Étant donné une mesure de probabilité (multivariée) nous caractérisons les mélanges de Gaussiennes qui minimisent la distance de Wasserstein quand la probabilité de mélange est sur un compact . (i) On montre d’abord que de telles probabilités de mélange sont solutions optimales d’un problème de transport où la marginale () est elle-même une inconnue via la probabilité de mélange . (ii) Ensuite en utilisant une propriété bien connue des Gaussiennes, ce problème de transport est lui-même vu comme un problème de moments généralisé. Si l’ensemble des paramètres admissibles est un semi-algébrique de base compact, alors on fournit un schéma numérique sans grille de discrétisation (la hiérarchie « moments – sommes-de-carrés »), pour approximer arbitrairement près la distance minimum optimale. Si la mesure n’est pas un mélange de Gaussiennes (avec paramètres dans ) alors une distance strictement positive est détectée à une certaine relaxation de la hiérarchie. Si est un mélange (fini) de Gaussiennes, alors une mesure de mélange atomique peut parfois être extraite de la solution optimale d’une relaxation quand la condition de « flatness » de Curto& Fiakow est satisfaite pour une matrice de moments.
Given a determinate (multivariate) probability measure , we characterize Gaussian mixtures which minimize the Wasserstein distance to when the mixing probability measure on the parameters of the Gaussians is supported on a compact set . (i) We first show that such mixtures are optimal solutions of a particular optimal transport (OT) problem where the marginal of the OT problem is also unknown via the mixing measure variable . Next (ii) by using a well-known specific property of Gaussian measures, this optimal transport is then viewed as a Generalized Moment Problem (GMP) and if the set of mixture parameters is a basic compact semi-algebraic set, we provide a “mesh-free” numerical scheme to approximate as closely as desired the optimal distance by solving a hierarchy of semidefinite relaxations of increasing size. In particular, we neither assume that the mixing measure is finitely supported nor that the variance is the same for all components. If the original measure is not a Gaussian mixture with parameters , then a strictly positive distance is detected at a finite step of the hierarchy. If the original measure is a Gaussian mixture with parameters , then all semidefinite relaxations of the hierarchy have same zero optimal value. Moreover if the mixing measure is atomic with finite support, its components can sometimes be extracted from an optimal solution at some semidefinite relaxation of the hierarchy when Curto & Fialkow’s flatness condition holds for some moment matrix.
Révisé le :
Accepté le :
Publié le :
DOI : 10.5802/crmath.657
Keywords: Gaussian mixtures, Wasserstein distance, semidefinite relaxations, Moment-SOS hierarchy
Mots-clés : Mélanges de Gaussiennes, distance de Wasserstein, relaxations semidéfinies, hiérarchie moments-sommes-de-carrés
Jean B. Lasserre 1
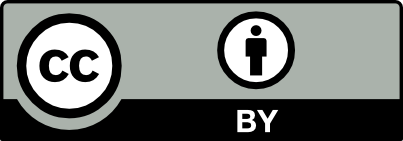
@article{CRMATH_2024__362_G11_1455_0, author = {Jean B. Lasserre}, title = {Gaussian mixtures closest to a given measure via optimal transport}, journal = {Comptes Rendus. Math\'ematique}, pages = {1455--1473}, publisher = {Acad\'emie des sciences, Paris}, volume = {362}, year = {2024}, doi = {10.5802/crmath.657}, zbl = {07945488}, language = {en}, }
Jean B. Lasserre. Gaussian mixtures closest to a given measure via optimal transport. Comptes Rendus. Mathématique, Volume 362 (2024), pp. 1455-1473. doi : 10.5802/crmath.657. https://comptes-rendus.academie-sciences.fr/mathematique/articles/10.5802/crmath.657/
[1] Moment varieties of Gaussian mixtures, J. Algebr. Stat., Volume 7 (2016) no. 1, pp. 14-28 | DOI | MR | Zbl
[2] Handbook on semidefinite, conic and polynomial optimization (Miguel F. Anjos; Jean B. Lasserre, eds.), International Series in Operations Research & Management Science, 166, Springer, 2012, xii+960 pages | DOI | MR | Zbl
[3] Algebraic identifiability of Gaussian mixtures, Int. Math. Res. Not., Volume 2018 (2018) no. 21, pp. 6556-6580 | DOI | MR | Zbl
[4] Estimation and inference for the Wasserstein distance between mixing measures in topic models (2023) (https://arxiv.org/abs/2206.12768)
[5] Robustly learning mixtures of arbitrary Gaussians, STOC ’22—Proceedings of the 54th Annual ACM SIGACT Symposium on Theory of Computing, Association for Computing Machinery (2022), pp. 1234-1247 | DOI | MR | Zbl
[6] Orthogonal polynomials of several variables, Encyclopedia of Mathematics and Its Applications, 81, Cambridge University Press, 2001, xvi+390 pages | DOI | MR | Zbl
[7] A mixture of mixture models for a classification problem: the unity measure error, Comput. Stat. Data Anal., Volume 51 (2007) no. 5, pp. 2573-2585 | DOI | MR | Zbl
[8] Strong identifiability and optimal minimax rates for finite mixture estimation, Ann. Stat., Volume 46 (2018) no. 6A, pp. 2844-2870 | DOI | MR | Zbl
[9] The moment-SOS hierarchy—lectures in probability, statistics, computational geometry, control and nonlinear PDEs, Series on Optimization and its Applications, 4, World Scientific, 2021, xvii+229 pages | MR | Zbl
[10] GloptiPoly 3: moments, optimization and semidefinite programming, Optim. Methods Softw., Volume 24 (2009) no. 4-5, pp. 761-779 | DOI | MR | Zbl
[11] Efficiently learning mixtures of two Gaussians, STOC’10—Proceedings of the 2010 ACM International Symposium on Theory of Computing, Association for Computing Machinery (2010), pp. 553-562 | DOI | MR | Zbl
[12] Polynomial-time sum-of-squares can robustly estimate mean and covariance of Gaussians optimally, Proceedings of The 33rd International Conference on Algorithmic Learning Theory (Sanjoy Dasgupta; Nika Haghtalab, eds.) (Proceedings of Machine Learning Research), Volume 167, PMLR (2022), pp. 638-667 | MR
[13] Moments, positive polynomials and their applications, Imperial College Press Optimization Series, 1, Imperial College Press, 2010, xxii+361 pages | MR | Zbl
[14] The existence of Gaussian cubature formulas, J. Approx. Theory, Volume 164 (2012) no. 5, pp. 572-585 | DOI | MR | Zbl
[15] An introduction to polynomial and semi-algebraic optimization, Cambridge Texts in Applied Mathematics, Cambridge University Press, 2015, xiv+339 pages | DOI | MR | Zbl
[16] Moment matrices: applications in mixtures, Ann. Stat., Volume 17 (1989) no. 2, pp. 722-740 | DOI | MR | Zbl
[17] Finite mixture models, Wiley Ser. Probab. Math. Stat., John Wiley & Sons, 2000 | DOI | MR | Zbl
[18] Settling the polynomial learnability of mixtures of Gaussians, 2010 IEEE 51st Annual Symposium on Foundations of Computer Science—FOCS 2010, IEEE Computer Society (2010), pp. 93-102 | DOI | MR
[19] Exact mean integrated squared error, Ann. Stat., Volume 20 (1992) no. 2, pp. 712-736 | DOI | MR | Zbl
[20] On square positive extensions and cubature formulas, J. Comput. Appl. Math., Volume 199 (2007) no. 1, pp. 80-88 | DOI | MR | Zbl
[21] Convergence of latent mixing measures in finite and infinite mixture models, Ann. Stat., Volume 41 (2013) no. 1, pp. 370-400 | DOI | MR | Zbl
[22] SOS is not obviously automatizable, even approximately, 8th Innovations in Theoretical Computer Science Conference (LIPIcs. Leibniz Int. Proc. Inform.), Volume 67, Schloss Dagstuhl. Leibniz-Zent. Inform., 2017 (No. 59, 10 pages) | DOI | MR | Zbl
[23] A study of Gaussian mixture models of color and texture features for image classification and segmentation, Pattern Recognition, Volume 39 (2006) no. 4, pp. 695-706 | DOI | Zbl
[24] Positive polynomials on compact semi-algebraic sets, Indiana Univ. Math. J., Volume 42 (1993) no. 3, pp. 969-984 | DOI | MR | Zbl
[25] Generating daily changes in market variables using a multivariate mixture of normal distributions, Proceeding of the 2001 Winter Simulation Conference (Cat. No.01CH37304) (2001), pp. 283-289 | DOI
[26] Optimal estimation of Gaussian mixtures via denoised method of moments, Ann. Stat., Volume 48 (2020) no. 4, pp. 1981-2007 | DOI | MR | Zbl
[27] Solving inverse problems with piecewise linear estimators: from Gaussian mixture models to structured sparsity, IEEE Trans. Image Process., Volume 21 (2012) no. 5, pp. 2481-2499 | DOI | MR | Zbl
Cité par Sources :
Commentaires - Politique