We numerically investigate an adaptive version of the parareal algorithm in the context of molecular dynamics. This adaptive variant has been originally introduced in [1]. We focus here on test cases of physical interest where the dynamics of the system is modelled by the Langevin equation and is simulated using the molecular dynamics software LAMMPS. In this work, the parareal algorithm uses a family of machine-learning spectral neighbor analysis potentials (SNAP) as fine, reference, potentials and embedded-atom method potentials (EAM) as coarse potentials. We consider a self-interstitial atom in a tungsten lattice and compute the average residence time of the system in metastable states. Our numerical results demonstrate significant computational gains using the adaptive parareal algorithm in comparison to a sequential integration of the Langevin dynamics. We also identify a large regime of numerical parameters for which statistical accuracy is reached without being a consequence of trajectorial accuracy.
Révisé le :
Accepté le :
Première publication :
Publié le :
Olga Gorynina 1, 2 ; Frédéric Legoll 3, 2 ; Tony Lelièvre 1, 2 ; Danny Perez 4
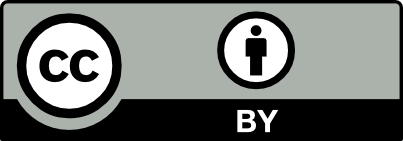
@article{CRMECA_2023__351_S1_479_0, author = {Olga Gorynina and Fr\'ed\'eric Legoll and Tony Leli\`evre and Danny Perez}, title = {Combining machine-learned and empirical force fields with the parareal algorithm: application to the diffusion of atomistic defects}, journal = {Comptes Rendus. M\'ecanique}, pages = {479--503}, publisher = {Acad\'emie des sciences, Paris}, volume = {351}, number = {S1}, year = {2023}, doi = {10.5802/crmeca.220}, language = {en}, }
TY - JOUR AU - Olga Gorynina AU - Frédéric Legoll AU - Tony Lelièvre AU - Danny Perez TI - Combining machine-learned and empirical force fields with the parareal algorithm: application to the diffusion of atomistic defects JO - Comptes Rendus. Mécanique PY - 2023 SP - 479 EP - 503 VL - 351 IS - S1 PB - Académie des sciences, Paris DO - 10.5802/crmeca.220 LA - en ID - CRMECA_2023__351_S1_479_0 ER -
%0 Journal Article %A Olga Gorynina %A Frédéric Legoll %A Tony Lelièvre %A Danny Perez %T Combining machine-learned and empirical force fields with the parareal algorithm: application to the diffusion of atomistic defects %J Comptes Rendus. Mécanique %D 2023 %P 479-503 %V 351 %N S1 %I Académie des sciences, Paris %R 10.5802/crmeca.220 %G en %F CRMECA_2023__351_S1_479_0
Olga Gorynina; Frédéric Legoll; Tony Lelièvre; Danny Perez. Combining machine-learned and empirical force fields with the parareal algorithm: application to the diffusion of atomistic defects. Comptes Rendus. Mécanique, The scientific legacy of Roland Glowinski, Volume 351 (2023) no. S1, pp. 479-503. doi : 10.5802/crmeca.220. https://comptes-rendus.academie-sciences.fr/mecanique/articles/10.5802/crmeca.220/
[1] An adaptive parareal algorithm: application to the simulation of molecular dynamics trajectories, SIAM J. Sci. Comput., Volume 44 (2022) no. 1, p. B146-B176 | DOI | MR | Zbl
[2] Free Energy Computations. A mathematical perspective, Imperial College Press, 2010 | DOI | Zbl
[3] Computational methods for long-timescale atomistic simulations, Handbook of Materials Modeling: Method: Theory and Modeling (W. Andreoni; S. Yip, eds.), Springer, 2020, pp. 683-688 | DOI
[4] Accelerated molecular dynamics methods in a massively parallel world, Handbook of Materials Modeling: Methods: Theory and Modeling (W. Andreoni; S. Yip, eds.), Springer, 2020, pp. 745-772 | DOI
[5] Résolution d’EDP par un schéma en temps pararéel (A “parareal” in time discretization of PDE’s), C. R. Acad. Sci. Paris Sér. I Math., Volume 332 (2001) no. 7, pp. 661-668 | DOI | Zbl
[6] A micro-macro parareal algorithm: application to singularly perturbed ordinary differential equations, SIAM J. Sci. Comput., Volume 35 (2013) no. 4, p. A1951-A1986 | DOI | MR | Zbl
[7] LAMMPS – a flexible simulation tool for particle-based materials modeling at the atomic, meso, and continuum scales, Comput. Phys. Commun., Volume 271 (2022), 108171 | DOI | Zbl
[8] Spectral neighbor analysis method for automated generation of quantum-accurate interatomic potentials, J. Comput. Phys., Volume 285 (2015), pp. 316-330 | DOI | MR | Zbl
[9] Embedded-atom method: Derivation and application to impurities, surfaces, and other defects in metals, Phys. Rev. B, Volume 29 (1984) no. 12, pp. 6443-6453 | DOI
[10] Stochastic boundary conditions for molecular dynamics simulations of ST2 water, Chem. Phys. Lett., Volume 105 (1984) no. 5, pp. 495-500 | DOI
[11] A parareal time discretization for nonlinear PDE’s with application to the pricing of an American put, Recent developments in domain decomposition methods (L. F. Pavarino; A. Toselli, eds.) (Lecture Notes in Computational Science and Engineering), Volume 23, Springer, 2002, pp. 189-202 | DOI | Zbl
[12] Analysis of the parareal time-parallel time-integration method, SIAM J. Sci. Comput., Volume 29 (2007), pp. 556-578 | DOI | MR | Zbl
[13] A unified analysis framework for iterative parallel-in-time algorithms, SIAM J. Sci. Comput., Volume 45 (2023) no. 5, p. A2275-A2303 | DOI | MR | Zbl
[14] 50 years of time parallel time integration, Multiple Shooting and Time Domain Decomposition Methods (T. Carraro; M. Geiger; S. Körkel; R. Rannacher, eds.) (Contributions in Mathematical and Computational Sciences), Volume 9, Springer, 2015, pp. 69-114 | DOI | MR | Zbl
[15] Parallel in time algorithms with reduction methods for solving chemical kinetics, Commun. Appl. Math. Comput. Sci., Volume 5 (2010) no. 2, pp. 241-263 | DOI | MR | Zbl
[16] Parareal in time algorithm for kinetic systems based on model reduction, High-dimensional partial differential equations in science and engineering (A. Bandrauk; M. C. Delfour; C. Le Bris, eds.) (CRM Proceedings & Lecture Notes), Volume 41, American Mathematical Society, 2007, pp. 183-194 | MR | Zbl
[17] Parallel in time simulation of multiscale stochastic chemical kinetics, Multiscale Model. Simul., Volume 8 (2009), pp. 46-68 | DOI | MR | Zbl
[18] Symmetric parareal algorithms for Hamiltonian systems, ESAIM, Math. Model. Numer. Anal., Volume 47 (2013) no. 3, pp. 717-742 | DOI | Numdam | MR | Zbl
[19] Stable parareal in time method for first- and second-order hyperbolic systems, SIAM J. Sci. Comput., Volume 35 (2013) no. 1, p. A52-A78 | DOI | MR | Zbl
[20] Parallelization in time of (stochastic) ordinary differential equations (Preprint available at https://www.stat.uchicago.edu/~guillaumebal/PAPERS/paralleltime.pdf)
[21] The parareal algorithm for American options, C. R. Acad. Sci. Paris Sér. I Math., Volume 354 (2016) no. 11, pp. 1132-1138 | DOI | Numdam | MR | Zbl
[22] Parareal computation of stochastic differential equations with time-scale separation: a numerical convergence study, Comput. Vis. Sci., Volume 23 (2020), 9 | DOI | MR | Zbl
[23] A convergent algorithm for time parallelization applied to reservoir simulation, Domain decomposition methods in science and engineering (R. Kornhuber; R. Hoppe; J. Périaux; O. Pironneau; O. Widlund; J. Xu, eds.) (Lecture Notes in Computational Science and Engineering), Volume 40, Springer, 2005, pp. 469-476 | DOI | MR | Zbl
[24] Time-decomposed parallel time-integrators: theory and feasibility studies for fluid, structure, and fluid–structure applications, Int. J. Numer. Methods Eng., Volume 58 (2003) no. 9, pp. 1397-1434 | DOI | MR | Zbl
[25] Parallel in time algorithms for nonlinear iterative methods, ESAIM, Proc. Surv., Volume 63 (2018), pp. 248-257 | DOI | MR | Zbl
[26] An adaptive parareal algorithm, J. Comput. Appl. Math., Volume 377 (2020), 112915 | DOI | MR | Zbl
[27] Random numbers for parallel computers: Requirements and methods, with emphasis on GPUs, Math. Comput. Simul., Volume 135 (2017), pp. 3-17 | DOI | MR | Zbl
[28] Visualization and analysis of atomistic simulation data with OVITO – the Open Visualization Tool, Model. Simul. Mat. Sci. Eng., Volume 18 (2010) no. 1, 015012 | DOI
- Parareal with a Physics-Informed Neural Network as Coarse Propagator, Euro-Par 2023: Parallel Processing, Volume 14100 (2023), p. 649 | DOI:10.1007/978-3-031-39698-4_44
Cité par 1 document. Sources : Crossref
Commentaires - Politique