1 Introduction
The adaptive and the innate immunity are the two parts of the mammalian immune system. The adaptive immune system is based on molecules corresponding to MHC antigens, T-cell receptors, B-cell receptors and antibodies. However, the innate immunity provides the first line of defence against infection [1] and constitutes a set of disease-resistance mechanisms that are not specific to a particular pathogen but that include cellular and molecular components that recognize classes of molecules peculiar to frequently encountered pathogens [2]. Among these components, Toll-like receptors (TLRs) are a major group of proteins that recognize molecular components of infectious agents, known as pathogen associated molecular patterns (PAMPs) [2]. To date, 13 TLRs have been described in mammals, although it has been shown that not all species contain this full component of receptors [3]. The structure of these genes is similar and characterized by the presence of an ectodomain, a signal transmembrane segment and a highly conserved cytoplasmic domain homologous to the human interleukin-1 receptor (IL1R) and human IL-18 receptor (IL-18R) and designated TIR domain [4,5]. In mammals, TIR domains are involved in mediating interactions in the Toll-like receptor and interleukin-1 signalling pathways [6]. Among TLR genes, TLR2 is located on the outer membrane and forms a dimer complex with TLR1 or TLR6 to recognize peptidoglycans, lipoproteins or lipoteichoic acid of Gram-positive bacteria [7,8]. This gene is widely expressed across species and recognises the greatest number of PAMPs, detecting components from bacteria, viruses and fungi [9,10]. TLR2 was suggested to exhibit high levels of polymorphism in several mammal species [11–13].
Evolutionary patterns of genes of the innate immune system are still under intense debate. The classical view considers this polymorphism of the evolutionary ancient TLR genes to be strongly optimized by natural selection and, therefore, should evolve under purifying selection [14]. Indeed, several point mutations affecting TLR genes were suggested to alter the immune response [15] or to increase susceptibility to infection in sheep [16] and in humans [17,18]. However, recent studies [19,20] have suggested that TLR genes involved in pathogen recognition are evolving in direct response to pathogen-mediated selective pressures. Evidences of adaptive substitutions were observed in bovine TLR2 and TLR5 [12,21], in TLR4 in primates and birds [22,23], and in TLR2 in birds [19] and in sheep [13].
Hares from Tunisia are found across the whole country along a steep ecological gradient ranging from a Mediterranean humid climate in the north down to a Saharan climate in the south. Population genetic data on these hares that were based on nuclear and mitochondrial DNA markers (microsatellites, transferrin intron sequences, mtCR1 sequences) indicated relatively high levels of gene flow and high genetic diversity [24,25]. However, variability of the adaptive immunity MHC class II genes showed more spatial partitioning than the supposedly neutral microsatellite markers, parallel to strong positive selection on these immune genes [26]. Moreover, the observed pattern of positive selection followed climatic variation across the country suggesting occurrence of different pathogen pressures in the different ecoregions. In this study, we examined the level of genetic diversity of the TIR domain of the TLR2 gene of the innate immune system in hares from three regions in Tunisia with two very different climates (NT–North Tunisia, with Mediterranean climate, and ST–South Tunisia, with arid Saharan climate) and one region between these two regions (CT–Central Tunisia, a transition zone with semi-arid climate). We aimed to investigate the level of genetic diversity within and among populations and to compare diversity patterns of TIR2 sequences to the earlier results from other markers [24–26]. In addition, we intended to test whether the observed pattern of diversity has been shaped by neutral or selective processes and if climatic differences may affect the occurrence of protein variants. We looked also for evidence of positive and purifying selection at single codons of the analysed sequences. Finally, the tertiary structure of TIR2 encoded proteins was predicted using computational program and homology modelling methods.
2 Material and methods
2.1 Samples
A total of 110 hares were collected by hunters at fifteen locations in Tunisia across a distance of less than 500 km between the northern Mediterranean seaboard with Mediterranean climate and high annual rainfall (ca. 916 mm) and the arid northern parts of the Saharan desert with less than 100 mm annual rainfall. Localities and sample size of these specimens are shown in Fig. 1 along with assignment of localities to the three regions NT–North Tunisia, CT–Central Tunisia, ST–South Tunisia. Those three regions were operationally considered three populations.

Sampling regions of hares from North, Central and South Tunisia. Sample sizes appear in parentheses. Hares were grouped into three populations according to climatic, geographic and phenotypic data. The northern population with samples from six regions (FER: Fernana; JEN: Jendouba; TAB: Tabarka; BEJ: Béja, STH: Sidi Thabet and KLB: Kélibia); the central population with samples from six regions (NAD: Nadhour; WES: Weslatia; KAL: Kalâa; BKL: Bekalta; CHE: Cherarda; SND: Sned); and the southern population with samples from three regions (DOU: Douz; TAT: Tataouine, and BGD: Ben Guerdène).
2.2 DNA amplification and typing via next-generation sequencing
Protocols used for DNA extraction are described in previous publications [25,27,28]. We targeted a 372 bp fragment of Toll like receptor 2 corresponding to the Toll-interleukin-1 receptor domain protein (TIR2) in a total of 110 hare specimens. Briefly, library preparation was performed by firstly amplifying each sample using the primer pair 5′-ATGCGTTCGTGTCCTACAGC-3′ and TLR-R 5′-CTCAAGTTCCCCCAGAACC-3′. A second round of PCRs was carried out to attach unique DNA barcodes to all samples and achieve compatibility with Illumina's MiSeq flow cell. PCR products were then purified, and after the quality and quantity of PCR products were estimated, all samples were pooled and sent to the Microsynth (AG) for sequencing on an Illumina Miseq using 2 × 250 bp chemistry.
Initial TIR2 sequence data processing was achieved as outlined in Biedrzycka et al. [29] and Sebastien et al. [30] using the different amplicon sequencing analysis tools available at: http://www.evobiolab.biol.amu.edu.pl/amplisat/.
2.3 Analysis of polymorphism and genetic differentiation
DNA polymorphism within populations (haplotype diversity h, nucleotide diversity π, and mean number of pairwise differences k) was estimated using DNASP v. 5 [31]. The Tajima's D [32], also implemented in the same program, was used to test the hypothesis that sequence variation of the TIR2 domain does not differ from neutral expectations. A test of deviation from Hardy–Weinberg equilibrium was calculated using GENEPOP 4.0 [33] separately for each population. The GENETIX program v. 4.05 [34] was used to calculate allele frequencies, observed (HO) and expected heterozygosity (HE). The FSTAT program, version 2.9.3 [35] was used to calculate population-specific values of allelic richness (Rs) based on a rarefaction approach to account for different sample sizes.
Population differentiation was determined by calculation of standardized pairwise FST (10,000 permutations) in GENETIX.
A Median-Joining network [36] was constructed in order to model the phylogenetic relationships among the observed haplotypes and their distribution among the three populations. The network was rooted by one outgroup haplotype of European rabbits (Oryctolagus cuniculus) to obtain an indication of its evolutionary direction.
2.4 Selection analyses
Different approaches were used to test whether positive selection historically operated on the TIR2 sequences. First, we used CODEML [37] to test for site-specific positive selection. Different codon-based models of selection exist in PAML, which generally produce equivalent results although some tests are suggested to be more conservative than others [38]. To increase the likelihood of detection of positive selection, we used the less conservative M7/M8 test to examine the extent of selection acting on the TIR2 domain. The null model M7 (beta) was compared to M8 (beta plus omega). The comparison was performed using the likelihood ratio test (LRT): twice the log-likelihood difference was compared with a χ2 distribution with degrees of freedom equal to the difference in the number of parameters between both models. Significant amino acids sites under positive selection were considered using the Bayes Empirical Bayes (BEB) approach with posterior probability at 95% cut-off [39].
In a second approach, the OmegaMap program [40] based on a Bayesian population genetics approximation to the coalescent theory, was used as proposed by Smith et al. [41]. It generates means and credible intervals for the selection parameter (dN/dS = ω) and recombination rate (ρ = 4 N r) for each codon, respectively (N and r represent the effective population size and the per codon rate of recombination). Two Markov chain Monte Carlo runs of 250,000 iterations (25,000 iteration burn-in) on population allele frequencies at each locus were compared for convergence. Codons are considered as positively selected with posterior probabilities greater than 95%.
Finally, we tested for codon-specific signatures of positive and negative selection across the TIR2 sequences using the DATAMONKEY webserver (http://www.datamonkey.org/; last accessed 15th August 2017) [42]. We first identified recombination break points with genetic algorithm recombination detection (GARD) [43]. The output was then used to run four different maximum likelihood methods for detection of selection: SLAC (single likelihood ancestral counting), FEL (fixed effects likelihood), REL (random effects likelihood), and Mixed Effects Model of Evolution (MEME) [44]. Significance levels of P < 0.25 in SLAC and FEL and P < 0.05 in MEME and Bayes factors > 50 in REL were considered as indicating positively selected sites. We considered a codon to be positively selected only if it was identified by at least two of the methods [42].
In addition to the applied tests, and in order to infer molecular signatures of contemporary selection, we used the model-based approach of Beaumont and Nichols [45], based on TIR2 genotypes, implemented in Lositan [46] to compare the observed FST values estimated at each locus to a null distribution of FST conditional on heterozygosity. The TIR2 sequences as well as the genotypes of fourteen microsatellite loci studied earlier in these hares in the same laboratory [24] were tested for neutrality under 50,000 simulations, estimated neutral mean FST, an infinite alleles mutation model, a 99% confidence interval and a false discovery rate of 0.1%.
2.5 Testing for climate effects on occurrence of TIR2 protein variants
Given that TIR2 protein variant A was the most frequent in all three populations and all other variants were by far less frequent, we focused on examining whether the presence of this variant either in a homozygous or heterozygous genotype may be affected by climate independent of geographic position of the collected hares. We used the WORLDCLIM data set for 2.5-min intervals (Version 1.4, http://www.worldclim.org/bioclim.htm) to extract mean climate data for all sampling locations and used the following climate variables Bio 1, 5, 6, 8, 9, 10, 11, 12, 13, 14, 15, 16, 17, 18, 19 to run a principal-component analysis (PCA). The non-rotated PCA was based on a variance-covariance matrix of ln-transformed original values with eigen values greater than 1.0 times the mean eigen value. The individual scores of each of the two extracted factors (i.e. climate factor 1 and climate factor 2; see § “Results”) were then used in our logistic model as independent variables together with geographical latitude and longitude. The major problems for the modelling, however, were that latitude and individual scores for climate factor 1were highly correlated (r = +0.938), meaning that one variable could be almost fully substituted by the other. Therefore, we run two separate models, one with latitude, longitude, and climate factor 2 scores, and one with climate factor 1 scores, longitude, and climate factor 2 scores. According to information theory [47], the model with the lowest AICc value should be chosen for argumentation and statistical conclusion.
The models were run in R [48] and their syntaxes were as follows:
Gm = glm(tlrprotA ∼ long + lat + Fac2, data = dat, family = binomial(link = “logit”))
Gm = glm(tlrprotA ∼ long + Fac1 + Fac2, data = dat, family = binomial(link = “logit”))where “tlrprotA” is the protein variant A being present either in a homozygous or heterozygous genotype, or absent, “long” is geographical longitude, “lat” is geographical latitude, “Fac1”, and “Fac2” are the climate factors 1 and 2 as obtained from the PCA.
2.6 Protein structure analysis and in silico prediction of mutation effects
Fold recognition of TIR2 was performed with HHPRED (https://www.toolkit.tuebingen.mpg.de/#/tools/hhpred, last accessed 15 August 2017) [49]. The crystal structure of the human Toll like Receptor 2 TIR signalling domain (PDB: 1FYX_A; 2.8 Å resolution) was identified as the best template for residues 14–134 (HHPred E-value = 2.6 × 10−17). The tertiary structure prediction was generated using MODELLER (https://www.toolkit.tuebingen.mpg.de/#/tools/modeller, last accessed 15 August 2017) [50] based on the above-mentioned template. Amino acid residues were mapped onto this structure, and a PyMOL script file was generated for visualization using PyMOL (version 1.8) (http://www.pymol.org) [51].
Finally, to assess the functional effect of all amino acid substitutions, the Protein Variation Effect Analyser (PROVEAN) [52] was used. The default confidence threshold of −2.5 was used to determine if an amino acid replacement is likely to influence the protein function. The most frequent allele sequence was used as a template, and every fixed amino acid replacement per sequence was used as a query.
3 Results and discussion
This is the first study presenting a partial sequence analysis of TLR2 gene of a hare species (Lepus capensis). Several studies addressing genetic variability of TLRs of mammals have shown different levels of diversity [13,19,38,53]. Moreover, genetic diversity was clearly different between the LRR molecules, the transmembrane, and the TIR domains with the last region being very conservative. The analysis of genetic variability of TLR3 in 80 wild and domestic rabbit lineages [53] revealed 41 single nucleotide polymorphisms (SNPs) of which fourteen were non-synonymous. Among these non-synonymous mutations, eleven were detected in the LRR molecules and only 1 in the TIR region. Smith et al. [13] have detected 18 polymorphic sites in ten sheep breeds with two of them localized in the TIR domain. Here, we analysed genetic diversity and selection of the TIR domain corresponding to positions 1999 to 2370 of the total TLR2 gene of the rabbit O. cuniculus (GenBank accession No.: NM_001082781). We detected 25 alleles (GenBank accession numbers MH493864-MH493888) based on 20 variable sites of which seven were non-synonymous in the 372 TIR2 sequence fragments studied (Fig. 2). The observed diversity is summarized through the population genetic parameters reported in Table 1. Overall haplotype diversity was 0.733, whereas overall nucleotide diversity was 0.00399 and mean number of pairwise differences was 1.483. The values calculated separately for the three populations indicated that genetic diversity was lower in the southern population. These results were further confirmed by the geographical distribution of the 25 detected alleles. The numbers of alleles per population were 19, 14, and 4 in NT, CT and ST, respectively (Appendix A). In general, in all three populations, allele 2 was highly frequent, whereas most other alleles were detected at very low frequencies (Appendix A). A geographical comparison of the obtained genotypes revealed many alleles specific to single populations (“private alleles”). Only four alleles were shared between the three populations (Appendix A), whereas six alleles were specific to NT, twelve alleles to CT and no allele to ST. Finally, allelic richness (Rs), which measure the number of alleles independent of sample size, was high and similar in NT (12.13) and CT (12.47) and low in ST (4.00) (Appendix A).
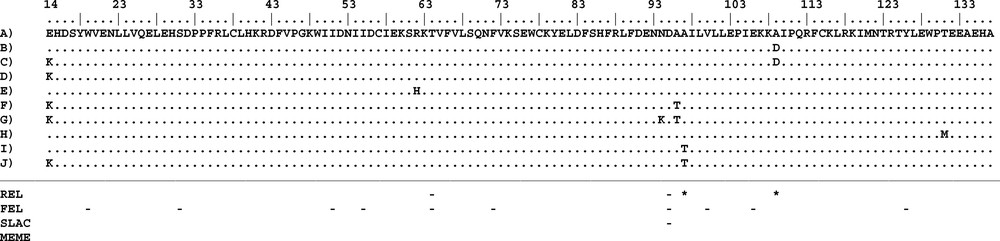
Amino acid sequence alignment of TIR2 haplotypes. A to J are the names of the different proteins detected in the TIR2 gene. Positively and negatively selected codons identified by each method are indicated with “*” and “–”, respectively. Numbering of amino acid positions is based on the full gene sequence in O. cuniculus (NM_001082781).
TIR2 diversity estimates in the three Tunisian hare populations: N, number of sequences; S, number of segregating sites; H, number of alleles; π, nucleotide diversity; Hd, haplotype diversity; K, mean number of pairwise differences; D, Tajima's D statistic test; Rs, allelic richness; HO, observed heterozygosities; HE, expected heterozygosities.
N | S | H | π | Hd | k | D | Rs | H E | H O | |
NT | 50 | 12 | 14 | 0.00603 | 0.695 | 2.24163 | −0.48291 | 12.126 | 0.6808 | 0.6400 |
CT | 130 | 16 | 19 | 0.00714 | 0.789 | 2.65760 | −0.40866 | 12.471 | 0.7833 | 0.7846 |
ST | 40 | 4 | 4 | 0.00356 | 0.547 | 1.32436 | 0.97479 | 4.000 | 0.5338 | 0.6000 |
ALL | 220 | 20 | 25 | 0.00399 | 0.733 | 1.48281 | −1.54535 | – | – | – |
The high genetic diversity of the presently studied gene segment is congruent with results of other genetic markers [24,25] confirming the general tendency of hare populations in Tunisia to display high values of genetic diversity. This might indicate a population growth from bottlenecked ancestral populations [24,25] or/and ancient and recent gene flow from neighbouring regions not sampled in the studies carried out until now [24,27]. In addition, the high diversity of the immune genes studied including the current marker might be influenced by the strong climate and habitat variation. However, in line with the studied MHC loci that were found to be under strong positive selection, but unlike the neutral microsatellite markers, the genetic diversity (i.e. allelic richness) of the currently studied TLR2 locus sequences was relatively low in the ST with its arid climate. Notably, the currently found genetic diversity values across the three populations were similar between the three regions as calculated from mtCR1 [24] and transferrin [25] sequences. The currently reduced diversity in the ST population might suggest that the diversity of the functionally important TIR2 domain is more affected than other markers studied until now by genetic drift. Indeed, Knaffer et al. [54] showed that TLR diversity was affected to a greater extent by contemporary bottlenecks than MHC and microsatellite loci in saddleback (Philesturnus carunculatus) populations. The currently observed loss of genetic diversity towards the southern arid region might be also explained by natural selection. As observed for MHC genes [26], rare allele advantage–as a form of balancing selection–might be proposed to be an important determinant of TIR2 variability in the studied hare populations. Actually, we observed a high number of rare and private alleles; more specifically, 17 rare alleles (68%) were detected and were found solely as heterozygous alleles, as expected in the context of balancing selection [55]. However, the Tajima's D test did not reject the null hypothesis that all studied populations are evolving under neutrality (Appendix A). Moreover, only the NT population deviated from Hardy Weinberg expectations (Appendix A).
Pairwise FST values ranged between −0.001 (NT-ST) and 0.037 (CT-ST) (Appendix A). This low differentiation was also confirmed by the median network of the obtained haplotypes (Fig. 3) that indicated little phylogenetic divergence and an absence of geographically meaningful phylogroups. This finding is in accordance with earlier results based on partial transferrin sequences [25] and genotypes of fourteen microsatellite loci [27]. As most common alleles are generally common across the studied regions, we do not see any profound reorganization of regional TLR2 gene pools despite the occurrence of a quite remarkable overall number of private alleles at low frequencies. The presence of a high number of unique haplotypes might suggest that evolution in TLR genes are mainly driven by point mutations rather than recombination and gene conversion. Indeed, no recombination was obtained in the currently studied sequences when using GARD.
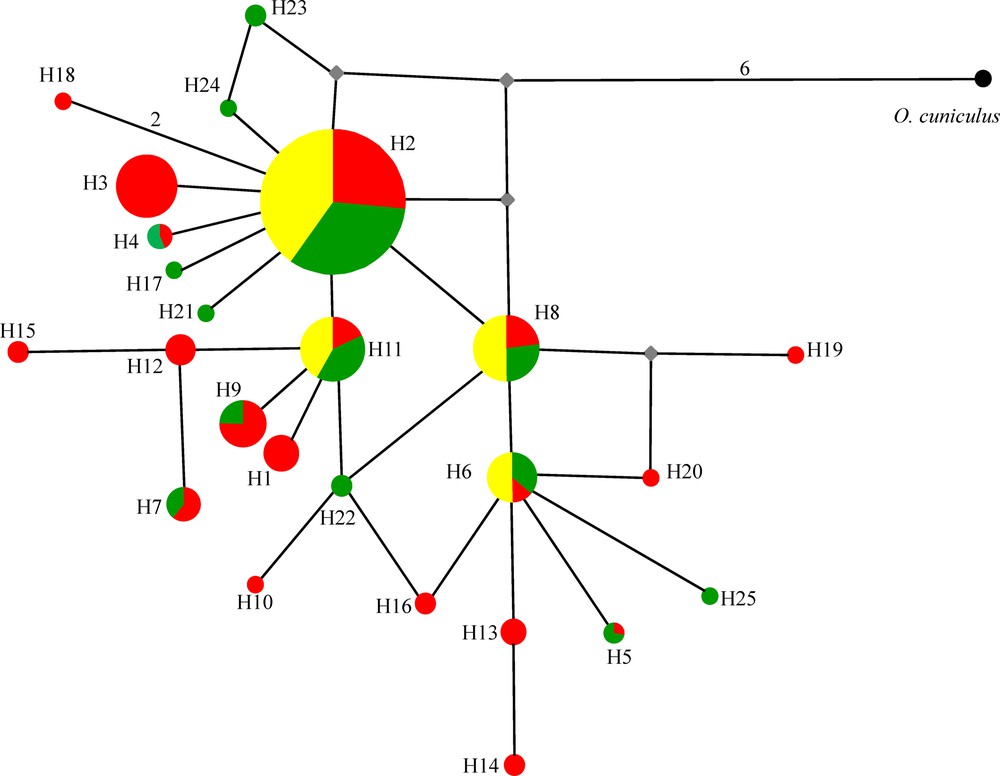
Median-joining network showing the phylogenetic relationships among TIR2 haplotypes. Relative haplotype (= allele) frequencies correspond to haplotype circle size (see Appendix A). Mutation steps are indicated by numbers on lines connecting haplotypes if higher than one. Small grey square indicates inferred haplotype. Green circle/pie segment: northern population, red circle/pie segment: central population, and yellow circle/pie segment: southern population.
The PCA of the chosen climate factors yielded two principal components (factors): the first factor that explained 87.4% of the variance of the climate variables; according to the loadings of the bioclimatic variables into this factor (i.e. the correlation of the individual scores with the transformed values of the climate variables used for the PCA), it could be interpreted as a general precipitation factor. The second factor could be interpreted as a factor of ambient temperature during cold and wet periods of the year. The two logistic models of the most common TIR2 protein variant A that we run to examine whether its occurrence was affected by climatic variation independent of the geographic sample location returned very similar AICc values (model with latitude in: AICc = 46.023 and model with climate factor 1 in: AICc = 47.552). This did not allow preferring one over the other model [47]. For the model with latitude instead of climate factor 1 the values of relative variable importance (RVI) amounted to 0.71 for longitude, 0.63 for latitude, and 0.44 for climate factor 2. Hence, there was only a longitudinal effect, when accepting the RVI threshold value of 0.70 for statistically important variables [47]. For the model with climate factor 1 instead of latitude in the RVI values amounted to 0.71 for longitude, 0.61 for climate factor 2, and 0.30 for climate factor 1. Obviously, whereas there was a significant longitude effect, neither latitude nor any climate factor had a significant effect on the occurrence of the most common TIR2 protein A across the three climatic zones in Tunisia. However, the high explanatory power of latitude in terms of the climate factor 1 did not allow us to investigate the role of the currently used climate variable on the distribution of the TIR2 protein by fully accounting for the pure geographical sample distribution, i.e. potential neutral population genetic causes. A wider geographic sample arrangement, e.g., across larger parts of North Africa, may, however, allow running such a model. It might also confirm the currently identified TIR2 protein variant “A” as a general key protein independent of climatic variation, or contrary yield a significant climatic effect on its distribution. It might also help explain the meaning of the high number of protein variants that we have currently specifically detected in the more humid and less hot climate zone of Tunisia.
Immune genes are expected to be under strong natural selection due to their essential roles in recognizing and eliminating infectious agents. Indeed, a signature of positive selection has been reported for several TLRs genes including TLR2 [1,19,38,56]. However, most of the positions suggested under positive selection were reported for outside of the TIR domains. Areal et al. [19] found that positively selected sites are mainly localized in the LRR domain whereas the TIR domains of several TLR genes contained only few sites or none under positive selection in several mammalian species. Similarly, Smith et al. [13] found nine positively-selected sites that were all positioned within the extracellular domain of the ovine TLR2. According to Ishengoma and Agaba [38] the mapping of positively-selected sites to the three major TLR domains revealed that 92 to 100% sites were located in the extracellular domain of the studied TLR genes. In our study we applied several tests to detect positive and negative selection, but no sites were found to have evolved under positive selection according to PAML, DATAMONKEY, and OmegaMap. For PAML, the null model in CODEML was preferred over the alternative model (P (ΔLRT) > 0.05) in the model comparison between M7 (ln L = −707.836516) vs M8 (ln L = −706.062386) suggesting that the analysed sites were evolving under strong purifying selection or neutrally. In DATAMONKEY, two positions (84 and 96; Fig. 2) were suggested to be under positive selection by REL. However, those signals for the latter two positions were not confirmed by any of the other DATAMONKEY tests (Fig. 2). Therefore, we considered them as false positive signals [42]. Accordingly, the outlier test revealed that the studied locus was evolving neutrally (Fig. 4). However, negative selection was suggested in a total of ten amino acid positions among which only two (51 and 82) were confirmed by more than one of the different DATAMONKEY tests (Fig. 2).
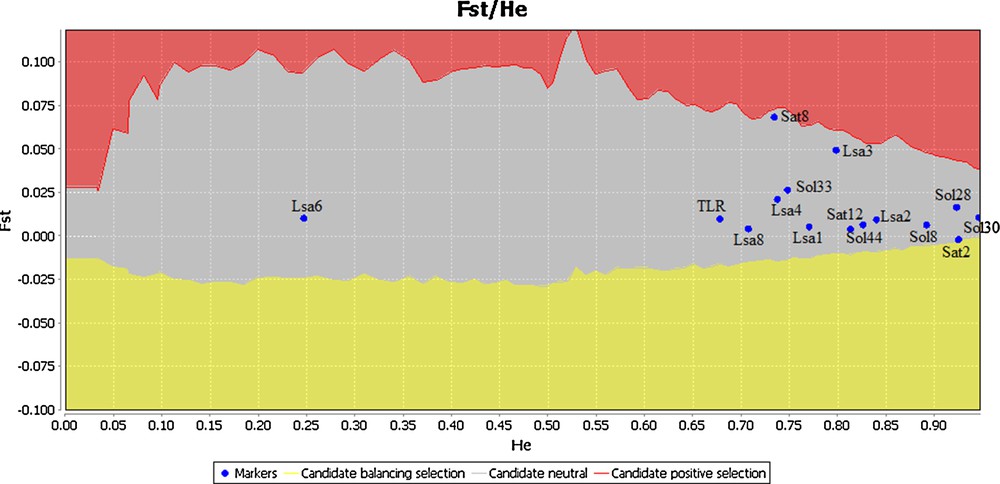
Plot of the outlier tests based on TLR2 genotypes and fourteen microsatellite loci for the three populations. The upper and lower 99% confidence interval boundaries are indicated by solid line.
The TIR domain of the TLRs is characterized by three Box regions (Boxes 1, 2, and 3) which are important in signal transduction; they are highly conserved and should consequently be under strong purifying selection [57,58]. As expected, due to their functional constraints, none of the seven non-synonymous sites were located in these boxes. The mapping of these non-synonymous sites to the protein structure of the TIR domain (Fig. 5) showed that they were distributed along the different loop regions (14 in AA loop; 62 in BC loop; 94, 96, and 97 in CD loop; 109 in DD loop; 131 in EE loop). The functional effects of the loop regions are better known for the BB loop; it has been suggested that non-synonymous mutations in this region inhibit the response to lipopolysaccharide (LPS) as observed in mouse TLR4 [59,60] or to affect signal transduction as observed in sheep TLR2. This functional importance of the loop regions is confirmed in the current study by our PROVEAN analysis that indicated that three substitutions (E13K, A94T and A97T) among the non-synonymous positions have deleterious effects. However, comparisons of TIR domains between different species revealed the occurrence of large insertions or deletions in several loop regions of these domains leading to size variation of the TIR regions among the available sequences [57]. Two hypotheses might be suggested to explain the observed diversity profiles: first, Barreiro et al. [61] suggested that non-viral TLRs have a more flexible evolution and therefore tolerate non-synonymous mutations which, in some circumstances, can be subject to positive selection and become fixed in some populations. This higher tolerance is because the function of non-viral TLRs is more redundant than of viral TLRs. Indeed, several surface TLRs are able to recognize the same bacteria and fungi components. Therefore, a non-synonymous mutation in one TLR does not necessarily mean the extinction of the function and does not compromise immunity [57]; second, TIR domains function through self-association and interaction with other TIR domains (homotypic interactions) to create scaffolds that facilitate the formation of protein complexes. TIR domains have also been shown to interact with proteins that do not contain TIR domains (heterotypic interactions). Such interactions could be an indication of co-evolution and positive selection or fixation of deleterious mutations in either genome can lead to subsequent selection acting on the other.
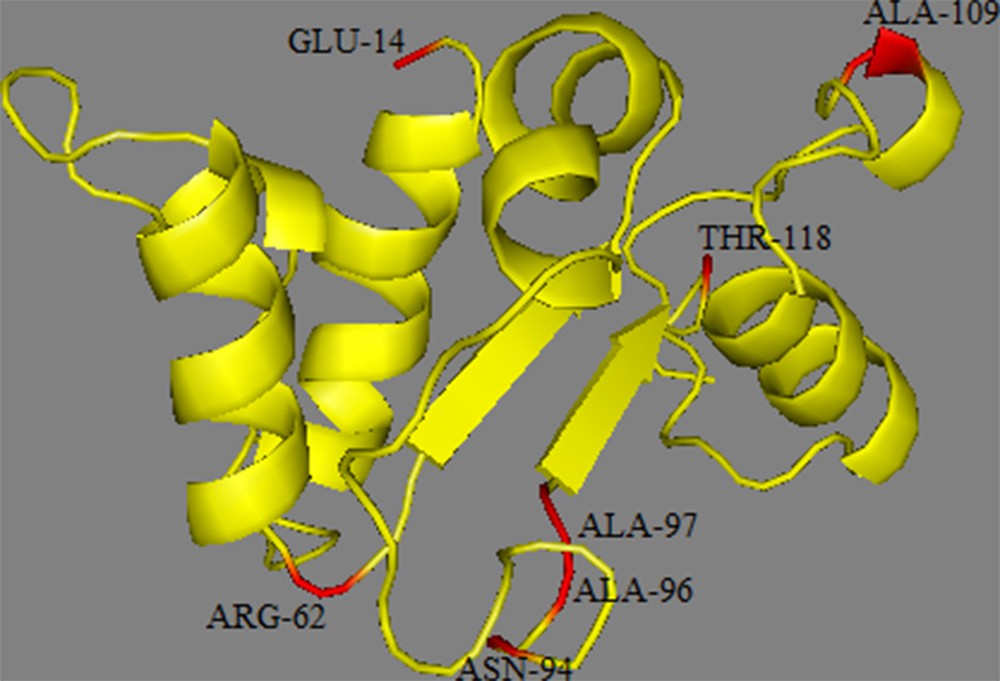
Structural modelling of the TIR domain of TLR2. In our homology model are located the non-synonymous mutations.
4 Conclusions
The currently observed pattern of genetic diversity and genetic differentiation in TIR2 sequences in hare populations from Tunisia might result from a combination of various neutral population genetic processes, such as historical reduction of effective population size, subdivision, and migration, as well as natural selection. However, we are currently unable to disentangle these different effects in the studied populations. Moreover, a combination of such potential evolutionary factors might blur their detection with the different statistical methods. Evidence of purifying selection was found in the analysed sequences conforming to the general evolution pattern of these genes. However, climatic factors as potential surrogates of varying pathogenic landscapes may also affect the distribution of protein variants, but to prove such effects, a wider geographical sampling would be necessary.
Disclosure of interest
The authors declare that they have no competing interest.
Acknowledgements
Laboratory work was done at the Genetics laboratory of the Research Institute of Wildlife Ecology (Vienna, Austria). We wish to express our thanks to Mrs. Anita Haiden (Vienna) for valuable help in the laboratory. Partial financial support was provided by “Wildlife Research–Franz Suchentrunk”.
Appendix A Allele frequencies and HWE test P-value (P) for the three Tunisian hare populations. The sample size is indicated between parentheses for each population. The corresponding protein for each allele is also given. Ten protein variants were detected and were named from A to J (see Fig. 2).
Allele | Protein | NT (25) | CT (65) | ST (20) |
1 | A | 0.0077 | ||
2 | A | 0.5400 | 0.4308 | 0.6500 |
3 | A | 0.1077 | ||
4 | B | 0.0200 | 0.0154 | |
5 | C | 0.0200 | 0.0077 | |
6 | D | 0.0200 | 0.0538 | 0.0750 |
7 | A | 0.0200 | 0.0308 | |
8 | A | 0.0800 | 0.0692 | 0.1500 |
9 | A | 0.0200 | 0.0615 | |
10 | E | 0.0385 | ||
11 | A | 0.1200 | 0.0538 | 0.1250 |
12 | A | 0.0308 | ||
13 | F | 0.0231 | ||
14 | G | 0.0154 | ||
15 | A | 0.0154 | ||
16 | D | 0.0154 | ||
17 | H | 0.0200 | ||
18 | A | 0.0077 | ||
19 | A | 0.0077 | ||
20 | D | 0.0077 | ||
21 | A | 0.0200 | ||
22 | A | 0.0400 | ||
23 | I | 0.0400 | ||
24 | I | 0.0200 | ||
25 | J | 0.0200 | ||
P | 0.0123 | 0.0519 | 0.1849 | |
F ST | NT–CT 0.012 |
NT–ST –0.001 |
CT–ST 0.037** |