1 Introduction
Artificial selection in domestic species has been based for centuries on the own phenotypes of animals. During the 20th century, selection index theory first, then Best Linear Unbiased Prediction (BLUP–a more sophisticated approach relying on mixed linear models) allowed the use of information on phenotypes of relatives to predict “breeding values” of candidates for selection. This led to the successful selection of easily recorded phenotypic traits with moderate or high heritability. But to be efficient for traits difficult to measure or with low heritability required costly phenotyping investments. During the last 25 years, a number of Quantitative Trait Loci (i.e. regions of the genome responsible for a fraction of the genetic variance of a trait) have been mapped with genetic markers, paving the way to marker-assisted selection (MAS). A genetic marker is a polymorphic sequence, usually without biological effect, but easy to genotype, and, consequently, widely used in genetic studies. The MAS approach was successful for traits with a simple genetic determinism, but provided disappointing results in many more complex situations. The two main reasons for this low efficiency were the limited and always overestimated part of the genetic variance explained by these small numbers of QTL, and also the low association (or linkage disequilibrium) between markers and QTL at the population level. In 2001, Meuwissen et al. [1] proposed a novel approach where the breeding value could be estimated from markers spanning the entire genome. With this approach, genetic effects are estimated for each marker and then summed up to predict the overall breeding value of any animal (Fig. 1). This estimation of marker effects is carried out within a reference population, i.e. a large group of individuals with both phenotypes and marker genotypes information. These effects are then applied to candidates for selection with marker genotype information, but without known phenotypes. To be effective, this approach is very demanding in terms of both number of individuals genotyped and number of markers on the genome. Its application was unfeasible until the development of large-scale and cheap genotyping methods.
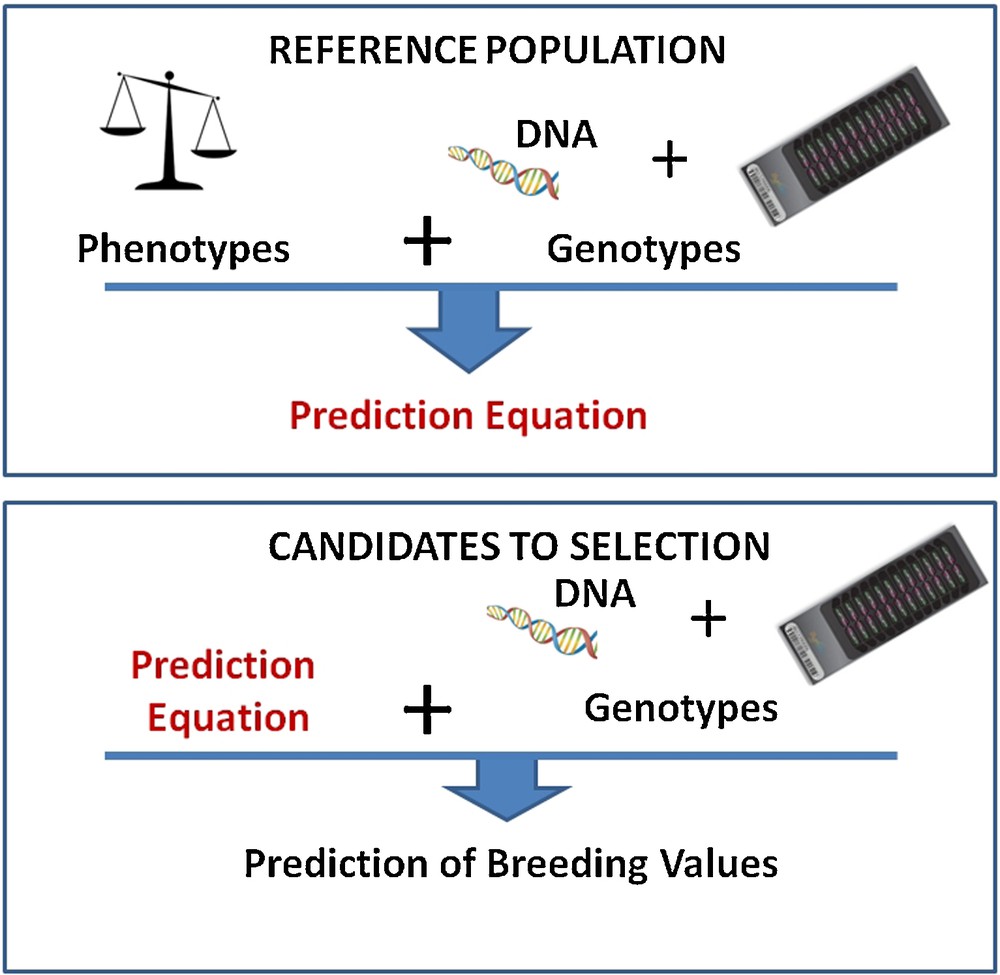
Principles of genomic selection. Top: a prediction equation is obtained from a reference population with phenotypes and genotypes; bottom: this prediction equation is used on candidates with genotypic information only.
2 The success story of genomic selection in dairy cattle
Before the genomic era, genetic improvement of dairy cattle was relying on a vast phenotype recording system distributed over most French farms. The best bulls were selected after a lengthy progeny test based on the performances of more than 100 daughters spread in many herds. They were used through artificial insemination to breed the next generation. Each bull was genetically evaluated on about 40 traits relative to milk production and composition, resistance to mastitis (i.e. udder infection), fertility, conformation, calving conditions, longevity, etc. In 2007, just after the first draft of the bovine genome was assembled, the Illumina Company together with an international consortium developed a chip to genotype over 54,000 single nucleotide polymorphisms (SNP) simultaneously. These markers represented only a small proportion of all discovered SNP, but they were highly polymorphic in a large range of breeds and evenly spaced over the genome. This chip was immediately used to genotype existing progeny-tested bulls. With these first reference populations, genomic breeding values were accurate enough to replace progeny testing. They were made official in 2009 in different countries, allowing the dissemination of semen of young bulls with genomic evaluation only. This revolutionized selection: progeny testing was no longer necessary, simplifying the selection process and decreasing its cost; due to a strong reduction in generation interval, the yearly genetic trend could be doubled; due to their lower production cost, a much larger number of bulls could be selected and marketed, leading to a better management of genetic resources, limiting inbreeding trends [2], and more easily satisfying a diversity of needs and objectives; selection for more balanced and sustainable objectives was easier, including low heritability traits such as fertility or mastitis resistance. Because good accuracy of breeding values requires large reference populations, international collaborations started between breeding cooperatives, leading to the emergence of large consortia such as Eurogenomics in Holstein (nine European countries). Then, in order to decrease the genotyping costs, a low-density chip was designed, with very good imputation accuracy [3], i.e. with excellent prediction of missing markers. A virtuous circle was created: the large number of genotyped animals decreased the cost of genotyping, leading to an increase in volume. At the farm level, this tool is now used to optimize within herd selection, matings and replacement, as each genotyped female is as accurately evaluated as artificial insemination males. In December 2015, the French national database included 400,000 genotyped animals, including 100,000 for 2015 alone. Now, this large number of genotyped cows is the major resource for population reference replacement and updating. In small dairy breeds or in beef breeds with fewer artificial insemination bulls, such reference populations partly consisting of cows with own performances are the only way to implement genomic selection. In 2016, 12 French cattle breeds, including several small ones, use genomic selection in their breeding program. This is a crucial evolution, because initially only the largest breeds were able to benefit from this innovation, creating a technological gap with the smaller ones.
3 Factors of variation of genomic selection efficiency
Whatever the domestic species, the yearly genetic gain depends on four parameters: genetic variability of the trait, selection intensity, evaluation accuracy, and generation interval. The three latter can be modified by genomic selection. The main advantage of genomic selection is that candidates can be evaluated and therefore selected without their own phenotypic information, nor on their progeny. Therefore, selection can be applied very early, just after birth or even on embryos. Therefore, in a number of species and production systems, genomic selection may rely on a reduced generation interval. When the genotyping cost is low, a large number of candidates can be screened, and selection intensity can be increased. This large-scale screening also allows a better use of the available genetic resources. The evaluation can be carried out for any trait recorded in the reference population, which is of particular interest when the trait is difficult or impossible to record on the candidate itself (sex-limited traits, meat quality traits, disease resistance…).
The third determinant of selection efficiency, genomic evaluation accuracy (r) depends, for a given trait (1) on the accuracy of SNP effect estimation and (2) on the linkage disequilibrium between SNP and causal variants. The first parameter depends on the size (N) of the reference population and on the heritability of the trait (h2). The second parameter depends on the structure of the genome and the genetic architecture of the trait. A critical parameter is the number of independent segments segregating in the population. This number q is a function of the length of the genome (length = L, in Morgans) and of the effective size of the population Ne. When Ne is smaller, the conserved segments are longer and fewer markers are needed to trace them. In practice, a formula is commonly used to evaluate this accuracy [4]: r2 = N h2/(N h2 + q), with q a function of Ne and L.
4 Methods of evaluation
Different methods have been proposed to perform genomic evaluation. Because of the large number of markers, all of them consider the marker's effects as a random one whose value comes from a prior statistical distribution that differs depending on the method. Conceptually, the statistical models either include the effect of the markers explicitly or directly describe the genomic breeding values of all genotyped animals, with a covariance structure based on marker information. These two kinds of model are fully equivalent but, according to the situations, one or the other can be more convenient to implement or to interpret. Genomic Best Linear Unbiased Prediction (or GBLUP), proposed by [5], is a simple extension of the polygenic BLUP where the relationship matrix is based on marker information instead of on pedigree. In GBLUP, all markers have the same weight: the model ignores the true genetic determinism of the trait and the covariance between the genomic breeding values of two animals is proportional to their proportion of genome they share. GBLUP is especially efficient for very polygenic traits. Other evaluation methods aim at selecting the most predictive markers, supposedly located close to the causal variants. Many Bayesian methods have been proposed, which give larger weights to SNP potentially close to causal variants or assume that only a small proportion of the variants have a non-zero effect. In other words, they try to better account for QTL information (these methods are also very efficient for multi-marker QTL mapping [6]).
In most approaches, evaluation methods treat each SNP individually, ignoring their linkage disequilibrium. Haplotypes (defined by combinations of neighboring SNP) are more informative than biallelic SNPs and better reflect identity-by-descent situations. In the approach used in the French dairy cattle, the model includes several thousand QTL traced by trait-dependent SNP haplotypes, next to several thousands of SNPs quantifying the remaining residual polygenic term in a way comparable to GBLUP. This comprehensive model is at least as accurate as and more robust than the other approaches, at the expense of a higher complexity [7]. It also anticipates future evolutions with causal variants: when fully known, a causal variant can easily replace the haplotype used as its proxy.
5 Genomic selection 2.0
After the first pioneering work in dairy cattle, genomic selection is becoming a reality in an increasing number of animal and plant populations and species. Nevertheless, it is still a very recent innovation and many evolutions are expected in the near future.
5.1 Extension to many populations
In most species, the cost of genomic selection still limits its extension. Many breeding schemes cannot afford the investment to create a reference population, and genotyping costs can still hamper practical implementation: a critical parameter is the ratio of the genotyping over phenotyping costs. In very prolific species, genotyping with a very low-density chip is economically justified: when all parents in the selection nucleus are genotyped at a high density, only a few hundred markers are needed to trace all the chromosome segments in the selection candidates (their progeny), reducing the overall genotyping costs. This example shows that the dairy cattle situation cannot be simply transposed. Genomic selection must be adapted to the biological and economic conditions.
5.2 Robust methods using biological information and causal variants
It has been observed that genomic selection efficiency is strongly dependent on the relationship between the selection candidates and the reference population. For a given trait, if the genetic relationships at causal polymorphisms were known, selection efficiency would be maximal. In practice, only a proxy based on genetic markers is used. This proxy is more accurate when relationships are higher, and becomes very poor for almost unrelated individuals. In addition, the marker effects estimated in the reference population reflect the marker–QTL association in this population. When the number of generations separating this population from selection candidates increases, recombination events accumulate between the QTL and their surrounding markers, leading to a loss in efficiency. Therefore, it is anticipated that genomic evaluation would be more robust to lack of close relationships if causal variants or very close markers would be used.
For the exact same reason, present genomic prediction methods are not efficient for across-breed selection. A prediction equation built in one breed has nearly no predictive ability in another breed [8], except when breeds are closely related. This was initially interpreted as a too low marker density leading to an observed QTL-markers linkage disequilibrium in the reference population, which was not conserved in the candidates. But using a much higher density (with a HD chip with one marker every 4 kb or 0.004 cM) did not improve much the situation. It was then shown through simulations in cattle that the across-breed genomic relationship coefficient at causal variants can be well approximated only using very close markers (in the same kilobase interval), whereas the other more distant markers on the genome generate noise and must be left out [9]. Of course, the efficiency will depend on the proportion of QTL segregating in different populations and on the stability of their effects. These points are still open questions under investigation. However, across-breed selection might be the most appealing for small breeds to assemble reference populations that are large enough.
After an initial black box strategy, it is believed now that genomic evaluation can be more accurate and more persistent by integrating biological knowledge. Different teams launched large-scale QTL mapping programs by association analysis at the complete sequence level to identify a large number of candidate variants, either causal or in a very close neighborhood of the causal variants, even for QTL explaining as low as 1% of the genetic variance. Reference populations assembled for genomic selection, with tens of thousands individuals, are used as mapping resource populations. Mapping resolution is further improved by combining results from different breeds, because linkage disequilibrium decays must faster across breeds than within a breed. The full sequences of these large resource populations are not directly available, but can be accurately imputed. This was the primary motivation of the “1000 bull genomes” international project [10], with 1682 whole genome sequences already available in July 2015. In this project, the sequenced bulls were primarily selected as the most influential ancestors of their breeds to maximize imputation accuracy.
Assuming many causal variants can be identified, they can be included in genomic selection in a straightforward way. The chips used for genomic selection allow for a custom part designed by the users, which includes these candidate variants. Used on a large scale in commercial populations, this chip helps confirm the effect of these candidate variants and integrate them in the genomic prediction model.
5.3 New phenotypes and new breeding goals
Another major expected evolution is the greater flexibility in the choice of traits to select and of breeding objectives. With genomic selection, a trait can be selected as soon as a reference population of sufficient size can be assembled. In cattle, different opportunities are arising [11], based on the use of innovative recording techniques (such as mid infrared spectrometry for milk composition and milk properties), use of precision farming data (for health, reproduction, behavior…), use of commercial data (sanitary cards, carcass traits from slaughterhouses), or international collaboration for traits expensive to measure (e.g., feed efficiency or methane emission). Many initiatives are under way to generate reference populations for traits that were long believed to be impossible to select.
6 Conclusion
Genomic selection has been very successful in cattle because it provides more genetic gain at a similar or lower cost. But other important and often overlooked consequences are the huge opportunities it offers for traits difficult to select, for traits not yet selected, but important for sustainable production, and for a better management of the genetic variability on the long term. Genomic selection is a very recent innovation. Strong evolutions have started, including reduction in genotyping costs, phenotyping strategies for new traits, approaches for the creation or the replacement of reference populations, increase in robustness and persistency of genomic predictions using causal mutations identified from genome sequences, or genomic prediction of genetic × environment interactions.
Disclosure of interest
The authors declare that they have no competing interest.