1 Introduction
The genus Lycium L. (Solanaceae) comprises approximately 70 species of spiny shrubs and small trees. The fruit of the Lycium species are all red in color, with very similar physical appearance and anatomical structure. Most species occur in arid and subarid regions, but some occur in subsaline regions or along the seacoast [1–3]. Lycium chinense Mill. and Lycium barbarum are perennial foliage plants endemic to Korea, Japan, and China and are widely used for medicinal purposes with a history of almost 2000 years’ use [4,5]. Lycii fructus, Lycii folium, and Lycii cortex of L. chinense contain betaine, rutin, tocopherols, chlorogenic acid, kukoamine A, b-sitosterol, and various fatty acids [6–8]. These plants, especially L. chinense, have been used to replenish the vital essence of the liver and kidney and to improve eyesight. Chinese physicians also prescribe them to strengthen muscles and bones [9].
L. chinense is well known as a key medicinal plant, and knowledge of germplasm genetic diversity and population structure are critical for its utilization in genotype identification and genetic improvement [10]. Traditionally, L. chinense genotypes have been authenticated by morphological and histological analyses. Recently, chemical analysis methods such as high-performance liquid chromatography have also been used for different Lycium species, but these have failed to distinguish closely related species due to similar chemical compounds [11]. Peng et al. [12] established a Fourier-transform infrared spectroscopy method to identify seven species and three varieties of Lycium. With the rapid development of modern biological methods, identification of species relationships using traditional anatomical and physiochemical methods is being supplemented by DNA fingerprinting techniques. In recent years, DNA-based molecular markers, such as random amplified polymorphic DNA (RAPD), sequenced characterized amplified regions (SCAR), and chloroplast and internal transcribed spacer DNA sequences [4,13–18] have been used to authenticate the species and analyze genetic variation. Due to their high polymorphism, co-dominance, and reproducibility, microsatellite or simple sequence repeat (SSR) markers have proved to be highly efficient molecular tools for marker-assisted selection, the analysis of genetic diversity, population genetic analysis, tracking desirable traits in large-scale breeding programs, as anchor points for map-based gene cloning strategies, and for other purposes in various species. However, so far, only a minor attempt has been made to isolate and characterize L. chinense SSRs [19]. It is important to understand genetic variation and genetic structure for conservation and sustainable use of Lycium species. In the present study, we used the Structure software program [20] to evaluate the genetic diversity and population structure of 139 accessions of L. chinense using a set of 18 newly developed microsatellite markers. The information may provide a more rational basis for expanding the gene pool and for identifying materials harboring valuable alleles to improve L. chinense.
2 Materials and methods
2.1 Plant materials
One-hundred and thirty nine L. chinense accessions, originating from four different countries, were obtained from the National Genebank of the Rural Development Administration (RDA-Genebank), Republic of Korea. The samples were mainly from the Republic of Korea (120) and China (17). A description of the accessions used in this study is shown in Table 1.
List of the 139 Lycium chinense accessions used in this study and their model-based groupings.
S. no. | Cultivar name or collection region | Origin | Model-based Subpopulationa |
1 | Yuseong1 | Korea | Admixture |
2 | Yuseong2 | Japan | S1 |
3 | Cheongyangjaerae | Korea | S2 |
4 | Jinbujaerae | Korea | S2 |
5 | Jindojaerae | Korea | S2 |
6 | Keumsanjaerae | Korea | S2 |
7 | Haenamjaerae | Korea | S3 |
8 | Collected from China | China | S3 |
9 | Collected from China | China | S1 |
10 | Collected from China | China | S3 |
11 | Myeonan | Korea | S1 |
12 | Bulro | Korea | S1 |
13 | Cheongdae | Korea | S1 |
14 | Jangmyeong | Korea | S1 |
15 | Cheongun | Korea | S1 |
16 | Cheongyang6 | Korea | S1 |
17 | Cheongyang7 | Korea | S2 |
18 | CL129-145 | Korea | S1 |
19 | CL124-23 | Korea | S1 |
20 | CL129-161 | Korea | S1 |
21 | CL7-20 | Korea | S2 |
22 | CL32 | Korea | S1 |
23 | CB01185-27 | Korea | S1 |
24 | Collected from China | China | S3 |
25 | Collected from China | China | S3 |
26 | Collected from China | China | S3 |
27 | Collected from China | China | S2 |
28 | Collected from China | China | S1 |
29 | Collected from China | China | S1 |
30 | Collected from China | China | S2 |
31 | Collected from China | China | S3 |
32 | CL2-32 | Korea | S2 |
33 | CL105-84 | Korea | S1 |
34 | CL15-106 | Korea | S1 |
35 | CL31-83 | Korea | Admixture |
36 | CL37-4 | Korea | Admixture |
37 | CL42-17 | Korea | S1 |
38 | CL123-575 | Korea | S2 |
39 | B0148-10 | Korea | S1 |
40 | CL54-36 | Korea | S1 |
41 | CL54-82 | Korea | Admixture |
42 | CL58-83 | Korea | S2 |
43 | CL47-157 | Korea | S2 |
44 | CL57-157 | Korea | S1 |
45 | CB01191-53 | Korea | S2 |
46 | CL60-1 | Korea | S1 |
47 | CL70-21 | Korea | S2 |
48 | CL70-177 | Korea | S2 |
49 | CL81-30 | Korea | S1 |
50 | CB01193-23 | Korea | Admixture |
51 | CB01128-120 | Korea | Admixture |
52 | CB01188-333 | Korea | S1 |
53 | Yuseong2(S)60Co32kr-3 | Korea | S1 |
54 | CL3-21 | Korea | S2 |
55 | CL31-15 | Korea | S3 |
56 | CL32-13 | Korea | S2 |
57 | CB04329-114 | Korea | S1 |
58 | CB04329-13 | Korea | S1 |
59 | 99148-10 | Korea | S2 |
60 | C0148-94 | Korea | S1 |
61 | D0148-72 | Korea | S2 |
62 | B0148-43 | Korea | S1 |
63 | B0148-78 | Korea | S1 |
64 | Y0148-2 | Korea | S3 |
65 | CL129-45 | Korea | S1 |
66 | CB00146-176 | Korea | S3 |
67 | CB00148-46 | Korea | S1 |
68 | CB01200-162 | Korea | S1 |
69 | CB00153-8 | Korea | S1 |
70 | CL137-65 | Korea | S2 |
71 | CB00156-101 | Korea | S3 |
72 | CB00159-140 | Korea | S1 |
73 | CB00171-1 | Korea | S2 |
74 | CB00169-37 | Korea | S1 |
75 | CB00169-109 | Korea | S1 |
76 | CL138-92 | Korea | S2 |
77 | CB00171-189 | Korea | S2 |
78 | CB00169-324 | Korea | S1 |
79 | CL129-16 | Korea | S2 |
80 | CB00164-206 | Korea | S1 |
81 | CB00130-345 | Korea | S1 |
82 | CL137-65 | Korea | S2 |
83 | CL137-39 | Korea | S1 |
84 | Collected from Mongolia | Mongolia | S3 |
85 | Landrace1 (Chengyang) | Korea | Admixture |
86 | Landrace2 (Chengyang) | Korea | S2 |
87 | Landrace3 (Kongju) | Korea | S2 |
88 | Landrace4 (Kongju) | Korea | S2 |
89 | Landrace5 (Boryeong) | Korea | S2 |
90 | Landrace6 (Wando) | Korea | S2 |
91 | Landrace7 (Munkyeong) | Korea | S2 |
92 | Landrace8 (Munkyeong) | Korea | S2 |
93 | Landrace9 (Sancheong) | Korea | S2 |
94 | Landrace10 (Sancheong) | Korea | S3 |
95 | Landrace11 (Yeongcheon) | Korea | S2 |
96 | Landrace12 (Yeongcheon) | Korea | S2 |
97 | Landrace13 (Geochang) | Korea | S2 |
98 | Landrace14 (Goseong) | Korea | S2 |
99 | Landrace15 (Pyeongchang) | Korea | S2 |
100 | Landrace17 (Pyeongchang) | Korea | S2 |
101 | Collected from China | China | S1 |
102 | Collected from China | China | S3 |
103 | CB01191-53 | Korea | S2 |
104 | CB01191-36 | Korea | S1 |
105 | CB01204-287 | Korea | S1 |
106 | CB01210-12 | Korea | S1 |
107 | CB01208-228 | Korea | S2 |
108 | Collected from China | China | S3 |
109 | Collected from China | China | S3 |
110 | CB02214-11 | Korea | S1 |
111 | Collected from China | China | S3 |
112 | Collected from China | China | S3 |
113 | CB03282-102 | Korea | S1 |
114 | CB02214-111 | Korea | S1 |
115 | CB02214-131 | Korea | S1 |
116 | CB01185-20 | Korea | S1 |
117 | CB03286-89 | Korea | Admixture |
118 | CB03289-172 | Korea | S2 |
119 | CBP03310-250 | Korea | S1 |
120 | Cheongyang8 | Korea | S1 |
121 | Cheongyang9 | Korea | S3 |
122 | CBP03302-5 | Korea | S1 |
123 | 99797 | Korea | Admixture |
124 | 99892 | Korea | S1 |
125 | Cheongyang13 | Korea | S1 |
126 | Cheongyang14 | Korea | S1 |
127 | CBP05400-2 | Korea | Admixture |
128 | CBP05400-4 | Korea | S1 |
129 | Hwaboon | Korea | S1 |
130 | 99148-10 | Korea | S2 |
131 | 99412-1 | Korea | S2 |
132 | B0148-43 | Korea | S1 |
133 | B0148-78 | Korea | S1 |
134 | D0148-62 | Korea | S2 |
135 | D0148-72 | Korea | S2 |
136 | C0148-74 | Korea | S1 |
137 | C0148-120 | Korea | S1 |
138 | Y0148-2 | Korea | S3 |
139 | Y0148-24 | Korea | S3 |
a As defined by the program STRUCTURE.
2.2 SSR genotyping
A set of 18 highly polymorphic microsatellite markers enriched using a modified biotin–streptavidin capture method as described earlier [19] was used for the present study (Table 1). A three-primer system [21] including a universal M13 oligonucleotide (TGTAAAACGACGGCCAGT) labeled with one of the fluorescent dyes (6-FAM, NED, or HEX) was used, which allows PCR products to be triplexed during electrophoresis. A special forward primer composed by the concatenation of the M13 oligonucleotide and the specific forward primer was used with the normal reverse primer for SSR PCR amplification. Primer sequences and PCR amplification conditions for each set of primers have been described previously [19]. SSR alleles were resolved on an ABI PRISM 3100 DNA sequencer (Applied Biosystems, Foster City, CA, USA) using GENESCAN 3.7 software and were sized precisely using GeneScan 500 ROX (6-carbon-X-rhodamine) molecular size standards (35–500 bp) with GENOTYPER 3.7 software (Applied Biosystems).
2.3 Data analysis
The number of alleles, gene diversity (GD), heterozygosity (H), and polymorphism information content (PIC) per locus as well as the genetic distance were calculated with the PowerMarker 3.25 program [22]. The unweighted pair group method with an arithmetic mean (UPGMA) tree from shared allele frequencies was constructed using the MEGA 4.0 program [23], which is embedded in PowerMarker.
The possible population was analyzed using the Structure 2.2 model-based program [20] with a burn-in of 10,000, a run length of 150,000, and a model allowing for an admixture and correlated allele frequencies. Five runs of Structure were performed by setting the number of populations (K) from 1 to 12, and an average likelihood value, L(K), was calculated for each K across all runs. The model choice criterion to detect the most probable value of K was ΔK, which is an ad hoc quantity related to the second order change of the log probability of data with respect to the number of clusters inferred by Structure [24].
The molecular variance for model-based subgroups, FST, and the correlation of alleles within subpopulations were calculated using an analysis of molecular variance (AMOVA) approach in the Arlequin 3.11 program [25].
3 Results
3.1 SSR polymorphism
The 18 SSR markers revealed 108 alleles among the 139 L. chinense accessions representing the four countries (Table 1). The SSR loci diversity data are summarized in Table 2. The allelic richness per locus varied widely among the markers, ranging from two (GB-LCM-029; GB-LCM-111; GB-LCM-119; GB-LCM-199) to 17 (GB-LCM-022) alleles (average, six alleles). The frequency of major alleles per locus varied from 0.254 (GB-LCM-167) to 0.959 (GB-LCM-092). The allelic frequency database showed that rare alleles (frequency < 0.05) comprised 63.9% of all alleles, whereas intermediate (frequency of 0.05–0.50) and abundant alleles (frequency > 0.50) comprised 23.1 and 13.0% of all detected alleles, respectively. These results indicated the presence of a relatively large proportion of rare alleles, and most alleles were at a low frequency among the L. chinense accessions studied (Fig. 1). The high frequency of rare alleles (36.3%) among L. chinense accessions (especially among Korean accessions) indicates that they make a greater contribution to the overall genetic diversity of the collection. Hence, it is important to include rare alleles to maximize the genetic variation in the gene bank collections and to utilize them for breeding. The values for heterozygosity ranged from 0.00 at GB-LCM-037 to 1.00 at GB-LCM-025 with an average of 0.439. The average gene diversity and PIC values were 0.3792 and 0.3296, with a range from 0.0793 (GB-LCM-092) to 0.8023 (GB-LCM-167) and from 0.0775 (GB-LCM-092) to 0.7734 (GB-LCM-167), respectively.
Total number of alleles and the genetic diversity index for 18 simple sequence repeat (SSR) loci in the 139 Lycium chinense accessions.
Locus | GeneBank accession | Primers | NG | NA | MAF | NRa | GD | H | PIC |
GB-LCM-004 | FJ487889 | F: ACATTTTGAATCTCCCCGT | 4 | 4 | 0.801 | 2 | 0.3307 | 0.3971 | 0.2960 |
R: GGGAATCAAGATCAATAGTCA | |||||||||
GB-LCM-022 | FJ487891 | F: AAGACAGCACGCCAAAAA | 21 | 17 | 0.788 | 15 | 0.3716 | 0.2793 | 0.3629 |
R: TGTATGATCCCTAAGTCCCG | |||||||||
GB-LCM-025 | FJ487892 | F: TGGATGGTCTATGCATGTTG | 2 | 3 | 0.500 | 1 | 0.5142 | 1.0000 | 0.3962 |
R: AGCCACCCCCAACTAAAA | |||||||||
GB-LCM-029 | FJ487893 | F: CTGCTTAAACGATTGCCG | 2 | 2 | 0.939 | 0 | 0.1148 | 0.1223 | 0.1082 |
R: CAAGCCACCAAACCTTCA | |||||||||
GB-LCM-037 | FJ487894 | F: GTGTGTGGGGTCTGAGC | 3 | 3 | 0.563 | 1 | 0.4954 | 0.0074 | 0.3763 |
R: GAAAGAGCCCAATGCAAA | |||||||||
GB-LCM-044 | FJ487895 | F: TCTCCTTCGGACCCATTT | 8 | 7 | 0.817 | 5 | 0.3111 | 0.1655 | 0.2816 |
R: CAAAGTCACAACGTCGCA | |||||||||
GB-LCM-075 | FJ487896 | F: CTCCTGAATACCCTGGGC | 19 | 16 | 0.597 | 13 | 0.5632 | 0.6855 | 0.5048 |
R: TTGGCATAAGGTGCTCGT | |||||||||
GB-LCM-087 | FJ487897 | F: TTATCGTTGATGGTGGGG | 7 | 7 | 0.903 | 6 | 0.1818 | 0.1799 | 0.1769 |
R: AGAAGAAGCAGCAGCACG | |||||||||
GB-LCM-092 | FJ487898 | F: TTTGGAATGAAACGACGG | 5 | 3 | 0.959 | 2 | 0.0793 | 0.0410 | 0.0775 |
R: GGATCCACAGATTCATCACC | |||||||||
GB-LCM-104 | FJ487899 | F: GCCAAAAGAAGGAATGGG | 3 | 3 | 0.814 | 1 | 0.3056 | 0.3723 | 0.2631 |
R: ACACCCCCGAGACTTAGC | |||||||||
GB-LCM-111 | FJ487900 | F: AATGTACATCGCCCCCA | 2 | 2 | 0.888 | 0 | 0.1982 | 0.2230 | 0.1785 |
R: CGAGCTAAATCTCGAGGG | |||||||||
GB-LCM-119 | FJ487901 | F: GATTCAGGCCGAATGAGA | 2 | 2 | 0.511 | 0 | 0.4998 | 0.9784 | 0.3749 |
R: GATTCGGAGCCTGCTTTT | |||||||||
GB-LCM-120 | FJ487902 | F: CGTGACTAGTGCCCGAAC | 6 | 7 | 0.928 | 6 | 0.1366 | 0.1367 | 0.1331 |
R: CACATGGCGTATGGACAA | |||||||||
GB-LCM-145 | FJ487903 | F: CCTGAGAGCTGATGTGGC | 4 | 3 | 0.547 | 1 | 0.5190 | 0.8898 | 0.4100 |
R: TGTATGATCCCACTCGCC | |||||||||
GB-LCM-166 | FJ487904 | F: CTTGAAGATGGAGGAAAGCA | 6 | 4 | 0.489 | 1 | 0.5569 | 0.9474 | 0.4580 |
R: AGGAGGAGAAGGGGGAAG | |||||||||
GB-LCM-167 | FJ487905 | F: CCATTTGCACCACAAAGG | 28 | 15 | 0.254 | 11 | 0.8023 | 0.8551 | 0.7734 |
R: CCCAAAATTAAAGGGGCA | |||||||||
GB-LCM-199 | FJ487907 | F: GATGTTGGTCTTGGGCTG | 2 | 2 | 0.885 | 0 | 0.2037 | 0.2302 | 0.1830 |
R: TAAGGGCCCTCTTCAACG | |||||||||
GB-LCM-217 | FJ487908 | F: CTGCTTAAACGATTGCCG | 14 | 8 | 0.470 | 4 | 0.6418 | 0.3985 | 0.5785 |
R: GAGCAAGCGCAACACTTT | |||||||||
Total | 138 | 108 | 69 | ||||||
Mean | 7.7 | 6 | 3.8 | 0.3792 | 0.4394 | 0.3296 |
a Alleles with a frequency less than 5%.
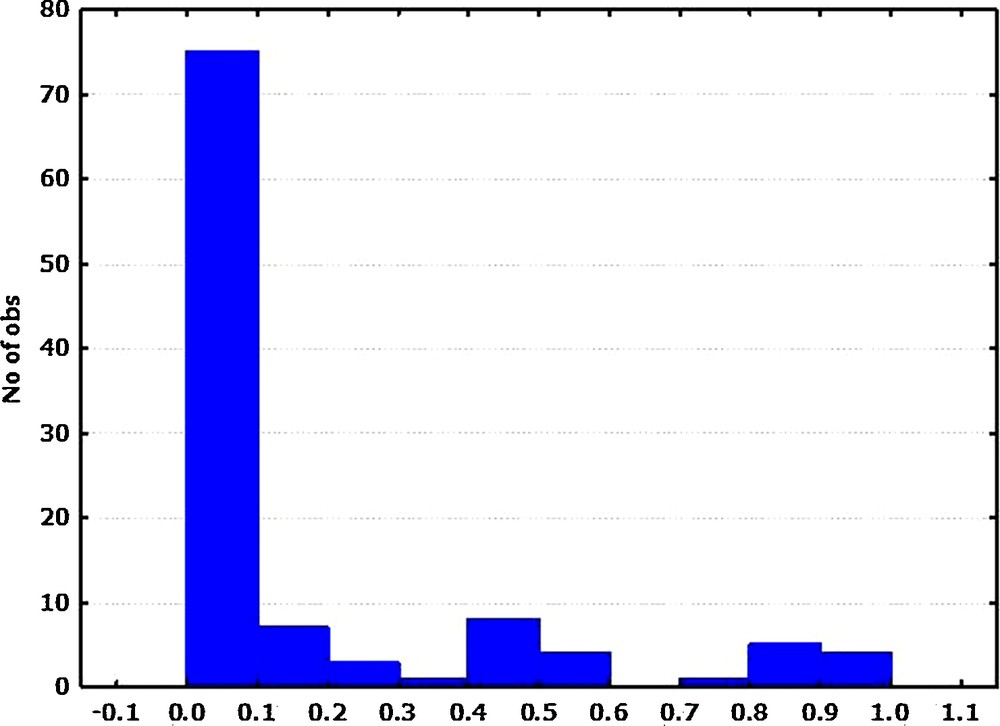
Allele frequency histograms for the 108 alleles in the 139 Lycium chinense accessions.
3.2 Population structure analysis
Effective conservation and management strategies for L. chinense accessions require a fundamental understanding of their population structure. The model-based clustering method was performed using all 139 accessions and 18 SSR markers [20]. At this level, individual proportions of membership in each group, estimated using the multi-allele data set, suggested the existence of some population structure. Estimated likelihood values for a given K in five independent runs yielded consistent results, but the distribution of L(K) did not show a clear mode for the true K (Fig. 2) due to expected behaviour when factors such as inbreeding and departures from Hardy–Weinberg equilibrium are present [26]. These factors could lead to an overestimation of the number of K populations. Thus, another ad hoc quantity (ΔK) was used to overcome the difficulty of interpreting the real K values [24]. ΔK was developed and tested under different simulation routines in which real population structure was present. ΔK showed a clear peak at the true value of K. In this study, the highest value of ΔK for the 139 accessions was K = 3 (Fig. 3), which was consistent with clustering based on the genetic distance (Fig. 4), so we choose a value of K = 3 for the final analysis. The relatively small value of the alpha parameter (α = 0.099) indicates that most accessions originated from one primary ancestor, with a few admixed individuals [26]. As shown in Fig. 4, most of the accessions were clearly classified into one of the three subpopulations (S1–S3) including 65, 51, and 23 L. chinense accessions, respectively (Table 3). S1 consisted of 65 accessions, originating from three different countries but predominantly from Korea (60) and China (4). S2, with 51 accessions, consisted predominantly of Korean accessions (49), whereas the remaining accessions were from China (2). S3 consisted of 23 accessions, predominantly from China (11) and Korea (11) (Table 1). In addition to the accessions that were clearly assigned to a single population, i.e., greater than 70% of their inferred ancestry was derived from one of the model-based populations, 10 accessions (8.2%) in the sample were categorized with admixed ancestry (Fig. 4).
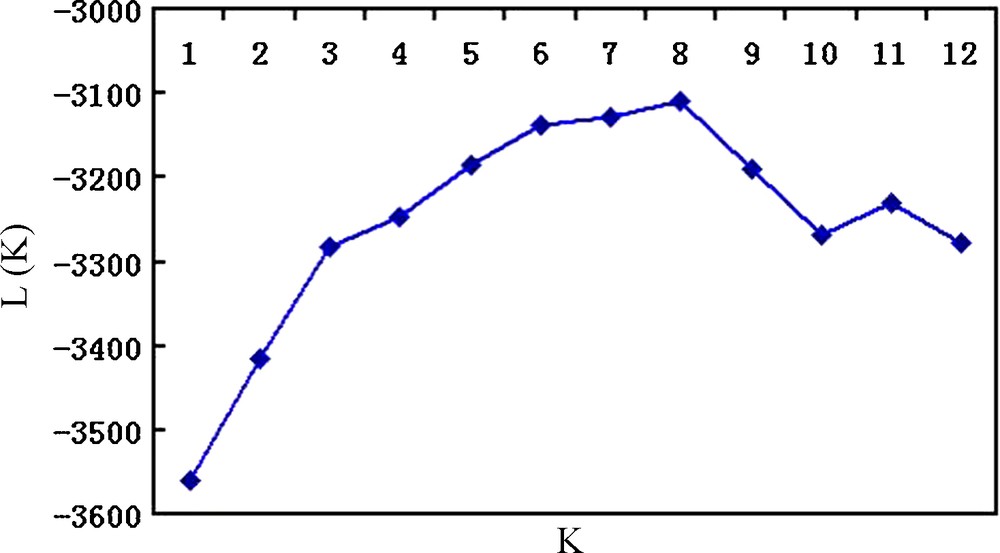
(Log) Likelihood of the data (n = 139), L(K), as a function of K (the number of groups used to stratify the sample). For each K value, five independent runs (blue diamonds) were considered and data were averaged over the replicates.
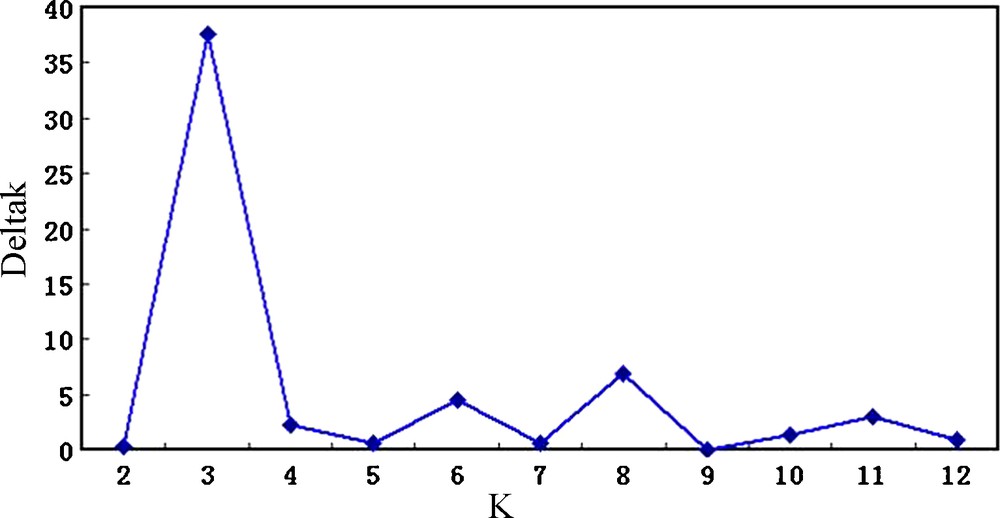
Values of ΔK, with its modal value detecting a true K of the three groups (K = 3).
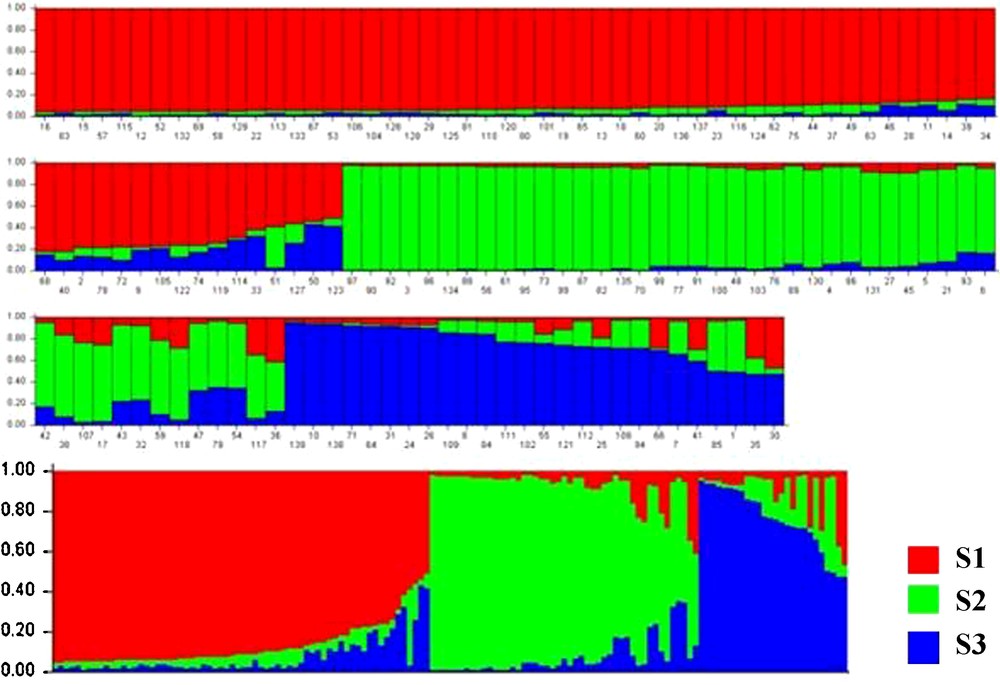
Model-based ancestry for each of the 139 accessions based on the 18 simple sequence repeat (SSR) markers used to build the Q matrix.
Comparisons among model-based populations with regard to average genetic diversity and population differentiation.
Inferred group | Diversity | F ST f | ||||||
n a | NAb | GDc | Hd | PICe | 1 | 2 | Overall | |
1 | 65 | 3.4 | 0.3350 | 0.4321 | 0.2902 | – | – | – |
2 | 51 | 3.4 | 0.3107 | 0.4152 | 0.2679 | 0.2616 | – | – |
3 | 23 | 4.7 | 0.4863 | 0.5222 | 0.4276 | 0.0849 | 0.1050 | – |
Overall | 139 | 6.0 | 0.3792 | 0.4394 | 0.3296 | – | – | 0.1178 |
a The number of accession.
b Average number of allele.
c Gene diversity.
d Heterozygosity.
e Polymorphic information content.
f For AMOVA-based estimates, P < 0.005 for 100 permutations for all population comparisons.
4 Genetic diversity and differentiation in model-based populations
The amount and organization of genetic diversity differed (Table 3). Among the three model-based populations, the S3 subgroup contained a higher allelic richness and an average of 4.7 alleles per locus, while S1 and S2 had the same alleles. S3 also had the highest genetic diversity and PIC (gene diversity = 0.4863; PIC = 0.4276), followed by S1
The overall AMOVA analysis revealed that 15.3% of the variation was due to differences among subpopulations, and the remaining 84.7% was due to differences within subpopulations. Pairwise estimates of FST indicated a different degree of differentiation among the three model-based populations, with values ranging from 0.0849 (between S1 and S3) to 0.2616 (between S1 and S2) (Table 3). The overall FST value was 0.1178, indicating moderate differentiation among the three groups.
5 Discussion
Traditional Chinese medicine has been used for thousands of years in China. Authentication of Chinese medicinal materials is an old but important issue. L. chinense is a key medicinal plant; pharmacological studies have demonstrated that it has a large variety of beneficial effects, such as reducing blood glucose and serum lipids, anti-aging, immunomodulating, anticancer, and anti-fatigue activities, and improvements in male fertility [8,27,28], but it is difficult to distinguish among the species using traditional morphological and histological analyses. Cheng et al. [13] investigated L. barbarum sold on the Taiwan market using RAPD analysis, and only two RAPD fingerprinting types were outlined, revealing low genetic diversity among the samples. Zhang et al. [14] developed the RAPD technique to distinguish L. barbarum from related Lycium species. Sze et al. [17] applied the SCAR marker to authenticate L. barbarum and its adulterants. Nevertheless, SSRs have become one of the most widely used molecular markers for various plant studies in recent years. In this study, we identified the genetic diversity and population structure of L. chinense accessions. The SSR loci newly developed by our group [19] were polymorphic and detected an average of 6.0 alleles per locus, with an average PIC value of 0.3296. The major allele frequency distribution was analyzed at each locus (Table 2). A high proportion of rare alleles might be of adaptive significance, so the capture and preservation of rare alleles and genotypes is an important objective of any conservation strategy [10]. The correlation analysis revealed that allelic richness was significantly and positively associated with the PIC value (r = 0.54, P < 0.05).
The SSRs revealed considerable genetic diversity in the 139 accessions with diverse origins (Fig. 5); the similarity coefficient levels ranged from 0.4287 to 1.0000, with an average value of 0.7614. The high level of genetic variation observed in this study among the different accessions revealed by SSRs reflected a high level of polymorphism at the DNA level.
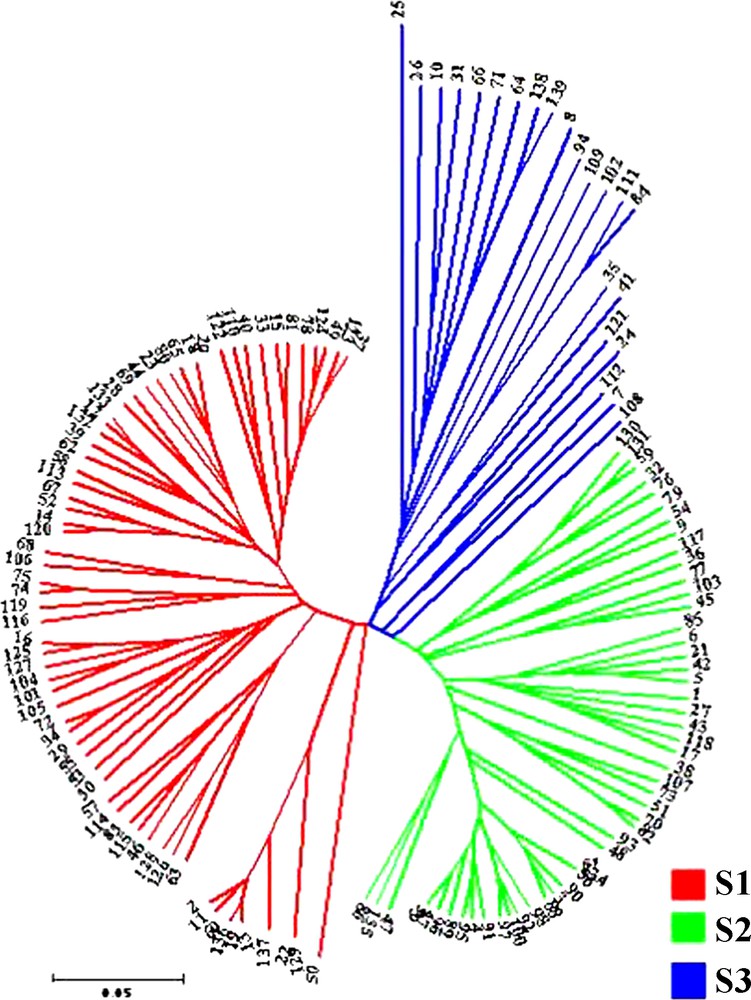
Unrooted neighbor-joining tree based on a Nei's genetic distance matrix among 139 accessions. The colors correspond to the model-based populations.
The Structure program implements a model-based clustering method for inferring population structure using genotype data consisting of unlinked markers (Pritchard et al. [20]). The model does not assume a particular mutation process, and in most cases, the estimated log probability of the data does not provide a correct estimate of the number of clusters, K [24]. We observed in our simulations that as the real K is reached, L(K) continues to increase slightly at larger Ks plateaus, and the variance between runs increases (Fig. 2). The distribution of L(K) did not show a clear mode for the true K, but we found that ΔK did show a clear peak at the true value of K [24] (Fig. 3).
The model-based structure analysis used here revealed the presence of three populations (S1–S3). When clustering based on genetic distance and structure analyses based on the model were compared, similar patterns of accession groupings were discovered (Figs. 3 and 4). The degree of admixture, alpha (α = 0.0999), was inferred from the data. When alpha is close to zero, most individuals are essentially from one population or another, whereas when alpha is greater than one, most individuals are admixed [24]. The distribution of the 139 accessions, which shared at least 70% ancestry within one of the three inferred groups, is summarized in Table 1. In addition to the groups identified by this analysis, 8.2% of accessions showed evidence of mixed population ancestry. The mixture is likely the result of breeding, domestication history, and resource exchange, which have had large effects on diversity structure. The independent population histories of the groups have also shaped the gene pools. Because genetic variability is present in breeding programs, human-mediated gene flow may exist within a population due to breeding, resulting in a large amount of variation attributed to differences within groups (84.7%) rather than among the three inferred groups. A moderate differentiation existed among the three groups. The genetic diversity in each model-based population was also measured (Table 3). Within the subpopulation had lower allele number than among the population, but S3 had the highest genetic diversity and PIC.
Assessing genetic diversity and population structure is an essential component of germplasm characterization and conservation. The results derived from genetic diversity analyses could be used for designing effective breeding programs aimed at broadening the genetic bases of accessions.
Conflict of interest statement
We declare that we have no financial and personal relationships with other people or organizations that can inappropriately influence our work, there is no professional or other personal interest of any nature or kind in any product, service and/or company that could be construed as influencing the position presented in, or the review of, the manuscript entitled, “Molecular genetic diversity and population structure in Lycium accessions using SSR markers”.
Acknowledgements
This study was supported by the agenda project (#200901OFT072045008) from the Rural Development Administration (RDA), the Republic of Korea.