This paper presents an empirical model for predicting the uniaxial compressive strength (UCS) of rocks using gene expression programming (GEP). A total of 44 datasets collected from the literature was used to construct the GEP model. The GEP model developed is evaluated using four conventional regression models and an artificial neural network (ANN) model in terms of three statistical indices. The comparison results confirmed that the proposed GEP model has the lowest root mean square error (RMSE) and the highest coefficient of determination () and correlation coefficient () values compared to the four conventional regression models and the ANN model in the literature. It is concluded that the proposed GEP model can be applied to predict the UCS of rocks.
Révisé le :
Accepté le :
Publié le :
Xinhua Xue 1
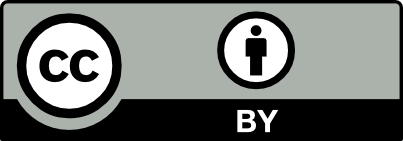
@article{CRMECA_2022__350_G1_159_0, author = {Xinhua Xue}, title = {A novel model for prediction of uniaxial compressive strength of rocks}, journal = {Comptes Rendus. M\'ecanique}, pages = {159--170}, publisher = {Acad\'emie des sciences, Paris}, volume = {350}, year = {2022}, doi = {10.5802/crmeca.109}, language = {en}, }
Xinhua Xue. A novel model for prediction of uniaxial compressive strength of rocks. Comptes Rendus. Mécanique, Volume 350 (2022), pp. 159-170. doi : 10.5802/crmeca.109. https://comptes-rendus.academie-sciences.fr/mecanique/articles/10.5802/crmeca.109/
[1] Determination of instantaneous breaking rate by geological strength index, block punch index and power of impact hammer for various rock mass conditions, Tunn. Undergr. Space Technol., Volume 26 (2011), pp. 534-540 | DOI
[2] An example for estimation of rock mass deformations around an underground opening by using numerical modeling, Int. J. Rock Mech. Min. Sci., Volume 47 (2010), pp. 272-278 | DOI
[3] Predicting uniaxial compressive strength by point load test: significance of cone penetration, Rock Mech. Rock Eng., Volume 39 (2006) no. 5, pp. 483-490 | DOI
[4] Use of the block punch test to predict the compressive and tensile strengths of rocks, Int. J. Rock Mech. Min. Sci., Volume 51 (2012), pp. 119-127 | DOI
[5] Estimation of uniaxial compressive strength of rock materials by index tests using regression analysis and fuzzy inference system, Eng. Geol., Volume 160 (2013), pp. 54-68 | DOI
[6] A new testing method for indirect determination of the unconfined compressive strength of rocks, Int. J. Rock Mech. Min. Sci., Volume 46 (2009), pp. 1349-1357 | DOI
[7] Brazilizan tensile strength tests on some anisotropic rocks, Int. J. Rock Mech. Min. Sci., Volume 58 (2013), pp. 1-7 | DOI
[8] Soft computing methods for estimating the uniaxial compressive strength of intact rock from index tests, Int. J. Rock Mech. Min. Sci., Volume 80 (2015), pp. 418-424 | DOI
[9] A visual determination method for uniaxial compressive strength estimation based on Croatia carbonate rock materials, Eng. Geol., Volume 231 (2017), pp. 68-80 | DOI
[10] Assessing the uniaxial compressive strength of extremely hard cryptocrystalline flint, Int. J. Rock Mech. Min. Sci., Volume 113 (2019), pp. 310-321 | DOI
[11] Prediction of strength properties of some schistose rocks from petrographic properties using artificial neural networks, Int. J. Rock Mech. Min. Sci., Volume 38 (2001), pp. 269-284 | DOI
[12] An example of artificial neural network (ANN) application for indirect estimation of rock parameters, Rock Mech. Rock Eng., Volume 41 (2007) no. 5, pp. 781-795 | DOI
[13] Prediction of compressive and tensile strength of Gaziantep basalts via neural networks and gene expression programming, Neural Comput. Appl., Volume 18 (2009), pp. 1031-1041 | DOI
[14] Modelling of the uniaxial compressive strength of some clay-bearing rocks using neural network, Appl. Soft Comput., Volume 11 (2011), pp. 2587-2594 | DOI
[15] Artificial neural networks and nonlinear regression techniques to assess the influence of slake durability cycles on the prediction of uniaxial compressive strength and modulus of elasticity for carbonate rocks, Int. J. Numer. Anal. Meth. Geomech., Volume 36 (2012), pp. 1636-1650 | DOI
[16] An application of adaptive neuro fuzzy inference system for estimating the uniaxial compressive strength of certain granitic rocks from their mineral contents, Expert Syst. Appl., Volume 40 (2013a), pp. 921-928 | DOI
[17] Prediction of uniaxial compressive strength of granitic rocks by various nonlinear tools and comparison of their performances, Int. J. Rock Mech. Min. Sci., Volume 62 (2013b), pp. 113-122 | DOI
[18] Comparative evaluation of artificial intelligence models for prediction of uniaxial compressive strength of travertine rocks, Case study: Azarshahr area, NW Iran, Model. Earth Syst. Environ., Volume 2 (2016), 76 | DOI
[19] Comparison of models for estimating uniaxial compressive strength of some sedimentary rocks from Qom Formation, Environ. Earth Sci., Volume 76 (2017), 753 | DOI
[20] Application of fuzzy inference system to predict uniaxial compressive strength and elastic modulus of migmatites, Environ. Earth Sci., Volume 78 (2019), 208 | DOI
[21] Comparison of three updating schemes using artificial neural network in flow forecasting, Hydrol. Earth Syst. Sci., Volume 8 (2004) no. 2, pp. 247-255 | DOI
[22] Application of artificial neural networks in groundwater table forecasting-a case study in a Singapore swamp forest, Hydrol. Earth Syst. Sci., Volume 20 (2016), pp. 1405-1412 | DOI
[23] Gene expression programming: a new adaptive algorithm for solving problems, Complex Syst., Volume 13 (2001) no. 2, pp. 87-129 | MR | Zbl
[24] Gene Expression Programming: Mathematical Modeling by an Artificial Intelligence, Springer-Verlag, Berlin, Heidelberg, 2006
[25] Lightweight concrete design using gene expression programing, Constr. Build. Mater., Volume 139 (2017), pp. 93-100 | DOI
[26] Predictive model to the bond strength of FRP-to concrete under direct pullout using gene expression programming, J. Civ. Eng. Manag., Volume 25 (2019) no. 8, pp. 773-784
[27] The Schmidt hammer in rock material characterization, Eng. Geol., Volume 81 (2005), pp. 1-14 | DOI
[28] Mechanical characterization of Granitic rocks of Hong Kong by improved index testing procedures with reference to weathering induced microstructural changes, Ph. D. Thesis, The University of Hong Kong (2006)
[29] Petrophysical and geomechanical properties of rocks from the oilfields of the Krishna–Godavari and Cauvery Basins, India, Bull. Eng. Geol. Environ., Volume 61 (2002), pp. 169-178 | DOI
[30] The effect of porosity on the relation between uniaxial compressive strength and point load index, Int. J. Rock Mech. Min. Sci., Volume 42 (2005), pp. 584-589 | DOI
[31] High performance prediction of soil compaction parameters using multi expression programming, Eng. Geol., Volume 276 (2020), 105758
[32] A novel hybrid surrogate intelligent model for creep index prediction based on particle swarm optimization and random forest, Eng. Geol., Volume 265 (2020), 105328 | DOI
Cité par Sources :
Commentaires - Politique