Shape memory alloy (SMA) actuators are an important application of smart materials for robotics. However, the nonlinear behavior of SMA leads to difficulties in real-time simulations using numerical methods. Artificial Intelligence can be used to bypass this problem. In this paper, we study several neural networks (NNs) to model the superelastic or pseudo-elasticity effect (SEE) as well as the shape memory effect (SME) used in SMA. Focusing on antagonistic actuating, we first model a single wire to train the best NN with the proper characteristics that fit the behavior of SEE. Then, we model the SME of two linear antagonistic SMA wires used as an actuator. In both systems, single and antagonistic wires, we train the networks to obtain the stress–strain diagrams representing the behavior. The network type and training algorithm are key factors and are evaluated depending on the RMSE values. As a result, we find that the long short-term memory NN, used with a regression layer on standardized data sets, models the butterfly-shaped behavior of the actuator system with less RMSE value.
Révisé le :
Accepté le :
Publié le :
Rodayna Hmede 1 ; Frédéric Chapelle 1 ; Yuri Lapusta 1
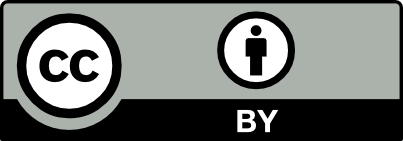
@article{CRMECA_2022__350_G1_143_0, author = {Rodayna Hmede and Fr\'ed\'eric Chapelle and Yuri Lapusta}, title = {Modeling the butterfly behavior of {SMA} actuators using neural networks}, journal = {Comptes Rendus. M\'ecanique}, pages = {143--157}, publisher = {Acad\'emie des sciences, Paris}, volume = {350}, year = {2022}, doi = {10.5802/crmeca.108}, language = {en}, }
TY - JOUR AU - Rodayna Hmede AU - Frédéric Chapelle AU - Yuri Lapusta TI - Modeling the butterfly behavior of SMA actuators using neural networks JO - Comptes Rendus. Mécanique PY - 2022 SP - 143 EP - 157 VL - 350 PB - Académie des sciences, Paris DO - 10.5802/crmeca.108 LA - en ID - CRMECA_2022__350_G1_143_0 ER -
Rodayna Hmede; Frédéric Chapelle; Yuri Lapusta. Modeling the butterfly behavior of SMA actuators using neural networks. Comptes Rendus. Mécanique, Volume 350 (2022), pp. 143-157. doi : 10.5802/crmeca.108. https://comptes-rendus.academie-sciences.fr/mecanique/articles/10.5802/crmeca.108/
[1] Smart materials, precision sensors/actuators, smart structures, and structronic systems, Mech. Adv. Mater. Struct., Volume 11 (2004) no. 4–5, pp. 367-393 | DOI
[2] Mechatronic design of a shape memory alloy actuator for automotive tumble flaps: a case study, IEEE Trans. Ind. Electron., Volume 56 (2009) no. 7, pp. 2644-2656 | DOI
[3] Self-centering and damping devices using SMA dual rings, Smart Mater. Struct., Volume 28 (2019) no. 8, 085005 | DOI
[4] A review on recent advances in soft surgical robots for endoscopic applications, Int. J. Med. Robot., Volume 15 (2019) no. 5, e2010 | DOI
[5] Superelastic NiTi SMA cables: Thermal-mechanical behavior, hysteretic modelling and seismic application, Eng. Struct., Volume 183 (2019), pp. 533-549 | DOI
[6] Shape memory alloys: a state of art review, IOP Conf. Ser. Mater. Sci. Eng., Volume 149 (2016), 012054 | DOI
[7] The superelastic anisotropy in a NiTi shape memory alloy thin sheet, Acta Mater., Volume 95 (2015), pp. 411-427 | DOI
[8] A mathematical model for the hysteresis in shape memory alloys, Contin. Mech. Thermodyn., Volume 1 (1989) no. 4, pp. 283-303 | DOI | MR
[9] Predicting the orientation-dependent stress-induced transformation and detwinning response of shape memory alloy single crystals, Metall. Mater. Trans. A, Volume 27 (1996) no. 2, pp. 269-279 | DOI
[10] Superelastic and cyclic response of NiTi SMA at various strain rates and temperatures, Mech. Mater., Volume 38 (2006) no. 5–6, pp. 463-474 | DOI
[11] Evaluation of the performance of a sliding-type base isolation system with a NiTi shape memory alloy device considering temperature effects, Eng. Struct., Volume 32 (2010) no. 1, pp. 238-249 | DOI
[12] Neural network application for robotic motion control-adaptation and learning, 1990 IJCNN International Joint Conference on Neural Networks, Volume 2, 1990, pp. 447-451 | DOI
[13] Robot control using neural networks, IEEE 1988 International Conference on Neural Networks, Volume 2, Neural Systems, Inc., Vancouver, BC, Canada, 1988, pp. 625-631
[14] Identification and decentralized adaptive control using dynamical neural networks with application to robotic manipulators, IEEE Trans. Neural Netw., Volume 4 (1993) no. 6, pp. 919-930 | DOI
[15] Optimal design of CMAC neural-network controller for robot manipulators, IEEE Trans. Syst. Man Cybern. Part C Appl. Rev., Volume 30 (2000) no. 1, pp. 22-31 | DOI
[16] Neural network architectures for robotic applications, IEEE Trans. Robot. Autom., Volume 5 (1989) no. 5, pp. 641-657 | DOI
[17] Neural network-based adaptive controller design of robotic manipulators with an observer, IEEE Trans. Neural Netw., Volume 12 (2001) no. 1, pp. 54-67 | DOI
[18] Smart materials, precision sensors/actuators, smart structures, and structronic systems, Mech. Adv. Mater. Struct., Volume 11 (2004) no. 4–5, pp. 367-393 | DOI
[19] A hysteresis functional link artificial neural network for identification and model predictive control of SMA actuator, J. Process Control, Volume 22 (2012) no. 4, pp. 766-777 | DOI
[20] Artificial neural network model for deflection analysis of superelastic shape memory alloy reinforced concrete beams, Can. J. Civ. Eng., Volume 37 (2010) no. 6, pp. 855-865 | DOI
[21] On the complexity of loading shallow neural networks, J. Complex., Volume 4 (1988) no. 3, pp. 177-192 | DOI | MR | Zbl
[22] Neural network direct control with online learning for shape memory alloy manipulators, Sensors, Volume 19 (2019) no. 11, 2576 | DOI
[23] Finite element analysis of a prestressed mechanism with multi-antagonistic and hysteretic SMA actuation, Meccanica, Volume 55 (2020) no. 5, pp. 1007-1024 | DOI | MR
[24] Advanced shape memory alloy material models for ANSYS, 2016 https://www.ozeninc.com/wp-content/uploads/2016/01/Advanced-Shape-Memory-Alloy-Material-Models-for-ANSYS.pdf
[25] Création de structures actives à l’aide d’alliages à mémoire de forme, Ph. D. Thesis, Blaise Pascal University (2016)
Cité par Sources :
Commentaires - Politique