[15] Representation formulas and pointwise properties for Barron functions (2021) | arXiv
[16] Error bounds for approximations with deep ReLU networks, Neural Netw., Volume 94 (2017), pp. 103-114 | DOI | Zbl
Cité par Sources :
Commentaires - Politique
Vous devez vous connecter pour continuer.
S'authentifier